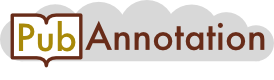
PMC:7210464 / 90209-92260
Annnotations
LitCovid-PD-FMA-UBERON
{"project":"LitCovid-PD-FMA-UBERON","denotations":[{"id":"T27","span":{"begin":0,"end":8},"obj":"Body_part"}],"attributes":[{"id":"A27","pred":"fma_id","subj":"T27","obj":"http://purl.org/sig/ont/fma/fma14542"}],"text":"Appendix C. Computation of counterfactuals\nOur main model is\n4 yct=∑τ=12∑k=1Kwithinαwithin,τkh¯ctkτy¯ctτ+∑τ=12∑k=1Kbetween∑r≠cαbetween,τkm¯crtkτy¯rtτ+∑τ=12∑k=1KWuhanρτkm¯c,Wuhan,tkτz¯tτ+xctβ+𝜖ct.\nIt is convenient to write it in vector form, 5 Ynt=∑s=114Hnt,s(αwithin)+Mnt,s(αbetween)Yn,t−s+∑τ=12Zntτρτ+Xntβ+𝜖nt,\nwhere Ynt=y1t⋯ynt′ and 𝜖nt are n × 1 vectors. Assuming that Yns = 0 if s ≤ 0, because our sample starts on January 19, and no laboratory confirmed case was reported before January 19 in cities outside Wuhan. Xnt=x1t′⋯xnt′′ is an n × k matrix of the control variables. Hnt,s(αwithin) is an n × n diagonal matrix corresponding to the s-day time lag, with parameters αwithin={αwithin,τk}k=1,⋯,Kwithin,τ=1,2. For example, for s = 1,⋯ , 7, the i th diagonal element of Hnt,s(αwithin) is 17∑k=1Kwithinαwithin,1kh¯ct,ik1, and for s = 8,⋯ , 14, the i th diagonal element of Hnt,s(αwithin) is 17∑k=1Kwithinαwithin,2kh¯ct,ik2. Mnt,s(αbetween) is constructed similarly. For example, for s = 1,⋯ , 7 and i≠j, the ij th element of Mnt,s(αbetween) is 17∑k=1Kbetweenαbetween,1km¯ijtk1. Zntτ is an n × KWuhan matrix corresponding to the transmission from Wuhan. For example, the ik th element of Znt1 is m¯i,Wuhan,tk1z¯t1.\nWe first estimate the parameters in Eq. 4 by 2SLS and obtain the residuals 𝜖^n1,⋯,𝜖^nT. Let ⋅^ denote the estimated value of parameters and ⋅~ denote the counterfactual changes. The counterfactual value of Ynt is computed recursively, Y~n1=∑τ=12Z~n1τρ^τ+Xn1β^+𝜖^n1,Y~n2=∑s=11H~n2,s(α^within)+M~n2,s(α^between)Y~n,2−s+∑τ=12Z~n2τρ^τ+Xn2β^+𝜖^n2,Y~n3=∑s=12H~n3,s(α^within)+M~n3,s(α^between)Y~n,3−s+∑τ=12Z~n3τρ^τ+Xn3β^+𝜖^n3,⋮\nThe counterfactual change for date t is ΔYnt=Y~nt−Ynt. The standard error of ΔYnt is obtained from 1000 bootstrap iterations. In each bootstrap iteration, cities are sampled with replacement and the model is estimated to obtain the parameters. The counterfactual predictions are obtained using the above equations with the estimated parameters and the counterfactual scenario (e.g., no cities adopted lockdown)."}
LitCovid-PD-MONDO
{"project":"LitCovid-PD-MONDO","denotations":[{"id":"T189","span":{"begin":372,"end":375},"obj":"Disease"}],"attributes":[{"id":"A189","pred":"mondo_id","subj":"T189","obj":"http://purl.obolibrary.org/obo/MONDO_0007921"}],"text":"Appendix C. Computation of counterfactuals\nOur main model is\n4 yct=∑τ=12∑k=1Kwithinαwithin,τkh¯ctkτy¯ctτ+∑τ=12∑k=1Kbetween∑r≠cαbetween,τkm¯crtkτy¯rtτ+∑τ=12∑k=1KWuhanρτkm¯c,Wuhan,tkτz¯tτ+xctβ+𝜖ct.\nIt is convenient to write it in vector form, 5 Ynt=∑s=114Hnt,s(αwithin)+Mnt,s(αbetween)Yn,t−s+∑τ=12Zntτρτ+Xntβ+𝜖nt,\nwhere Ynt=y1t⋯ynt′ and 𝜖nt are n × 1 vectors. Assuming that Yns = 0 if s ≤ 0, because our sample starts on January 19, and no laboratory confirmed case was reported before January 19 in cities outside Wuhan. Xnt=x1t′⋯xnt′′ is an n × k matrix of the control variables. Hnt,s(αwithin) is an n × n diagonal matrix corresponding to the s-day time lag, with parameters αwithin={αwithin,τk}k=1,⋯,Kwithin,τ=1,2. For example, for s = 1,⋯ , 7, the i th diagonal element of Hnt,s(αwithin) is 17∑k=1Kwithinαwithin,1kh¯ct,ik1, and for s = 8,⋯ , 14, the i th diagonal element of Hnt,s(αwithin) is 17∑k=1Kwithinαwithin,2kh¯ct,ik2. Mnt,s(αbetween) is constructed similarly. For example, for s = 1,⋯ , 7 and i≠j, the ij th element of Mnt,s(αbetween) is 17∑k=1Kbetweenαbetween,1km¯ijtk1. Zntτ is an n × KWuhan matrix corresponding to the transmission from Wuhan. For example, the ik th element of Znt1 is m¯i,Wuhan,tk1z¯t1.\nWe first estimate the parameters in Eq. 4 by 2SLS and obtain the residuals 𝜖^n1,⋯,𝜖^nT. Let ⋅^ denote the estimated value of parameters and ⋅~ denote the counterfactual changes. The counterfactual value of Ynt is computed recursively, Y~n1=∑τ=12Z~n1τρ^τ+Xn1β^+𝜖^n1,Y~n2=∑s=11H~n2,s(α^within)+M~n2,s(α^between)Y~n,2−s+∑τ=12Z~n2τρ^τ+Xn2β^+𝜖^n2,Y~n3=∑s=12H~n3,s(α^within)+M~n3,s(α^between)Y~n,3−s+∑τ=12Z~n3τρ^τ+Xn3β^+𝜖^n3,⋮\nThe counterfactual change for date t is ΔYnt=Y~nt−Ynt. The standard error of ΔYnt is obtained from 1000 bootstrap iterations. In each bootstrap iteration, cities are sampled with replacement and the model is estimated to obtain the parameters. The counterfactual predictions are obtained using the above equations with the estimated parameters and the counterfactual scenario (e.g., no cities adopted lockdown)."}
LitCovid-PD-CLO
{"project":"LitCovid-PD-CLO","denotations":[{"id":"T371","span":{"begin":178,"end":180},"obj":"http://purl.obolibrary.org/obo/CLO_0037066"},{"id":"T372","span":{"begin":655,"end":658},"obj":"http://purl.obolibrary.org/obo/CLO_0050236"},{"id":"T373","span":{"begin":696,"end":699},"obj":"http://purl.obolibrary.org/obo/CLO_0007052"},{"id":"T374","span":{"begin":734,"end":739},"obj":"http://purl.obolibrary.org/obo/CLO_0050050"},{"id":"T375","span":{"begin":988,"end":993},"obj":"http://purl.obolibrary.org/obo/CLO_0050050"}],"text":"Appendix C. Computation of counterfactuals\nOur main model is\n4 yct=∑τ=12∑k=1Kwithinαwithin,τkh¯ctkτy¯ctτ+∑τ=12∑k=1Kbetween∑r≠cαbetween,τkm¯crtkτy¯rtτ+∑τ=12∑k=1KWuhanρτkm¯c,Wuhan,tkτz¯tτ+xctβ+𝜖ct.\nIt is convenient to write it in vector form, 5 Ynt=∑s=114Hnt,s(αwithin)+Mnt,s(αbetween)Yn,t−s+∑τ=12Zntτρτ+Xntβ+𝜖nt,\nwhere Ynt=y1t⋯ynt′ and 𝜖nt are n × 1 vectors. Assuming that Yns = 0 if s ≤ 0, because our sample starts on January 19, and no laboratory confirmed case was reported before January 19 in cities outside Wuhan. Xnt=x1t′⋯xnt′′ is an n × k matrix of the control variables. Hnt,s(αwithin) is an n × n diagonal matrix corresponding to the s-day time lag, with parameters αwithin={αwithin,τk}k=1,⋯,Kwithin,τ=1,2. For example, for s = 1,⋯ , 7, the i th diagonal element of Hnt,s(αwithin) is 17∑k=1Kwithinαwithin,1kh¯ct,ik1, and for s = 8,⋯ , 14, the i th diagonal element of Hnt,s(αwithin) is 17∑k=1Kwithinαwithin,2kh¯ct,ik2. Mnt,s(αbetween) is constructed similarly. For example, for s = 1,⋯ , 7 and i≠j, the ij th element of Mnt,s(αbetween) is 17∑k=1Kbetweenαbetween,1km¯ijtk1. Zntτ is an n × KWuhan matrix corresponding to the transmission from Wuhan. For example, the ik th element of Znt1 is m¯i,Wuhan,tk1z¯t1.\nWe first estimate the parameters in Eq. 4 by 2SLS and obtain the residuals 𝜖^n1,⋯,𝜖^nT. Let ⋅^ denote the estimated value of parameters and ⋅~ denote the counterfactual changes. The counterfactual value of Ynt is computed recursively, Y~n1=∑τ=12Z~n1τρ^τ+Xn1β^+𝜖^n1,Y~n2=∑s=11H~n2,s(α^within)+M~n2,s(α^between)Y~n,2−s+∑τ=12Z~n2τρ^τ+Xn2β^+𝜖^n2,Y~n3=∑s=12H~n3,s(α^within)+M~n3,s(α^between)Y~n,3−s+∑τ=12Z~n3τρ^τ+Xn3β^+𝜖^n3,⋮\nThe counterfactual change for date t is ΔYnt=Y~nt−Ynt. The standard error of ΔYnt is obtained from 1000 bootstrap iterations. In each bootstrap iteration, cities are sampled with replacement and the model is estimated to obtain the parameters. The counterfactual predictions are obtained using the above equations with the estimated parameters and the counterfactual scenario (e.g., no cities adopted lockdown)."}
LitCovid-sentences
{"project":"LitCovid-sentences","denotations":[{"id":"T868","span":{"begin":0,"end":11},"obj":"Sentence"},{"id":"T869","span":{"begin":12,"end":42},"obj":"Sentence"},{"id":"T870","span":{"begin":43,"end":60},"obj":"Sentence"},{"id":"T871","span":{"begin":61,"end":195},"obj":"Sentence"},{"id":"T872","span":{"begin":196,"end":311},"obj":"Sentence"},{"id":"T873","span":{"begin":312,"end":357},"obj":"Sentence"},{"id":"T874","span":{"begin":358,"end":519},"obj":"Sentence"},{"id":"T875","span":{"begin":520,"end":579},"obj":"Sentence"},{"id":"T876","span":{"begin":580,"end":716},"obj":"Sentence"},{"id":"T877","span":{"begin":717,"end":928},"obj":"Sentence"},{"id":"T878","span":{"begin":929,"end":970},"obj":"Sentence"},{"id":"T879","span":{"begin":971,"end":1082},"obj":"Sentence"},{"id":"T880","span":{"begin":1083,"end":1157},"obj":"Sentence"},{"id":"T881","span":{"begin":1158,"end":1218},"obj":"Sentence"},{"id":"T882","span":{"begin":1219,"end":1306},"obj":"Sentence"},{"id":"T883","span":{"begin":1307,"end":1396},"obj":"Sentence"},{"id":"T884","span":{"begin":1397,"end":1639},"obj":"Sentence"},{"id":"T885","span":{"begin":1640,"end":1694},"obj":"Sentence"},{"id":"T886","span":{"begin":1695,"end":1765},"obj":"Sentence"},{"id":"T887","span":{"begin":1766,"end":1883},"obj":"Sentence"},{"id":"T888","span":{"begin":1884,"end":2051},"obj":"Sentence"}],"namespaces":[{"prefix":"_base","uri":"http://pubannotation.org/ontology/tao.owl#"}],"text":"Appendix C. Computation of counterfactuals\nOur main model is\n4 yct=∑τ=12∑k=1Kwithinαwithin,τkh¯ctkτy¯ctτ+∑τ=12∑k=1Kbetween∑r≠cαbetween,τkm¯crtkτy¯rtτ+∑τ=12∑k=1KWuhanρτkm¯c,Wuhan,tkτz¯tτ+xctβ+𝜖ct.\nIt is convenient to write it in vector form, 5 Ynt=∑s=114Hnt,s(αwithin)+Mnt,s(αbetween)Yn,t−s+∑τ=12Zntτρτ+Xntβ+𝜖nt,\nwhere Ynt=y1t⋯ynt′ and 𝜖nt are n × 1 vectors. Assuming that Yns = 0 if s ≤ 0, because our sample starts on January 19, and no laboratory confirmed case was reported before January 19 in cities outside Wuhan. Xnt=x1t′⋯xnt′′ is an n × k matrix of the control variables. Hnt,s(αwithin) is an n × n diagonal matrix corresponding to the s-day time lag, with parameters αwithin={αwithin,τk}k=1,⋯,Kwithin,τ=1,2. For example, for s = 1,⋯ , 7, the i th diagonal element of Hnt,s(αwithin) is 17∑k=1Kwithinαwithin,1kh¯ct,ik1, and for s = 8,⋯ , 14, the i th diagonal element of Hnt,s(αwithin) is 17∑k=1Kwithinαwithin,2kh¯ct,ik2. Mnt,s(αbetween) is constructed similarly. For example, for s = 1,⋯ , 7 and i≠j, the ij th element of Mnt,s(αbetween) is 17∑k=1Kbetweenαbetween,1km¯ijtk1. Zntτ is an n × KWuhan matrix corresponding to the transmission from Wuhan. For example, the ik th element of Znt1 is m¯i,Wuhan,tk1z¯t1.\nWe first estimate the parameters in Eq. 4 by 2SLS and obtain the residuals 𝜖^n1,⋯,𝜖^nT. Let ⋅^ denote the estimated value of parameters and ⋅~ denote the counterfactual changes. The counterfactual value of Ynt is computed recursively, Y~n1=∑τ=12Z~n1τρ^τ+Xn1β^+𝜖^n1,Y~n2=∑s=11H~n2,s(α^within)+M~n2,s(α^between)Y~n,2−s+∑τ=12Z~n2τρ^τ+Xn2β^+𝜖^n2,Y~n3=∑s=12H~n3,s(α^within)+M~n3,s(α^between)Y~n,3−s+∑τ=12Z~n3τρ^τ+Xn3β^+𝜖^n3,⋮\nThe counterfactual change for date t is ΔYnt=Y~nt−Ynt. The standard error of ΔYnt is obtained from 1000 bootstrap iterations. In each bootstrap iteration, cities are sampled with replacement and the model is estimated to obtain the parameters. The counterfactual predictions are obtained using the above equations with the estimated parameters and the counterfactual scenario (e.g., no cities adopted lockdown)."}