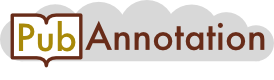
PMC:7216275 / 29025-40269
Annnotations
LitCovid-PubTator
{"project":"LitCovid-PubTator","denotations":[{"id":"293","span":{"begin":7627,"end":7630},"obj":"Gene"},{"id":"294","span":{"begin":7632,"end":7635},"obj":"Gene"},{"id":"295","span":{"begin":7641,"end":7644},"obj":"Gene"},{"id":"296","span":{"begin":7153,"end":7162},"obj":"Species"},{"id":"297","span":{"begin":7557,"end":7566},"obj":"Species"},{"id":"305","span":{"begin":7743,"end":7746},"obj":"Gene"},{"id":"306","span":{"begin":7753,"end":7756},"obj":"Gene"},{"id":"307","span":{"begin":7762,"end":7765},"obj":"Gene"},{"id":"308","span":{"begin":8268,"end":8271},"obj":"Gene"},{"id":"309","span":{"begin":8657,"end":8660},"obj":"Gene"},{"id":"310","span":{"begin":8743,"end":8752},"obj":"Species"},{"id":"311","span":{"begin":8954,"end":8963},"obj":"Species"},{"id":"317","span":{"begin":9456,"end":9459},"obj":"Gene"},{"id":"318","span":{"begin":9506,"end":9509},"obj":"Gene"},{"id":"319","span":{"begin":9546,"end":9555},"obj":"Species"},{"id":"320","span":{"begin":9764,"end":9773},"obj":"Species"},{"id":"321","span":{"begin":9885,"end":9894},"obj":"Species"},{"id":"324","span":{"begin":10145,"end":10149},"obj":"Chemical"},{"id":"325","span":{"begin":10245,"end":10249},"obj":"Chemical"},{"id":"330","span":{"begin":10510,"end":10514},"obj":"Chemical"},{"id":"331","span":{"begin":10516,"end":10520},"obj":"Chemical"},{"id":"332","span":{"begin":10698,"end":10702},"obj":"Chemical"},{"id":"333","span":{"begin":10733,"end":10737},"obj":"Chemical"}],"attributes":[{"id":"A293","pred":"tao:has_database_id","subj":"293","obj":"Gene:3009"},{"id":"A294","pred":"tao:has_database_id","subj":"294","obj":"Gene:8349"},{"id":"A295","pred":"tao:has_database_id","subj":"295","obj":"Gene:8352"},{"id":"A296","pred":"tao:has_database_id","subj":"296","obj":"Tax:2697049"},{"id":"A297","pred":"tao:has_database_id","subj":"297","obj":"Tax:2697049"},{"id":"A305","pred":"tao:has_database_id","subj":"305","obj":"Gene:8360"},{"id":"A306","pred":"tao:has_database_id","subj":"306","obj":"Gene:8352"},{"id":"A307","pred":"tao:has_database_id","subj":"307","obj":"Gene:8361"},{"id":"A308","pred":"tao:has_database_id","subj":"308","obj":"Gene:8360"},{"id":"A309","pred":"tao:has_database_id","subj":"309","obj":"Gene:8360"},{"id":"A310","pred":"tao:has_database_id","subj":"310","obj":"Tax:2697049"},{"id":"A311","pred":"tao:has_database_id","subj":"311","obj":"Tax:2697049"},{"id":"A317","pred":"tao:has_database_id","subj":"317","obj":"Gene:8352"},{"id":"A318","pred":"tao:has_database_id","subj":"318","obj":"Gene:8361"},{"id":"A319","pred":"tao:has_database_id","subj":"319","obj":"Tax:2697049"},{"id":"A320","pred":"tao:has_database_id","subj":"320","obj":"Tax:2697049"},{"id":"A321","pred":"tao:has_database_id","subj":"321","obj":"Tax:2697049"}],"namespaces":[{"prefix":"Tax","uri":"https://www.ncbi.nlm.nih.gov/taxonomy/"},{"prefix":"MESH","uri":"https://id.nlm.nih.gov/mesh/"},{"prefix":"Gene","uri":"https://www.ncbi.nlm.nih.gov/gene/"},{"prefix":"CVCL","uri":"https://web.expasy.org/cellosaurus/CVCL_"}],"text":"4. Results\n\n4.1. Measurement Model Assessment\nTo assess our measurement model, we verified the concurrent validity, discriminate validity, and composite reliability (CR) [77]. In addition to the square root values of the average variance extracted (AVE), we compared constructs to determine the discriminate validity [78]. Factor loading values should be greater than 0.70 [79]. For data validity and measurement, the value of the AVE should be greater than 0.5 [80], CR \u003e 0.7 [78], and rho \u003e 0.7 [81]. Table 3 provides the reliability and validity of our measurement scales and Table 4 provides the results of the Fornell–Larcker test used to check distinguished and divergent validity.\n\n4.2. Structured Model Assessment\nTo create intervals of confidence and t-values, we used bootstrapping (4000 re samples) to check for imagined associations between the concerned constructs of the planned structured model. Streukens, S., et al. [82] stated that bootstrap replications can vary considerably from a minimum of 500 to a maximum of 5000.In other words, statistic inconsistency is checked using the inconsistency of data using bootstrapping, which is a nonparametric resampling method, instead of using parametric statements to check the accuracy of approximation [83]. Efron, B., et al. [84] proposed using more than 1000 bootstrap samples.\nThe mediation effect is absent if the direct effect is not significant. Figure 1 illustrates the hypotheses testing of direct effects, which are also shown in Table 3. Table 3 also provides the fit statistics. Dependent variables indicate an important and positive precursor to their independent variables. Particularly, the role of E-Govt was a noteworthy predictor of epidemic protection. As can be seen from Figure 4 and Table 5, all of the hypotheses were supported [85] for the direct effect hypothesis at this step.\nTo check the importance of the structural path coefficients, we report the confidence interval [86]. These were supported because we did not add up zero values in any confidence interval [78]. At present, in standardized root mean square residual (SRMR) PLS path modeling, mostly model fit criteria are used. We checked the accuracy of the fit by using different tools like value of normed fit index (NFI), the non-normed fit index (NNFI), the comparative fit index (CFI), root mean square error of approximation (RMSEA), and SRMR. Values equal to or higher than 0.95 in NFI, NNFI, and CFI indicate the best fit. Sufficient adjustment was represented by RMSEA and SRMR with values less than 0.06 [87]. For comparatively good fit between the hypothesized model and observed data, a cut-off value near to 0.08 for SRMR and near 0.06 is best for RMSEA; Hu, L.T. et al. [88] stated that a zero value for SRMR indicates an ideal fit but if the value is smaller than 0.05,the fit is satisfactory fit [89]. We investigated the standards of the coefficient of determination (R2) to verify the predictive strength of our structure model. Collective consequences of exogenous contracts on endogenous constructs were indicated. The R2 of the endogenous latent variables is the vital decisive factor for this evaluation. This marker is used, from the perspective of a statistical model, to forecast future results or can be used to check the hypothesis on behalf of other related information. R2 also provides the results of the calculations and describes the practicality of the results [90]. Researchers can also use PLS procedure to check their model’s out-of-sample predictive power [91]. In-sample predictive power, we also refer to the R2 [92]. R2 varies from 0 to 1 and greater values show better descriptive power. Substantial, moderate, and weak descriptive powers are indicated by R2 values of 0.75, 0.50, and 0.25, respectively [93]. PLS-SEM is less dependent on the model fit concept compared to CB-SEM [79].\nAs recommended [74] RMSEA cut-off values equal to or less than 0.08 using modification in R2 report effect size (f2) indicated that the effect of our dependent variables on independent variables was very satisfactory.\n\n4.3. Effect Size and Predictive Relevance\nThe effect of the exogenous latent construct on the endogenous latent construct having three possible answers, i.e., substantial, moderate, and weak, was found using theF2 effect size. The blindfold method was used to check the strength of the research model. Cohen’s f2 is an identical measure of effect size that also permits checking the local effect size, which is the effect of one variable compared with the multivariate regression model [94]. If the cross-validated redundancy (Q2) is higher than 0,then the model is related to predicting that factor [95]. We focused on in-sample prediction more, compared to out-sample prediction, prognostic significance Q2, and relative relevance Q2, which are alternatives for evaluating a model’s practical relevance, in addition to consulting R2 outcomes as a gauge of a model’s predictive capabilities [95]. R2, Q2, path coefficients, and the effect size (f2) are the decisive factors we use for evaluation. In addition to this evaluation, researchers are required to check the inner model for potential co linearity issues. If the constructs are interrelated, then results approximated by the inner model are considered biased [96]. A model’s predictive accuracy is decided by the R2. The R2 value also characterizes the combined consequence of exogenous variables on the endogenous variable(s). The effect ranges from 0 to 1. A value of 1 indicates complete predictive accuracy as can see in Table 6.\nCohen’s f2 was calculated to check the effect size of each path model. When a construct was removed from the model, we calculated f2 while making no changes to R2. Researchers have to approximate two PLS path models for computing f2. The effect size of the omitted construct for a particular endogenous construct can be found by standard values: 0.02 for small, 0.15 for medium, and 0.35 for a large effect on the basis of the f2 value [96].\nThis discussion supports the use of our mediators and variables in the model.\n\n4.4. Multiple Mediating Effect Tests\nIn the PLS path model, mediator variables absorb the effect of an exogenous construct on an endogenous construct; this absorption of effect is known as meditation [96]. The mediation effect can be investigated using many tools, including PLS-SEM. Though researchers use an older method to determine the mediation effect in PLS-SEM, the procedure that identifies the effect of a precursor variable on the findings and results is judged by mediation; in other words, mediation considers transitional variables [97]. A mediating variable may have a transitional role in the association between dependent and independent variables, and engagement of this third variable is the important feature of the mediating effect [98]. Clarification and elaboration are the main effects of mediation [81]. As such, we included multi-mediation concepts in the results of social presence theory and for psychological aspects during outbreaks (see Table 7).\nThe results of the effects of exogenous variables (role of E-Govt and 2019-nCoV-WOM) on the endogenous variable (online social presence) with the mediating effect are provided in Table 7. Multiple mediation paths outcomes and potency (level) of mediation effects are demonstrated in Figure 5 and Figure 6. Precise indirect effects were investigated by bootstrapping techniques with the help of the bias correction technique. The two independent variables (role of E-Govt and2019-nCoV-WOM) affected online social presence; these effects support H1b, H2b, and H3c in that order, as mentioned above.\n\n4.5. Magnitude and Strength of Mediation\nTo determine whether H4b, H5b, H3c, and H4c are supported, we used the proposals by Hair et al. [85]. To finalize conclusions about the mediation effect; we calculated the amount and magnitude of mediation. We incorporated the variance accounted for (VAF) method to calculate the strength of mediation (Figure 5 and Figure 6). If VAF is less than 0.2, there is no mediation; if VAF is less than or equal to 0.8, there is a partial mediation; if VAF is greater than 0.8, there is full mediation. The magnitude and strength of epidemic protection (H4b: a1b1) and attitude toward epidemic outbreak (H5b: a2b2) mediated the association between the role of E-Govt and online social presence (Figure 5). We found via comparison that epidemic protection has a partial mediation effect on the role of E-Govt and online social presence because the VAF value was more than 0.2, which indicated that there is a partial mediation effect. As such, H4b is supported. Attitude toward epidemic outbreaks mediated the association between 2019-nCoV-WOM and online social presence; the VAF value was greater than 0.2, which indicated the presence of mediation. Therefore, we hypothesized imaginary harmonizing partial mediation because the effects of 2019-nCoV-WOMwere considerable both directly and indirectly and their products were positive [99].\nHair et al. [85] Concluded that complementary and competitive mediation can be differentiated if direct and indirect effects are more prominent. The condition in which direct and indirect effects work in the same direction is called complementary mediation. This means the outcome of the direct and indirect effect is positive. The magnitude and strength of the mediation effect of epidemic protection (H3c: a3b1) and attitude toward epidemic outbreak (H4c: a4b2) mediating the association of 2019-nCoV-WOMand online social presence is shown in Figure 6. In comparison, the VAF value was higher than 0.2, which indicated the presence and effect of mediation. Due to the prominent direct and indirect effects of 2019-nCoV-WOM, the complementary partial mediation was also positive. The comparison showed that the association between 2019-nCoV-WOM and online social presence was mediated by attitude toward epidemic outbreak. The VAF value was higher than 0.2, which indicated partial mediation, also supporting the multi-mediation hypothesis [99].\n\n4.6. Impact–Performance Map Analysis (IPMA)\nIPMA, also called impact–performance map or priority map analysis, is a useful approach in PLS-SEM. IPMA adds facets and measurements that consider the scores of latent variables reporting the path coefficient [99].\nApproaches to determining the role of precursor constructs and their significance for management actions are offered by the PLS-SEM studies based on IPMA. IPMA compares the significance and recital (performance of the variables) [99]. Analysis dimensions are used to demonstrate the results of path coefficient approximation extended by IPMA in Figure 7. The advantage of IPMA is the validation of total effects and a representation of their significance in a construct with an average score that indicates their performance. Our main purpose with the construct was to find the most significant component in the construct.\nOnline ratings are associated with higher enjoyment than negative reviews [100]. To the best of our knowledge, the broader tasks and household behaviors of the community and family members in social media and role of E-Govt have been relatively under-examined."}
LitCovid-PD-FMA-UBERON
{"project":"LitCovid-PD-FMA-UBERON","denotations":[{"id":"T21","span":{"begin":7632,"end":7635},"obj":"Body_part"},{"id":"T22","span":{"begin":7806,"end":7810},"obj":"Body_part"},{"id":"T23","span":{"begin":9053,"end":9057},"obj":"Body_part"},{"id":"T24","span":{"begin":10125,"end":10128},"obj":"Body_part"},{"id":"T25","span":{"begin":10182,"end":10185},"obj":"Body_part"},{"id":"T26","span":{"begin":10198,"end":10201},"obj":"Body_part"}],"attributes":[{"id":"A21","pred":"fma_id","subj":"T21","obj":"http://purl.org/sig/ont/fma/fma84130"},{"id":"A22","pred":"fma_id","subj":"T22","obj":"http://purl.org/sig/ont/fma/fma53667"},{"id":"A23","pred":"fma_id","subj":"T23","obj":"http://purl.org/sig/ont/fma/fma53667"},{"id":"A24","pred":"fma_id","subj":"T24","obj":"http://purl.org/sig/ont/fma/fma67847"},{"id":"A25","pred":"fma_id","subj":"T25","obj":"http://purl.org/sig/ont/fma/fma67847"},{"id":"A26","pred":"fma_id","subj":"T26","obj":"http://purl.org/sig/ont/fma/fma67847"}],"text":"4. Results\n\n4.1. Measurement Model Assessment\nTo assess our measurement model, we verified the concurrent validity, discriminate validity, and composite reliability (CR) [77]. In addition to the square root values of the average variance extracted (AVE), we compared constructs to determine the discriminate validity [78]. Factor loading values should be greater than 0.70 [79]. For data validity and measurement, the value of the AVE should be greater than 0.5 [80], CR \u003e 0.7 [78], and rho \u003e 0.7 [81]. Table 3 provides the reliability and validity of our measurement scales and Table 4 provides the results of the Fornell–Larcker test used to check distinguished and divergent validity.\n\n4.2. Structured Model Assessment\nTo create intervals of confidence and t-values, we used bootstrapping (4000 re samples) to check for imagined associations between the concerned constructs of the planned structured model. Streukens, S., et al. [82] stated that bootstrap replications can vary considerably from a minimum of 500 to a maximum of 5000.In other words, statistic inconsistency is checked using the inconsistency of data using bootstrapping, which is a nonparametric resampling method, instead of using parametric statements to check the accuracy of approximation [83]. Efron, B., et al. [84] proposed using more than 1000 bootstrap samples.\nThe mediation effect is absent if the direct effect is not significant. Figure 1 illustrates the hypotheses testing of direct effects, which are also shown in Table 3. Table 3 also provides the fit statistics. Dependent variables indicate an important and positive precursor to their independent variables. Particularly, the role of E-Govt was a noteworthy predictor of epidemic protection. As can be seen from Figure 4 and Table 5, all of the hypotheses were supported [85] for the direct effect hypothesis at this step.\nTo check the importance of the structural path coefficients, we report the confidence interval [86]. These were supported because we did not add up zero values in any confidence interval [78]. At present, in standardized root mean square residual (SRMR) PLS path modeling, mostly model fit criteria are used. We checked the accuracy of the fit by using different tools like value of normed fit index (NFI), the non-normed fit index (NNFI), the comparative fit index (CFI), root mean square error of approximation (RMSEA), and SRMR. Values equal to or higher than 0.95 in NFI, NNFI, and CFI indicate the best fit. Sufficient adjustment was represented by RMSEA and SRMR with values less than 0.06 [87]. For comparatively good fit between the hypothesized model and observed data, a cut-off value near to 0.08 for SRMR and near 0.06 is best for RMSEA; Hu, L.T. et al. [88] stated that a zero value for SRMR indicates an ideal fit but if the value is smaller than 0.05,the fit is satisfactory fit [89]. We investigated the standards of the coefficient of determination (R2) to verify the predictive strength of our structure model. Collective consequences of exogenous contracts on endogenous constructs were indicated. The R2 of the endogenous latent variables is the vital decisive factor for this evaluation. This marker is used, from the perspective of a statistical model, to forecast future results or can be used to check the hypothesis on behalf of other related information. R2 also provides the results of the calculations and describes the practicality of the results [90]. Researchers can also use PLS procedure to check their model’s out-of-sample predictive power [91]. In-sample predictive power, we also refer to the R2 [92]. R2 varies from 0 to 1 and greater values show better descriptive power. Substantial, moderate, and weak descriptive powers are indicated by R2 values of 0.75, 0.50, and 0.25, respectively [93]. PLS-SEM is less dependent on the model fit concept compared to CB-SEM [79].\nAs recommended [74] RMSEA cut-off values equal to or less than 0.08 using modification in R2 report effect size (f2) indicated that the effect of our dependent variables on independent variables was very satisfactory.\n\n4.3. Effect Size and Predictive Relevance\nThe effect of the exogenous latent construct on the endogenous latent construct having three possible answers, i.e., substantial, moderate, and weak, was found using theF2 effect size. The blindfold method was used to check the strength of the research model. Cohen’s f2 is an identical measure of effect size that also permits checking the local effect size, which is the effect of one variable compared with the multivariate regression model [94]. If the cross-validated redundancy (Q2) is higher than 0,then the model is related to predicting that factor [95]. We focused on in-sample prediction more, compared to out-sample prediction, prognostic significance Q2, and relative relevance Q2, which are alternatives for evaluating a model’s practical relevance, in addition to consulting R2 outcomes as a gauge of a model’s predictive capabilities [95]. R2, Q2, path coefficients, and the effect size (f2) are the decisive factors we use for evaluation. In addition to this evaluation, researchers are required to check the inner model for potential co linearity issues. If the constructs are interrelated, then results approximated by the inner model are considered biased [96]. A model’s predictive accuracy is decided by the R2. The R2 value also characterizes the combined consequence of exogenous variables on the endogenous variable(s). The effect ranges from 0 to 1. A value of 1 indicates complete predictive accuracy as can see in Table 6.\nCohen’s f2 was calculated to check the effect size of each path model. When a construct was removed from the model, we calculated f2 while making no changes to R2. Researchers have to approximate two PLS path models for computing f2. The effect size of the omitted construct for a particular endogenous construct can be found by standard values: 0.02 for small, 0.15 for medium, and 0.35 for a large effect on the basis of the f2 value [96].\nThis discussion supports the use of our mediators and variables in the model.\n\n4.4. Multiple Mediating Effect Tests\nIn the PLS path model, mediator variables absorb the effect of an exogenous construct on an endogenous construct; this absorption of effect is known as meditation [96]. The mediation effect can be investigated using many tools, including PLS-SEM. Though researchers use an older method to determine the mediation effect in PLS-SEM, the procedure that identifies the effect of a precursor variable on the findings and results is judged by mediation; in other words, mediation considers transitional variables [97]. A mediating variable may have a transitional role in the association between dependent and independent variables, and engagement of this third variable is the important feature of the mediating effect [98]. Clarification and elaboration are the main effects of mediation [81]. As such, we included multi-mediation concepts in the results of social presence theory and for psychological aspects during outbreaks (see Table 7).\nThe results of the effects of exogenous variables (role of E-Govt and 2019-nCoV-WOM) on the endogenous variable (online social presence) with the mediating effect are provided in Table 7. Multiple mediation paths outcomes and potency (level) of mediation effects are demonstrated in Figure 5 and Figure 6. Precise indirect effects were investigated by bootstrapping techniques with the help of the bias correction technique. The two independent variables (role of E-Govt and2019-nCoV-WOM) affected online social presence; these effects support H1b, H2b, and H3c in that order, as mentioned above.\n\n4.5. Magnitude and Strength of Mediation\nTo determine whether H4b, H5b, H3c, and H4c are supported, we used the proposals by Hair et al. [85]. To finalize conclusions about the mediation effect; we calculated the amount and magnitude of mediation. We incorporated the variance accounted for (VAF) method to calculate the strength of mediation (Figure 5 and Figure 6). If VAF is less than 0.2, there is no mediation; if VAF is less than or equal to 0.8, there is a partial mediation; if VAF is greater than 0.8, there is full mediation. The magnitude and strength of epidemic protection (H4b: a1b1) and attitude toward epidemic outbreak (H5b: a2b2) mediated the association between the role of E-Govt and online social presence (Figure 5). We found via comparison that epidemic protection has a partial mediation effect on the role of E-Govt and online social presence because the VAF value was more than 0.2, which indicated that there is a partial mediation effect. As such, H4b is supported. Attitude toward epidemic outbreaks mediated the association between 2019-nCoV-WOM and online social presence; the VAF value was greater than 0.2, which indicated the presence of mediation. Therefore, we hypothesized imaginary harmonizing partial mediation because the effects of 2019-nCoV-WOMwere considerable both directly and indirectly and their products were positive [99].\nHair et al. [85] Concluded that complementary and competitive mediation can be differentiated if direct and indirect effects are more prominent. The condition in which direct and indirect effects work in the same direction is called complementary mediation. This means the outcome of the direct and indirect effect is positive. The magnitude and strength of the mediation effect of epidemic protection (H3c: a3b1) and attitude toward epidemic outbreak (H4c: a4b2) mediating the association of 2019-nCoV-WOMand online social presence is shown in Figure 6. In comparison, the VAF value was higher than 0.2, which indicated the presence and effect of mediation. Due to the prominent direct and indirect effects of 2019-nCoV-WOM, the complementary partial mediation was also positive. The comparison showed that the association between 2019-nCoV-WOM and online social presence was mediated by attitude toward epidemic outbreak. The VAF value was higher than 0.2, which indicated partial mediation, also supporting the multi-mediation hypothesis [99].\n\n4.6. Impact–Performance Map Analysis (IPMA)\nIPMA, also called impact–performance map or priority map analysis, is a useful approach in PLS-SEM. IPMA adds facets and measurements that consider the scores of latent variables reporting the path coefficient [99].\nApproaches to determining the role of precursor constructs and their significance for management actions are offered by the PLS-SEM studies based on IPMA. IPMA compares the significance and recital (performance of the variables) [99]. Analysis dimensions are used to demonstrate the results of path coefficient approximation extended by IPMA in Figure 7. The advantage of IPMA is the validation of total effects and a representation of their significance in a construct with an average score that indicates their performance. Our main purpose with the construct was to find the most significant component in the construct.\nOnline ratings are associated with higher enjoyment than negative reviews [100]. To the best of our knowledge, the broader tasks and household behaviors of the community and family members in social media and role of E-Govt have been relatively under-examined."}
LitCovid-PD-UBERON
{"project":"LitCovid-PD-UBERON","denotations":[{"id":"T14","span":{"begin":568,"end":574},"obj":"Body_part"}],"attributes":[{"id":"A14","pred":"uberon_id","subj":"T14","obj":"http://purl.obolibrary.org/obo/UBERON_0002542"}],"text":"4. Results\n\n4.1. Measurement Model Assessment\nTo assess our measurement model, we verified the concurrent validity, discriminate validity, and composite reliability (CR) [77]. In addition to the square root values of the average variance extracted (AVE), we compared constructs to determine the discriminate validity [78]. Factor loading values should be greater than 0.70 [79]. For data validity and measurement, the value of the AVE should be greater than 0.5 [80], CR \u003e 0.7 [78], and rho \u003e 0.7 [81]. Table 3 provides the reliability and validity of our measurement scales and Table 4 provides the results of the Fornell–Larcker test used to check distinguished and divergent validity.\n\n4.2. Structured Model Assessment\nTo create intervals of confidence and t-values, we used bootstrapping (4000 re samples) to check for imagined associations between the concerned constructs of the planned structured model. Streukens, S., et al. [82] stated that bootstrap replications can vary considerably from a minimum of 500 to a maximum of 5000.In other words, statistic inconsistency is checked using the inconsistency of data using bootstrapping, which is a nonparametric resampling method, instead of using parametric statements to check the accuracy of approximation [83]. Efron, B., et al. [84] proposed using more than 1000 bootstrap samples.\nThe mediation effect is absent if the direct effect is not significant. Figure 1 illustrates the hypotheses testing of direct effects, which are also shown in Table 3. Table 3 also provides the fit statistics. Dependent variables indicate an important and positive precursor to their independent variables. Particularly, the role of E-Govt was a noteworthy predictor of epidemic protection. As can be seen from Figure 4 and Table 5, all of the hypotheses were supported [85] for the direct effect hypothesis at this step.\nTo check the importance of the structural path coefficients, we report the confidence interval [86]. These were supported because we did not add up zero values in any confidence interval [78]. At present, in standardized root mean square residual (SRMR) PLS path modeling, mostly model fit criteria are used. We checked the accuracy of the fit by using different tools like value of normed fit index (NFI), the non-normed fit index (NNFI), the comparative fit index (CFI), root mean square error of approximation (RMSEA), and SRMR. Values equal to or higher than 0.95 in NFI, NNFI, and CFI indicate the best fit. Sufficient adjustment was represented by RMSEA and SRMR with values less than 0.06 [87]. For comparatively good fit between the hypothesized model and observed data, a cut-off value near to 0.08 for SRMR and near 0.06 is best for RMSEA; Hu, L.T. et al. [88] stated that a zero value for SRMR indicates an ideal fit but if the value is smaller than 0.05,the fit is satisfactory fit [89]. We investigated the standards of the coefficient of determination (R2) to verify the predictive strength of our structure model. Collective consequences of exogenous contracts on endogenous constructs were indicated. The R2 of the endogenous latent variables is the vital decisive factor for this evaluation. This marker is used, from the perspective of a statistical model, to forecast future results or can be used to check the hypothesis on behalf of other related information. R2 also provides the results of the calculations and describes the practicality of the results [90]. Researchers can also use PLS procedure to check their model’s out-of-sample predictive power [91]. In-sample predictive power, we also refer to the R2 [92]. R2 varies from 0 to 1 and greater values show better descriptive power. Substantial, moderate, and weak descriptive powers are indicated by R2 values of 0.75, 0.50, and 0.25, respectively [93]. PLS-SEM is less dependent on the model fit concept compared to CB-SEM [79].\nAs recommended [74] RMSEA cut-off values equal to or less than 0.08 using modification in R2 report effect size (f2) indicated that the effect of our dependent variables on independent variables was very satisfactory.\n\n4.3. Effect Size and Predictive Relevance\nThe effect of the exogenous latent construct on the endogenous latent construct having three possible answers, i.e., substantial, moderate, and weak, was found using theF2 effect size. The blindfold method was used to check the strength of the research model. Cohen’s f2 is an identical measure of effect size that also permits checking the local effect size, which is the effect of one variable compared with the multivariate regression model [94]. If the cross-validated redundancy (Q2) is higher than 0,then the model is related to predicting that factor [95]. We focused on in-sample prediction more, compared to out-sample prediction, prognostic significance Q2, and relative relevance Q2, which are alternatives for evaluating a model’s practical relevance, in addition to consulting R2 outcomes as a gauge of a model’s predictive capabilities [95]. R2, Q2, path coefficients, and the effect size (f2) are the decisive factors we use for evaluation. In addition to this evaluation, researchers are required to check the inner model for potential co linearity issues. If the constructs are interrelated, then results approximated by the inner model are considered biased [96]. A model’s predictive accuracy is decided by the R2. The R2 value also characterizes the combined consequence of exogenous variables on the endogenous variable(s). The effect ranges from 0 to 1. A value of 1 indicates complete predictive accuracy as can see in Table 6.\nCohen’s f2 was calculated to check the effect size of each path model. When a construct was removed from the model, we calculated f2 while making no changes to R2. Researchers have to approximate two PLS path models for computing f2. The effect size of the omitted construct for a particular endogenous construct can be found by standard values: 0.02 for small, 0.15 for medium, and 0.35 for a large effect on the basis of the f2 value [96].\nThis discussion supports the use of our mediators and variables in the model.\n\n4.4. Multiple Mediating Effect Tests\nIn the PLS path model, mediator variables absorb the effect of an exogenous construct on an endogenous construct; this absorption of effect is known as meditation [96]. The mediation effect can be investigated using many tools, including PLS-SEM. Though researchers use an older method to determine the mediation effect in PLS-SEM, the procedure that identifies the effect of a precursor variable on the findings and results is judged by mediation; in other words, mediation considers transitional variables [97]. A mediating variable may have a transitional role in the association between dependent and independent variables, and engagement of this third variable is the important feature of the mediating effect [98]. Clarification and elaboration are the main effects of mediation [81]. As such, we included multi-mediation concepts in the results of social presence theory and for psychological aspects during outbreaks (see Table 7).\nThe results of the effects of exogenous variables (role of E-Govt and 2019-nCoV-WOM) on the endogenous variable (online social presence) with the mediating effect are provided in Table 7. Multiple mediation paths outcomes and potency (level) of mediation effects are demonstrated in Figure 5 and Figure 6. Precise indirect effects were investigated by bootstrapping techniques with the help of the bias correction technique. The two independent variables (role of E-Govt and2019-nCoV-WOM) affected online social presence; these effects support H1b, H2b, and H3c in that order, as mentioned above.\n\n4.5. Magnitude and Strength of Mediation\nTo determine whether H4b, H5b, H3c, and H4c are supported, we used the proposals by Hair et al. [85]. To finalize conclusions about the mediation effect; we calculated the amount and magnitude of mediation. We incorporated the variance accounted for (VAF) method to calculate the strength of mediation (Figure 5 and Figure 6). If VAF is less than 0.2, there is no mediation; if VAF is less than or equal to 0.8, there is a partial mediation; if VAF is greater than 0.8, there is full mediation. The magnitude and strength of epidemic protection (H4b: a1b1) and attitude toward epidemic outbreak (H5b: a2b2) mediated the association between the role of E-Govt and online social presence (Figure 5). We found via comparison that epidemic protection has a partial mediation effect on the role of E-Govt and online social presence because the VAF value was more than 0.2, which indicated that there is a partial mediation effect. As such, H4b is supported. Attitude toward epidemic outbreaks mediated the association between 2019-nCoV-WOM and online social presence; the VAF value was greater than 0.2, which indicated the presence of mediation. Therefore, we hypothesized imaginary harmonizing partial mediation because the effects of 2019-nCoV-WOMwere considerable both directly and indirectly and their products were positive [99].\nHair et al. [85] Concluded that complementary and competitive mediation can be differentiated if direct and indirect effects are more prominent. The condition in which direct and indirect effects work in the same direction is called complementary mediation. This means the outcome of the direct and indirect effect is positive. The magnitude and strength of the mediation effect of epidemic protection (H3c: a3b1) and attitude toward epidemic outbreak (H4c: a4b2) mediating the association of 2019-nCoV-WOMand online social presence is shown in Figure 6. In comparison, the VAF value was higher than 0.2, which indicated the presence and effect of mediation. Due to the prominent direct and indirect effects of 2019-nCoV-WOM, the complementary partial mediation was also positive. The comparison showed that the association between 2019-nCoV-WOM and online social presence was mediated by attitude toward epidemic outbreak. The VAF value was higher than 0.2, which indicated partial mediation, also supporting the multi-mediation hypothesis [99].\n\n4.6. Impact–Performance Map Analysis (IPMA)\nIPMA, also called impact–performance map or priority map analysis, is a useful approach in PLS-SEM. IPMA adds facets and measurements that consider the scores of latent variables reporting the path coefficient [99].\nApproaches to determining the role of precursor constructs and their significance for management actions are offered by the PLS-SEM studies based on IPMA. IPMA compares the significance and recital (performance of the variables) [99]. Analysis dimensions are used to demonstrate the results of path coefficient approximation extended by IPMA in Figure 7. The advantage of IPMA is the validation of total effects and a representation of their significance in a construct with an average score that indicates their performance. Our main purpose with the construct was to find the most significant component in the construct.\nOnline ratings are associated with higher enjoyment than negative reviews [100]. To the best of our knowledge, the broader tasks and household behaviors of the community and family members in social media and role of E-Govt have been relatively under-examined."}
2_test
{"project":"2_test","denotations":[{"id":"32344770-22529829-49254362","span":{"begin":4579,"end":4581},"obj":"22529829"},{"id":"32344770-13880271-49254363","span":{"begin":4693,"end":4695},"obj":"13880271"},{"id":"32344770-13880271-49254364","span":{"begin":4985,"end":4987},"obj":"13880271"},{"id":"T27469","span":{"begin":4579,"end":4581},"obj":"22529829"},{"id":"T61498","span":{"begin":4693,"end":4695},"obj":"13880271"},{"id":"T60861","span":{"begin":4985,"end":4987},"obj":"13880271"}],"text":"4. Results\n\n4.1. Measurement Model Assessment\nTo assess our measurement model, we verified the concurrent validity, discriminate validity, and composite reliability (CR) [77]. In addition to the square root values of the average variance extracted (AVE), we compared constructs to determine the discriminate validity [78]. Factor loading values should be greater than 0.70 [79]. For data validity and measurement, the value of the AVE should be greater than 0.5 [80], CR \u003e 0.7 [78], and rho \u003e 0.7 [81]. Table 3 provides the reliability and validity of our measurement scales and Table 4 provides the results of the Fornell–Larcker test used to check distinguished and divergent validity.\n\n4.2. Structured Model Assessment\nTo create intervals of confidence and t-values, we used bootstrapping (4000 re samples) to check for imagined associations between the concerned constructs of the planned structured model. Streukens, S., et al. [82] stated that bootstrap replications can vary considerably from a minimum of 500 to a maximum of 5000.In other words, statistic inconsistency is checked using the inconsistency of data using bootstrapping, which is a nonparametric resampling method, instead of using parametric statements to check the accuracy of approximation [83]. Efron, B., et al. [84] proposed using more than 1000 bootstrap samples.\nThe mediation effect is absent if the direct effect is not significant. Figure 1 illustrates the hypotheses testing of direct effects, which are also shown in Table 3. Table 3 also provides the fit statistics. Dependent variables indicate an important and positive precursor to their independent variables. Particularly, the role of E-Govt was a noteworthy predictor of epidemic protection. As can be seen from Figure 4 and Table 5, all of the hypotheses were supported [85] for the direct effect hypothesis at this step.\nTo check the importance of the structural path coefficients, we report the confidence interval [86]. These were supported because we did not add up zero values in any confidence interval [78]. At present, in standardized root mean square residual (SRMR) PLS path modeling, mostly model fit criteria are used. We checked the accuracy of the fit by using different tools like value of normed fit index (NFI), the non-normed fit index (NNFI), the comparative fit index (CFI), root mean square error of approximation (RMSEA), and SRMR. Values equal to or higher than 0.95 in NFI, NNFI, and CFI indicate the best fit. Sufficient adjustment was represented by RMSEA and SRMR with values less than 0.06 [87]. For comparatively good fit between the hypothesized model and observed data, a cut-off value near to 0.08 for SRMR and near 0.06 is best for RMSEA; Hu, L.T. et al. [88] stated that a zero value for SRMR indicates an ideal fit but if the value is smaller than 0.05,the fit is satisfactory fit [89]. We investigated the standards of the coefficient of determination (R2) to verify the predictive strength of our structure model. Collective consequences of exogenous contracts on endogenous constructs were indicated. The R2 of the endogenous latent variables is the vital decisive factor for this evaluation. This marker is used, from the perspective of a statistical model, to forecast future results or can be used to check the hypothesis on behalf of other related information. R2 also provides the results of the calculations and describes the practicality of the results [90]. Researchers can also use PLS procedure to check their model’s out-of-sample predictive power [91]. In-sample predictive power, we also refer to the R2 [92]. R2 varies from 0 to 1 and greater values show better descriptive power. Substantial, moderate, and weak descriptive powers are indicated by R2 values of 0.75, 0.50, and 0.25, respectively [93]. PLS-SEM is less dependent on the model fit concept compared to CB-SEM [79].\nAs recommended [74] RMSEA cut-off values equal to or less than 0.08 using modification in R2 report effect size (f2) indicated that the effect of our dependent variables on independent variables was very satisfactory.\n\n4.3. Effect Size and Predictive Relevance\nThe effect of the exogenous latent construct on the endogenous latent construct having three possible answers, i.e., substantial, moderate, and weak, was found using theF2 effect size. The blindfold method was used to check the strength of the research model. Cohen’s f2 is an identical measure of effect size that also permits checking the local effect size, which is the effect of one variable compared with the multivariate regression model [94]. If the cross-validated redundancy (Q2) is higher than 0,then the model is related to predicting that factor [95]. We focused on in-sample prediction more, compared to out-sample prediction, prognostic significance Q2, and relative relevance Q2, which are alternatives for evaluating a model’s practical relevance, in addition to consulting R2 outcomes as a gauge of a model’s predictive capabilities [95]. R2, Q2, path coefficients, and the effect size (f2) are the decisive factors we use for evaluation. In addition to this evaluation, researchers are required to check the inner model for potential co linearity issues. If the constructs are interrelated, then results approximated by the inner model are considered biased [96]. A model’s predictive accuracy is decided by the R2. The R2 value also characterizes the combined consequence of exogenous variables on the endogenous variable(s). The effect ranges from 0 to 1. A value of 1 indicates complete predictive accuracy as can see in Table 6.\nCohen’s f2 was calculated to check the effect size of each path model. When a construct was removed from the model, we calculated f2 while making no changes to R2. Researchers have to approximate two PLS path models for computing f2. The effect size of the omitted construct for a particular endogenous construct can be found by standard values: 0.02 for small, 0.15 for medium, and 0.35 for a large effect on the basis of the f2 value [96].\nThis discussion supports the use of our mediators and variables in the model.\n\n4.4. Multiple Mediating Effect Tests\nIn the PLS path model, mediator variables absorb the effect of an exogenous construct on an endogenous construct; this absorption of effect is known as meditation [96]. The mediation effect can be investigated using many tools, including PLS-SEM. Though researchers use an older method to determine the mediation effect in PLS-SEM, the procedure that identifies the effect of a precursor variable on the findings and results is judged by mediation; in other words, mediation considers transitional variables [97]. A mediating variable may have a transitional role in the association between dependent and independent variables, and engagement of this third variable is the important feature of the mediating effect [98]. Clarification and elaboration are the main effects of mediation [81]. As such, we included multi-mediation concepts in the results of social presence theory and for psychological aspects during outbreaks (see Table 7).\nThe results of the effects of exogenous variables (role of E-Govt and 2019-nCoV-WOM) on the endogenous variable (online social presence) with the mediating effect are provided in Table 7. Multiple mediation paths outcomes and potency (level) of mediation effects are demonstrated in Figure 5 and Figure 6. Precise indirect effects were investigated by bootstrapping techniques with the help of the bias correction technique. The two independent variables (role of E-Govt and2019-nCoV-WOM) affected online social presence; these effects support H1b, H2b, and H3c in that order, as mentioned above.\n\n4.5. Magnitude and Strength of Mediation\nTo determine whether H4b, H5b, H3c, and H4c are supported, we used the proposals by Hair et al. [85]. To finalize conclusions about the mediation effect; we calculated the amount and magnitude of mediation. We incorporated the variance accounted for (VAF) method to calculate the strength of mediation (Figure 5 and Figure 6). If VAF is less than 0.2, there is no mediation; if VAF is less than or equal to 0.8, there is a partial mediation; if VAF is greater than 0.8, there is full mediation. The magnitude and strength of epidemic protection (H4b: a1b1) and attitude toward epidemic outbreak (H5b: a2b2) mediated the association between the role of E-Govt and online social presence (Figure 5). We found via comparison that epidemic protection has a partial mediation effect on the role of E-Govt and online social presence because the VAF value was more than 0.2, which indicated that there is a partial mediation effect. As such, H4b is supported. Attitude toward epidemic outbreaks mediated the association between 2019-nCoV-WOM and online social presence; the VAF value was greater than 0.2, which indicated the presence of mediation. Therefore, we hypothesized imaginary harmonizing partial mediation because the effects of 2019-nCoV-WOMwere considerable both directly and indirectly and their products were positive [99].\nHair et al. [85] Concluded that complementary and competitive mediation can be differentiated if direct and indirect effects are more prominent. The condition in which direct and indirect effects work in the same direction is called complementary mediation. This means the outcome of the direct and indirect effect is positive. The magnitude and strength of the mediation effect of epidemic protection (H3c: a3b1) and attitude toward epidemic outbreak (H4c: a4b2) mediating the association of 2019-nCoV-WOMand online social presence is shown in Figure 6. In comparison, the VAF value was higher than 0.2, which indicated the presence and effect of mediation. Due to the prominent direct and indirect effects of 2019-nCoV-WOM, the complementary partial mediation was also positive. The comparison showed that the association between 2019-nCoV-WOM and online social presence was mediated by attitude toward epidemic outbreak. The VAF value was higher than 0.2, which indicated partial mediation, also supporting the multi-mediation hypothesis [99].\n\n4.6. Impact–Performance Map Analysis (IPMA)\nIPMA, also called impact–performance map or priority map analysis, is a useful approach in PLS-SEM. IPMA adds facets and measurements that consider the scores of latent variables reporting the path coefficient [99].\nApproaches to determining the role of precursor constructs and their significance for management actions are offered by the PLS-SEM studies based on IPMA. IPMA compares the significance and recital (performance of the variables) [99]. Analysis dimensions are used to demonstrate the results of path coefficient approximation extended by IPMA in Figure 7. The advantage of IPMA is the validation of total effects and a representation of their significance in a construct with an average score that indicates their performance. Our main purpose with the construct was to find the most significant component in the construct.\nOnline ratings are associated with higher enjoyment than negative reviews [100]. To the best of our knowledge, the broader tasks and household behaviors of the community and family members in social media and role of E-Govt have been relatively under-examined."}
LitCovid-PD-MONDO
{"project":"LitCovid-PD-MONDO","denotations":[{"id":"T35","span":{"begin":2931,"end":2933},"obj":"Disease"},{"id":"T36","span":{"begin":3085,"end":3087},"obj":"Disease"},{"id":"T37","span":{"begin":3345,"end":3347},"obj":"Disease"},{"id":"T38","span":{"begin":3594,"end":3596},"obj":"Disease"},{"id":"T39","span":{"begin":3603,"end":3605},"obj":"Disease"},{"id":"T40","span":{"begin":3743,"end":3745},"obj":"Disease"},{"id":"T41","span":{"begin":3963,"end":3965},"obj":"Disease"},{"id":"T42","span":{"begin":4924,"end":4926},"obj":"Disease"},{"id":"T43","span":{"begin":4990,"end":4992},"obj":"Disease"},{"id":"T44","span":{"begin":5364,"end":5366},"obj":"Disease"},{"id":"T45","span":{"begin":5372,"end":5374},"obj":"Disease"},{"id":"T46","span":{"begin":5745,"end":5747},"obj":"Disease"}],"attributes":[{"id":"A35","pred":"mondo_id","subj":"T35","obj":"http://purl.obolibrary.org/obo/MONDO_0019903"},{"id":"A36","pred":"mondo_id","subj":"T36","obj":"http://purl.obolibrary.org/obo/MONDO_0019903"},{"id":"A37","pred":"mondo_id","subj":"T37","obj":"http://purl.obolibrary.org/obo/MONDO_0019903"},{"id":"A38","pred":"mondo_id","subj":"T38","obj":"http://purl.obolibrary.org/obo/MONDO_0019903"},{"id":"A39","pred":"mondo_id","subj":"T39","obj":"http://purl.obolibrary.org/obo/MONDO_0019903"},{"id":"A40","pred":"mondo_id","subj":"T40","obj":"http://purl.obolibrary.org/obo/MONDO_0019903"},{"id":"A41","pred":"mondo_id","subj":"T41","obj":"http://purl.obolibrary.org/obo/MONDO_0019903"},{"id":"A42","pred":"mondo_id","subj":"T42","obj":"http://purl.obolibrary.org/obo/MONDO_0019903"},{"id":"A43","pred":"mondo_id","subj":"T43","obj":"http://purl.obolibrary.org/obo/MONDO_0019903"},{"id":"A44","pred":"mondo_id","subj":"T44","obj":"http://purl.obolibrary.org/obo/MONDO_0019903"},{"id":"A45","pred":"mondo_id","subj":"T45","obj":"http://purl.obolibrary.org/obo/MONDO_0019903"},{"id":"A46","pred":"mondo_id","subj":"T46","obj":"http://purl.obolibrary.org/obo/MONDO_0019903"}],"text":"4. Results\n\n4.1. Measurement Model Assessment\nTo assess our measurement model, we verified the concurrent validity, discriminate validity, and composite reliability (CR) [77]. In addition to the square root values of the average variance extracted (AVE), we compared constructs to determine the discriminate validity [78]. Factor loading values should be greater than 0.70 [79]. For data validity and measurement, the value of the AVE should be greater than 0.5 [80], CR \u003e 0.7 [78], and rho \u003e 0.7 [81]. Table 3 provides the reliability and validity of our measurement scales and Table 4 provides the results of the Fornell–Larcker test used to check distinguished and divergent validity.\n\n4.2. Structured Model Assessment\nTo create intervals of confidence and t-values, we used bootstrapping (4000 re samples) to check for imagined associations between the concerned constructs of the planned structured model. Streukens, S., et al. [82] stated that bootstrap replications can vary considerably from a minimum of 500 to a maximum of 5000.In other words, statistic inconsistency is checked using the inconsistency of data using bootstrapping, which is a nonparametric resampling method, instead of using parametric statements to check the accuracy of approximation [83]. Efron, B., et al. [84] proposed using more than 1000 bootstrap samples.\nThe mediation effect is absent if the direct effect is not significant. Figure 1 illustrates the hypotheses testing of direct effects, which are also shown in Table 3. Table 3 also provides the fit statistics. Dependent variables indicate an important and positive precursor to their independent variables. Particularly, the role of E-Govt was a noteworthy predictor of epidemic protection. As can be seen from Figure 4 and Table 5, all of the hypotheses were supported [85] for the direct effect hypothesis at this step.\nTo check the importance of the structural path coefficients, we report the confidence interval [86]. These were supported because we did not add up zero values in any confidence interval [78]. At present, in standardized root mean square residual (SRMR) PLS path modeling, mostly model fit criteria are used. We checked the accuracy of the fit by using different tools like value of normed fit index (NFI), the non-normed fit index (NNFI), the comparative fit index (CFI), root mean square error of approximation (RMSEA), and SRMR. Values equal to or higher than 0.95 in NFI, NNFI, and CFI indicate the best fit. Sufficient adjustment was represented by RMSEA and SRMR with values less than 0.06 [87]. For comparatively good fit between the hypothesized model and observed data, a cut-off value near to 0.08 for SRMR and near 0.06 is best for RMSEA; Hu, L.T. et al. [88] stated that a zero value for SRMR indicates an ideal fit but if the value is smaller than 0.05,the fit is satisfactory fit [89]. We investigated the standards of the coefficient of determination (R2) to verify the predictive strength of our structure model. Collective consequences of exogenous contracts on endogenous constructs were indicated. The R2 of the endogenous latent variables is the vital decisive factor for this evaluation. This marker is used, from the perspective of a statistical model, to forecast future results or can be used to check the hypothesis on behalf of other related information. R2 also provides the results of the calculations and describes the practicality of the results [90]. Researchers can also use PLS procedure to check their model’s out-of-sample predictive power [91]. In-sample predictive power, we also refer to the R2 [92]. R2 varies from 0 to 1 and greater values show better descriptive power. Substantial, moderate, and weak descriptive powers are indicated by R2 values of 0.75, 0.50, and 0.25, respectively [93]. PLS-SEM is less dependent on the model fit concept compared to CB-SEM [79].\nAs recommended [74] RMSEA cut-off values equal to or less than 0.08 using modification in R2 report effect size (f2) indicated that the effect of our dependent variables on independent variables was very satisfactory.\n\n4.3. Effect Size and Predictive Relevance\nThe effect of the exogenous latent construct on the endogenous latent construct having three possible answers, i.e., substantial, moderate, and weak, was found using theF2 effect size. The blindfold method was used to check the strength of the research model. Cohen’s f2 is an identical measure of effect size that also permits checking the local effect size, which is the effect of one variable compared with the multivariate regression model [94]. If the cross-validated redundancy (Q2) is higher than 0,then the model is related to predicting that factor [95]. We focused on in-sample prediction more, compared to out-sample prediction, prognostic significance Q2, and relative relevance Q2, which are alternatives for evaluating a model’s practical relevance, in addition to consulting R2 outcomes as a gauge of a model’s predictive capabilities [95]. R2, Q2, path coefficients, and the effect size (f2) are the decisive factors we use for evaluation. In addition to this evaluation, researchers are required to check the inner model for potential co linearity issues. If the constructs are interrelated, then results approximated by the inner model are considered biased [96]. A model’s predictive accuracy is decided by the R2. The R2 value also characterizes the combined consequence of exogenous variables on the endogenous variable(s). The effect ranges from 0 to 1. A value of 1 indicates complete predictive accuracy as can see in Table 6.\nCohen’s f2 was calculated to check the effect size of each path model. When a construct was removed from the model, we calculated f2 while making no changes to R2. Researchers have to approximate two PLS path models for computing f2. The effect size of the omitted construct for a particular endogenous construct can be found by standard values: 0.02 for small, 0.15 for medium, and 0.35 for a large effect on the basis of the f2 value [96].\nThis discussion supports the use of our mediators and variables in the model.\n\n4.4. Multiple Mediating Effect Tests\nIn the PLS path model, mediator variables absorb the effect of an exogenous construct on an endogenous construct; this absorption of effect is known as meditation [96]. The mediation effect can be investigated using many tools, including PLS-SEM. Though researchers use an older method to determine the mediation effect in PLS-SEM, the procedure that identifies the effect of a precursor variable on the findings and results is judged by mediation; in other words, mediation considers transitional variables [97]. A mediating variable may have a transitional role in the association between dependent and independent variables, and engagement of this third variable is the important feature of the mediating effect [98]. Clarification and elaboration are the main effects of mediation [81]. As such, we included multi-mediation concepts in the results of social presence theory and for psychological aspects during outbreaks (see Table 7).\nThe results of the effects of exogenous variables (role of E-Govt and 2019-nCoV-WOM) on the endogenous variable (online social presence) with the mediating effect are provided in Table 7. Multiple mediation paths outcomes and potency (level) of mediation effects are demonstrated in Figure 5 and Figure 6. Precise indirect effects were investigated by bootstrapping techniques with the help of the bias correction technique. The two independent variables (role of E-Govt and2019-nCoV-WOM) affected online social presence; these effects support H1b, H2b, and H3c in that order, as mentioned above.\n\n4.5. Magnitude and Strength of Mediation\nTo determine whether H4b, H5b, H3c, and H4c are supported, we used the proposals by Hair et al. [85]. To finalize conclusions about the mediation effect; we calculated the amount and magnitude of mediation. We incorporated the variance accounted for (VAF) method to calculate the strength of mediation (Figure 5 and Figure 6). If VAF is less than 0.2, there is no mediation; if VAF is less than or equal to 0.8, there is a partial mediation; if VAF is greater than 0.8, there is full mediation. The magnitude and strength of epidemic protection (H4b: a1b1) and attitude toward epidemic outbreak (H5b: a2b2) mediated the association between the role of E-Govt and online social presence (Figure 5). We found via comparison that epidemic protection has a partial mediation effect on the role of E-Govt and online social presence because the VAF value was more than 0.2, which indicated that there is a partial mediation effect. As such, H4b is supported. Attitude toward epidemic outbreaks mediated the association between 2019-nCoV-WOM and online social presence; the VAF value was greater than 0.2, which indicated the presence of mediation. Therefore, we hypothesized imaginary harmonizing partial mediation because the effects of 2019-nCoV-WOMwere considerable both directly and indirectly and their products were positive [99].\nHair et al. [85] Concluded that complementary and competitive mediation can be differentiated if direct and indirect effects are more prominent. The condition in which direct and indirect effects work in the same direction is called complementary mediation. This means the outcome of the direct and indirect effect is positive. The magnitude and strength of the mediation effect of epidemic protection (H3c: a3b1) and attitude toward epidemic outbreak (H4c: a4b2) mediating the association of 2019-nCoV-WOMand online social presence is shown in Figure 6. In comparison, the VAF value was higher than 0.2, which indicated the presence and effect of mediation. Due to the prominent direct and indirect effects of 2019-nCoV-WOM, the complementary partial mediation was also positive. The comparison showed that the association between 2019-nCoV-WOM and online social presence was mediated by attitude toward epidemic outbreak. The VAF value was higher than 0.2, which indicated partial mediation, also supporting the multi-mediation hypothesis [99].\n\n4.6. Impact–Performance Map Analysis (IPMA)\nIPMA, also called impact–performance map or priority map analysis, is a useful approach in PLS-SEM. IPMA adds facets and measurements that consider the scores of latent variables reporting the path coefficient [99].\nApproaches to determining the role of precursor constructs and their significance for management actions are offered by the PLS-SEM studies based on IPMA. IPMA compares the significance and recital (performance of the variables) [99]. Analysis dimensions are used to demonstrate the results of path coefficient approximation extended by IPMA in Figure 7. The advantage of IPMA is the validation of total effects and a representation of their significance in a construct with an average score that indicates their performance. Our main purpose with the construct was to find the most significant component in the construct.\nOnline ratings are associated with higher enjoyment than negative reviews [100]. To the best of our knowledge, the broader tasks and household behaviors of the community and family members in social media and role of E-Govt have been relatively under-examined."}
LitCovid-PD-CLO
{"project":"LitCovid-PD-CLO","denotations":[{"id":"T106","span":{"begin":487,"end":490},"obj":"http://purl.obolibrary.org/obo/CLO_0051142"},{"id":"T107","span":{"begin":631,"end":635},"obj":"http://purl.obolibrary.org/obo/UBERON_0000473"},{"id":"T108","span":{"begin":1000,"end":1001},"obj":"http://purl.obolibrary.org/obo/CLO_0001020"},{"id":"T109","span":{"begin":1020,"end":1021},"obj":"http://purl.obolibrary.org/obo/CLO_0001020"},{"id":"T110","span":{"begin":1151,"end":1152},"obj":"http://purl.obolibrary.org/obo/CLO_0001020"},{"id":"T111","span":{"begin":1277,"end":1278},"obj":"http://purl.obolibrary.org/obo/CLO_0001021"},{"id":"T112","span":{"begin":1450,"end":1457},"obj":"http://purl.obolibrary.org/obo/UBERON_0000473"},{"id":"T113","span":{"begin":1686,"end":1687},"obj":"http://purl.obolibrary.org/obo/CLO_0001020"},{"id":"T114","span":{"begin":2643,"end":2644},"obj":"http://purl.obolibrary.org/obo/CLO_0001020"},{"id":"T115","span":{"begin":2747,"end":2748},"obj":"http://purl.obolibrary.org/obo/CLO_0001020"},{"id":"T116","span":{"begin":3218,"end":3219},"obj":"http://purl.obolibrary.org/obo/CLO_0001020"},{"id":"T117","span":{"begin":3986,"end":3988},"obj":"http://purl.obolibrary.org/obo/CLO_0002972"},{"id":"T118","span":{"begin":4402,"end":4404},"obj":"http://purl.obolibrary.org/obo/CLO_0002972"},{"id":"T119","span":{"begin":4579,"end":4581},"obj":"http://purl.obolibrary.org/obo/CLO_0001527"},{"id":"T120","span":{"begin":4701,"end":4708},"obj":"http://purl.obolibrary.org/obo/CLO_0009985"},{"id":"T121","span":{"begin":4867,"end":4868},"obj":"http://purl.obolibrary.org/obo/CLO_0001020"},{"id":"T122","span":{"begin":4939,"end":4940},"obj":"http://purl.obolibrary.org/obo/CLO_0001020"},{"id":"T123","span":{"begin":4950,"end":4951},"obj":"http://purl.obolibrary.org/obo/CLO_0001020"},{"id":"T124","span":{"begin":5038,"end":5040},"obj":"http://purl.obolibrary.org/obo/CLO_0002972"},{"id":"T125","span":{"begin":5316,"end":5317},"obj":"http://purl.obolibrary.org/obo/CLO_0001020"},{"id":"T126","span":{"begin":5510,"end":5511},"obj":"http://purl.obolibrary.org/obo/CLO_0001020"},{"id":"T127","span":{"begin":5593,"end":5595},"obj":"http://purl.obolibrary.org/obo/CLO_0002972"},{"id":"T128","span":{"begin":5661,"end":5662},"obj":"http://purl.obolibrary.org/obo/CLO_0001020"},{"id":"T129","span":{"begin":5715,"end":5717},"obj":"http://purl.obolibrary.org/obo/CLO_0002972"},{"id":"T130","span":{"begin":5815,"end":5817},"obj":"http://purl.obolibrary.org/obo/CLO_0002972"},{"id":"T131","span":{"begin":5864,"end":5865},"obj":"http://purl.obolibrary.org/obo/CLO_0001020"},{"id":"T132","span":{"begin":5977,"end":5978},"obj":"http://purl.obolibrary.org/obo/CLO_0001020"},{"id":"T133","span":{"begin":6012,"end":6014},"obj":"http://purl.obolibrary.org/obo/CLO_0002972"},{"id":"T134","span":{"begin":6137,"end":6142},"obj":"http://purl.obolibrary.org/obo/UBERON_0000473"},{"id":"T135","span":{"begin":6519,"end":6520},"obj":"http://purl.obolibrary.org/obo/CLO_0001020"},{"id":"T136","span":{"begin":6657,"end":6658},"obj":"http://purl.obolibrary.org/obo/CLO_0001020"},{"id":"T137","span":{"begin":6687,"end":6688},"obj":"http://purl.obolibrary.org/obo/CLO_0001020"},{"id":"T138","span":{"begin":8143,"end":8144},"obj":"http://purl.obolibrary.org/obo/CLO_0001020"},{"id":"T139","span":{"begin":8469,"end":8472},"obj":"http://purl.obolibrary.org/obo/CLO_0051582"},{"id":"T140","span":{"begin":8473,"end":8474},"obj":"http://purl.obolibrary.org/obo/CLO_0001020"},{"id":"T141","span":{"begin":8620,"end":8621},"obj":"http://purl.obolibrary.org/obo/CLO_0001020"},{"id":"T142","span":{"begin":10215,"end":10216},"obj":"http://purl.obolibrary.org/obo/CLO_0001020"},{"id":"T143","span":{"begin":10777,"end":10778},"obj":"http://purl.obolibrary.org/obo/CLO_0001020"},{"id":"T144","span":{"begin":10819,"end":10820},"obj":"http://purl.obolibrary.org/obo/CLO_0001020"}],"text":"4. Results\n\n4.1. Measurement Model Assessment\nTo assess our measurement model, we verified the concurrent validity, discriminate validity, and composite reliability (CR) [77]. In addition to the square root values of the average variance extracted (AVE), we compared constructs to determine the discriminate validity [78]. Factor loading values should be greater than 0.70 [79]. For data validity and measurement, the value of the AVE should be greater than 0.5 [80], CR \u003e 0.7 [78], and rho \u003e 0.7 [81]. Table 3 provides the reliability and validity of our measurement scales and Table 4 provides the results of the Fornell–Larcker test used to check distinguished and divergent validity.\n\n4.2. Structured Model Assessment\nTo create intervals of confidence and t-values, we used bootstrapping (4000 re samples) to check for imagined associations between the concerned constructs of the planned structured model. Streukens, S., et al. [82] stated that bootstrap replications can vary considerably from a minimum of 500 to a maximum of 5000.In other words, statistic inconsistency is checked using the inconsistency of data using bootstrapping, which is a nonparametric resampling method, instead of using parametric statements to check the accuracy of approximation [83]. Efron, B., et al. [84] proposed using more than 1000 bootstrap samples.\nThe mediation effect is absent if the direct effect is not significant. Figure 1 illustrates the hypotheses testing of direct effects, which are also shown in Table 3. Table 3 also provides the fit statistics. Dependent variables indicate an important and positive precursor to their independent variables. Particularly, the role of E-Govt was a noteworthy predictor of epidemic protection. As can be seen from Figure 4 and Table 5, all of the hypotheses were supported [85] for the direct effect hypothesis at this step.\nTo check the importance of the structural path coefficients, we report the confidence interval [86]. These were supported because we did not add up zero values in any confidence interval [78]. At present, in standardized root mean square residual (SRMR) PLS path modeling, mostly model fit criteria are used. We checked the accuracy of the fit by using different tools like value of normed fit index (NFI), the non-normed fit index (NNFI), the comparative fit index (CFI), root mean square error of approximation (RMSEA), and SRMR. Values equal to or higher than 0.95 in NFI, NNFI, and CFI indicate the best fit. Sufficient adjustment was represented by RMSEA and SRMR with values less than 0.06 [87]. For comparatively good fit between the hypothesized model and observed data, a cut-off value near to 0.08 for SRMR and near 0.06 is best for RMSEA; Hu, L.T. et al. [88] stated that a zero value for SRMR indicates an ideal fit but if the value is smaller than 0.05,the fit is satisfactory fit [89]. We investigated the standards of the coefficient of determination (R2) to verify the predictive strength of our structure model. Collective consequences of exogenous contracts on endogenous constructs were indicated. The R2 of the endogenous latent variables is the vital decisive factor for this evaluation. This marker is used, from the perspective of a statistical model, to forecast future results or can be used to check the hypothesis on behalf of other related information. R2 also provides the results of the calculations and describes the practicality of the results [90]. Researchers can also use PLS procedure to check their model’s out-of-sample predictive power [91]. In-sample predictive power, we also refer to the R2 [92]. R2 varies from 0 to 1 and greater values show better descriptive power. Substantial, moderate, and weak descriptive powers are indicated by R2 values of 0.75, 0.50, and 0.25, respectively [93]. PLS-SEM is less dependent on the model fit concept compared to CB-SEM [79].\nAs recommended [74] RMSEA cut-off values equal to or less than 0.08 using modification in R2 report effect size (f2) indicated that the effect of our dependent variables on independent variables was very satisfactory.\n\n4.3. Effect Size and Predictive Relevance\nThe effect of the exogenous latent construct on the endogenous latent construct having three possible answers, i.e., substantial, moderate, and weak, was found using theF2 effect size. The blindfold method was used to check the strength of the research model. Cohen’s f2 is an identical measure of effect size that also permits checking the local effect size, which is the effect of one variable compared with the multivariate regression model [94]. If the cross-validated redundancy (Q2) is higher than 0,then the model is related to predicting that factor [95]. We focused on in-sample prediction more, compared to out-sample prediction, prognostic significance Q2, and relative relevance Q2, which are alternatives for evaluating a model’s practical relevance, in addition to consulting R2 outcomes as a gauge of a model’s predictive capabilities [95]. R2, Q2, path coefficients, and the effect size (f2) are the decisive factors we use for evaluation. In addition to this evaluation, researchers are required to check the inner model for potential co linearity issues. If the constructs are interrelated, then results approximated by the inner model are considered biased [96]. A model’s predictive accuracy is decided by the R2. The R2 value also characterizes the combined consequence of exogenous variables on the endogenous variable(s). The effect ranges from 0 to 1. A value of 1 indicates complete predictive accuracy as can see in Table 6.\nCohen’s f2 was calculated to check the effect size of each path model. When a construct was removed from the model, we calculated f2 while making no changes to R2. Researchers have to approximate two PLS path models for computing f2. The effect size of the omitted construct for a particular endogenous construct can be found by standard values: 0.02 for small, 0.15 for medium, and 0.35 for a large effect on the basis of the f2 value [96].\nThis discussion supports the use of our mediators and variables in the model.\n\n4.4. Multiple Mediating Effect Tests\nIn the PLS path model, mediator variables absorb the effect of an exogenous construct on an endogenous construct; this absorption of effect is known as meditation [96]. The mediation effect can be investigated using many tools, including PLS-SEM. Though researchers use an older method to determine the mediation effect in PLS-SEM, the procedure that identifies the effect of a precursor variable on the findings and results is judged by mediation; in other words, mediation considers transitional variables [97]. A mediating variable may have a transitional role in the association between dependent and independent variables, and engagement of this third variable is the important feature of the mediating effect [98]. Clarification and elaboration are the main effects of mediation [81]. As such, we included multi-mediation concepts in the results of social presence theory and for psychological aspects during outbreaks (see Table 7).\nThe results of the effects of exogenous variables (role of E-Govt and 2019-nCoV-WOM) on the endogenous variable (online social presence) with the mediating effect are provided in Table 7. Multiple mediation paths outcomes and potency (level) of mediation effects are demonstrated in Figure 5 and Figure 6. Precise indirect effects were investigated by bootstrapping techniques with the help of the bias correction technique. The two independent variables (role of E-Govt and2019-nCoV-WOM) affected online social presence; these effects support H1b, H2b, and H3c in that order, as mentioned above.\n\n4.5. Magnitude and Strength of Mediation\nTo determine whether H4b, H5b, H3c, and H4c are supported, we used the proposals by Hair et al. [85]. To finalize conclusions about the mediation effect; we calculated the amount and magnitude of mediation. We incorporated the variance accounted for (VAF) method to calculate the strength of mediation (Figure 5 and Figure 6). If VAF is less than 0.2, there is no mediation; if VAF is less than or equal to 0.8, there is a partial mediation; if VAF is greater than 0.8, there is full mediation. The magnitude and strength of epidemic protection (H4b: a1b1) and attitude toward epidemic outbreak (H5b: a2b2) mediated the association between the role of E-Govt and online social presence (Figure 5). We found via comparison that epidemic protection has a partial mediation effect on the role of E-Govt and online social presence because the VAF value was more than 0.2, which indicated that there is a partial mediation effect. As such, H4b is supported. Attitude toward epidemic outbreaks mediated the association between 2019-nCoV-WOM and online social presence; the VAF value was greater than 0.2, which indicated the presence of mediation. Therefore, we hypothesized imaginary harmonizing partial mediation because the effects of 2019-nCoV-WOMwere considerable both directly and indirectly and their products were positive [99].\nHair et al. [85] Concluded that complementary and competitive mediation can be differentiated if direct and indirect effects are more prominent. The condition in which direct and indirect effects work in the same direction is called complementary mediation. This means the outcome of the direct and indirect effect is positive. The magnitude and strength of the mediation effect of epidemic protection (H3c: a3b1) and attitude toward epidemic outbreak (H4c: a4b2) mediating the association of 2019-nCoV-WOMand online social presence is shown in Figure 6. In comparison, the VAF value was higher than 0.2, which indicated the presence and effect of mediation. Due to the prominent direct and indirect effects of 2019-nCoV-WOM, the complementary partial mediation was also positive. The comparison showed that the association between 2019-nCoV-WOM and online social presence was mediated by attitude toward epidemic outbreak. The VAF value was higher than 0.2, which indicated partial mediation, also supporting the multi-mediation hypothesis [99].\n\n4.6. Impact–Performance Map Analysis (IPMA)\nIPMA, also called impact–performance map or priority map analysis, is a useful approach in PLS-SEM. IPMA adds facets and measurements that consider the scores of latent variables reporting the path coefficient [99].\nApproaches to determining the role of precursor constructs and their significance for management actions are offered by the PLS-SEM studies based on IPMA. IPMA compares the significance and recital (performance of the variables) [99]. Analysis dimensions are used to demonstrate the results of path coefficient approximation extended by IPMA in Figure 7. The advantage of IPMA is the validation of total effects and a representation of their significance in a construct with an average score that indicates their performance. Our main purpose with the construct was to find the most significant component in the construct.\nOnline ratings are associated with higher enjoyment than negative reviews [100]. To the best of our knowledge, the broader tasks and household behaviors of the community and family members in social media and role of E-Govt have been relatively under-examined."}
LitCovid-PD-GO-BP
{"project":"LitCovid-PD-GO-BP","denotations":[{"id":"T6","span":{"begin":11127,"end":11136},"obj":"http://purl.obolibrary.org/obo/GO_0007610"}],"text":"4. Results\n\n4.1. Measurement Model Assessment\nTo assess our measurement model, we verified the concurrent validity, discriminate validity, and composite reliability (CR) [77]. In addition to the square root values of the average variance extracted (AVE), we compared constructs to determine the discriminate validity [78]. Factor loading values should be greater than 0.70 [79]. For data validity and measurement, the value of the AVE should be greater than 0.5 [80], CR \u003e 0.7 [78], and rho \u003e 0.7 [81]. Table 3 provides the reliability and validity of our measurement scales and Table 4 provides the results of the Fornell–Larcker test used to check distinguished and divergent validity.\n\n4.2. Structured Model Assessment\nTo create intervals of confidence and t-values, we used bootstrapping (4000 re samples) to check for imagined associations between the concerned constructs of the planned structured model. Streukens, S., et al. [82] stated that bootstrap replications can vary considerably from a minimum of 500 to a maximum of 5000.In other words, statistic inconsistency is checked using the inconsistency of data using bootstrapping, which is a nonparametric resampling method, instead of using parametric statements to check the accuracy of approximation [83]. Efron, B., et al. [84] proposed using more than 1000 bootstrap samples.\nThe mediation effect is absent if the direct effect is not significant. Figure 1 illustrates the hypotheses testing of direct effects, which are also shown in Table 3. Table 3 also provides the fit statistics. Dependent variables indicate an important and positive precursor to their independent variables. Particularly, the role of E-Govt was a noteworthy predictor of epidemic protection. As can be seen from Figure 4 and Table 5, all of the hypotheses were supported [85] for the direct effect hypothesis at this step.\nTo check the importance of the structural path coefficients, we report the confidence interval [86]. These were supported because we did not add up zero values in any confidence interval [78]. At present, in standardized root mean square residual (SRMR) PLS path modeling, mostly model fit criteria are used. We checked the accuracy of the fit by using different tools like value of normed fit index (NFI), the non-normed fit index (NNFI), the comparative fit index (CFI), root mean square error of approximation (RMSEA), and SRMR. Values equal to or higher than 0.95 in NFI, NNFI, and CFI indicate the best fit. Sufficient adjustment was represented by RMSEA and SRMR with values less than 0.06 [87]. For comparatively good fit between the hypothesized model and observed data, a cut-off value near to 0.08 for SRMR and near 0.06 is best for RMSEA; Hu, L.T. et al. [88] stated that a zero value for SRMR indicates an ideal fit but if the value is smaller than 0.05,the fit is satisfactory fit [89]. We investigated the standards of the coefficient of determination (R2) to verify the predictive strength of our structure model. Collective consequences of exogenous contracts on endogenous constructs were indicated. The R2 of the endogenous latent variables is the vital decisive factor for this evaluation. This marker is used, from the perspective of a statistical model, to forecast future results or can be used to check the hypothesis on behalf of other related information. R2 also provides the results of the calculations and describes the practicality of the results [90]. Researchers can also use PLS procedure to check their model’s out-of-sample predictive power [91]. In-sample predictive power, we also refer to the R2 [92]. R2 varies from 0 to 1 and greater values show better descriptive power. Substantial, moderate, and weak descriptive powers are indicated by R2 values of 0.75, 0.50, and 0.25, respectively [93]. PLS-SEM is less dependent on the model fit concept compared to CB-SEM [79].\nAs recommended [74] RMSEA cut-off values equal to or less than 0.08 using modification in R2 report effect size (f2) indicated that the effect of our dependent variables on independent variables was very satisfactory.\n\n4.3. Effect Size and Predictive Relevance\nThe effect of the exogenous latent construct on the endogenous latent construct having three possible answers, i.e., substantial, moderate, and weak, was found using theF2 effect size. The blindfold method was used to check the strength of the research model. Cohen’s f2 is an identical measure of effect size that also permits checking the local effect size, which is the effect of one variable compared with the multivariate regression model [94]. If the cross-validated redundancy (Q2) is higher than 0,then the model is related to predicting that factor [95]. We focused on in-sample prediction more, compared to out-sample prediction, prognostic significance Q2, and relative relevance Q2, which are alternatives for evaluating a model’s practical relevance, in addition to consulting R2 outcomes as a gauge of a model’s predictive capabilities [95]. R2, Q2, path coefficients, and the effect size (f2) are the decisive factors we use for evaluation. In addition to this evaluation, researchers are required to check the inner model for potential co linearity issues. If the constructs are interrelated, then results approximated by the inner model are considered biased [96]. A model’s predictive accuracy is decided by the R2. The R2 value also characterizes the combined consequence of exogenous variables on the endogenous variable(s). The effect ranges from 0 to 1. A value of 1 indicates complete predictive accuracy as can see in Table 6.\nCohen’s f2 was calculated to check the effect size of each path model. When a construct was removed from the model, we calculated f2 while making no changes to R2. Researchers have to approximate two PLS path models for computing f2. The effect size of the omitted construct for a particular endogenous construct can be found by standard values: 0.02 for small, 0.15 for medium, and 0.35 for a large effect on the basis of the f2 value [96].\nThis discussion supports the use of our mediators and variables in the model.\n\n4.4. Multiple Mediating Effect Tests\nIn the PLS path model, mediator variables absorb the effect of an exogenous construct on an endogenous construct; this absorption of effect is known as meditation [96]. The mediation effect can be investigated using many tools, including PLS-SEM. Though researchers use an older method to determine the mediation effect in PLS-SEM, the procedure that identifies the effect of a precursor variable on the findings and results is judged by mediation; in other words, mediation considers transitional variables [97]. A mediating variable may have a transitional role in the association between dependent and independent variables, and engagement of this third variable is the important feature of the mediating effect [98]. Clarification and elaboration are the main effects of mediation [81]. As such, we included multi-mediation concepts in the results of social presence theory and for psychological aspects during outbreaks (see Table 7).\nThe results of the effects of exogenous variables (role of E-Govt and 2019-nCoV-WOM) on the endogenous variable (online social presence) with the mediating effect are provided in Table 7. Multiple mediation paths outcomes and potency (level) of mediation effects are demonstrated in Figure 5 and Figure 6. Precise indirect effects were investigated by bootstrapping techniques with the help of the bias correction technique. The two independent variables (role of E-Govt and2019-nCoV-WOM) affected online social presence; these effects support H1b, H2b, and H3c in that order, as mentioned above.\n\n4.5. Magnitude and Strength of Mediation\nTo determine whether H4b, H5b, H3c, and H4c are supported, we used the proposals by Hair et al. [85]. To finalize conclusions about the mediation effect; we calculated the amount and magnitude of mediation. We incorporated the variance accounted for (VAF) method to calculate the strength of mediation (Figure 5 and Figure 6). If VAF is less than 0.2, there is no mediation; if VAF is less than or equal to 0.8, there is a partial mediation; if VAF is greater than 0.8, there is full mediation. The magnitude and strength of epidemic protection (H4b: a1b1) and attitude toward epidemic outbreak (H5b: a2b2) mediated the association between the role of E-Govt and online social presence (Figure 5). We found via comparison that epidemic protection has a partial mediation effect on the role of E-Govt and online social presence because the VAF value was more than 0.2, which indicated that there is a partial mediation effect. As such, H4b is supported. Attitude toward epidemic outbreaks mediated the association between 2019-nCoV-WOM and online social presence; the VAF value was greater than 0.2, which indicated the presence of mediation. Therefore, we hypothesized imaginary harmonizing partial mediation because the effects of 2019-nCoV-WOMwere considerable both directly and indirectly and their products were positive [99].\nHair et al. [85] Concluded that complementary and competitive mediation can be differentiated if direct and indirect effects are more prominent. The condition in which direct and indirect effects work in the same direction is called complementary mediation. This means the outcome of the direct and indirect effect is positive. The magnitude and strength of the mediation effect of epidemic protection (H3c: a3b1) and attitude toward epidemic outbreak (H4c: a4b2) mediating the association of 2019-nCoV-WOMand online social presence is shown in Figure 6. In comparison, the VAF value was higher than 0.2, which indicated the presence and effect of mediation. Due to the prominent direct and indirect effects of 2019-nCoV-WOM, the complementary partial mediation was also positive. The comparison showed that the association between 2019-nCoV-WOM and online social presence was mediated by attitude toward epidemic outbreak. The VAF value was higher than 0.2, which indicated partial mediation, also supporting the multi-mediation hypothesis [99].\n\n4.6. Impact–Performance Map Analysis (IPMA)\nIPMA, also called impact–performance map or priority map analysis, is a useful approach in PLS-SEM. IPMA adds facets and measurements that consider the scores of latent variables reporting the path coefficient [99].\nApproaches to determining the role of precursor constructs and their significance for management actions are offered by the PLS-SEM studies based on IPMA. IPMA compares the significance and recital (performance of the variables) [99]. Analysis dimensions are used to demonstrate the results of path coefficient approximation extended by IPMA in Figure 7. The advantage of IPMA is the validation of total effects and a representation of their significance in a construct with an average score that indicates their performance. Our main purpose with the construct was to find the most significant component in the construct.\nOnline ratings are associated with higher enjoyment than negative reviews [100]. To the best of our knowledge, the broader tasks and household behaviors of the community and family members in social media and role of E-Govt have been relatively under-examined."}
LitCovid-sentences
{"project":"LitCovid-sentences","denotations":[{"id":"T242","span":{"begin":0,"end":2},"obj":"Sentence"},{"id":"T243","span":{"begin":3,"end":10},"obj":"Sentence"},{"id":"T244","span":{"begin":12,"end":16},"obj":"Sentence"},{"id":"T245","span":{"begin":17,"end":45},"obj":"Sentence"},{"id":"T246","span":{"begin":46,"end":175},"obj":"Sentence"},{"id":"T247","span":{"begin":176,"end":322},"obj":"Sentence"},{"id":"T248","span":{"begin":323,"end":378},"obj":"Sentence"},{"id":"T249","span":{"begin":379,"end":502},"obj":"Sentence"},{"id":"T250","span":{"begin":503,"end":687},"obj":"Sentence"},{"id":"T251","span":{"begin":689,"end":693},"obj":"Sentence"},{"id":"T252","span":{"begin":694,"end":721},"obj":"Sentence"},{"id":"T253","span":{"begin":722,"end":910},"obj":"Sentence"},{"id":"T254","span":{"begin":911,"end":1269},"obj":"Sentence"},{"id":"T255","span":{"begin":1270,"end":1341},"obj":"Sentence"},{"id":"T256","span":{"begin":1342,"end":1413},"obj":"Sentence"},{"id":"T257","span":{"begin":1414,"end":1509},"obj":"Sentence"},{"id":"T258","span":{"begin":1510,"end":1551},"obj":"Sentence"},{"id":"T259","span":{"begin":1552,"end":1648},"obj":"Sentence"},{"id":"T260","span":{"begin":1649,"end":1732},"obj":"Sentence"},{"id":"T261","span":{"begin":1733,"end":1863},"obj":"Sentence"},{"id":"T262","span":{"begin":1864,"end":1964},"obj":"Sentence"},{"id":"T263","span":{"begin":1965,"end":2056},"obj":"Sentence"},{"id":"T264","span":{"begin":2057,"end":2172},"obj":"Sentence"},{"id":"T265","span":{"begin":2173,"end":2395},"obj":"Sentence"},{"id":"T266","span":{"begin":2396,"end":2476},"obj":"Sentence"},{"id":"T267","span":{"begin":2477,"end":2565},"obj":"Sentence"},{"id":"T268","span":{"begin":2566,"end":2863},"obj":"Sentence"},{"id":"T269","span":{"begin":2864,"end":2992},"obj":"Sentence"},{"id":"T270","span":{"begin":2993,"end":3080},"obj":"Sentence"},{"id":"T271","span":{"begin":3081,"end":3172},"obj":"Sentence"},{"id":"T272","span":{"begin":3173,"end":3344},"obj":"Sentence"},{"id":"T273","span":{"begin":3345,"end":3445},"obj":"Sentence"},{"id":"T274","span":{"begin":3446,"end":3544},"obj":"Sentence"},{"id":"T275","span":{"begin":3545,"end":3602},"obj":"Sentence"},{"id":"T276","span":{"begin":3603,"end":3674},"obj":"Sentence"},{"id":"T277","span":{"begin":3675,"end":3796},"obj":"Sentence"},{"id":"T278","span":{"begin":3797,"end":3872},"obj":"Sentence"},{"id":"T279","span":{"begin":3873,"end":4090},"obj":"Sentence"},{"id":"T280","span":{"begin":4092,"end":4096},"obj":"Sentence"},{"id":"T281","span":{"begin":4097,"end":4133},"obj":"Sentence"},{"id":"T282","span":{"begin":4134,"end":4318},"obj":"Sentence"},{"id":"T283","span":{"begin":4319,"end":4393},"obj":"Sentence"},{"id":"T284","span":{"begin":4394,"end":4583},"obj":"Sentence"},{"id":"T285","span":{"begin":4584,"end":4697},"obj":"Sentence"},{"id":"T286","span":{"begin":4698,"end":4989},"obj":"Sentence"},{"id":"T287","span":{"begin":4990,"end":5089},"obj":"Sentence"},{"id":"T288","span":{"begin":5090,"end":5206},"obj":"Sentence"},{"id":"T289","span":{"begin":5207,"end":5315},"obj":"Sentence"},{"id":"T290","span":{"begin":5316,"end":5367},"obj":"Sentence"},{"id":"T291","span":{"begin":5368,"end":5478},"obj":"Sentence"},{"id":"T292","span":{"begin":5479,"end":5509},"obj":"Sentence"},{"id":"T293","span":{"begin":5510,"end":5584},"obj":"Sentence"},{"id":"T294","span":{"begin":5585,"end":5655},"obj":"Sentence"},{"id":"T295","span":{"begin":5656,"end":5748},"obj":"Sentence"},{"id":"T296","span":{"begin":5749,"end":5818},"obj":"Sentence"},{"id":"T297","span":{"begin":5819,"end":5930},"obj":"Sentence"},{"id":"T298","span":{"begin":5931,"end":6026},"obj":"Sentence"},{"id":"T299","span":{"begin":6027,"end":6104},"obj":"Sentence"},{"id":"T300","span":{"begin":6106,"end":6110},"obj":"Sentence"},{"id":"T301","span":{"begin":6111,"end":6142},"obj":"Sentence"},{"id":"T302","span":{"begin":6143,"end":6311},"obj":"Sentence"},{"id":"T303","span":{"begin":6312,"end":6389},"obj":"Sentence"},{"id":"T304","span":{"begin":6390,"end":6656},"obj":"Sentence"},{"id":"T305","span":{"begin":6657,"end":6863},"obj":"Sentence"},{"id":"T306","span":{"begin":6864,"end":6933},"obj":"Sentence"},{"id":"T307","span":{"begin":6934,"end":7082},"obj":"Sentence"},{"id":"T308","span":{"begin":7083,"end":7270},"obj":"Sentence"},{"id":"T309","span":{"begin":7271,"end":7388},"obj":"Sentence"},{"id":"T310","span":{"begin":7389,"end":7507},"obj":"Sentence"},{"id":"T311","span":{"begin":7508,"end":7679},"obj":"Sentence"},{"id":"T312","span":{"begin":7681,"end":7685},"obj":"Sentence"},{"id":"T313","span":{"begin":7686,"end":7721},"obj":"Sentence"},{"id":"T314","span":{"begin":7722,"end":7823},"obj":"Sentence"},{"id":"T315","span":{"begin":7824,"end":7928},"obj":"Sentence"},{"id":"T316","span":{"begin":7929,"end":8048},"obj":"Sentence"},{"id":"T317","span":{"begin":8049,"end":8216},"obj":"Sentence"},{"id":"T318","span":{"begin":8217,"end":8419},"obj":"Sentence"},{"id":"T319","span":{"begin":8420,"end":8647},"obj":"Sentence"},{"id":"T320","span":{"begin":8648,"end":8674},"obj":"Sentence"},{"id":"T321","span":{"begin":8675,"end":8863},"obj":"Sentence"},{"id":"T322","span":{"begin":8864,"end":9052},"obj":"Sentence"},{"id":"T323","span":{"begin":9053,"end":9197},"obj":"Sentence"},{"id":"T324","span":{"begin":9198,"end":9310},"obj":"Sentence"},{"id":"T325","span":{"begin":9311,"end":9380},"obj":"Sentence"},{"id":"T326","span":{"begin":9381,"end":9607},"obj":"Sentence"},{"id":"T327","span":{"begin":9608,"end":9711},"obj":"Sentence"},{"id":"T328","span":{"begin":9712,"end":9833},"obj":"Sentence"},{"id":"T329","span":{"begin":9834,"end":9976},"obj":"Sentence"},{"id":"T330","span":{"begin":9977,"end":10099},"obj":"Sentence"},{"id":"T331","span":{"begin":10101,"end":10105},"obj":"Sentence"},{"id":"T332","span":{"begin":10106,"end":10144},"obj":"Sentence"},{"id":"T333","span":{"begin":10145,"end":10244},"obj":"Sentence"},{"id":"T334","span":{"begin":10245,"end":10360},"obj":"Sentence"},{"id":"T335","span":{"begin":10361,"end":10515},"obj":"Sentence"},{"id":"T336","span":{"begin":10516,"end":10595},"obj":"Sentence"},{"id":"T337","span":{"begin":10596,"end":10715},"obj":"Sentence"},{"id":"T338","span":{"begin":10716,"end":10886},"obj":"Sentence"},{"id":"T339","span":{"begin":10887,"end":10983},"obj":"Sentence"},{"id":"T340","span":{"begin":10984,"end":11064},"obj":"Sentence"},{"id":"T341","span":{"begin":11065,"end":11244},"obj":"Sentence"}],"namespaces":[{"prefix":"_base","uri":"http://pubannotation.org/ontology/tao.owl#"}],"text":"4. Results\n\n4.1. Measurement Model Assessment\nTo assess our measurement model, we verified the concurrent validity, discriminate validity, and composite reliability (CR) [77]. In addition to the square root values of the average variance extracted (AVE), we compared constructs to determine the discriminate validity [78]. Factor loading values should be greater than 0.70 [79]. For data validity and measurement, the value of the AVE should be greater than 0.5 [80], CR \u003e 0.7 [78], and rho \u003e 0.7 [81]. Table 3 provides the reliability and validity of our measurement scales and Table 4 provides the results of the Fornell–Larcker test used to check distinguished and divergent validity.\n\n4.2. Structured Model Assessment\nTo create intervals of confidence and t-values, we used bootstrapping (4000 re samples) to check for imagined associations between the concerned constructs of the planned structured model. Streukens, S., et al. [82] stated that bootstrap replications can vary considerably from a minimum of 500 to a maximum of 5000.In other words, statistic inconsistency is checked using the inconsistency of data using bootstrapping, which is a nonparametric resampling method, instead of using parametric statements to check the accuracy of approximation [83]. Efron, B., et al. [84] proposed using more than 1000 bootstrap samples.\nThe mediation effect is absent if the direct effect is not significant. Figure 1 illustrates the hypotheses testing of direct effects, which are also shown in Table 3. Table 3 also provides the fit statistics. Dependent variables indicate an important and positive precursor to their independent variables. Particularly, the role of E-Govt was a noteworthy predictor of epidemic protection. As can be seen from Figure 4 and Table 5, all of the hypotheses were supported [85] for the direct effect hypothesis at this step.\nTo check the importance of the structural path coefficients, we report the confidence interval [86]. These were supported because we did not add up zero values in any confidence interval [78]. At present, in standardized root mean square residual (SRMR) PLS path modeling, mostly model fit criteria are used. We checked the accuracy of the fit by using different tools like value of normed fit index (NFI), the non-normed fit index (NNFI), the comparative fit index (CFI), root mean square error of approximation (RMSEA), and SRMR. Values equal to or higher than 0.95 in NFI, NNFI, and CFI indicate the best fit. Sufficient adjustment was represented by RMSEA and SRMR with values less than 0.06 [87]. For comparatively good fit between the hypothesized model and observed data, a cut-off value near to 0.08 for SRMR and near 0.06 is best for RMSEA; Hu, L.T. et al. [88] stated that a zero value for SRMR indicates an ideal fit but if the value is smaller than 0.05,the fit is satisfactory fit [89]. We investigated the standards of the coefficient of determination (R2) to verify the predictive strength of our structure model. Collective consequences of exogenous contracts on endogenous constructs were indicated. The R2 of the endogenous latent variables is the vital decisive factor for this evaluation. This marker is used, from the perspective of a statistical model, to forecast future results or can be used to check the hypothesis on behalf of other related information. R2 also provides the results of the calculations and describes the practicality of the results [90]. Researchers can also use PLS procedure to check their model’s out-of-sample predictive power [91]. In-sample predictive power, we also refer to the R2 [92]. R2 varies from 0 to 1 and greater values show better descriptive power. Substantial, moderate, and weak descriptive powers are indicated by R2 values of 0.75, 0.50, and 0.25, respectively [93]. PLS-SEM is less dependent on the model fit concept compared to CB-SEM [79].\nAs recommended [74] RMSEA cut-off values equal to or less than 0.08 using modification in R2 report effect size (f2) indicated that the effect of our dependent variables on independent variables was very satisfactory.\n\n4.3. Effect Size and Predictive Relevance\nThe effect of the exogenous latent construct on the endogenous latent construct having three possible answers, i.e., substantial, moderate, and weak, was found using theF2 effect size. The blindfold method was used to check the strength of the research model. Cohen’s f2 is an identical measure of effect size that also permits checking the local effect size, which is the effect of one variable compared with the multivariate regression model [94]. If the cross-validated redundancy (Q2) is higher than 0,then the model is related to predicting that factor [95]. We focused on in-sample prediction more, compared to out-sample prediction, prognostic significance Q2, and relative relevance Q2, which are alternatives for evaluating a model’s practical relevance, in addition to consulting R2 outcomes as a gauge of a model’s predictive capabilities [95]. R2, Q2, path coefficients, and the effect size (f2) are the decisive factors we use for evaluation. In addition to this evaluation, researchers are required to check the inner model for potential co linearity issues. If the constructs are interrelated, then results approximated by the inner model are considered biased [96]. A model’s predictive accuracy is decided by the R2. The R2 value also characterizes the combined consequence of exogenous variables on the endogenous variable(s). The effect ranges from 0 to 1. A value of 1 indicates complete predictive accuracy as can see in Table 6.\nCohen’s f2 was calculated to check the effect size of each path model. When a construct was removed from the model, we calculated f2 while making no changes to R2. Researchers have to approximate two PLS path models for computing f2. The effect size of the omitted construct for a particular endogenous construct can be found by standard values: 0.02 for small, 0.15 for medium, and 0.35 for a large effect on the basis of the f2 value [96].\nThis discussion supports the use of our mediators and variables in the model.\n\n4.4. Multiple Mediating Effect Tests\nIn the PLS path model, mediator variables absorb the effect of an exogenous construct on an endogenous construct; this absorption of effect is known as meditation [96]. The mediation effect can be investigated using many tools, including PLS-SEM. Though researchers use an older method to determine the mediation effect in PLS-SEM, the procedure that identifies the effect of a precursor variable on the findings and results is judged by mediation; in other words, mediation considers transitional variables [97]. A mediating variable may have a transitional role in the association between dependent and independent variables, and engagement of this third variable is the important feature of the mediating effect [98]. Clarification and elaboration are the main effects of mediation [81]. As such, we included multi-mediation concepts in the results of social presence theory and for psychological aspects during outbreaks (see Table 7).\nThe results of the effects of exogenous variables (role of E-Govt and 2019-nCoV-WOM) on the endogenous variable (online social presence) with the mediating effect are provided in Table 7. Multiple mediation paths outcomes and potency (level) of mediation effects are demonstrated in Figure 5 and Figure 6. Precise indirect effects were investigated by bootstrapping techniques with the help of the bias correction technique. The two independent variables (role of E-Govt and2019-nCoV-WOM) affected online social presence; these effects support H1b, H2b, and H3c in that order, as mentioned above.\n\n4.5. Magnitude and Strength of Mediation\nTo determine whether H4b, H5b, H3c, and H4c are supported, we used the proposals by Hair et al. [85]. To finalize conclusions about the mediation effect; we calculated the amount and magnitude of mediation. We incorporated the variance accounted for (VAF) method to calculate the strength of mediation (Figure 5 and Figure 6). If VAF is less than 0.2, there is no mediation; if VAF is less than or equal to 0.8, there is a partial mediation; if VAF is greater than 0.8, there is full mediation. The magnitude and strength of epidemic protection (H4b: a1b1) and attitude toward epidemic outbreak (H5b: a2b2) mediated the association between the role of E-Govt and online social presence (Figure 5). We found via comparison that epidemic protection has a partial mediation effect on the role of E-Govt and online social presence because the VAF value was more than 0.2, which indicated that there is a partial mediation effect. As such, H4b is supported. Attitude toward epidemic outbreaks mediated the association between 2019-nCoV-WOM and online social presence; the VAF value was greater than 0.2, which indicated the presence of mediation. Therefore, we hypothesized imaginary harmonizing partial mediation because the effects of 2019-nCoV-WOMwere considerable both directly and indirectly and their products were positive [99].\nHair et al. [85] Concluded that complementary and competitive mediation can be differentiated if direct and indirect effects are more prominent. The condition in which direct and indirect effects work in the same direction is called complementary mediation. This means the outcome of the direct and indirect effect is positive. The magnitude and strength of the mediation effect of epidemic protection (H3c: a3b1) and attitude toward epidemic outbreak (H4c: a4b2) mediating the association of 2019-nCoV-WOMand online social presence is shown in Figure 6. In comparison, the VAF value was higher than 0.2, which indicated the presence and effect of mediation. Due to the prominent direct and indirect effects of 2019-nCoV-WOM, the complementary partial mediation was also positive. The comparison showed that the association between 2019-nCoV-WOM and online social presence was mediated by attitude toward epidemic outbreak. The VAF value was higher than 0.2, which indicated partial mediation, also supporting the multi-mediation hypothesis [99].\n\n4.6. Impact–Performance Map Analysis (IPMA)\nIPMA, also called impact–performance map or priority map analysis, is a useful approach in PLS-SEM. IPMA adds facets and measurements that consider the scores of latent variables reporting the path coefficient [99].\nApproaches to determining the role of precursor constructs and their significance for management actions are offered by the PLS-SEM studies based on IPMA. IPMA compares the significance and recital (performance of the variables) [99]. Analysis dimensions are used to demonstrate the results of path coefficient approximation extended by IPMA in Figure 7. The advantage of IPMA is the validation of total effects and a representation of their significance in a construct with an average score that indicates their performance. Our main purpose with the construct was to find the most significant component in the construct.\nOnline ratings are associated with higher enjoyment than negative reviews [100]. To the best of our knowledge, the broader tasks and household behaviors of the community and family members in social media and role of E-Govt have been relatively under-examined."}