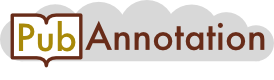
PMC:7333997 / 2571-31813
Annnotations
LitCovid_Glycan-Motif-Structure
Id | Subject | Object | Predicate | Lexical cue |
---|---|---|---|---|
T1 | 8477-8481 | https://glytoucan.org/Structures/Glycans/G56516VH | denotes | g/m3 |
T2 | 8477-8481 | https://glytoucan.org/Structures/Glycans/G91237TK | denotes | g/m3 |
T3 | 8521-8525 | https://glytoucan.org/Structures/Glycans/G56516VH | denotes | g/m3 |
T4 | 8521-8525 | https://glytoucan.org/Structures/Glycans/G91237TK | denotes | g/m3 |
T5 | 8619-8623 | https://glytoucan.org/Structures/Glycans/G56516VH | denotes | g/m3 |
T6 | 8619-8623 | https://glytoucan.org/Structures/Glycans/G91237TK | denotes | g/m3 |
T7 | 8900-8904 | https://glytoucan.org/Structures/Glycans/G56516VH | denotes | g/m3 |
T8 | 8900-8904 | https://glytoucan.org/Structures/Glycans/G91237TK | denotes | g/m3 |
T9 | 8954-8958 | https://glytoucan.org/Structures/Glycans/G56516VH | denotes | g/m3 |
T10 | 8954-8958 | https://glytoucan.org/Structures/Glycans/G91237TK | denotes | g/m3 |
T11 | 9045-9049 | https://glytoucan.org/Structures/Glycans/G56516VH | denotes | g/m3 |
T12 | 9045-9049 | https://glytoucan.org/Structures/Glycans/G91237TK | denotes | g/m3 |
T13 | 10041-10045 | https://glytoucan.org/Structures/Glycans/G56516VH | denotes | g/m3 |
T14 | 10041-10045 | https://glytoucan.org/Structures/Glycans/G91237TK | denotes | g/m3 |
T15 | 15474-15478 | https://glytoucan.org/Structures/Glycans/G56516VH | denotes | g/m3 |
T16 | 15474-15478 | https://glytoucan.org/Structures/Glycans/G91237TK | denotes | g/m3 |
T17 | 15583-15587 | https://glytoucan.org/Structures/Glycans/G56516VH | denotes | g/m3 |
T18 | 15583-15587 | https://glytoucan.org/Structures/Glycans/G91237TK | denotes | g/m3 |
T19 | 15773-15777 | https://glytoucan.org/Structures/Glycans/G56516VH | denotes | g/m3 |
T20 | 15773-15777 | https://glytoucan.org/Structures/Glycans/G91237TK | denotes | g/m3 |
T21 | 15954-15958 | https://glytoucan.org/Structures/Glycans/G56516VH | denotes | g/m3 |
T22 | 15954-15958 | https://glytoucan.org/Structures/Glycans/G91237TK | denotes | g/m3 |
T23 | 16068-16072 | https://glytoucan.org/Structures/Glycans/G56516VH | denotes | g/m3 |
T24 | 16068-16072 | https://glytoucan.org/Structures/Glycans/G91237TK | denotes | g/m3 |
T25 | 16197-16201 | https://glytoucan.org/Structures/Glycans/G56516VH | denotes | g/m3 |
T26 | 16197-16201 | https://glytoucan.org/Structures/Glycans/G91237TK | denotes | g/m3 |
T27 | 16779-16783 | https://glytoucan.org/Structures/Glycans/G56516VH | denotes | g/m3 |
T28 | 16779-16783 | https://glytoucan.org/Structures/Glycans/G91237TK | denotes | g/m3 |
T29 | 17071-17075 | https://glytoucan.org/Structures/Glycans/G56516VH | denotes | g/m3 |
T30 | 17071-17075 | https://glytoucan.org/Structures/Glycans/G91237TK | denotes | g/m3 |
T31 | 17774-17778 | https://glytoucan.org/Structures/Glycans/G56516VH | denotes | g/m3 |
T32 | 17774-17778 | https://glytoucan.org/Structures/Glycans/G91237TK | denotes | g/m3 |
T33 | 18483-18487 | https://glytoucan.org/Structures/Glycans/G56516VH | denotes | g/m3 |
T34 | 18483-18487 | https://glytoucan.org/Structures/Glycans/G91237TK | denotes | g/m3 |
T35 | 18523-18527 | https://glytoucan.org/Structures/Glycans/G56516VH | denotes | g/m3 |
T36 | 18523-18527 | https://glytoucan.org/Structures/Glycans/G91237TK | denotes | g/m3 |
T37 | 18556-18560 | https://glytoucan.org/Structures/Glycans/G56516VH | denotes | g/m3 |
T38 | 18556-18560 | https://glytoucan.org/Structures/Glycans/G91237TK | denotes | g/m3 |
T39 | 18618-18622 | https://glytoucan.org/Structures/Glycans/G56516VH | denotes | g/m3 |
T40 | 18618-18622 | https://glytoucan.org/Structures/Glycans/G91237TK | denotes | g/m3 |
T41 | 19368-19372 | https://glytoucan.org/Structures/Glycans/G56516VH | denotes | g/m3 |
T42 | 19368-19372 | https://glytoucan.org/Structures/Glycans/G91237TK | denotes | g/m3 |
T43 | 23977-23981 | https://glytoucan.org/Structures/Glycans/G56516VH | denotes | g/m3 |
T44 | 23977-23981 | https://glytoucan.org/Structures/Glycans/G91237TK | denotes | g/m3 |
T45 | 25310-25314 | https://glytoucan.org/Structures/Glycans/G56516VH | denotes | g/m3 |
T46 | 25310-25314 | https://glytoucan.org/Structures/Glycans/G91237TK | denotes | g/m3 |
T47 | 27887-27891 | https://glytoucan.org/Structures/Glycans/G56516VH | denotes | g/m3 |
T48 | 27887-27891 | https://glytoucan.org/Structures/Glycans/G91237TK | denotes | g/m3 |
T49 | 27944-27948 | https://glytoucan.org/Structures/Glycans/G56516VH | denotes | g/m3 |
T50 | 27944-27948 | https://glytoucan.org/Structures/Glycans/G91237TK | denotes | g/m3 |
T51 | 27961-27965 | https://glytoucan.org/Structures/Glycans/G56516VH | denotes | g/m3 |
T52 | 27961-27965 | https://glytoucan.org/Structures/Glycans/G91237TK | denotes | g/m3 |
T53 | 27983-27987 | https://glytoucan.org/Structures/Glycans/G56516VH | denotes | g/m3 |
T54 | 27983-27987 | https://glytoucan.org/Structures/Glycans/G91237TK | denotes | g/m3 |
LitCovid-PMC-OGER-BB
Id | Subject | Object | Predicate | Lexical cue |
---|---|---|---|---|
T17 | 406-414 | GO:0051866 | denotes | adaptive |
T18 | 982-987 | NCBITaxon:10239 | denotes | virus |
T19 | 1218-1223 | NCBITaxon:10239 | denotes | virus |
T20 | 1281-1288 | NCBITaxon:10239 | denotes | viruses |
T21 | 1780-1786 | NCBITaxon:9606 | denotes | people |
T22 | 1844-1854 | CHEBI:33893;CHEBI:33893 | denotes | pollutants |
T23 | 1888-1895 | CHEBI:32168;CHEBI:32168 | denotes | sulphur |
T24 | 1896-1902 | CHEBI:25741;CHEBI:25741 | denotes | oxides |
T25 | 1910-1925 | CHEBI:35196;CHEBI:35196 | denotes | nitrogen oxides |
T26 | 1933-1948 | CHEBI:17245;CHEBI:17245 | denotes | carbon monoxide |
T27 | 1958-1972 | CHEBI:16526;CHEBI:16526 | denotes | carbon dioxide |
T28 | 1974-1977 | CHEBI:16526;CHEBI:16526 | denotes | CO2 |
T29 | 2197-2203 | GO:0016265 | denotes | deaths |
T30 | 2303-2309 | NCBITaxon:9606 | denotes | people |
T31 | 2310-2313 | GO:0016265 | denotes | die |
T32 | 2341-2344 | CHEBI:8102;CHEBI:8102 | denotes | PM2 |
T33 | 2388-2406 | UBERON:0001004 | denotes | respiratory system |
T34 | 2432-2436 | UBERON:0002048 | denotes | lung |
T35 | 2485-2489 | UBERON:0002048 | denotes | lung |
T36 | 2518-2527 | UBERON:0002048 | denotes | pulmonary |
T37 | 2537-2542 | UBERON:0000948 | denotes | heart |
T38 | 2555-2566 | UBERON:0001004 | denotes | respiratory |
T39 | 3131-3138 | NCBITaxon:10239 | denotes | viruses |
T40 | 3329-3334 | NCBITaxon:10239 | denotes | virus |
T41 | 3342-3351 | SP_7 | denotes | SARS-CoV2 |
T42 | 3369-3377 | SP_7 | denotes | COVID-19 |
T43 | 3462-3468 | NCBITaxon:9606 | denotes | people |
T44 | 3526-3531 | NCBITaxon:10239 | denotes | virus |
T45 | 4194-4197 | CHEBI:16526;CHEBI:16526 | denotes | CO2 |
T46 | 4373-4379 | GO:0016265 | denotes | deaths |
T47 | 4385-4393 | SP_7 | denotes | COVID-19 |
T48 | 4431-4437 | GO:0016265 | denotes | deaths |
T49 | 4454-4470 | CHEBI:33101;CHEBI:33101 | denotes | nitrogen dioxide |
T50 | 4471-4474 | CHEBI:17997;CHEBI:17997 | denotes | NO2 |
T51 | 4903-4914 | NCBITaxon:11118 | denotes | coronavirus |
T52 | 5019-5027 | GO:0007612 | denotes | learning |
T53 | 5784-5795 | NCBITaxon:11118 | denotes | coronavirus |
T54 | 5838-5844 | GO:0016265 | denotes | deaths |
T55 | 6038-6049 | CHEBI:25212;CHEBI:25212 | denotes | particulate |
T56 | 6050-6060 | CHEBI:33893;CHEBI:33893 | denotes | pollutants |
T57 | 6091-6102 | NCBITaxon:11118 | denotes | coronavirus |
T58 | 6576-6579 | CHEBI:8102;CHEBI:8102 | denotes | PM2 |
T59 | 7131-7139 | SP_7 | denotes | COVID-19 |
T60 | 8361-8369 | SP_7 | denotes | COVID-19 |
T61 | 9175-9183 | SP_7 | denotes | COVID-19 |
T62 | 12804-12812 | SP_7 | denotes | COVID-19 |
T63 | 14331-14334 | CHEBI:8102;CHEBI:8102 | denotes | PM2 |
T64 | 15410-15413 | CHEBI:8102;CHEBI:8102 | denotes | PM2 |
T65 | 15426-15435 | CHEBI:33893;CHEBI:33893 | denotes | pollutant |
T66 | 16908-16911 | CHEBI:8102;CHEBI:8102 | denotes | PM2 |
T67 | 17857-17860 | CHEBI:8102;CHEBI:8102 | denotes | PM2 |
T68 | 17875-17881 | CHEBI:8102;CHEBI:8102 | denotes | matter |
T69 | 18754-18757 | CHEBI:8102;CHEBI:8102 | denotes | PM2 |
T70 | 18942-18948 | UBERON:0002020 | denotes | matter |
T71 | 19504-19508 | CHEBI:33290;CHEBI:33290 | denotes | food |
T72 | 19514-19517 | CHEBI:8102;CHEBI:8102 | denotes | PM2 |
T73 | 20109-20112 | CHEBI:8102;CHEBI:8102 | denotes | PM2 |
T74 | 20216-20223 | GO:0065007 | denotes | control |
T75 | 20325-20328 | CHEBI:8102;CHEBI:8102 | denotes | PM2 |
T76 | 20512-20514 | CL:0002322 | denotes | ES |
T77 | 20534-20536 | CL:0002322 | denotes | ES |
T78 | 20647-20654 | GO:0065007 | denotes | control |
T79 | 20712-20714 | CL:0002322 | denotes | ES |
T80 | 20909-20911 | CL:0002322 | denotes | ES |
T81 | 20976-20978 | CL:0002322 | denotes | ES |
T82 | 21021-21023 | CL:0002322 | denotes | ES |
T83 | 21096-21098 | CL:0002322 | denotes | ES |
T84 | 21142-21144 | CL:0002322 | denotes | ES |
T85 | 21160-21162 | CL:0002322 | denotes | ES |
T86 | 21551-21553 | CL:0002322 | denotes | ES |
T87 | 21569-21571 | CL:0002322 | denotes | ES |
T88 | 21674-21676 | CL:0002322 | denotes | ES |
T89 | 21693-21695 | CL:0002322 | denotes | ES |
T90 | 22187-22189 | CL:0002322 | denotes | ES |
T91 | 22207-22209 | CL:0002322 | denotes | ES |
T92 | 22965-22967 | CL:0002322 | denotes | ES |
T93 | 23292-23296 | UBERON:0000104 | denotes | life |
T94 | 23652-23659 | GO:0065007 | denotes | monitor |
T95 | 23781-23785 | UBERON:0000104 | denotes | life |
T96 | 25273-25276 | CHEBI:8102;CHEBI:8102 | denotes | PM2 |
T97 | 25328-25338 | CHEBI:15854;CHEBI:15854 | denotes | quarantine |
T98 | 25834-25837 | CHEBI:8102;CHEBI:8102 | denotes | PM2 |
T99 | 27741-27744 | CHEBI:8102;CHEBI:8102 | denotes | PM2 |
T100 | 28630-28632 | CL:0002322 | denotes | ES |
T10699 | 27204-27212 | GO:0051866 | denotes | adaptive |
T55010 | 19932-19935 | CHEBI:8102;CHEBI:8102 | denotes | ent |
T70895 | 20039-20047 | GO:0065007 | denotes | in capi |
T71376 | 20147-20150 | CHEBI:8102;CHEBI:8102 | denotes | e i |
T21234 | 20334-20336 | CL:0002322 | denotes | .1 |
T4018 | 20357-20359 | CL:0002322 | denotes | s |
T89422 | 20469-20476 | GO:0065007 | denotes | itants |
T30581 | 20534-20536 | CL:0002322 | denotes | ES |
T57333 | 20732-20734 | CL:0002322 | denotes | er |
T88886 | 20799-20801 | CL:0002322 | denotes | bl |
T83449 | 20844-20846 | CL:0002322 | denotes | el |
T41730 | 20920-20922 | CL:0002322 | denotes | ti |
T40092 | 20965-20967 | CL:0002322 | denotes | 12 |
T88635 | 20983-20985 | CL:0002322 | denotes | . |
T19457 | 21423-21425 | CL:0002322 | denotes | P |
T11760 | 21441-21443 | CL:0002322 | denotes | e, |
T9560 | 21546-21548 | CL:0002322 | denotes | 0 |
T80611 | 21565-21567 | CL:0002322 | denotes | P |
T42912 | 22058-22060 | CL:0002322 | denotes | t |
T33214 | 22078-22080 | CL:0002322 | denotes | a. |
T35384 | 22838-22840 | CL:0002322 | denotes | bo |
T57601 | 23165-23169 | UBERON:0000104 | denotes | nt r |
T27039 | 23525-23532 | GO:0065007 | denotes | greater |
T23617 | 23654-23658 | UBERON:0000104 | denotes | nito |
T49927 | 25192-25195 | CHEBI:8102;CHEBI:8102 | denotes | (1 |
T66344 | 25248-25258 | CHEBI:15854;CHEBI:15854 | denotes | a, to Skop |
T83987 | 25753-25756 | CHEBI:8102;CHEBI:8102 | denotes | ute |
T84902 | 27741-27744 | CHEBI:8102;CHEBI:8102 | denotes | PM2 |
T24115 | 28630-28632 | CL:0002322 | denotes | ES |
LitCovid-PD-FMA-UBERON
Id | Subject | Object | Predicate | Lexical cue | fma_id |
---|---|---|---|---|---|
T3 | 597-601 | Body_part | denotes | face | http://purl.org/sig/ont/fma/fma24728 |
T4 | 2388-2406 | Body_part | denotes | respiratory system | http://purl.org/sig/ont/fma/fma7158 |
T5 | 2432-2436 | Body_part | denotes | lung | http://purl.org/sig/ont/fma/fma7195 |
T6 | 2485-2489 | Body_part | denotes | lung | http://purl.org/sig/ont/fma/fma7195 |
T7 | 2537-2542 | Body_part | denotes | heart | http://purl.org/sig/ont/fma/fma7088 |
T8 | 2671-2675 | Body_part | denotes | hand | http://purl.org/sig/ont/fma/fma9712 |
T9 | 3713-3717 | Body_part | denotes | hand | http://purl.org/sig/ont/fma/fma9712 |
T10 | 9642-9646 | Body_part | denotes | hand | http://purl.org/sig/ont/fma/fma9712 |
T11 | 10018-10025 | Body_part | denotes | Capital | http://purl.org/sig/ont/fma/fma23727 |
T12 | 13881-13885 | Body_part | denotes | hand | http://purl.org/sig/ont/fma/fma9712 |
T13 | 15717-15724 | Body_part | denotes | Capital | http://purl.org/sig/ont/fma/fma23727 |
T14 | 15898-15905 | Body_part | denotes | Capital | http://purl.org/sig/ont/fma/fma23727 |
T15 | 16266-16273 | Body_part | denotes | Capital | http://purl.org/sig/ont/fma/fma23727 |
T16 | 16423-16430 | Body_part | denotes | Capital | http://purl.org/sig/ont/fma/fma23727 |
T17 | 17902-17909 | Body_part | denotes | Capital | http://purl.org/sig/ont/fma/fma23727 |
T18 | 18066-18073 | Body_part | denotes | Capital | http://purl.org/sig/ont/fma/fma23727 |
T19 | 19128-19135 | Body_part | denotes | Capital | http://purl.org/sig/ont/fma/fma23727 |
T20 | 24441-24444 | Body_part | denotes | map | http://purl.org/sig/ont/fma/fma67847 |
LitCovid-PD-UBERON
Id | Subject | Object | Predicate | Lexical cue | uberon_id |
---|---|---|---|---|---|
T3 | 597-601 | Body_part | denotes | face | http://purl.obolibrary.org/obo/UBERON_0001456 |
T4 | 2388-2406 | Body_part | denotes | respiratory system | http://purl.obolibrary.org/obo/UBERON_0001004 |
T5 | 2432-2436 | Body_part | denotes | lung | http://purl.obolibrary.org/obo/UBERON_0002048 |
T6 | 2485-2489 | Body_part | denotes | lung | http://purl.obolibrary.org/obo/UBERON_0002048 |
T7 | 2537-2542 | Body_part | denotes | heart | http://purl.obolibrary.org/obo/UBERON_0000948 |
T8 | 2671-2675 | Body_part | denotes | hand | http://purl.obolibrary.org/obo/UBERON_0002398 |
T9 | 3713-3717 | Body_part | denotes | hand | http://purl.obolibrary.org/obo/UBERON_0002398 |
T10 | 9642-9646 | Body_part | denotes | hand | http://purl.obolibrary.org/obo/UBERON_0002398 |
T11 | 13881-13885 | Body_part | denotes | hand | http://purl.obolibrary.org/obo/UBERON_0002398 |
LitCovid-PD-MONDO
Id | Subject | Object | Predicate | Lexical cue | mondo_id |
---|---|---|---|---|---|
T11 | 1392-1401 | Disease | denotes | influenza | http://purl.obolibrary.org/obo/MONDO_0005812 |
T12 | 2477-2483 | Disease | denotes | stroke | http://purl.obolibrary.org/obo/MONDO_0005098|http://purl.obolibrary.org/obo/MONDO_0011057 |
T14 | 2485-2496 | Disease | denotes | lung cancer | http://purl.obolibrary.org/obo/MONDO_0008903 |
T15 | 2490-2496 | Disease | denotes | cancer | http://purl.obolibrary.org/obo/MONDO_0004992 |
T16 | 2498-2535 | Disease | denotes | chronic obstructive pulmonary disease | http://purl.obolibrary.org/obo/MONDO_0005002 |
T17 | 2518-2535 | Disease | denotes | pulmonary disease | http://purl.obolibrary.org/obo/MONDO_0005275 |
T18 | 2537-2550 | Disease | denotes | heart disease | http://purl.obolibrary.org/obo/MONDO_0005267 |
T19 | 2555-2577 | Disease | denotes | respiratory infections | http://purl.obolibrary.org/obo/MONDO_0024355 |
T20 | 2586-2595 | Disease | denotes | pneumonia | http://purl.obolibrary.org/obo/MONDO_0005249 |
T21 | 3342-3346 | Disease | denotes | SARS | http://purl.obolibrary.org/obo/MONDO_0005091 |
T22 | 3369-3377 | Disease | denotes | COVID-19 | http://purl.obolibrary.org/obo/MONDO_0100096 |
T23 | 4385-4393 | Disease | denotes | COVID-19 | http://purl.obolibrary.org/obo/MONDO_0100096 |
T24 | 7131-7139 | Disease | denotes | COVID-19 | http://purl.obolibrary.org/obo/MONDO_0100096 |
T25 | 8361-8369 | Disease | denotes | COVID-19 | http://purl.obolibrary.org/obo/MONDO_0100096 |
T26 | 9175-9183 | Disease | denotes | COVID-19 | http://purl.obolibrary.org/obo/MONDO_0100096 |
T27 | 11704-11707 | Disease | denotes | EFE | http://purl.obolibrary.org/obo/MONDO_0009169 |
T28 | 12804-12812 | Disease | denotes | COVID-19 | http://purl.obolibrary.org/obo/MONDO_0100096 |
LitCovid-PD-CLO
Id | Subject | Object | Predicate | Lexical cue |
---|---|---|---|---|
T22 | 57-58 | http://purl.obolibrary.org/obo/CLO_0001020 | denotes | a |
T23 | 597-601 | http://purl.obolibrary.org/obo/UBERON_0001456 | denotes | face |
T24 | 833-834 | http://purl.obolibrary.org/obo/CLO_0001020 | denotes | a |
T25 | 929-930 | http://purl.obolibrary.org/obo/CLO_0001020 | denotes | a |
T26 | 980-981 | http://purl.obolibrary.org/obo/CLO_0001020 | denotes | a |
T27 | 982-987 | http://purl.obolibrary.org/obo/NCBITaxon_10239 | denotes | virus |
T28 | 1218-1223 | http://purl.obolibrary.org/obo/NCBITaxon_10239 | denotes | virus |
T29 | 1281-1288 | http://purl.obolibrary.org/obo/NCBITaxon_10239 | denotes | viruses |
T30 | 1328-1329 | http://purl.obolibrary.org/obo/CLO_0001020 | denotes | a |
T31 | 1691-1694 | http://purl.obolibrary.org/obo/CLO_0051582 | denotes | has |
T32 | 2085-2097 | http://purl.obolibrary.org/obo/OBI_0000245 | denotes | Organization |
T33 | 2248-2260 | http://purl.obolibrary.org/obo/OBI_0000245 | denotes | Organization |
T34 | 2262-2266 | http://purl.obolibrary.org/obo/CLO_0001185 | denotes | 2018 |
T35 | 2388-2406 | http://purl.obolibrary.org/obo/UBERON_0001004 | denotes | respiratory system |
T36 | 2432-2436 | http://purl.obolibrary.org/obo/UBERON_0002048 | denotes | lung |
T37 | 2432-2436 | http://www.ebi.ac.uk/efo/EFO_0000934 | denotes | lung |
T38 | 2485-2489 | http://purl.obolibrary.org/obo/UBERON_0002048 | denotes | lung |
T39 | 2485-2489 | http://www.ebi.ac.uk/efo/EFO_0000934 | denotes | lung |
T40 | 2537-2542 | http://purl.obolibrary.org/obo/UBERON_0000948 | denotes | heart |
T41 | 2537-2542 | http://purl.obolibrary.org/obo/UBERON_0007100 | denotes | heart |
T42 | 2537-2542 | http://purl.obolibrary.org/obo/UBERON_0015228 | denotes | heart |
T43 | 2537-2542 | http://www.ebi.ac.uk/efo/EFO_0000815 | denotes | heart |
T44 | 2677-2678 | http://purl.obolibrary.org/obo/CLO_0001020 | denotes | a |
T45 | 2700-2704 | http://purl.obolibrary.org/obo/CLO_0001185 | denotes | 2018 |
T46 | 3061-3065 | http://purl.obolibrary.org/obo/CLO_0001185 | denotes | 2018 |
T47 | 3083-3087 | http://purl.obolibrary.org/obo/CLO_0001185 | denotes | 2018 |
T48 | 3131-3138 | http://purl.obolibrary.org/obo/NCBITaxon_10239 | denotes | viruses |
T49 | 3284-3296 | http://purl.obolibrary.org/obo/OBI_0000245 | denotes | organization |
T50 | 3309-3310 | http://purl.obolibrary.org/obo/CLO_0001020 | denotes | a |
T51 | 3329-3334 | http://purl.obolibrary.org/obo/NCBITaxon_10239 | denotes | virus |
T52 | 3378-3381 | http://purl.obolibrary.org/obo/CLO_0051582 | denotes | has |
T53 | 3397-3405 | http://purl.obolibrary.org/obo/CLO_0001658 | denotes | activity |
T54 | 3409-3410 | http://purl.obolibrary.org/obo/CLO_0001020 | denotes | a |
T55 | 3426-3429 | http://purl.obolibrary.org/obo/CLO_0051582 | denotes | has |
T56 | 3526-3531 | http://purl.obolibrary.org/obo/NCBITaxon_10239 | denotes | virus |
T57 | 3730-3733 | http://purl.obolibrary.org/obo/CLO_0051582 | denotes | has |
T58 | 3827-3830 | http://purl.obolibrary.org/obo/CLO_0051582 | denotes | has |
T59 | 4005-4008 | http://purl.obolibrary.org/obo/CLO_0051582 | denotes | has |
T60 | 4628-4631 | http://purl.obolibrary.org/obo/CLO_0051582 | denotes | has |
T61 | 4840-4841 | http://purl.obolibrary.org/obo/CLO_0001020 | denotes | a |
T62 | 4986-4987 | http://purl.obolibrary.org/obo/CLO_0001020 | denotes | a |
T63 | 5017-5018 | http://purl.obolibrary.org/obo/CLO_0001020 | denotes | a |
T64 | 5272-5274 | http://purl.obolibrary.org/obo/CLO_0001000 | denotes | 35 |
T65 | 5484-5485 | http://purl.obolibrary.org/obo/CLO_0001020 | denotes | a |
T66 | 5702-5705 | http://purl.obolibrary.org/obo/CLO_0051582 | denotes | has |
T67 | 5712-5713 | http://purl.obolibrary.org/obo/CLO_0001020 | denotes | a |
T68 | 5857-5858 | http://purl.obolibrary.org/obo/CLO_0001020 | denotes | a |
T69 | 6068-6069 | http://purl.obolibrary.org/obo/CLO_0001020 | denotes | a |
T70 | 6532-6533 | http://purl.obolibrary.org/obo/CLO_0001020 | denotes | a |
T71 | 6689-6690 | http://purl.obolibrary.org/obo/CLO_0001020 | denotes | a |
T72 | 7869-7875 | http://purl.obolibrary.org/obo/CLO_0001929 | denotes | Berlin |
T73 | 8048-8051 | http://purl.obolibrary.org/obo/CLO_0051582 | denotes | has |
T74 | 8654-8658 | http://purl.obolibrary.org/obo/CLO_0001185 | denotes | 2018 |
T75 | 8909-8913 | http://purl.obolibrary.org/obo/CLO_0001185 | denotes | 2018 |
T76 | 8971-8977 | http://purl.obolibrary.org/obo/CLO_0001929 | denotes | Berlin |
T77 | 9054-9058 | http://purl.obolibrary.org/obo/CLO_0001185 | denotes | 2018 |
T78 | 9447-9448 | http://purl.obolibrary.org/obo/CLO_0001020 | denotes | a |
T79 | 9521-9522 | http://purl.obolibrary.org/obo/CLO_0001020 | denotes | a |
T80 | 9838-9839 | http://purl.obolibrary.org/obo/CLO_0001020 | denotes | a |
T81 | 9921-9922 | http://purl.obolibrary.org/obo/CLO_0001020 | denotes | a |
T82 | 10790-10793 | http://purl.obolibrary.org/obo/CLO_0002742 | denotes | del |
T83 | 10807-10809 | http://purl.obolibrary.org/obo/CLO_0001302 | denotes | 34 |
T84 | 10911-10913 | http://purl.obolibrary.org/obo/CLO_0053733 | denotes | 11 |
T85 | 11462-11468 | http://purl.obolibrary.org/obo/NCBITaxon_9005 | denotes | Turkey |
T86 | 11784-11786 | http://purl.obolibrary.org/obo/CLO_0050509 | denotes | 27 |
T87 | 12030-12032 | http://purl.obolibrary.org/obo/CLO_0050510 | denotes | 18 |
T88 | 12369-12375 | http://purl.obolibrary.org/obo/CLO_0001929 | denotes | Berlin |
T89 | 12638-12639 | http://purl.obolibrary.org/obo/CLO_0001020 | denotes | a |
T90 | 12709-12710 | http://purl.obolibrary.org/obo/CLO_0001020 | denotes | a |
T91 | 12898-12908 | http://purl.obolibrary.org/obo/CLO_0001658 | denotes | activities |
T92 | 13149-13150 | http://purl.obolibrary.org/obo/CLO_0001020 | denotes | a |
T93 | 13917-13919 | http://purl.obolibrary.org/obo/CLO_0053799 | denotes | 45 |
T94 | 14311-14312 | http://purl.obolibrary.org/obo/CLO_0001020 | denotes | a |
T95 | 14799-14802 | http://purl.obolibrary.org/obo/PR_000001343 | denotes | aim |
T96 | 14905-14906 | http://purl.obolibrary.org/obo/CLO_0001020 | denotes | a |
T97 | 14922-14924 | http://purl.obolibrary.org/obo/CLO_0050509 | denotes | 27 |
T98 | 14946-14947 | http://purl.obolibrary.org/obo/CLO_0001020 | denotes | a |
T99 | 15071-15072 | http://purl.obolibrary.org/obo/CLO_0001020 | denotes | a |
T100 | 15121-15123 | http://purl.obolibrary.org/obo/CLO_0053733 | denotes | 11 |
T101 | 15213-15214 | http://purl.obolibrary.org/obo/CLO_0001020 | denotes | a |
T102 | 15449-15450 | http://purl.obolibrary.org/obo/CLO_0001020 | denotes | a |
T103 | 15508-15509 | http://purl.obolibrary.org/obo/CLO_0001020 | denotes | a |
T104 | 15558-15559 | http://purl.obolibrary.org/obo/CLO_0001020 | denotes | a |
T105 | 15589-15592 | http://purl.obolibrary.org/obo/CLO_0051582 | denotes | has |
T106 | 15684-15685 | http://purl.obolibrary.org/obo/CLO_0001020 | denotes | a |
T107 | 15873-15874 | http://purl.obolibrary.org/obo/CLO_0001020 | denotes | a |
T108 | 16028-16031 | http://purl.obolibrary.org/obo/CLO_0051582 | denotes | has |
T109 | 16032-16033 | http://purl.obolibrary.org/obo/CLO_0001020 | denotes | a |
T110 | 16232-16234 | http://purl.obolibrary.org/obo/CLO_0050510 | denotes | 18 |
T111 | 16881-16882 | http://purl.obolibrary.org/obo/CLO_0001020 | denotes | a |
T112 | 17067-17069 | http://purl.obolibrary.org/obo/CLO_0001382 | denotes | 48 |
T113 | 17080-17081 | http://purl.obolibrary.org/obo/CLO_0001020 | denotes | a |
T114 | 17116-17118 | http://purl.obolibrary.org/obo/CLO_0001000 | denotes | 35 |
T115 | 17286-17287 | http://purl.obolibrary.org/obo/CLO_0001020 | denotes | a |
T116 | 17619-17620 | http://purl.obolibrary.org/obo/CLO_0001020 | denotes | a |
T117 | 17726-17727 | http://purl.obolibrary.org/obo/CLO_0001020 | denotes | a |
T118 | 18373-18374 | http://purl.obolibrary.org/obo/CLO_0001020 | denotes | a |
T119 | 18748-18749 | http://purl.obolibrary.org/obo/CLO_0001020 | denotes | a |
T120 | 18885-18889 | http://purl.obolibrary.org/obo/CLO_0001550 | denotes | a 10 |
T121 | 18971-18972 | http://purl.obolibrary.org/obo/CLO_0001020 | denotes | a |
T122 | 19053-19056 | http://purl.obolibrary.org/obo/CLO_0001562 | denotes | a 2 |
T123 | 19053-19056 | http://purl.obolibrary.org/obo/CLO_0001563 | denotes | a 2 |
T124 | 19315-19316 | http://purl.obolibrary.org/obo/CLO_0001020 | denotes | a |
T125 | 19343-19344 | http://purl.obolibrary.org/obo/CLO_0001020 | denotes | a |
T126 | 19549-19551 | http://purl.obolibrary.org/obo/CLO_0001000 | denotes | 35 |
T127 | 19563-19564 | http://purl.obolibrary.org/obo/CLO_0001020 | denotes | a |
T128 | 19870-19882 | http://purl.obolibrary.org/obo/OBI_0000245 | denotes | Organization |
T129 | 19884-19888 | http://purl.obolibrary.org/obo/CLO_0001185 | denotes | 2018 |
T130 | 19916-19917 | http://purl.obolibrary.org/obo/CLO_0001020 | denotes | a |
T131 | 20512-20514 | http://purl.obolibrary.org/obo/CLO_0053755 | denotes | ES |
T132 | 20531-20536 | http://purl.obolibrary.org/obo/CLO_0008479 | denotes | Pp/ES |
T133 | 20574-20575 | http://purl.obolibrary.org/obo/CLO_0001020 | denotes | a |
T134 | 20709-20714 | http://purl.obolibrary.org/obo/CLO_0008479 | denotes | Pp/ES |
T135 | 20782-20783 | http://purl.obolibrary.org/obo/CLO_0001020 | denotes | a |
T136 | 20906-20911 | http://purl.obolibrary.org/obo/CLO_0008479 | denotes | Pp/ES |
T137 | 20954-20955 | http://purl.obolibrary.org/obo/CLO_0001020 | denotes | a |
T138 | 20973-20978 | http://purl.obolibrary.org/obo/CLO_0008479 | denotes | Pp/ES |
T139 | 21018-21023 | http://purl.obolibrary.org/obo/CLO_0008479 | denotes | Pp/ES |
T140 | 21093-21098 | http://purl.obolibrary.org/obo/CLO_0008479 | denotes | Pp/ES |
T141 | 21139-21144 | http://purl.obolibrary.org/obo/CLO_0008479 | denotes | Pp/ES |
T142 | 21157-21162 | http://purl.obolibrary.org/obo/CLO_0008479 | denotes | Pp/ES |
T143 | 21457-21458 | http://purl.obolibrary.org/obo/CLO_0001020 | denotes | a |
T144 | 21521-21522 | http://purl.obolibrary.org/obo/CLO_0001020 | denotes | a |
T145 | 21548-21553 | http://purl.obolibrary.org/obo/CLO_0008479 | denotes | Pp/ES |
T146 | 21566-21571 | http://purl.obolibrary.org/obo/CLO_0008479 | denotes | Pp/ES |
T147 | 21671-21676 | http://purl.obolibrary.org/obo/CLO_0008479 | denotes | Pp/ES |
T148 | 21690-21695 | http://purl.obolibrary.org/obo/CLO_0008479 | denotes | Pp/ES |
T149 | 21822-21823 | http://purl.obolibrary.org/obo/CLO_0001020 | denotes | a |
T150 | 21990-21993 | http://purl.obolibrary.org/obo/CLO_0051582 | denotes | has |
T151 | 22163-22164 | http://purl.obolibrary.org/obo/CLO_0001020 | denotes | a |
T152 | 22184-22189 | http://purl.obolibrary.org/obo/CLO_0008479 | denotes | Pp/ES |
T153 | 22204-22209 | http://purl.obolibrary.org/obo/CLO_0008479 | denotes | Pp/ES |
T154 | 22404-22405 | http://purl.obolibrary.org/obo/CLO_0001020 | denotes | a |
T155 | 22762-22763 | http://purl.obolibrary.org/obo/CLO_0001020 | denotes | a |
T156 | 22828-22829 | http://purl.obolibrary.org/obo/CLO_0001020 | denotes | a |
T157 | 22926-22929 | http://purl.obolibrary.org/obo/CLO_0051582 | denotes | has |
T158 | 22930-22931 | http://purl.obolibrary.org/obo/CLO_0001020 | denotes | a |
T159 | 22962-22967 | http://purl.obolibrary.org/obo/CLO_0008479 | denotes | Pp/ES |
T160 | 23606-23607 | http://purl.obolibrary.org/obo/CLO_0001020 | denotes | a |
T161 | 23719-23724 | http://purl.obolibrary.org/obo/CLO_0008479 | denotes | Pp/Es |
T162 | 24012-24013 | http://purl.obolibrary.org/obo/CLO_0001020 | denotes | a |
T163 | 24257-24259 | http://purl.obolibrary.org/obo/CLO_0050507 | denotes | 22 |
T164 | 24335-24336 | http://purl.obolibrary.org/obo/CLO_0001020 | denotes | a |
T165 | 24432-24433 | http://purl.obolibrary.org/obo/CLO_0001020 | denotes | a |
T166 | 24739-24740 | http://purl.obolibrary.org/obo/CLO_0001020 | denotes | a |
T167 | 24770-24771 | http://purl.obolibrary.org/obo/CLO_0001020 | denotes | a |
T168 | 25138-25139 | http://purl.obolibrary.org/obo/CLO_0001020 | denotes | a |
T169 | 25526-25527 | http://purl.obolibrary.org/obo/CLO_0001020 | denotes | a |
T170 | 25649-25650 | http://purl.obolibrary.org/obo/CLO_0001020 | denotes | a |
T171 | 25702-25703 | http://purl.obolibrary.org/obo/CLO_0001020 | denotes | a |
T172 | 25862-25864 | http://purl.obolibrary.org/obo/CLO_0001000 | denotes | 35 |
T173 | 25973-25974 | http://purl.obolibrary.org/obo/CLO_0001020 | denotes | a |
T174 | 26005-26006 | http://purl.obolibrary.org/obo/CLO_0001020 | denotes | a |
T175 | 26019-26020 | http://purl.obolibrary.org/obo/CLO_0001020 | denotes | a |
T176 | 26335-26336 | http://purl.obolibrary.org/obo/CLO_0001020 | denotes | a |
T177 | 26679-26680 | http://purl.obolibrary.org/obo/CLO_0001020 | denotes | a |
T178 | 26746-26747 | http://purl.obolibrary.org/obo/CLO_0001020 | denotes | a |
T179 | 26835-26836 | http://purl.obolibrary.org/obo/CLO_0001020 | denotes | a |
T180 | 26844-26845 | http://purl.obolibrary.org/obo/CLO_0001020 | denotes | a |
T181 | 27021-27031 | http://purl.obolibrary.org/obo/CLO_0001658 | denotes | activities |
T182 | 27519-27520 | http://purl.obolibrary.org/obo/CLO_0001020 | denotes | a |
T183 | 27727-27728 | http://purl.obolibrary.org/obo/CLO_0001020 | denotes | a |
T184 | 27853-27854 | http://purl.obolibrary.org/obo/CLO_0001020 | denotes | a |
T185 | 27918-27919 | http://purl.obolibrary.org/obo/CLO_0001020 | denotes | a |
T186 | 28081-28082 | http://purl.obolibrary.org/obo/CLO_0001020 | denotes | a |
T187 | 28127-28128 | http://purl.obolibrary.org/obo/CLO_0001020 | denotes | a |
T188 | 28157-28158 | http://purl.obolibrary.org/obo/CLO_0001020 | denotes | a |
T189 | 28172-28174 | http://purl.obolibrary.org/obo/CLO_0050507 | denotes | 22 |
T190 | 28231-28232 | http://purl.obolibrary.org/obo/CLO_0001020 | denotes | a |
T191 | 28272-28273 | http://purl.obolibrary.org/obo/CLO_0001020 | denotes | a |
T192 | 28627-28632 | http://purl.obolibrary.org/obo/CLO_0008479 | denotes | Pp/ES |
LitCovid-PD-CHEBI
Id | Subject | Object | Predicate | Lexical cue | chebi_id |
---|---|---|---|---|---|
T2 | 1883-1885 | Chemical | denotes | PM | http://purl.obolibrary.org/obo/CHEBI_141444|http://purl.obolibrary.org/obo/CHEBI_16410|http://purl.obolibrary.org/obo/CHEBI_53551 |
T5 | 1888-1895 | Chemical | denotes | sulphur | http://purl.obolibrary.org/obo/CHEBI_17909|http://purl.obolibrary.org/obo/CHEBI_26833 |
T7 | 1896-1902 | Chemical | denotes | oxides | http://purl.obolibrary.org/obo/CHEBI_25741 |
T8 | 1910-1925 | Chemical | denotes | nitrogen oxides | http://purl.obolibrary.org/obo/CHEBI_35196 |
T9 | 1910-1918 | Chemical | denotes | nitrogen | http://purl.obolibrary.org/obo/CHEBI_25555 |
T10 | 1919-1925 | Chemical | denotes | oxides | http://purl.obolibrary.org/obo/CHEBI_25741 |
T11 | 1927-1930 | Chemical | denotes | NOx | http://purl.obolibrary.org/obo/CHEBI_35196 |
T12 | 1933-1948 | Chemical | denotes | carbon monoxide | http://purl.obolibrary.org/obo/CHEBI_17245 |
T13 | 1933-1939 | Chemical | denotes | carbon | http://purl.obolibrary.org/obo/CHEBI_27594|http://purl.obolibrary.org/obo/CHEBI_33415 |
T15 | 1950-1952 | Chemical | denotes | CO | http://purl.obolibrary.org/obo/CHEBI_17245 |
T16 | 1958-1972 | Chemical | denotes | carbon dioxide | http://purl.obolibrary.org/obo/CHEBI_16526 |
T17 | 1958-1964 | Chemical | denotes | carbon | http://purl.obolibrary.org/obo/CHEBI_27594|http://purl.obolibrary.org/obo/CHEBI_33415 |
T19 | 1974-1977 | Chemical | denotes | CO2 | http://purl.obolibrary.org/obo/CHEBI_16526 |
T20 | 2621-2623 | Chemical | denotes | Gu | http://purl.obolibrary.org/obo/CHEBI_42820 |
T21 | 3174-3176 | Chemical | denotes | Lu | http://purl.obolibrary.org/obo/CHEBI_33382 |
T22 | 4194-4197 | Chemical | denotes | CO2 | http://purl.obolibrary.org/obo/CHEBI_16526 |
T23 | 4454-4470 | Chemical | denotes | nitrogen dioxide | http://purl.obolibrary.org/obo/CHEBI_33101 |
T24 | 4454-4462 | Chemical | denotes | nitrogen | http://purl.obolibrary.org/obo/CHEBI_25555 |
T25 | 4471-4474 | Chemical | denotes | NO2 | http://purl.obolibrary.org/obo/CHEBI_16301|http://purl.obolibrary.org/obo/CHEBI_33101 |
T27 | 10468-10471 | Chemical | denotes | Abu | http://purl.obolibrary.org/obo/CHEBI_35621 |
T28 | 10707-10709 | Chemical | denotes | La | http://purl.obolibrary.org/obo/CHEBI_33336 |
T29 | 11061-11063 | Chemical | denotes | La | http://purl.obolibrary.org/obo/CHEBI_33336 |
T30 | 11194-11196 | Chemical | denotes | La | http://purl.obolibrary.org/obo/CHEBI_33336 |
T31 | 11366-11368 | Chemical | denotes | La | http://purl.obolibrary.org/obo/CHEBI_33336 |
T32 | 11875-11877 | Chemical | denotes | DW | http://purl.obolibrary.org/obo/CHEBI_73831 |
T33 | 12935-12941 | Chemical | denotes | carbon | http://purl.obolibrary.org/obo/CHEBI_27594|http://purl.obolibrary.org/obo/CHEBI_33415 |
T35 | 20485-20487 | Chemical | denotes | Pp | http://purl.obolibrary.org/obo/CHEBI_26294 |
T36 | 20512-20514 | Chemical | denotes | ES | http://purl.obolibrary.org/obo/CHEBI_73509 |
T37 | 20531-20533 | Chemical | denotes | Pp | http://purl.obolibrary.org/obo/CHEBI_26294 |
T38 | 20534-20536 | Chemical | denotes | ES | http://purl.obolibrary.org/obo/CHEBI_73509 |
T39 | 20709-20711 | Chemical | denotes | Pp | http://purl.obolibrary.org/obo/CHEBI_26294 |
T40 | 20712-20714 | Chemical | denotes | ES | http://purl.obolibrary.org/obo/CHEBI_73509 |
T41 | 20906-20908 | Chemical | denotes | Pp | http://purl.obolibrary.org/obo/CHEBI_26294 |
T42 | 20909-20911 | Chemical | denotes | ES | http://purl.obolibrary.org/obo/CHEBI_73509 |
T43 | 20973-20975 | Chemical | denotes | Pp | http://purl.obolibrary.org/obo/CHEBI_26294 |
T44 | 20976-20978 | Chemical | denotes | ES | http://purl.obolibrary.org/obo/CHEBI_73509 |
T45 | 21018-21020 | Chemical | denotes | Pp | http://purl.obolibrary.org/obo/CHEBI_26294 |
T46 | 21021-21023 | Chemical | denotes | ES | http://purl.obolibrary.org/obo/CHEBI_73509 |
T47 | 21093-21095 | Chemical | denotes | Pp | http://purl.obolibrary.org/obo/CHEBI_26294 |
T48 | 21096-21098 | Chemical | denotes | ES | http://purl.obolibrary.org/obo/CHEBI_73509 |
T49 | 21139-21141 | Chemical | denotes | Pp | http://purl.obolibrary.org/obo/CHEBI_26294 |
T50 | 21142-21144 | Chemical | denotes | ES | http://purl.obolibrary.org/obo/CHEBI_73509 |
T51 | 21157-21159 | Chemical | denotes | Pp | http://purl.obolibrary.org/obo/CHEBI_26294 |
T52 | 21160-21162 | Chemical | denotes | ES | http://purl.obolibrary.org/obo/CHEBI_73509 |
T53 | 21548-21550 | Chemical | denotes | Pp | http://purl.obolibrary.org/obo/CHEBI_26294 |
T54 | 21551-21553 | Chemical | denotes | ES | http://purl.obolibrary.org/obo/CHEBI_73509 |
T55 | 21566-21568 | Chemical | denotes | Pp | http://purl.obolibrary.org/obo/CHEBI_26294 |
T56 | 21569-21571 | Chemical | denotes | ES | http://purl.obolibrary.org/obo/CHEBI_73509 |
T57 | 21671-21673 | Chemical | denotes | Pp | http://purl.obolibrary.org/obo/CHEBI_26294 |
T58 | 21674-21676 | Chemical | denotes | ES | http://purl.obolibrary.org/obo/CHEBI_73509 |
T59 | 21690-21692 | Chemical | denotes | Pp | http://purl.obolibrary.org/obo/CHEBI_26294 |
T60 | 21693-21695 | Chemical | denotes | ES | http://purl.obolibrary.org/obo/CHEBI_73509 |
T61 | 22184-22186 | Chemical | denotes | Pp | http://purl.obolibrary.org/obo/CHEBI_26294 |
T62 | 22187-22189 | Chemical | denotes | ES | http://purl.obolibrary.org/obo/CHEBI_73509 |
T63 | 22204-22206 | Chemical | denotes | Pp | http://purl.obolibrary.org/obo/CHEBI_26294 |
T64 | 22207-22209 | Chemical | denotes | ES | http://purl.obolibrary.org/obo/CHEBI_73509 |
T65 | 22764-22767 | Chemical | denotes | GDP | http://purl.obolibrary.org/obo/CHEBI_17552|http://purl.obolibrary.org/obo/CHEBI_58189 |
T67 | 22962-22964 | Chemical | denotes | Pp | http://purl.obolibrary.org/obo/CHEBI_26294 |
T68 | 22965-22967 | Chemical | denotes | ES | http://purl.obolibrary.org/obo/CHEBI_73509 |
T69 | 23719-23721 | Chemical | denotes | Pp | http://purl.obolibrary.org/obo/CHEBI_26294 |
T70 | 23722-23724 | Chemical | denotes | Es | http://purl.obolibrary.org/obo/CHEBI_33393 |
T71 | 28498-28509 | Chemical | denotes | fossil fuel | http://purl.obolibrary.org/obo/CHEBI_35230 |
T72 | 28505-28509 | Chemical | denotes | fuel | http://purl.obolibrary.org/obo/CHEBI_33292 |
T73 | 28627-28629 | Chemical | denotes | Pp | http://purl.obolibrary.org/obo/CHEBI_26294 |
T74 | 28630-28632 | Chemical | denotes | ES | http://purl.obolibrary.org/obo/CHEBI_73509 |
LitCovid-PubTator
Id | Subject | Object | Predicate | Lexical cue | tao:has_database_id |
---|---|---|---|---|---|
21 | 1087-1091 | Species | denotes | H1N1 | Tax:114727 |
37 | 1780-1786 | Species | denotes | people | Tax:9606 |
38 | 2303-2309 | Species | denotes | people | Tax:9606 |
39 | 1888-1902 | Chemical | denotes | sulphur oxides | |
40 | 1910-1918 | Chemical | denotes | nitrogen | MESH:D009584 |
41 | 1933-1948 | Chemical | denotes | carbon monoxide | MESH:D002248 |
42 | 1950-1952 | Chemical | denotes | CO | MESH:D002248 |
43 | 1958-1972 | Chemical | denotes | carbon dioxide | MESH:D002245 |
44 | 1974-1977 | Chemical | denotes | CO2 | MESH:D002245 |
45 | 2197-2203 | Disease | denotes | deaths | MESH:D003643 |
46 | 2477-2483 | Disease | denotes | stroke | MESH:D020521 |
47 | 2485-2496 | Disease | denotes | lung cancer | MESH:D008175 |
48 | 2498-2535 | Disease | denotes | chronic obstructive pulmonary disease | MESH:D029424 |
49 | 2537-2550 | Disease | denotes | heart disease | MESH:D006331 |
50 | 2555-2577 | Disease | denotes | respiratory infections | MESH:D012141 |
51 | 2586-2595 | Disease | denotes | pneumonia | MESH:D011014 |
57 | 3342-3351 | Species | denotes | SARS-CoV2 | Tax:2697049 |
58 | 3462-3468 | Species | denotes | people | Tax:9606 |
59 | 3719-3722 | Chemical | denotes | oil | MESH:D009821 |
60 | 3817-3820 | Chemical | denotes | oil | MESH:D009821 |
61 | 3369-3377 | Disease | denotes | COVID-19 | MESH:C000657245 |
73 | 4903-4914 | Species | denotes | coronavirus | Tax:11118 |
74 | 5784-5795 | Species | denotes | coronavirus | Tax:11118 |
75 | 6091-6102 | Species | denotes | coronavirus | Tax:11118 |
76 | 4194-4197 | Chemical | denotes | CO2 | MESH:D002245 |
77 | 4454-4462 | Chemical | denotes | nitrogen | MESH:D009584 |
78 | 4373-4379 | Disease | denotes | deaths | MESH:D003643 |
79 | 4385-4393 | Disease | denotes | COVID-19 | MESH:C000657245 |
80 | 4431-4437 | Disease | denotes | deaths | MESH:D003643 |
81 | 5760-5769 | Disease | denotes | mortality | MESH:D003643 |
82 | 5838-5844 | Disease | denotes | deaths | MESH:D003643 |
83 | 6103-6112 | Disease | denotes | mortality | MESH:D003643 |
86 | 7105-7114 | Disease | denotes | paralysis | MESH:D010243 |
87 | 7131-7139 | Disease | denotes | COVID-19 | MESH:C000657245 |
89 | 8361-8369 | Disease | denotes | COVID-19 | MESH:C000657245 |
92 | 11462-11468 | Species | denotes | Turkey | Tax:9103 |
93 | 10850-10857 | Disease | denotes | Colombo | |
95 | 9175-9183 | Disease | denotes | COVID-19 | MESH:C000657245 |
98 | 12935-12941 | Chemical | denotes | carbon | MESH:D002244 |
99 | 12804-12812 | Disease | denotes | COVID-19 | MESH:C000657245 |
101 | 16315-16322 | Disease | denotes | Colombo | |
104 | 16142-16148 | Chemical | denotes | Astana | |
105 | 15967-15974 | Disease | denotes | Colombo | |
107 | 19514-19519 | Chemical | denotes | PM2.5 | |
110 | 20534-20536 | Chemical | denotes | ES | |
111 | 20712-20714 | Chemical | denotes | ES | |
113 | 21701-21708 | Disease | denotes | Colombo | |
115 | 22965-22967 | Chemical | denotes | ES | |
117 | 23722-23724 | Chemical | denotes | Es | MESH:D004540 |
119 | 24077-24080 | Chemical | denotes | PM2 | |
121 | 25834-25839 | Chemical | denotes | PM2.5 | |
123 | 28428-28435 | Disease | denotes | Colombo |
LitCovid-PD-GO-BP
Id | Subject | Object | Predicate | Lexical cue |
---|---|---|---|---|
T3 | 209-217 | http://purl.obolibrary.org/obo/GO_0007610 | denotes | behavior |
T4 | 2866-2872 | http://purl.obolibrary.org/obo/GO_0040007 | denotes | growth |
T5 | 3668-3677 | http://purl.obolibrary.org/obo/GO_0006810 | denotes | transport |
T6 | 5019-5027 | http://purl.obolibrary.org/obo/GO_0007612 | denotes | learning |
T7 | 12554-12562 | http://purl.obolibrary.org/obo/GO_0007610 | denotes | behavior |
T8 | 24980-24988 | http://purl.obolibrary.org/obo/GO_0007610 | denotes | behavior |
T9 | 26809-26817 | http://purl.obolibrary.org/obo/GO_0007610 | denotes | behavior |
T10 | 28532-28543 | http://purl.obolibrary.org/obo/GO_0065007 | denotes | regulations |
T11 | 28932-28941 | http://purl.obolibrary.org/obo/GO_0006810 | denotes | transport |
LitCovid-sentences
Id | Subject | Object | Predicate | Lexical cue |
---|---|---|---|---|
T21 | 0-15 | Sentence | denotes | 1 Introduction |
T22 | 16-450 | Sentence | denotes | Currently, socio-ecological systems have a great impact on companies, cities and territories; the sustainability and technology associated with smart cities are merged to better understand the behavior of this type of systems, and the data provides cities and territories with the information necessary for sufficient monitoring and evaluation leading to coherent environmental policies in adaptive environments (Waylen et al., 2019). |
T23 | 451-658 | Sentence | denotes | It is necessary to formulate new socio-ecological models that allow describing the coevolution of the economy, the environment and society in the face of the dynamics of wealth and population (Ursino, 2019). |
T24 | 659-800 | Sentence | denotes | However, few models efficiently predict the entry of random variables into these complex processes, which validate their evolution over time. |
T25 | 801-988 | Sentence | denotes | One of the clearest examples of a chaotic variable is climate, however, there are other variables that can quickly intervene in a socio-ecological system and wreak havoc, such as a virus. |
T26 | 989-1224 | Sentence | denotes | For example, in Brisbane, Australia ecological factors appear to have played an important role in H1N1 transmission cycles (Hu et al., 2012), with temperature and precipitation being substantial variables in the evolution of the virus. |
T27 | 1225-1437 | Sentence | denotes | There are some other works of socioeconomic studies and viruses such as (Mamelund et al., 2019), where a study is carried out between the socioeconomic levels and the influenza-related pandemics of 1918 and 2009. |
T28 | 1438-1644 | Sentence | denotes | The foregoing demonstrates the importance of correlating the factors that can substantially alter the socio-ecological systems in which we live and to be able to study their evolution and impact on society. |
T29 | 1645-1827 | Sentence | denotes | One of the key factors in recent years, which has been the subject of several scientific studies, is the impact of poor air quality on people’s health and its consequences over time. |
T30 | 1828-2225 | Sentence | denotes | The emission of pollutants such as particulate matter (PM), sulphur oxides (SOx), nitrogen oxides (NOx), carbon monoxide (CO) and carbon dioxide (CO2), are the pollutants that are generated in greater quantity and according to estimates of the World Health Organization (WHO) 2016 produce annually in cities and rural areas around the world about 4.2 million premature deaths (Cohen et al., 2017). |
T31 | 2226-2657 | Sentence | denotes | The WHO (World Health Organization, 2018) estimates that about seven million people die each year from exposure to PM2.5 particles, which enter directly into the respiratory system and are deposited in the lung region causing serious diseases such as stroke, lung cancer, chronic obstructive pulmonary disease, heart disease and respiratory infections such as pneumonia. (Cachon et al., 2014), (Gu et al., 2017), (Ng et al., 2019). |
T32 | 2658-3089 | Sentence | denotes | On the other hand, a World Bank report of 2018 shows in graphs the most relevant socio-economic and socio-ecological aspects that impact the world, where global warming, poor air quality and urban population growth among others, leave chilling figures, as 91% of the world population lives in places with poor air quality, places like cities that increased by 55% their urban residents between 1960 and 2018 (The World Bank, 2018). |
T33 | 3090-3191 | Sentence | denotes | In December 2019, one of the most deadly viruses in the last 100 years is reported (Lu et al., 2020). |
T34 | 3192-3320 | Sentence | denotes | China reports this new pathogen to the WHO on December 31, and only three months later this organization declares it a pandemic. |
T35 | 3321-3506 | Sentence | denotes | The new virus called SARS-CoV2 and the cause of COVID-19 has stopped global activity in a few months and has taken the lives of thousands of people in different cities around the world. |
T36 | 3507-3699 | Sentence | denotes | The impact of this virus on the socio-economic level is causing markets to tremble, world stock markets to collapse, all flights to be cancelled and borders and transport systems to be closed. |
T37 | 3700-3861 | Sentence | denotes | On the other hand, oil demand has dropped and producers are running out of places to store all the excess barrels of oil as it has fallen below $0 US per barrel. |
T38 | 3862-3984 | Sentence | denotes | However, this pandemic also caused air quality to improve in many of the world’s cities, reducing environmental pollution. |
T39 | 3985-4170 | Sentence | denotes | This global closure has made it possible to obtain interesting environmental data for analysis and several scientific investigations related precisely to these socio-ecological changes. |
T40 | 4171-4287 | Sentence | denotes | In China, for example, CO2 emissions were reduced by 25% and by 6% worldwide according to (Hanaoka and Masui, 2020). |
T41 | 4288-4485 | Sentence | denotes | In (Dutheil et al., 2020), an initial comparative analysis was made of the number of deaths from COVID-19 and the number of annual air quality deaths with respect to nitrogen dioxide NO2 emissions. |
T42 | 4486-4727 | Sentence | denotes | This analysis was based on data obtained by satellite (NASA, 2020) showing the advantages that the isolation of the population in their homes has had due to the emergency by the shutdown of industries and vehicle mobility (Tan et al., 2009). |
T43 | 4728-4915 | Sentence | denotes | The same information from NASA, plus information taken from ESA, was used in (Muhammad et al., 2020) to perform a compilation of satellite environmental data before and after coronavirus. |
T44 | 4916-5093 | Sentence | denotes | The figures in this paper show the temporary environmental benefit as a major positive impact and as a learning model for governments to enable new socio-environmental policies. |
T45 | 5094-5158 | Sentence | denotes | This last analysis was done for Europe, China and North America. |
T46 | 5159-5432 | Sentence | denotes | Likewise, in (Mollalo et al., 2020) models are made of the type of spatial dependence and weighted regression of 35 variables from the environmental to the socioeconomic ones related to the incidence of the disease in the first 90 days of the outbreak in the United States. |
T47 | 5433-5676 | Sentence | denotes | These results, according to Mollalo, will serve as a basis for future geographic modeling of any disease, as well as for policy with targeted, science-based interventions that can be extrapolated to other cities and countries around the world. |
T48 | 5677-5796 | Sentence | denotes | Finally, Ogen’s research has found a direct relationship between contamination and mortality caused by the coronavirus. |
T49 | 5797-5987 | Sentence | denotes | The study concludes that 78% of the 4443 deaths recorded on a single day in Europe (19 march) occurred in five specific, highly contaminated areas: four regions of northern Italy and Madrid. |
T50 | 5988-6183 | Sentence | denotes | These results indicate that long-term exposure to particulate pollutants may be a major contributor to coronavirus mortality, not only in these regions, but in the rest of the world (Ogen, 2020). |
T51 | 6184-6418 | Sentence | denotes | All these analyses described above are necessary to evaluate the socio-ecological and socio-economic changes in all the cities of the world and try to show the positive impacts in order to obtain some benefits from this global crisis. |
T52 | 6419-6655 | Sentence | denotes | Ultimately, this paper uses data from the weather stations of the 50 most polluted cities in the world and makes a comparison of air quality with respect to PM2.5 particulate matter before and during the quarantine of each capital city. |
T53 | 6657-6671 | Sentence | denotes | 2 Methodology |
T54 | 6672-6828 | Sentence | denotes | In the year 2019 a study was presented on Meteosim online platform where they made an analysis on the most polluting capitals in the world (Meteosim, 2019). |
T55 | 6829-7026 | Sentence | denotes | This research indicates that one of the most dangerous pollutants is fine particulate matter with diameters ≤2.5 μm (PM2.5), so the analysis of the fifty most contaminated capitals was carried out. |
T56 | 7027-7236 | Sentence | denotes | Based on the above information and according to the automotive and industrial paralysis in the world by COVID-19, for this research they were collected the PM2.5 data from an online platform (WAQ Index, 2020). |
T57 | 7237-7465 | Sentence | denotes | This tool, called World Air Quality Index (WAQI) is used for obtained the information historical of Air Quality Data in especial for this paper of PM2.5 particulate matter of each capital before quarantine and during quarantine. |
T58 | 7466-7593 | Sentence | denotes | For each capital city, the information was corroborated with the territorial entity of data administration at the public level. |
T59 | 7594-7715 | Sentence | denotes | For example, Bogotá, Colombia was verified on the IBOCA platform of the Secretary of the Environment (Environment, 2019). |
T60 | 7716-7864 | Sentence | denotes | For Delhi, India was verified on the Real Time Ambient Air Quality Data Platform of the Department of Environment (Department of Environment, 2020). |
T61 | 7865-7971 | Sentence | denotes | For Berlin, Germany was verify with the Umwelt Bundesamt platform of the German Environment Agency (2020). |
T62 | 7972-7996 | Sentence | denotes | And so on for each city. |
T63 | 7997-8086 | Sentence | denotes | In this context, the research process in the paper has been divided into five main steps. |
T64 | 8087-8089 | Sentence | denotes | 1. |
T65 | 8090-8144 | Sentence | denotes | Identification of the 50 most polluted capital cities. |
T66 | 8145-8147 | Sentence | denotes | 2. |
T67 | 8148-8185 | Sentence | denotes | Review of the quarantine information. |
T68 | 8186-8188 | Sentence | denotes | 3. |
T69 | 8189-8244 | Sentence | denotes | Data collection of population and the weather stations. |
T70 | 8245-8247 | Sentence | denotes | 4. |
T71 | 8248-8270 | Sentence | denotes | PM2.5 Data extraction. |
T72 | 8271-8273 | Sentence | denotes | 5. |
T73 | 8274-8296 | Sentence | denotes | Graphics and analysis. |
T74 | 8298-8369 | Sentence | denotes | 3 World’s most contaminated capital cities and quarantined by COVID-19 |
T75 | 8370-8659 | Sentence | denotes | One of the most important guidelines carried out by the WHO in matters of air quality is not to exceed 10 μg/m3 of annual average concentration or 25 μg/m3 of 24-h concentrations, for that reason Meteosim (2019), presents the cities that exceed 10 μg/m3 of annual average in the year 2018. |
T76 | 8660-8760 | Sentence | denotes | Table 1 shows the start of the quarantine or the alarm status of the world’s most polluted capitals. |
T77 | 8761-8959 | Sentence | denotes | As shown in the table, firstly Delhi (India) is the capital most polluted by fine particulate matter, reaching an annual average of 113,5 μg/m3 for 2018, secondly Dhaka (Bangladesh) with 97,1 μg/m3. |
T78 | 8960-9059 | Sentence | denotes | Lisbon and Berlin are least polluted capitals, presenting an annual average of 11,7 μg/m3 for 2018. |
T79 | 9060-9184 | Sentence | denotes | Regarding the aforementioned ranking, each capital was taken and the lockdown date was sought due to the effect of COVID-19. |
T80 | 9185-9231 | Sentence | denotes | 12% of the capitals do not apply any lockdown. |
T81 | 9232-9329 | Sentence | denotes | The first countries to start blocking mobility to colleges, universities and apply telework were: |
T82 | 9330-9349 | Sentence | denotes | Mongolia and China. |
T83 | 9350-9416 | Sentence | denotes | In March 9th lockdowns began to be applied in the other countries. |
T84 | 9417-9628 | Sentence | denotes | Not all countries carried out a lockdown, there were some exceptions such as Kazakhstan (Astana), where a state of emergency is declared on March 15th, as well as in Romania on 24 March and Indonesia on April 2. |
T85 | 9629-9815 | Sentence | denotes | On the other hand, other countries took partial or more drastic lockdown measures, for example, in Slovakia (Bratislava) it is allowed to walk or exercise outdoors with the mask protect. |
T86 | 9816-9944 | Sentence | denotes | Mexico City, declared a voluntary quarantine and Bangkok (Thailand) and Belgrade (Serbia), have declared a curfew since April 4. |
T87 | 9945-9999 | Sentence | denotes | Table 1 Location-start to quarantine (Meteosim, 2019). |
T88 | 10000-10080 | Sentence | denotes | Country Location (Capital cities) PM2.5 μg/m3 (Annual) Date (Quarantine) Studies |
T89 | 10081-10120 | Sentence | denotes | India Delhi 113,5 25/03/20 Soler (2020) |
T90 | 10121-10172 | Sentence | denotes | Bangladesh Dhaka 97,1 16/03/20 Global Voices (2020) |
T91 | 10173-10224 | Sentence | denotes | Afghanistan Kabul 61,8 28/03/20 Europapress (2020a) |
T92 | 10225-10255 | Sentence | denotes | Bahrain (Barein) Manama 59,8 ∗ |
T93 | 10256-10310 | Sentence | denotes | Mongolia Ulaanbaatar 58,5 25/01/20 Anandsaikhan (2020) |
T94 | 10311-10360 | Sentence | denotes | Kuwait Kubait City 56 9/03/20 Europapress (2020b) |
T95 | 10361-10414 | Sentence | denotes | Nepal Kathmandu 54,4 24/03/20 The Jakarta Post (2020) |
T96 | 10415-10463 | Sentence | denotes | China Bejing 50,9 29/01/20 BBC News Mundo (2020) |
T97 | 10464-10484 | Sentence | denotes | UAE Abu Dhabi 48,8 ∗ |
T98 | 10485-10539 | Sentence | denotes | Indonesia Jakarta 45,3 2/04/20 The Jakarta Post (2020) |
T99 | 10540-10583 | Sentence | denotes | Uganda Kampala 40,8 1/04/20 Museveni (2020) |
T100 | 10584-10634 | Sentence | denotes | Vietnam Hanoi 40,8 19/03/20 TheStraitsTimes (2020) |
T101 | 10635-10661 | Sentence | denotes | Pakistan Islammabad 38,6 ∗ |
T102 | 10662-10728 | Sentence | denotes | Bosnia & Hersegovina sarajevo, 38,4 17/03/20 La Vanguardia (2020a) |
T103 | 10729-10779 | Sentence | denotes | Uzbekistan Tashkent 34,3 24/03/20 Pikulicka (2020) |
T104 | 10780-10839 | Sentence | denotes | Macedonia del norte Skopje 34 10/04/20 BalkanInsight (2020) |
T105 | 10840-10889 | Sentence | denotes | Sri Lanka Colombo 32 12/03/20 Europapress (2020c) |
T106 | 10890-10940 | Sentence | denotes | Kosovo Pristina 30,4 11/04/20 Gazetaexpress (2020) |
T107 | 10941-10988 | Sentence | denotes | Kazahstan Astana 29,8 15/03/20 Gussarova (2020) |
T108 | 10989-11031 | Sentence | denotes | Chile Santiago 29,4 26/03/20 Perfil (2020) |
T109 | 11032-11082 | Sentence | denotes | Bulgaria Sofia 28,2 20/03/20 La Vanguardia (2020b) |
T110 | 11083-11120 | Sentence | denotes | Peru Lima 28 19/03/20 (AS Peru, 2020) |
T111 | 11121-11163 | Sentence | denotes | Iran Tehran 26,1 25/03/20 CNN Mundo (2020) |
T112 | 11164-11215 | Sentence | denotes | Thailand Bangkok 25,2 4/04/20 La Vanguardia (2020c) |
T113 | 11216-11256 | Sentence | denotes | Poland Warsaw 24,2 13/03/20 Solis (2020) |
T114 | 11257-11307 | Sentence | denotes | Serbia Belgrade 23,9 10/04/20 BalkanInsight (2020) |
T115 | 11308-11332 | Sentence | denotes | South Korea Seoul 23,3 ∗ |
T116 | 11333-11386 | Sentence | denotes | Romania Bucharest 20,3 24/03/20 (La vanguardia, 2020) |
T117 | 11387-11412 | Sentence | denotes | Cambodia PhnomPenh 20,1 ∗ |
T118 | 11413-11461 | Sentence | denotes | Mexico Mexico city 19,7 23/03/20 Mancilla (2020) |
T119 | 11462-11506 | Sentence | denotes | Turkey Ankara 19,6 4/04/20 GardaWorld (2020) |
T120 | 11507-11554 | Sentence | denotes | Isarel Tel Aviv 19,5 19/03/20 Itón Gadol (2020) |
T121 | 11555-11606 | Sentence | denotes | Lithuania Vilnius 18,2 14/03/20 Europapress (2020d) |
T122 | 11607-11650 | Sentence | denotes | Nicosia Cyprus 17,4 21/03/20 (GOV.UK, 2020) |
T123 | 11651-11714 | Sentence | denotes | Czech Republic Prague 17,4 16/03/20 Sociedad Agencia EFE (2020) |
T124 | 11715-11761 | Sentence | denotes | Slovakia Bratislavia 17,2 16/03/20 Tort (2020) |
T125 | 11762-11805 | Sentence | denotes | Hungary Budapest 16,5 27/03/20 Dunai (2020) |
T126 | 11806-11845 | Sentence | denotes | France Paris 15,6 17/03/20 Solis (2020) |
T127 | 11846-11884 | Sentence | denotes | Austria Vienna 15,2 15/03/20 DW (2020) |
T128 | 11885-11905 | Sentence | denotes | Taiwan Taipei 14,9 ∗ |
T129 | 11906-11953 | Sentence | denotes | Singapore Singapore 14,8 8/04/20 Naurana (2020) |
T130 | 11954-12007 | Sentence | denotes | Philippines Manila 14,3 17/03/20 (Europapress, 2020e) |
T131 | 12008-12057 | Sentence | denotes | Belgium Brussels 14,1 18/03/20 Theguardian (2020) |
T132 | 12058-12120 | Sentence | denotes | Colombia Bogota 13,9 25/03/20 (Eltiempo.comEltiempo.com, 2020) |
T133 | 12121-12180 | Sentence | denotes | Ukraine Kyiv 13,8 17/03/20 (Kyivpost.comKyivpost.com, 2020) |
T134 | 12181-12243 | Sentence | denotes | Japan Tokyo 13,1 25/03/20 (Japantimes.comJapantimes.com, 2020) |
T135 | 12244-12295 | Sentence | denotes | Switzerland Bern 12,8 16/03/20 (Swissinfo.ch, 2020) |
T136 | 12296-12360 | Sentence | denotes | United Kingdom London 12 23/03/20 (nbcnews.comnbcnews.com, 2020) |
T137 | 12361-12410 | Sentence | denotes | Germany Berlin 11,7 13/03/20 (Lanacion.com, 2020) |
T138 | 12411-12455 | Sentence | denotes | Portugal Lisbon 11,7 20/03/20 Sampson (2020) |
T139 | 12456-12471 | Sentence | denotes | ∗No quarantine. |
T140 | 12473-12517 | Sentence | denotes | 4 PM2.5 assessment before–during quarantine |
T141 | 12518-12813 | Sentence | denotes | This research seeks to evaluate the behavior of the main most polluting cities in the world from the comparison between a typical week, before quarantine (BQut), (considered in this document a week measured before entering confinement), and an atypical week, during quarantine (Qut) by COVID-19. |
T142 | 12814-12952 | Sentence | denotes | This week (Qut) is considered for analysis, due to the restriction of most economic activities that involve reducing the carbon footprint. |
T143 | 12953-13095 | Sentence | denotes | As an example, we have restrictive measures regarding citizen mobility and, therefore, vehicle mobility and industrial production are reduced. |
T144 | 13096-13210 | Sentence | denotes | These dates were selected according to Table 1, with a study range of one month before and after the start of Qut. |
T145 | 13211-13412 | Sentence | denotes | The PM2.5 values are obtained from the online platform (WAQ Index, 2020) and official weather stations of each city, the analyzed information depends on obtaining or recording data from the study date. |
T146 | 13413-13559 | Sentence | denotes | Cases are presented where meteorological stations that record PM2.5 are not obtained but only PM10, therefore, these cities could not be analyzed. |
T147 | 13560-13712 | Sentence | denotes | Likewise, there are days of the weeks that present alterations due to atmospheric conditions in each city, therefore, no data was taken from these days. |
T148 | 13713-13823 | Sentence | denotes | For 50 analized capital cities, 20% do not record PM2.5 data, corresponding to capitals from the countries of: |
T149 | 13824-13867 | Sentence | denotes | Germany, Philippines, Romania and Bulgaria. |
T150 | 13868-14095 | Sentence | denotes | On the other hand, for capitals with PM2.5 data, 45% of capitals correspond to the continent of Asia, followed by 42% to the continent of Europe, 10% to the continent of America and the remaining 25% to the continent of Africa. |
T151 | 14096-14233 | Sentence | denotes | The air pollution level, health implications and cautionary statement according to the PM2.5 conditions can be seen in (WAQ Index, 2020). |
T152 | 14234-14379 | Sentence | denotes | Likewise, in the analyzed countries presented below, decreases, increases or a constant level of PM2.5 emissions are observed during confinement. |
T153 | 14380-14567 | Sentence | denotes | This can be attributed to the confinement level in each country, at the beginning of the quarantine, or to the electricity generation increase depending on the generation technology used. |
T154 | 14568-14817 | Sentence | denotes | It is important to clarify that not all countries indicate reductions in their PM2.5 particles emitted during the analyzed week (Qut); each capital reflects special conditions that must be analyzed individually and this is not the aim of this paper. |
T155 | 14819-14828 | Sentence | denotes | 4.1 Asia |
T156 | 14829-14901 | Sentence | denotes | From Fig. 1, Fig. 2 , the most polluting capitals of Asia are presented. |
T157 | 14902-15125 | Sentence | denotes | At a general level, 27% of the capitals had a tendency to decrease the PM2.5 emission during Qut, however, the cities Kathmandu, Hanoi, Jakarta, Singapore and Tokyo had a tendency to increase the PM2.5 concentration by 11%. |
T158 | 15126-15313 | Sentence | denotes | It should be noted that Tokyo, with the exception of the other cities, did not present a mandatory quarantine but as an option of the Government, they requested non-mandatory teleworking. |
T159 | 15314-15479 | Sentence | denotes | For the analyzed Asian capitals, Dhaka in typical times (BQut), is the capital with the highest PM2.5 particles pollutant, registering a weekly average of 183 μg/m3. |
T160 | 15480-15545 | Sentence | denotes | This capital city, presents a reduction of 24% in the Qut period. |
T161 | 15546-15716 | Sentence | denotes | Delhi, with a weekly average of 140 μg/m3, has the highest reduction in particles polluting compared to other Asian countries, presenting a 40% reduction during Qut week. |
T162 | 15717-15850 | Sentence | denotes | Capital cities with an average concentration of 121.91 μg/m3 (Kabul, Ullabantar and Kuwait City) show average reductions of 33% BQut. |
T163 | 15851-15897 | Sentence | denotes | However, Bejing shows a lower reduction of 8%. |
T164 | 15898-16020 | Sentence | denotes | Capital cities with an average concentration of 106.83 μg/m3 (Kabul, Colombo and Tashkent) show average reductions of 28%. |
T165 | 16021-16073 | Sentence | denotes | Tehran has a typical week concentration of 90 μg/m3. |
T166 | 16074-16132 | Sentence | denotes | However, its reduction during the quarantine week was 39%. |
T167 | 16133-16258 | Sentence | denotes | Finally, Astana, with an average weekly concentration of 61.25 μg/m3, reduced its concentration by 18% during the Qut season. |
T168 | 16259-16415 | Sentence | denotes | Fig. 1 Capital cities Ankara, Astana, Bangkok, Beijing, Colombo, Delhi, Dhaka, Kabul and Hanoi, PM2.5 levels before and during quarantine (WAQ Index, 2020). |
T169 | 16416-16592 | Sentence | denotes | Fig. 2 Capital cities Jakarta, Kathmandu, Kubait city, Ulaanbaatar, Tashkent, Tehran, Tel Aviv, Tokio and Singapore PM2.5 levels before and during quarantine (WAQ Index, 2020). |
T170 | 16594-16605 | Sentence | denotes | 4.2 Europe |
T171 | 16606-16687 | Sentence | denotes | From Fig. 3, Fig. 4 it can be seen the seventeen (17) European capitals analyzed. |
T172 | 16688-16823 | Sentence | denotes | In general, European capital cities in typical weeks record PM2.5 concentrations below 80 μg/m3, with an AQI between Good and Moderate. |
T173 | 16824-16949 | Sentence | denotes | 50% of the studied European capitals, during the Qut had a tendency to decrease the PM2.5 concentration at an average of 23%. |
T174 | 16950-17016 | Sentence | denotes | However, the other 50% show an increase in the confinement season. |
T175 | 17017-17120 | Sentence | denotes | Budapest, with an annual average concentration of 48 μg/m3, is a city that presents an increase of 35%. |
T176 | 17121-17321 | Sentence | denotes | This capital does not present total confinement, they are allowed to exercise, go to work, with measures that allow them to circulate more widely, going from having a good to moderate AQI during BQut. |
T177 | 17322-17406 | Sentence | denotes | Bratislava, does not apply quarantine, leaving citizens to walk freely and exercise. |
T178 | 17407-17542 | Sentence | denotes | However, the date presented in Table 1, there are distance restrictions, suspension of classes and closure of sectors such as hostelry. |
T179 | 17543-17648 | Sentence | denotes | This European capital presents an increase in the Qut week, which went from a good to moderate AQI level. |
T180 | 17649-17779 | Sentence | denotes | Paris, London, Vienna, Brussels and Prague, are capitals that generally have a good AQI level, with an average record of 31 μg/m3. |
T181 | 17780-17894 | Sentence | denotes | However, in the confinement season, an increase in the concentrations of the PM2.5 particulate matter is observed. |
T182 | 17895-18058 | Sentence | denotes | Fig. 3 Capital cities Belgrade, Bern, Bratislavia, Brussels, Budapest, Cyprus, Kyiv, Lisbon and London PM2.5 levels before and during quarantine (WAQ Index, 2020). |
T183 | 18059-18211 | Sentence | denotes | Fig. 4 Capital cities Paris, Prague, Pristina, Sarajevo, Skopje, Vienna, Vilnius and Warsaw PM2.5 levels before and during quarantine (WAQ Index, 2020). |
T184 | 18213-18236 | Sentence | denotes | 4.3 America and Africa |
T185 | 18237-18319 | Sentence | denotes | Regarding the American Continent, four (4) capitals are analyzed in this research. |
T186 | 18320-18488 | Sentence | denotes | These capitals during typical periods, BQut, present a moderate AQI level, where Bogota is the capital with the highest PM2.5 concentration with an average of 98 μg/m3. |
T187 | 18489-18561 | Sentence | denotes | Followed by, Mexico City with 74 μg/m3, Santiago de Chile with 68 μg/m3. |
T188 | 18562-18623 | Sentence | denotes | Finally, Lima registers an average concentration of 58 μg/m3. |
T189 | 18624-18770 | Sentence | denotes | As well as, the period in confinement in Bogota, presents the greatest decrease in the cities analyzed during the Qut, with a 57% PM2.5 reduction. |
T190 | 18771-18949 | Sentence | denotes | Santiago de Chile, does not present quarantine in the entire city but in seven specific communes, which registers a 10% reduction in the concentration of this particulate matter. |
T191 | 18950-19120 | Sentence | denotes | Mexico City presents a voluntary quarantine by the population without government restrictions, however a 2% reduction is observed during the study week in Qut. (Fig. 5 ). |
T192 | 19121-19269 | Sentence | denotes | Fig. 5 Capital cities Bogotá, Lima, Mexico City, Santiago de Chile and Kampala (Africa) PM2.5 levels before and during quarantine (WAQ Index, 2020). |
T193 | 19270-19400 | Sentence | denotes | Kampala, as the only registered capital with a high AQI level, registers a weekly average of 146 μg/m3 during the study week BQut. |
T194 | 19401-19584 | Sentence | denotes | During the quarantine season and with the prohibition of vehicles and the closure of all stores except food, the PM2.5 concentration was reduced by 35%, going to a moderate AQI level. |
T195 | 19586-19612 | Sentence | denotes | 5 Results and discussions |
T196 | 19613-19720 | Sentence | denotes | The state of air quality is based on the environmental monitoring stations that are available in each city. |
T197 | 19721-19834 | Sentence | denotes | These stations determine the hourly concentration of air pollutant particles, including PM2.5 and PM10 particles. |
T198 | 19835-19950 | Sentence | denotes | According to the WHO (World Health Organization, 2018), air pollution represents a major environmental health risk. |
T199 | 19951-20162 | Sentence | denotes | For this reason, it is so important to have air quality monitored in cities, essentially in capital cities, and in this case, it is more important to monitor PM2.5 due to the risks explained in the introduction. |
T200 | 20163-20331 | Sentence | denotes | Therefore, it is essential to know how environmental control of capitals is, for this, it is essential to know the number of meteorological stations that measure PM2.5. |
T201 | 20333-20410 | Sentence | denotes | 5.1 Air quality stations vs. population of the most polluted capitals cities |
T202 | 20411-20554 | Sentence | denotes | Fig. 6 presents the relationship between quantity of inhabitants per one (Pp) environmental station (ES) is presented. (Pp/ES) in each capital. |
T203 | 20555-20616 | Sentence | denotes | It is reflected by a range of colors applied to each capital. |
T204 | 20617-20828 | Sentence | denotes | The main cities where greater control of air quality is observed, measured according to the Pp/ES ratio, are registered in quartile 2 (Q2) and quartile 3 (Q3), with a dark and light blue color, the which are: i. |
T205 | 20829-20916 | Sentence | denotes | Tel Aviv, Brussels and Pristina, these capitals present an average of 61,926 Pp/ES. ii. |
T206 | 20917-20984 | Sentence | denotes | Bratislavia, Bern, Sarajevo, present a ratio of 126,317 Pp/ES. iii. |
T207 | 20985-21028 | Sentence | denotes | Vienna, and Ulaanbaatar, 170,930 Pp/ES. iv. |
T208 | 21029-21099 | Sentence | denotes | Budapest, Lisbon and Prague present an average ratio of 244,696 Pp/ES. |
T209 | 21100-21219 | Sentence | denotes | Capitals that register between 300,000 Pp/ES and 500,000 Pp/ES, are registered between quartile (Q1) and quartile (Q3). |
T210 | 21220-21330 | Sentence | denotes | In Q1, the capitals London and Bangkok are observed, which present populations and stations above the average. |
T211 | 21331-21375 | Sentence | denotes | Fig. 6 Population vs Environmental stations. |
T212 | 21376-21502 | Sentence | denotes | In Q3 are the capital cities Skopje, Kathmandu, Paris and Belgrade, who register a population and stations lower than average. |
T213 | 21503-21648 | Sentence | denotes | The capitals with a registry between 500,000 Pp/ES and 700,000 Pp/ES are Bogota, Tehran, Tokyo, Vilnues, Kyiv, Warsaw, Kubait City, and Santiago. |
T214 | 21649-21741 | Sentence | denotes | Finally, from 700,000 Pp/ES to 1,000,000 Pp/ES, are Colombo, Ankara, Mexico City and Cyprus. |
T215 | 21742-21797 | Sentence | denotes | These capitals cities are identified between Q1 and Q3. |
T216 | 21798-21921 | Sentence | denotes | In Q4 the capitals with a low number of stations measuring PM2.5 can be seen, due to the number of inhabitants of each one. |
T217 | 21922-21984 | Sentence | denotes | Above 3,000,000 Jakarta, Hanoi, Lima and Dhaka are identified. |
T218 | 21985-22080 | Sentence | denotes | Lima has several weather stations, however, there is only one station that PM2.5 register data. |
T219 | 22081-22223 | Sentence | denotes | The cities with the highest pollution, Delhi (India) and Beijing (China), present a ratio of 1,766,333 Pp/ES and 1,227,059 Pp/ES respectively. |
T220 | 22224-22545 | Sentence | denotes | Although meteorological stations are installed based on population, it is observed that countries such as Singapore, Hanoi, Kakarta and Dhaka with populations over 5,000,000, have a number of below-average meteorological stations, which makes monitoring difficult and not It allows to have data of the real contamination. |
T221 | 22546-22691 | Sentence | denotes | Likewise, it should be considered that the analysis of any study is oriented to an economic system that restricts the conditions of each country. |
T222 | 22692-22889 | Sentence | denotes | In this case, when analyzed by continents and population, Europe with a GDP higher than the South American or Asian economies, presents a greater boost in reducing emissions of polluting particles. |
T223 | 22890-23001 | Sentence | denotes | This, indicating that the continent has a good AQI index and an optimal Pp/ES ratio for environmental analysis. |
T224 | 23002-23152 | Sentence | denotes | Their concern for the environment and compliance with the Kyoto protocol and the commitments to COP21 are reflected in their environmental indicators. |
T225 | 23153-23311 | Sentence | denotes | This continent recognizes that air quality influences labor productivity, investments in healthcare expenses and improvement in quality of life, among others. |
T226 | 23312-23556 | Sentence | denotes | In contrast, the Asian continent, with an unfavorable view compared to other continents, it is observed that the Asian economies are the main contributors to environmental pollution, which is why it attributes to greater inequality and poverty. |
T227 | 23557-23731 | Sentence | denotes | This allows linking to this study (Fig. 6) where a low update is observed in technologies that monitor the quality air in Asian cities, this reflected in the low Pp/Es ratio. |
T228 | 23732-23864 | Sentence | denotes | This indicates the low commitment to improve the life quality and the low incentives to reduce the cost attributed by air pollution. |
T229 | 23865-23982 | Sentence | denotes | In general, the most densified in population cities reflect PM2.5 contamination high with rates higher than 50 μg/m3. |
T230 | 23984-24004 | Sentence | denotes | 5.2 Global analysis |
T231 | 24005-24129 | Sentence | denotes | Making a global balance of the analyzed countries, the variation of the PM2.5 concentration had an average reduction of 12%. |
T232 | 24130-24395 | Sentence | denotes | The highest reduction occurs in the African continent with one (1) country analyzed (33%), followed by the American continent (22%) and the Asian continent (16%); finally, the European continent, in which a favorable reduction result is not generally observed (5%). |
T233 | 24396-24463 | Sentence | denotes | Fig. 7 shows the PM2.5 variation in a global map of capital cities. |
T234 | 24464-24540 | Sentence | denotes | The absolute value of the variation can be identified by size of the circle. |
T235 | 24541-24785 | Sentence | denotes | In red we find the cities with increases in their PM2.5 measurement and in pink the cities with PM2.5 reduction, the city with the greatest PM2.5 reduction within the data collected is Bogota, with a reduction of 57% compared to a typical week. |
T236 | 24786-24828 | Sentence | denotes | Fig. 7 PM2.5 Reduction in quarantine week. |
T237 | 24829-24943 | Sentence | denotes | Fig. 8 shows in detail the PM2.5 quantitative variation of the analyzed cities, as well as its mean concentration. |
T238 | 24944-25072 | Sentence | denotes | The gray color represents the PM2.5 behavior under typical conditions, and the light blue color represents the confinement mode. |
T239 | 25073-25154 | Sentence | denotes | Dhaka, the most polluted capital of this particulate matter, had a 14% reduction. |
T240 | 25155-25392 | Sentence | denotes | It is observed as the first seventeen (17) most contaminated capitals in the world, from Dhaka, to Skopje, exceed the PM2.5 average concentrations (75.78 μg/m3) from before quarantine, maintaining an AQI level from moderate to unhealthy. |
T241 | 25393-25543 | Sentence | denotes | Likewise, during typical times twelve (12) countries present an AQI level below the quarantine average (Bern - Kyiv) almost all with a good AQI level. |
T242 | 25544-25719 | Sentence | denotes | During the quarantine, sixteen (16) capitals exceed the PM2.5 registered average (66.92%), equivalent to a moderate AQI level, and nine (9) cities registered a good AQI level. |
T243 | 25720-25887 | Sentence | denotes | Specifically, the three most polluted capital cities that are Dhaka, followed by Kampala and Delhi, reduced their PM2.5 concentration by 14%, 35% and 40% respectively. |
T244 | 25888-26036 | Sentence | denotes | The capital city with the highest PM2.5 reduction during quarantine was Bogotá, with a percentage of 57%, going from a moderate to a good AQI level. |
T245 | 26037-26100 | Sentence | denotes | Kubait City, presents the second largest PM2.5 reduction (42%). |
T246 | 26101-26177 | Sentence | denotes | Finally, with reductions over 40%, there are the cities of Delhi and Tehran. |
T247 | 26178-26253 | Sentence | denotes | Fig. 8 Weekly average without quarantine vs weekly average with quarantine. |
T248 | 26254-26516 | Sentence | denotes | In Europe, the continent with the best environmental conditions in normal times, a very high increase in PM2.5 is observed in the cities of Prague, Vienna and Bratislava, cities very close to each other; apparently, an isolated effect produced by winds or fires. |
T249 | 26518-26532 | Sentence | denotes | 6 Conclusions |
T250 | 26533-26804 | Sentence | denotes | Based on the data collection of the concentration of the most harmful particles for health (PM2.5), in the different capital cities of the world, a comparative analysis of the concentration was carried out during a typical time of normal mobility and during the lockdown. |
T251 | 26805-26969 | Sentence | denotes | The behavior patterns show as a result a decrease in their concentration during the confinement season, favorably restoring the air quality of most cities analyzed. |
T252 | 26970-27246 | Sentence | denotes | If these data are subsequently correlated with the activities stopped during the confinement of each city, one can think of public policies that promote new socio-ecological models, as well as coherent environmental policies in these adaptive environments that are the cities. |
T253 | 27247-27388 | Sentence | denotes | In general, the results showed that automobile demobilization and factory shutdowns play an important role in reducing pollution in capitals. |
T254 | 27389-27647 | Sentence | denotes | For the fifty countries analyzed, Bogotá (Colombia)(Insider, 2019), as one of the cities with the most traffic in the world, with a 65% concentration of traffic during the day, presents the PM2.5 greatest reduction. during the period of confinement with 57%. |
T255 | 27648-27768 | Sentence | denotes | Likewise, Delhi (India), the most polluted capital city in the world, presents a decrease in PM2.5 contamination of 40%. |
T256 | 27769-27818 | Sentence | denotes | Some specific conclusions of the research are: i. |
T257 | 27819-27993 | Sentence | denotes | During lockdown, Europe maintains a Good AQI level of less than 50 μg/m3, followed by America with a Moderate AQI level (57 μg/m3), Asia (82 μg/m3) and Africa (95 μg/m3). ii. |
T258 | 27994-28181 | Sentence | denotes | America presented the highest distinction in the PM2.5 decrease air pollution. between a typical period of conventional mobility and a period of confinement, with a reduction of 22%. iii. |
T259 | 28182-28357 | Sentence | denotes | The capitals that in typical days (BQut) present a moderate AQI level and which presented a PM2.5 decrease between 60% and 20% during the period of confinement (Qut), such as: |
T260 | 28358-28564 | Sentence | denotes | Bogotá, Kubait City, Delhi, Tehran, Taskhkent, Ulaanbaatar, kabul and Colombo; They must present alternatives to reduce the displacement of fossil fuel vehicles and stricter regulations for their factories. |
T261 | 28565-28769 | Sentence | denotes | This study considered the relationship between population and Pp/ES air quality stations, where the number of stations per inhabitant is obviously higher in developed countries than in emerging countries. |
T262 | 28770-29036 | Sentence | denotes | Therefore, it is important to consider that the lack of access to data on air pollution, especially in emerging countries and where the use of public and private transport systems is high, causes errors or alterations in real information on the state of air quality. |
T263 | 29038-29071 | Sentence | denotes | Declaration of competing interest |
T264 | 29072-29242 | Sentence | denotes | The authors declare that they have no known competing financial interests or personal relationships that could have appeared to influence the work reported in this paper. |
LitCovid-PD-HP
Id | Subject | Object | Predicate | Lexical cue | hp_id |
---|---|---|---|---|---|
T3 | 2477-2483 | Phenotype | denotes | stroke | http://purl.obolibrary.org/obo/HP_0001297 |
T4 | 2485-2496 | Phenotype | denotes | lung cancer | http://purl.obolibrary.org/obo/HP_0100526 |
T5 | 2498-2535 | Phenotype | denotes | chronic obstructive pulmonary disease | http://purl.obolibrary.org/obo/HP_0006510 |
T6 | 2555-2577 | Phenotype | denotes | respiratory infections | http://purl.obolibrary.org/obo/HP_0011947 |
T7 | 2586-2595 | Phenotype | denotes | pneumonia | http://purl.obolibrary.org/obo/HP_0002090 |
T8 | 7105-7114 | Phenotype | denotes | paralysis | http://purl.obolibrary.org/obo/HP_0003470 |
MyTest
Id | Subject | Object | Predicate | Lexical cue |
---|---|---|---|---|
32650158-30690371-28447745 | 444-448 | 30690371 | denotes | 2019 |
32650158-30841411-28447746 | 652-656 | 30841411 | denotes | 2019 |
32650158-22572115-28447747 | 1124-1128 | 22572115 | denotes | 2012 |
32650158-28408086-28447748 | 2219-2223 | 28408086 | denotes | 2017 |
32650158-24333687-28447749 | 2613-2617 | 24333687 | denotes | 2014 |
32650158-28431315-28447750 | 2632-2636 | 28431315 | denotes | 2017 |
32650158-31185348-28447751 | 2651-2655 | 31185348 | denotes | 2019 |
32650158-31950516-28447752 | 3185-3189 | 31950516 | denotes | 2020 |
32650158-32334164-28447753 | 4823-4827 | 32334164 | denotes | 2020 |
32650158-32335404-28447754 | 5189-5193 | 32335404 | denotes | 2020 |
32650158-32302812-28447755 | 6177-6181 | 32302812 | denotes | 2020 |
2_test
Id | Subject | Object | Predicate | Lexical cue |
---|---|---|---|---|
32650158-30690371-28447745 | 444-448 | 30690371 | denotes | 2019 |
32650158-30841411-28447746 | 652-656 | 30841411 | denotes | 2019 |
32650158-22572115-28447747 | 1124-1128 | 22572115 | denotes | 2012 |
32650158-28408086-28447748 | 2219-2223 | 28408086 | denotes | 2017 |
32650158-24333687-28447749 | 2613-2617 | 24333687 | denotes | 2014 |
32650158-28431315-28447750 | 2632-2636 | 28431315 | denotes | 2017 |
32650158-31185348-28447751 | 2651-2655 | 31185348 | denotes | 2019 |
32650158-31950516-28447752 | 3185-3189 | 31950516 | denotes | 2020 |
32650158-32334164-28447753 | 4823-4827 | 32334164 | denotes | 2020 |
32650158-32335404-28447754 | 5189-5193 | 32335404 | denotes | 2020 |
32650158-32302812-28447755 | 6177-6181 | 32302812 | denotes | 2020 |