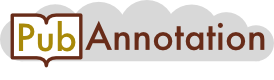
PMC:7210464 / 78924-85618
Annnotations
LitCovid-PD-FMA-UBERON
{"project":"LitCovid-PD-FMA-UBERON","denotations":[{"id":"T24","span":{"begin":0,"end":8},"obj":"Body_part"},{"id":"T25","span":{"begin":3891,"end":3896},"obj":"Body_part"}],"attributes":[{"id":"A24","pred":"fma_id","subj":"T24","obj":"http://purl.org/sig/ont/fma/fma14542"},{"id":"A25","pred":"fma_id","subj":"T25","obj":"http://purl.org/sig/ont/fma/fma7490"}],"text":"Appendix A. First stage regressions\nWeather conditions affect disease transmissions either directly if the virus can more easily survive and spread in certain environment, or indirectly by changing human behavior. Table 9 reports results of the first stage of the IV regressions (Table 4) using the full sample. In columns (1) and (2), the dependent variables are the numbers of newly confirmed COVID-19 cases in the own city in the preceding first and second weeks, respectively. In columns (3) and (4), the dependent variables are the sum of inverse log distance weighted numbers of newly confirmed COVID-19 cases in other cities in the preceding first and second weeks, respectively. These are the endogenous variables in the IV regressions. The weather variables in the preceding first and second weeks are included in the control variables. The weather variables in the preceding third and fourth weeks are the excluded instruments, and their coefficients are reported in the table. Because the variables are averages in 7-day moving windows, the error term may be serially correlated, and we include city by week fixed effects. Also included in the control variables are the average numbers of new cases in Wuhan in the preceding first and second weeks, interacted with the inverse log distance or the population flow.\nTable 9 First stage regressions\nDependent variable Average # of new cases\nOwn city Other cities\n1-week lag 2-week lag 1-week lag 2-week lag\n(1) (2) (3) (4)\nOwn City\nMaximum temperature, 3-week lag 0.200*** − 0.0431 0.564 − 2.022***\n(0.0579) (0.0503) (0.424) (0.417)\nPrecipitation, 3-week lag − 0.685 − 0.865* 4.516 − 1.998\n(0.552) (0.480) (4.045) (3.982)\nWind speed, 3-week lag 0.508** 0.299 − 0.827 3.247*\n(0.256) (0.223) (1.878) (1.849)\nPrecipitation × wind speed, 3-week lag − 0.412** 0.122 − 1.129 − 2.091\n(0.199) (0.173) (1.460) (1.437)\nMaximum temperature, 4-week lag 0.162*** 0.125** 1.379*** 1.181***\n(0.0560) (0.0487) (0.410) (0.404)\nPrecipitation, 4-week lag 0.0250 − 0.503 2.667 8.952***\n(0.440) (0.383) (3.224) (3.174)\nWind speed, 4-week lag 0.179 0.214 − 1.839 1.658\n(0.199) (0.173) (1.458) (1.435)\nPrecipitation × wind speed, 4-week lag − 0.354** − 0.0270 1.107 − 2.159**\n(0.145) (0.126) (1.059) (1.043)\nOther cities, weight = inverse distance\nMaximum temperature, 3-week lag − 0.0809*** − 0.00633 0.0520 1.152***\n(0.0203) (0.0176) (0.149) (0.146)\nPrecipitation, 3-week lag 4.366*** − 2.370*** 17.99*** − 72.68***\n(0.639) (0.556) (4.684) (4.611)\nWind speed, 3-week lag 0.326*** − 0.222** − 1.456 − 11.02***\n(0.126) (0.110) (0.926) (0.912)\nPrecipitation × wind speed, 3-week lag − 1.780*** 0.724*** − 6.750*** 27.73***\n(0.227) (0.197) (1.663) (1.637)\nMaximum temperature, 4-week lag − 0.0929*** − 0.0346* − 0.518*** 0.0407\n(0.0220) (0.0191) (0.161) (0.159)\nPrecipitation, 4-week lag 3.357*** − 0.578 46.57*** − 25.31***\n(0.504) (0.438) (3.691) (3.633)\nWind speed, 4-week lag 0.499*** 0.214** 4.660*** − 4.639***\n(0.107) (0.0934) (0.787) (0.774)\nPrecipitation × wind speed, 4-week lag − 1.358*** − 0.0416 − 17.26*** 8.967***\n(0.178) (0.155) (1.303) (1.282)\nF statistic 11.41 8.46 19.10 36.32\np value 0.0000 0.0000 0.0000 0.0000\nObservations 12,768 12,768 12,768 12,768\nNumber of cities 304 304 304 304\n# cases in Wuhan Yes Yes Yes Yes\nContemporaneous weather controls Yes Yes Yes Yes\nCity FE Yes Yes Yes Yes\nDate FE Yes Yes Yes Yes\nCity by week FE Yes Yes Yes Yes\nThis table shows the results of the first stage IV regressions. The weather variables are weekly averages of daily weather readings. Coefficients of the weather variables which are used as excluded instrumental variables are reported. *** p \u003c 0.01, ** p \u003c 0.05, * p \u003c 0.1\nBecause the spread of the virus depends on both the number of infectious people and the weather conditions, the coefficients in the first stage regressions do not have structural interpretations. The Wald tests on the joint significance of the excluded instruments are conducted and their F statistics are reported. The excluded instruments have good predictive power.\nThe implementation of local public health measures is likely correlated with the extent of the virus spread, so weather conditions that affect virus transmissions may also affect the likelihood that the policy is adopted. The influence of weather conditions on policy adoption may be complicated, so we use the Cluster-Lasso method of Belloni et al. (2016) to select the weather variables that have good predictive power on the adoption of closed management of communities or family outdoor restrictions. Let dct be the dummy variable of the adoption of the local public health measure, i.e., dct = 1 if the policy is in place in city c at day t. qct is a vector of candidate weather variables, including weekly averages of daily mean temperature, maximum temperature, minimum temperature, dew point, station-level pressure, sea-level pressure, visibility, wind speed, maximum wind speed, snow depth, precipitation, dummy for adverse weather conditions, squared terms of these variables, and interactions among them. First, city and day fixed effects are removed. d¨ct=dct−1n∑cdct−1T∑tdct+1nT∑ctdct and q¨ct is defined similarly. The Cluster-Lasso method solves the following minimization problem: 1nT∑ctd¨ct−q¨ct′b2+λnT∑kϕk|bk|.λ and ϕ are penalty parameters. A larger penalty value forces more coefficients to zero. The penalty parameters are picked using the theoretical result of Belloni et al. (2016). The estimation uses the Stata package by Ahrens et al. (2019). Table 10 lists the selected weather variables, which are used as the instruments in Table 8.\nTable 10 Variables selected\nDependent variable: closed management of communities\nDew point 1-week lag\nDiurnal temperature range 1-week lag\nDew point 2-week lag\nSea-level pressure 2-week lag\nDew point 3-week lag\nVisibility 4-week lag\nPrecipitation 4-week lag\nDependent variable: family outdoor restrictions\nStation pressure 1-week lag\nDummy for adverse weather conditions such as fog, rain, and drizzle 1-week lag\nMaximum temperature 2-week lag\nSea-level pressure 2-week lag\nAverage temperature 3-week lag\nMinimum temperature 3-week lag\nVisibility 3-week lag\nThis table shows the weather variables selected by lassopack (Ahrens et al. 2019), which implements the Cluster-Lasso method of Belloni et al. (2016). City and date fixed effects are included. Candidate variables include weekly averages of daily mean temperature, maximum temperature, minimum temperature, dew point, station-level pressure, sea-level pressure, visibility, wind speed, maximum wind speed, snow depth, precipitation, dummy for adverse weather conditions, squared terms of these variables, and interactions among them"}
LitCovid-PD-MONDO
{"project":"LitCovid-PD-MONDO","denotations":[{"id":"T175","span":{"begin":395,"end":403},"obj":"Disease"},{"id":"T176","span":{"begin":601,"end":609},"obj":"Disease"},{"id":"T177","span":{"begin":3735,"end":3745},"obj":"Disease"}],"attributes":[{"id":"A175","pred":"mondo_id","subj":"T175","obj":"http://purl.obolibrary.org/obo/MONDO_0100096"},{"id":"A176","pred":"mondo_id","subj":"T176","obj":"http://purl.obolibrary.org/obo/MONDO_0100096"},{"id":"A177","pred":"mondo_id","subj":"T177","obj":"http://purl.obolibrary.org/obo/MONDO_0005550"}],"text":"Appendix A. First stage regressions\nWeather conditions affect disease transmissions either directly if the virus can more easily survive and spread in certain environment, or indirectly by changing human behavior. Table 9 reports results of the first stage of the IV regressions (Table 4) using the full sample. In columns (1) and (2), the dependent variables are the numbers of newly confirmed COVID-19 cases in the own city in the preceding first and second weeks, respectively. In columns (3) and (4), the dependent variables are the sum of inverse log distance weighted numbers of newly confirmed COVID-19 cases in other cities in the preceding first and second weeks, respectively. These are the endogenous variables in the IV regressions. The weather variables in the preceding first and second weeks are included in the control variables. The weather variables in the preceding third and fourth weeks are the excluded instruments, and their coefficients are reported in the table. Because the variables are averages in 7-day moving windows, the error term may be serially correlated, and we include city by week fixed effects. Also included in the control variables are the average numbers of new cases in Wuhan in the preceding first and second weeks, interacted with the inverse log distance or the population flow.\nTable 9 First stage regressions\nDependent variable Average # of new cases\nOwn city Other cities\n1-week lag 2-week lag 1-week lag 2-week lag\n(1) (2) (3) (4)\nOwn City\nMaximum temperature, 3-week lag 0.200*** − 0.0431 0.564 − 2.022***\n(0.0579) (0.0503) (0.424) (0.417)\nPrecipitation, 3-week lag − 0.685 − 0.865* 4.516 − 1.998\n(0.552) (0.480) (4.045) (3.982)\nWind speed, 3-week lag 0.508** 0.299 − 0.827 3.247*\n(0.256) (0.223) (1.878) (1.849)\nPrecipitation × wind speed, 3-week lag − 0.412** 0.122 − 1.129 − 2.091\n(0.199) (0.173) (1.460) (1.437)\nMaximum temperature, 4-week lag 0.162*** 0.125** 1.379*** 1.181***\n(0.0560) (0.0487) (0.410) (0.404)\nPrecipitation, 4-week lag 0.0250 − 0.503 2.667 8.952***\n(0.440) (0.383) (3.224) (3.174)\nWind speed, 4-week lag 0.179 0.214 − 1.839 1.658\n(0.199) (0.173) (1.458) (1.435)\nPrecipitation × wind speed, 4-week lag − 0.354** − 0.0270 1.107 − 2.159**\n(0.145) (0.126) (1.059) (1.043)\nOther cities, weight = inverse distance\nMaximum temperature, 3-week lag − 0.0809*** − 0.00633 0.0520 1.152***\n(0.0203) (0.0176) (0.149) (0.146)\nPrecipitation, 3-week lag 4.366*** − 2.370*** 17.99*** − 72.68***\n(0.639) (0.556) (4.684) (4.611)\nWind speed, 3-week lag 0.326*** − 0.222** − 1.456 − 11.02***\n(0.126) (0.110) (0.926) (0.912)\nPrecipitation × wind speed, 3-week lag − 1.780*** 0.724*** − 6.750*** 27.73***\n(0.227) (0.197) (1.663) (1.637)\nMaximum temperature, 4-week lag − 0.0929*** − 0.0346* − 0.518*** 0.0407\n(0.0220) (0.0191) (0.161) (0.159)\nPrecipitation, 4-week lag 3.357*** − 0.578 46.57*** − 25.31***\n(0.504) (0.438) (3.691) (3.633)\nWind speed, 4-week lag 0.499*** 0.214** 4.660*** − 4.639***\n(0.107) (0.0934) (0.787) (0.774)\nPrecipitation × wind speed, 4-week lag − 1.358*** − 0.0416 − 17.26*** 8.967***\n(0.178) (0.155) (1.303) (1.282)\nF statistic 11.41 8.46 19.10 36.32\np value 0.0000 0.0000 0.0000 0.0000\nObservations 12,768 12,768 12,768 12,768\nNumber of cities 304 304 304 304\n# cases in Wuhan Yes Yes Yes Yes\nContemporaneous weather controls Yes Yes Yes Yes\nCity FE Yes Yes Yes Yes\nDate FE Yes Yes Yes Yes\nCity by week FE Yes Yes Yes Yes\nThis table shows the results of the first stage IV regressions. The weather variables are weekly averages of daily weather readings. Coefficients of the weather variables which are used as excluded instrumental variables are reported. *** p \u003c 0.01, ** p \u003c 0.05, * p \u003c 0.1\nBecause the spread of the virus depends on both the number of infectious people and the weather conditions, the coefficients in the first stage regressions do not have structural interpretations. The Wald tests on the joint significance of the excluded instruments are conducted and their F statistics are reported. The excluded instruments have good predictive power.\nThe implementation of local public health measures is likely correlated with the extent of the virus spread, so weather conditions that affect virus transmissions may also affect the likelihood that the policy is adopted. The influence of weather conditions on policy adoption may be complicated, so we use the Cluster-Lasso method of Belloni et al. (2016) to select the weather variables that have good predictive power on the adoption of closed management of communities or family outdoor restrictions. Let dct be the dummy variable of the adoption of the local public health measure, i.e., dct = 1 if the policy is in place in city c at day t. qct is a vector of candidate weather variables, including weekly averages of daily mean temperature, maximum temperature, minimum temperature, dew point, station-level pressure, sea-level pressure, visibility, wind speed, maximum wind speed, snow depth, precipitation, dummy for adverse weather conditions, squared terms of these variables, and interactions among them. First, city and day fixed effects are removed. d¨ct=dct−1n∑cdct−1T∑tdct+1nT∑ctdct and q¨ct is defined similarly. The Cluster-Lasso method solves the following minimization problem: 1nT∑ctd¨ct−q¨ct′b2+λnT∑kϕk|bk|.λ and ϕ are penalty parameters. A larger penalty value forces more coefficients to zero. The penalty parameters are picked using the theoretical result of Belloni et al. (2016). The estimation uses the Stata package by Ahrens et al. (2019). Table 10 lists the selected weather variables, which are used as the instruments in Table 8.\nTable 10 Variables selected\nDependent variable: closed management of communities\nDew point 1-week lag\nDiurnal temperature range 1-week lag\nDew point 2-week lag\nSea-level pressure 2-week lag\nDew point 3-week lag\nVisibility 4-week lag\nPrecipitation 4-week lag\nDependent variable: family outdoor restrictions\nStation pressure 1-week lag\nDummy for adverse weather conditions such as fog, rain, and drizzle 1-week lag\nMaximum temperature 2-week lag\nSea-level pressure 2-week lag\nAverage temperature 3-week lag\nMinimum temperature 3-week lag\nVisibility 3-week lag\nThis table shows the weather variables selected by lassopack (Ahrens et al. 2019), which implements the Cluster-Lasso method of Belloni et al. (2016). City and date fixed effects are included. Candidate variables include weekly averages of daily mean temperature, maximum temperature, minimum temperature, dew point, station-level pressure, sea-level pressure, visibility, wind speed, maximum wind speed, snow depth, precipitation, dummy for adverse weather conditions, squared terms of these variables, and interactions among them"}
LitCovid-PD-CLO
{"project":"LitCovid-PD-CLO","denotations":[{"id":"T300","span":{"begin":9,"end":10},"obj":"http://purl.obolibrary.org/obo/CLO_0001020"},{"id":"T301","span":{"begin":107,"end":112},"obj":"http://purl.obolibrary.org/obo/NCBITaxon_10239"},{"id":"T302","span":{"begin":198,"end":203},"obj":"http://purl.obolibrary.org/obo/NCBITaxon_9606"},{"id":"T303","span":{"begin":925,"end":936},"obj":"http://purl.obolibrary.org/obo/OBI_0000968"},{"id":"T304","span":{"begin":1428,"end":1431},"obj":"http://purl.obolibrary.org/obo/CLO_0050236"},{"id":"T305","span":{"begin":1439,"end":1442},"obj":"http://purl.obolibrary.org/obo/CLO_0050236"},{"id":"T306","span":{"begin":1450,"end":1453},"obj":"http://purl.obolibrary.org/obo/CLO_0050236"},{"id":"T307","span":{"begin":1461,"end":1464},"obj":"http://purl.obolibrary.org/obo/CLO_0050236"},{"id":"T308","span":{"begin":1474,"end":1479},"obj":"http://purl.obolibrary.org/obo/CLO_0001302"},{"id":"T309","span":{"begin":1518,"end":1521},"obj":"http://purl.obolibrary.org/obo/CLO_0050236"},{"id":"T310","span":{"begin":1613,"end":1616},"obj":"http://purl.obolibrary.org/obo/CLO_0050236"},{"id":"T311","span":{"begin":1699,"end":1702},"obj":"http://purl.obolibrary.org/obo/CLO_0050236"},{"id":"T312","span":{"begin":1799,"end":1802},"obj":"http://purl.obolibrary.org/obo/CLO_0050236"},{"id":"T313","span":{"begin":1895,"end":1898},"obj":"http://purl.obolibrary.org/obo/CLO_0050236"},{"id":"T314","span":{"begin":1990,"end":1993},"obj":"http://purl.obolibrary.org/obo/CLO_0050236"},{"id":"T315","span":{"begin":2075,"end":2078},"obj":"http://purl.obolibrary.org/obo/CLO_0050236"},{"id":"T316","span":{"begin":2172,"end":2175},"obj":"http://purl.obolibrary.org/obo/CLO_0050236"},{"id":"T317","span":{"begin":2311,"end":2314},"obj":"http://purl.obolibrary.org/obo/CLO_0050236"},{"id":"T318","span":{"begin":2409,"end":2412},"obj":"http://purl.obolibrary.org/obo/CLO_0050236"},{"id":"T319","span":{"begin":2504,"end":2507},"obj":"http://purl.obolibrary.org/obo/CLO_0050236"},{"id":"T320","span":{"begin":2613,"end":2616},"obj":"http://purl.obolibrary.org/obo/CLO_0050236"},{"id":"T321","span":{"begin":2717,"end":2720},"obj":"http://purl.obolibrary.org/obo/CLO_0050236"},{"id":"T322","span":{"begin":2817,"end":2820},"obj":"http://purl.obolibrary.org/obo/CLO_0050236"},{"id":"T323","span":{"begin":2909,"end":2912},"obj":"http://purl.obolibrary.org/obo/CLO_0050236"},{"id":"T324","span":{"begin":3018,"end":3021},"obj":"http://purl.obolibrary.org/obo/CLO_0050236"},{"id":"T325","span":{"begin":3599,"end":3611},"obj":"http://purl.obolibrary.org/obo/OBI_0000968"},{"id":"T326","span":{"begin":3699,"end":3704},"obj":"http://purl.obolibrary.org/obo/NCBITaxon_10239"},{"id":"T327","span":{"begin":3878,"end":3883},"obj":"http://purl.obolibrary.org/obo/UBERON_0000473"},{"id":"T328","span":{"begin":3891,"end":3896},"obj":"http://purl.obolibrary.org/obo/UBERON_0000982"},{"id":"T329","span":{"begin":3891,"end":3896},"obj":"http://purl.obolibrary.org/obo/UBERON_0004905"},{"id":"T330","span":{"begin":3926,"end":3937},"obj":"http://purl.obolibrary.org/obo/OBI_0000968"},{"id":"T331","span":{"begin":4002,"end":4013},"obj":"http://purl.obolibrary.org/obo/OBI_0000968"},{"id":"T332","span":{"begin":4137,"end":4142},"obj":"http://purl.obolibrary.org/obo/NCBITaxon_10239"},{"id":"T333","span":{"begin":4185,"end":4190},"obj":"http://purl.obolibrary.org/obo/NCBITaxon_10239"},{"id":"T334","span":{"begin":4696,"end":4697},"obj":"http://purl.obolibrary.org/obo/CLO_0001020"},{"id":"T335","span":{"begin":5303,"end":5304},"obj":"http://purl.obolibrary.org/obo/CLO_0001020"},{"id":"T336","span":{"begin":5581,"end":5592},"obj":"http://purl.obolibrary.org/obo/OBI_0000968"},{"id":"T337","span":{"begin":5703,"end":5706},"obj":"http://purl.obolibrary.org/obo/CLO_0050236"},{"id":"T338","span":{"begin":5740,"end":5743},"obj":"http://purl.obolibrary.org/obo/CLO_0050236"},{"id":"T339","span":{"begin":5761,"end":5764},"obj":"http://purl.obolibrary.org/obo/CLO_0050236"},{"id":"T340","span":{"begin":5791,"end":5794},"obj":"http://purl.obolibrary.org/obo/CLO_0050236"},{"id":"T341","span":{"begin":5812,"end":5815},"obj":"http://purl.obolibrary.org/obo/CLO_0050236"},{"id":"T342","span":{"begin":5834,"end":5837},"obj":"http://purl.obolibrary.org/obo/CLO_0050236"},{"id":"T343","span":{"begin":5859,"end":5862},"obj":"http://purl.obolibrary.org/obo/CLO_0050236"},{"id":"T344","span":{"begin":5935,"end":5938},"obj":"http://purl.obolibrary.org/obo/CLO_0050236"},{"id":"T345","span":{"begin":6014,"end":6017},"obj":"http://purl.obolibrary.org/obo/CLO_0050236"},{"id":"T346","span":{"begin":6045,"end":6048},"obj":"http://purl.obolibrary.org/obo/CLO_0050236"},{"id":"T347","span":{"begin":6075,"end":6078},"obj":"http://purl.obolibrary.org/obo/CLO_0050236"},{"id":"T348","span":{"begin":6106,"end":6109},"obj":"http://purl.obolibrary.org/obo/CLO_0050236"},{"id":"T349","span":{"begin":6137,"end":6140},"obj":"http://purl.obolibrary.org/obo/CLO_0050236"},{"id":"T350","span":{"begin":6159,"end":6162},"obj":"http://purl.obolibrary.org/obo/CLO_0050236"}],"text":"Appendix A. First stage regressions\nWeather conditions affect disease transmissions either directly if the virus can more easily survive and spread in certain environment, or indirectly by changing human behavior. Table 9 reports results of the first stage of the IV regressions (Table 4) using the full sample. In columns (1) and (2), the dependent variables are the numbers of newly confirmed COVID-19 cases in the own city in the preceding first and second weeks, respectively. In columns (3) and (4), the dependent variables are the sum of inverse log distance weighted numbers of newly confirmed COVID-19 cases in other cities in the preceding first and second weeks, respectively. These are the endogenous variables in the IV regressions. The weather variables in the preceding first and second weeks are included in the control variables. The weather variables in the preceding third and fourth weeks are the excluded instruments, and their coefficients are reported in the table. Because the variables are averages in 7-day moving windows, the error term may be serially correlated, and we include city by week fixed effects. Also included in the control variables are the average numbers of new cases in Wuhan in the preceding first and second weeks, interacted with the inverse log distance or the population flow.\nTable 9 First stage regressions\nDependent variable Average # of new cases\nOwn city Other cities\n1-week lag 2-week lag 1-week lag 2-week lag\n(1) (2) (3) (4)\nOwn City\nMaximum temperature, 3-week lag 0.200*** − 0.0431 0.564 − 2.022***\n(0.0579) (0.0503) (0.424) (0.417)\nPrecipitation, 3-week lag − 0.685 − 0.865* 4.516 − 1.998\n(0.552) (0.480) (4.045) (3.982)\nWind speed, 3-week lag 0.508** 0.299 − 0.827 3.247*\n(0.256) (0.223) (1.878) (1.849)\nPrecipitation × wind speed, 3-week lag − 0.412** 0.122 − 1.129 − 2.091\n(0.199) (0.173) (1.460) (1.437)\nMaximum temperature, 4-week lag 0.162*** 0.125** 1.379*** 1.181***\n(0.0560) (0.0487) (0.410) (0.404)\nPrecipitation, 4-week lag 0.0250 − 0.503 2.667 8.952***\n(0.440) (0.383) (3.224) (3.174)\nWind speed, 4-week lag 0.179 0.214 − 1.839 1.658\n(0.199) (0.173) (1.458) (1.435)\nPrecipitation × wind speed, 4-week lag − 0.354** − 0.0270 1.107 − 2.159**\n(0.145) (0.126) (1.059) (1.043)\nOther cities, weight = inverse distance\nMaximum temperature, 3-week lag − 0.0809*** − 0.00633 0.0520 1.152***\n(0.0203) (0.0176) (0.149) (0.146)\nPrecipitation, 3-week lag 4.366*** − 2.370*** 17.99*** − 72.68***\n(0.639) (0.556) (4.684) (4.611)\nWind speed, 3-week lag 0.326*** − 0.222** − 1.456 − 11.02***\n(0.126) (0.110) (0.926) (0.912)\nPrecipitation × wind speed, 3-week lag − 1.780*** 0.724*** − 6.750*** 27.73***\n(0.227) (0.197) (1.663) (1.637)\nMaximum temperature, 4-week lag − 0.0929*** − 0.0346* − 0.518*** 0.0407\n(0.0220) (0.0191) (0.161) (0.159)\nPrecipitation, 4-week lag 3.357*** − 0.578 46.57*** − 25.31***\n(0.504) (0.438) (3.691) (3.633)\nWind speed, 4-week lag 0.499*** 0.214** 4.660*** − 4.639***\n(0.107) (0.0934) (0.787) (0.774)\nPrecipitation × wind speed, 4-week lag − 1.358*** − 0.0416 − 17.26*** 8.967***\n(0.178) (0.155) (1.303) (1.282)\nF statistic 11.41 8.46 19.10 36.32\np value 0.0000 0.0000 0.0000 0.0000\nObservations 12,768 12,768 12,768 12,768\nNumber of cities 304 304 304 304\n# cases in Wuhan Yes Yes Yes Yes\nContemporaneous weather controls Yes Yes Yes Yes\nCity FE Yes Yes Yes Yes\nDate FE Yes Yes Yes Yes\nCity by week FE Yes Yes Yes Yes\nThis table shows the results of the first stage IV regressions. The weather variables are weekly averages of daily weather readings. Coefficients of the weather variables which are used as excluded instrumental variables are reported. *** p \u003c 0.01, ** p \u003c 0.05, * p \u003c 0.1\nBecause the spread of the virus depends on both the number of infectious people and the weather conditions, the coefficients in the first stage regressions do not have structural interpretations. The Wald tests on the joint significance of the excluded instruments are conducted and their F statistics are reported. The excluded instruments have good predictive power.\nThe implementation of local public health measures is likely correlated with the extent of the virus spread, so weather conditions that affect virus transmissions may also affect the likelihood that the policy is adopted. The influence of weather conditions on policy adoption may be complicated, so we use the Cluster-Lasso method of Belloni et al. (2016) to select the weather variables that have good predictive power on the adoption of closed management of communities or family outdoor restrictions. Let dct be the dummy variable of the adoption of the local public health measure, i.e., dct = 1 if the policy is in place in city c at day t. qct is a vector of candidate weather variables, including weekly averages of daily mean temperature, maximum temperature, minimum temperature, dew point, station-level pressure, sea-level pressure, visibility, wind speed, maximum wind speed, snow depth, precipitation, dummy for adverse weather conditions, squared terms of these variables, and interactions among them. First, city and day fixed effects are removed. d¨ct=dct−1n∑cdct−1T∑tdct+1nT∑ctdct and q¨ct is defined similarly. The Cluster-Lasso method solves the following minimization problem: 1nT∑ctd¨ct−q¨ct′b2+λnT∑kϕk|bk|.λ and ϕ are penalty parameters. A larger penalty value forces more coefficients to zero. The penalty parameters are picked using the theoretical result of Belloni et al. (2016). The estimation uses the Stata package by Ahrens et al. (2019). Table 10 lists the selected weather variables, which are used as the instruments in Table 8.\nTable 10 Variables selected\nDependent variable: closed management of communities\nDew point 1-week lag\nDiurnal temperature range 1-week lag\nDew point 2-week lag\nSea-level pressure 2-week lag\nDew point 3-week lag\nVisibility 4-week lag\nPrecipitation 4-week lag\nDependent variable: family outdoor restrictions\nStation pressure 1-week lag\nDummy for adverse weather conditions such as fog, rain, and drizzle 1-week lag\nMaximum temperature 2-week lag\nSea-level pressure 2-week lag\nAverage temperature 3-week lag\nMinimum temperature 3-week lag\nVisibility 3-week lag\nThis table shows the weather variables selected by lassopack (Ahrens et al. 2019), which implements the Cluster-Lasso method of Belloni et al. (2016). City and date fixed effects are included. Candidate variables include weekly averages of daily mean temperature, maximum temperature, minimum temperature, dew point, station-level pressure, sea-level pressure, visibility, wind speed, maximum wind speed, snow depth, precipitation, dummy for adverse weather conditions, squared terms of these variables, and interactions among them"}
LitCovid-PD-GO-BP
{"project":"LitCovid-PD-GO-BP","denotations":[{"id":"T15","span":{"begin":204,"end":212},"obj":"http://purl.obolibrary.org/obo/GO_0007610"}],"text":"Appendix A. First stage regressions\nWeather conditions affect disease transmissions either directly if the virus can more easily survive and spread in certain environment, or indirectly by changing human behavior. Table 9 reports results of the first stage of the IV regressions (Table 4) using the full sample. In columns (1) and (2), the dependent variables are the numbers of newly confirmed COVID-19 cases in the own city in the preceding first and second weeks, respectively. In columns (3) and (4), the dependent variables are the sum of inverse log distance weighted numbers of newly confirmed COVID-19 cases in other cities in the preceding first and second weeks, respectively. These are the endogenous variables in the IV regressions. The weather variables in the preceding first and second weeks are included in the control variables. The weather variables in the preceding third and fourth weeks are the excluded instruments, and their coefficients are reported in the table. Because the variables are averages in 7-day moving windows, the error term may be serially correlated, and we include city by week fixed effects. Also included in the control variables are the average numbers of new cases in Wuhan in the preceding first and second weeks, interacted with the inverse log distance or the population flow.\nTable 9 First stage regressions\nDependent variable Average # of new cases\nOwn city Other cities\n1-week lag 2-week lag 1-week lag 2-week lag\n(1) (2) (3) (4)\nOwn City\nMaximum temperature, 3-week lag 0.200*** − 0.0431 0.564 − 2.022***\n(0.0579) (0.0503) (0.424) (0.417)\nPrecipitation, 3-week lag − 0.685 − 0.865* 4.516 − 1.998\n(0.552) (0.480) (4.045) (3.982)\nWind speed, 3-week lag 0.508** 0.299 − 0.827 3.247*\n(0.256) (0.223) (1.878) (1.849)\nPrecipitation × wind speed, 3-week lag − 0.412** 0.122 − 1.129 − 2.091\n(0.199) (0.173) (1.460) (1.437)\nMaximum temperature, 4-week lag 0.162*** 0.125** 1.379*** 1.181***\n(0.0560) (0.0487) (0.410) (0.404)\nPrecipitation, 4-week lag 0.0250 − 0.503 2.667 8.952***\n(0.440) (0.383) (3.224) (3.174)\nWind speed, 4-week lag 0.179 0.214 − 1.839 1.658\n(0.199) (0.173) (1.458) (1.435)\nPrecipitation × wind speed, 4-week lag − 0.354** − 0.0270 1.107 − 2.159**\n(0.145) (0.126) (1.059) (1.043)\nOther cities, weight = inverse distance\nMaximum temperature, 3-week lag − 0.0809*** − 0.00633 0.0520 1.152***\n(0.0203) (0.0176) (0.149) (0.146)\nPrecipitation, 3-week lag 4.366*** − 2.370*** 17.99*** − 72.68***\n(0.639) (0.556) (4.684) (4.611)\nWind speed, 3-week lag 0.326*** − 0.222** − 1.456 − 11.02***\n(0.126) (0.110) (0.926) (0.912)\nPrecipitation × wind speed, 3-week lag − 1.780*** 0.724*** − 6.750*** 27.73***\n(0.227) (0.197) (1.663) (1.637)\nMaximum temperature, 4-week lag − 0.0929*** − 0.0346* − 0.518*** 0.0407\n(0.0220) (0.0191) (0.161) (0.159)\nPrecipitation, 4-week lag 3.357*** − 0.578 46.57*** − 25.31***\n(0.504) (0.438) (3.691) (3.633)\nWind speed, 4-week lag 0.499*** 0.214** 4.660*** − 4.639***\n(0.107) (0.0934) (0.787) (0.774)\nPrecipitation × wind speed, 4-week lag − 1.358*** − 0.0416 − 17.26*** 8.967***\n(0.178) (0.155) (1.303) (1.282)\nF statistic 11.41 8.46 19.10 36.32\np value 0.0000 0.0000 0.0000 0.0000\nObservations 12,768 12,768 12,768 12,768\nNumber of cities 304 304 304 304\n# cases in Wuhan Yes Yes Yes Yes\nContemporaneous weather controls Yes Yes Yes Yes\nCity FE Yes Yes Yes Yes\nDate FE Yes Yes Yes Yes\nCity by week FE Yes Yes Yes Yes\nThis table shows the results of the first stage IV regressions. The weather variables are weekly averages of daily weather readings. Coefficients of the weather variables which are used as excluded instrumental variables are reported. *** p \u003c 0.01, ** p \u003c 0.05, * p \u003c 0.1\nBecause the spread of the virus depends on both the number of infectious people and the weather conditions, the coefficients in the first stage regressions do not have structural interpretations. The Wald tests on the joint significance of the excluded instruments are conducted and their F statistics are reported. The excluded instruments have good predictive power.\nThe implementation of local public health measures is likely correlated with the extent of the virus spread, so weather conditions that affect virus transmissions may also affect the likelihood that the policy is adopted. The influence of weather conditions on policy adoption may be complicated, so we use the Cluster-Lasso method of Belloni et al. (2016) to select the weather variables that have good predictive power on the adoption of closed management of communities or family outdoor restrictions. Let dct be the dummy variable of the adoption of the local public health measure, i.e., dct = 1 if the policy is in place in city c at day t. qct is a vector of candidate weather variables, including weekly averages of daily mean temperature, maximum temperature, minimum temperature, dew point, station-level pressure, sea-level pressure, visibility, wind speed, maximum wind speed, snow depth, precipitation, dummy for adverse weather conditions, squared terms of these variables, and interactions among them. First, city and day fixed effects are removed. d¨ct=dct−1n∑cdct−1T∑tdct+1nT∑ctdct and q¨ct is defined similarly. The Cluster-Lasso method solves the following minimization problem: 1nT∑ctd¨ct−q¨ct′b2+λnT∑kϕk|bk|.λ and ϕ are penalty parameters. A larger penalty value forces more coefficients to zero. The penalty parameters are picked using the theoretical result of Belloni et al. (2016). The estimation uses the Stata package by Ahrens et al. (2019). Table 10 lists the selected weather variables, which are used as the instruments in Table 8.\nTable 10 Variables selected\nDependent variable: closed management of communities\nDew point 1-week lag\nDiurnal temperature range 1-week lag\nDew point 2-week lag\nSea-level pressure 2-week lag\nDew point 3-week lag\nVisibility 4-week lag\nPrecipitation 4-week lag\nDependent variable: family outdoor restrictions\nStation pressure 1-week lag\nDummy for adverse weather conditions such as fog, rain, and drizzle 1-week lag\nMaximum temperature 2-week lag\nSea-level pressure 2-week lag\nAverage temperature 3-week lag\nMinimum temperature 3-week lag\nVisibility 3-week lag\nThis table shows the weather variables selected by lassopack (Ahrens et al. 2019), which implements the Cluster-Lasso method of Belloni et al. (2016). City and date fixed effects are included. Candidate variables include weekly averages of daily mean temperature, maximum temperature, minimum temperature, dew point, station-level pressure, sea-level pressure, visibility, wind speed, maximum wind speed, snow depth, precipitation, dummy for adverse weather conditions, squared terms of these variables, and interactions among them"}
LitCovid-sentences
{"project":"LitCovid-sentences","denotations":[{"id":"T699","span":{"begin":0,"end":11},"obj":"Sentence"},{"id":"T700","span":{"begin":12,"end":35},"obj":"Sentence"},{"id":"T701","span":{"begin":36,"end":213},"obj":"Sentence"},{"id":"T702","span":{"begin":214,"end":311},"obj":"Sentence"},{"id":"T703","span":{"begin":312,"end":480},"obj":"Sentence"},{"id":"T704","span":{"begin":481,"end":686},"obj":"Sentence"},{"id":"T705","span":{"begin":687,"end":744},"obj":"Sentence"},{"id":"T706","span":{"begin":745,"end":845},"obj":"Sentence"},{"id":"T707","span":{"begin":846,"end":987},"obj":"Sentence"},{"id":"T708","span":{"begin":988,"end":1133},"obj":"Sentence"},{"id":"T709","span":{"begin":1134,"end":1324},"obj":"Sentence"},{"id":"T710","span":{"begin":1325,"end":1356},"obj":"Sentence"},{"id":"T711","span":{"begin":1357,"end":1398},"obj":"Sentence"},{"id":"T712","span":{"begin":1399,"end":1420},"obj":"Sentence"},{"id":"T713","span":{"begin":1421,"end":1464},"obj":"Sentence"},{"id":"T714","span":{"begin":1465,"end":1480},"obj":"Sentence"},{"id":"T715","span":{"begin":1481,"end":1489},"obj":"Sentence"},{"id":"T716","span":{"begin":1490,"end":1556},"obj":"Sentence"},{"id":"T717","span":{"begin":1557,"end":1590},"obj":"Sentence"},{"id":"T718","span":{"begin":1591,"end":1647},"obj":"Sentence"},{"id":"T719","span":{"begin":1648,"end":1679},"obj":"Sentence"},{"id":"T720","span":{"begin":1680,"end":1731},"obj":"Sentence"},{"id":"T721","span":{"begin":1732,"end":1763},"obj":"Sentence"},{"id":"T722","span":{"begin":1764,"end":1834},"obj":"Sentence"},{"id":"T723","span":{"begin":1835,"end":1866},"obj":"Sentence"},{"id":"T724","span":{"begin":1867,"end":1933},"obj":"Sentence"},{"id":"T725","span":{"begin":1934,"end":1967},"obj":"Sentence"},{"id":"T726","span":{"begin":1968,"end":2023},"obj":"Sentence"},{"id":"T727","span":{"begin":2024,"end":2055},"obj":"Sentence"},{"id":"T728","span":{"begin":2056,"end":2104},"obj":"Sentence"},{"id":"T729","span":{"begin":2105,"end":2136},"obj":"Sentence"},{"id":"T730","span":{"begin":2137,"end":2210},"obj":"Sentence"},{"id":"T731","span":{"begin":2211,"end":2242},"obj":"Sentence"},{"id":"T732","span":{"begin":2243,"end":2282},"obj":"Sentence"},{"id":"T733","span":{"begin":2283,"end":2352},"obj":"Sentence"},{"id":"T734","span":{"begin":2353,"end":2386},"obj":"Sentence"},{"id":"T735","span":{"begin":2387,"end":2452},"obj":"Sentence"},{"id":"T736","span":{"begin":2453,"end":2484},"obj":"Sentence"},{"id":"T737","span":{"begin":2485,"end":2545},"obj":"Sentence"},{"id":"T738","span":{"begin":2546,"end":2577},"obj":"Sentence"},{"id":"T739","span":{"begin":2578,"end":2656},"obj":"Sentence"},{"id":"T740","span":{"begin":2657,"end":2688},"obj":"Sentence"},{"id":"T741","span":{"begin":2689,"end":2760},"obj":"Sentence"},{"id":"T742","span":{"begin":2761,"end":2794},"obj":"Sentence"},{"id":"T743","span":{"begin":2795,"end":2857},"obj":"Sentence"},{"id":"T744","span":{"begin":2858,"end":2889},"obj":"Sentence"},{"id":"T745","span":{"begin":2890,"end":2949},"obj":"Sentence"},{"id":"T746","span":{"begin":2950,"end":2982},"obj":"Sentence"},{"id":"T747","span":{"begin":2983,"end":3061},"obj":"Sentence"},{"id":"T748","span":{"begin":3062,"end":3093},"obj":"Sentence"},{"id":"T749","span":{"begin":3094,"end":3128},"obj":"Sentence"},{"id":"T750","span":{"begin":3129,"end":3164},"obj":"Sentence"},{"id":"T751","span":{"begin":3165,"end":3205},"obj":"Sentence"},{"id":"T752","span":{"begin":3206,"end":3238},"obj":"Sentence"},{"id":"T753","span":{"begin":3239,"end":3271},"obj":"Sentence"},{"id":"T754","span":{"begin":3272,"end":3320},"obj":"Sentence"},{"id":"T755","span":{"begin":3321,"end":3344},"obj":"Sentence"},{"id":"T756","span":{"begin":3345,"end":3368},"obj":"Sentence"},{"id":"T757","span":{"begin":3369,"end":3400},"obj":"Sentence"},{"id":"T758","span":{"begin":3401,"end":3464},"obj":"Sentence"},{"id":"T759","span":{"begin":3465,"end":3533},"obj":"Sentence"},{"id":"T760","span":{"begin":3534,"end":3672},"obj":"Sentence"},{"id":"T761","span":{"begin":3673,"end":3868},"obj":"Sentence"},{"id":"T762","span":{"begin":3869,"end":3988},"obj":"Sentence"},{"id":"T763","span":{"begin":3989,"end":4041},"obj":"Sentence"},{"id":"T764","span":{"begin":4042,"end":4263},"obj":"Sentence"},{"id":"T765","span":{"begin":4264,"end":4546},"obj":"Sentence"},{"id":"T766","span":{"begin":4547,"end":5058},"obj":"Sentence"},{"id":"T767","span":{"begin":5059,"end":5171},"obj":"Sentence"},{"id":"T768","span":{"begin":5172,"end":5239},"obj":"Sentence"},{"id":"T769","span":{"begin":5240,"end":5302},"obj":"Sentence"},{"id":"T770","span":{"begin":5303,"end":5359},"obj":"Sentence"},{"id":"T771","span":{"begin":5360,"end":5448},"obj":"Sentence"},{"id":"T772","span":{"begin":5449,"end":5511},"obj":"Sentence"},{"id":"T773","span":{"begin":5512,"end":5604},"obj":"Sentence"},{"id":"T774","span":{"begin":5605,"end":5632},"obj":"Sentence"},{"id":"T775","span":{"begin":5633,"end":5685},"obj":"Sentence"},{"id":"T776","span":{"begin":5686,"end":5706},"obj":"Sentence"},{"id":"T777","span":{"begin":5707,"end":5743},"obj":"Sentence"},{"id":"T778","span":{"begin":5744,"end":5764},"obj":"Sentence"},{"id":"T779","span":{"begin":5765,"end":5794},"obj":"Sentence"},{"id":"T780","span":{"begin":5795,"end":5815},"obj":"Sentence"},{"id":"T781","span":{"begin":5816,"end":5837},"obj":"Sentence"},{"id":"T782","span":{"begin":5838,"end":5862},"obj":"Sentence"},{"id":"T783","span":{"begin":5863,"end":5910},"obj":"Sentence"},{"id":"T784","span":{"begin":5911,"end":5938},"obj":"Sentence"},{"id":"T785","span":{"begin":5939,"end":6017},"obj":"Sentence"},{"id":"T786","span":{"begin":6018,"end":6048},"obj":"Sentence"},{"id":"T787","span":{"begin":6049,"end":6078},"obj":"Sentence"},{"id":"T788","span":{"begin":6079,"end":6109},"obj":"Sentence"},{"id":"T789","span":{"begin":6110,"end":6140},"obj":"Sentence"},{"id":"T790","span":{"begin":6141,"end":6162},"obj":"Sentence"},{"id":"T791","span":{"begin":6163,"end":6238},"obj":"Sentence"},{"id":"T792","span":{"begin":6239,"end":6313},"obj":"Sentence"},{"id":"T793","span":{"begin":6314,"end":6355},"obj":"Sentence"},{"id":"T794","span":{"begin":6356,"end":6694},"obj":"Sentence"}],"namespaces":[{"prefix":"_base","uri":"http://pubannotation.org/ontology/tao.owl#"}],"text":"Appendix A. First stage regressions\nWeather conditions affect disease transmissions either directly if the virus can more easily survive and spread in certain environment, or indirectly by changing human behavior. Table 9 reports results of the first stage of the IV regressions (Table 4) using the full sample. In columns (1) and (2), the dependent variables are the numbers of newly confirmed COVID-19 cases in the own city in the preceding first and second weeks, respectively. In columns (3) and (4), the dependent variables are the sum of inverse log distance weighted numbers of newly confirmed COVID-19 cases in other cities in the preceding first and second weeks, respectively. These are the endogenous variables in the IV regressions. The weather variables in the preceding first and second weeks are included in the control variables. The weather variables in the preceding third and fourth weeks are the excluded instruments, and their coefficients are reported in the table. Because the variables are averages in 7-day moving windows, the error term may be serially correlated, and we include city by week fixed effects. Also included in the control variables are the average numbers of new cases in Wuhan in the preceding first and second weeks, interacted with the inverse log distance or the population flow.\nTable 9 First stage regressions\nDependent variable Average # of new cases\nOwn city Other cities\n1-week lag 2-week lag 1-week lag 2-week lag\n(1) (2) (3) (4)\nOwn City\nMaximum temperature, 3-week lag 0.200*** − 0.0431 0.564 − 2.022***\n(0.0579) (0.0503) (0.424) (0.417)\nPrecipitation, 3-week lag − 0.685 − 0.865* 4.516 − 1.998\n(0.552) (0.480) (4.045) (3.982)\nWind speed, 3-week lag 0.508** 0.299 − 0.827 3.247*\n(0.256) (0.223) (1.878) (1.849)\nPrecipitation × wind speed, 3-week lag − 0.412** 0.122 − 1.129 − 2.091\n(0.199) (0.173) (1.460) (1.437)\nMaximum temperature, 4-week lag 0.162*** 0.125** 1.379*** 1.181***\n(0.0560) (0.0487) (0.410) (0.404)\nPrecipitation, 4-week lag 0.0250 − 0.503 2.667 8.952***\n(0.440) (0.383) (3.224) (3.174)\nWind speed, 4-week lag 0.179 0.214 − 1.839 1.658\n(0.199) (0.173) (1.458) (1.435)\nPrecipitation × wind speed, 4-week lag − 0.354** − 0.0270 1.107 − 2.159**\n(0.145) (0.126) (1.059) (1.043)\nOther cities, weight = inverse distance\nMaximum temperature, 3-week lag − 0.0809*** − 0.00633 0.0520 1.152***\n(0.0203) (0.0176) (0.149) (0.146)\nPrecipitation, 3-week lag 4.366*** − 2.370*** 17.99*** − 72.68***\n(0.639) (0.556) (4.684) (4.611)\nWind speed, 3-week lag 0.326*** − 0.222** − 1.456 − 11.02***\n(0.126) (0.110) (0.926) (0.912)\nPrecipitation × wind speed, 3-week lag − 1.780*** 0.724*** − 6.750*** 27.73***\n(0.227) (0.197) (1.663) (1.637)\nMaximum temperature, 4-week lag − 0.0929*** − 0.0346* − 0.518*** 0.0407\n(0.0220) (0.0191) (0.161) (0.159)\nPrecipitation, 4-week lag 3.357*** − 0.578 46.57*** − 25.31***\n(0.504) (0.438) (3.691) (3.633)\nWind speed, 4-week lag 0.499*** 0.214** 4.660*** − 4.639***\n(0.107) (0.0934) (0.787) (0.774)\nPrecipitation × wind speed, 4-week lag − 1.358*** − 0.0416 − 17.26*** 8.967***\n(0.178) (0.155) (1.303) (1.282)\nF statistic 11.41 8.46 19.10 36.32\np value 0.0000 0.0000 0.0000 0.0000\nObservations 12,768 12,768 12,768 12,768\nNumber of cities 304 304 304 304\n# cases in Wuhan Yes Yes Yes Yes\nContemporaneous weather controls Yes Yes Yes Yes\nCity FE Yes Yes Yes Yes\nDate FE Yes Yes Yes Yes\nCity by week FE Yes Yes Yes Yes\nThis table shows the results of the first stage IV regressions. The weather variables are weekly averages of daily weather readings. Coefficients of the weather variables which are used as excluded instrumental variables are reported. *** p \u003c 0.01, ** p \u003c 0.05, * p \u003c 0.1\nBecause the spread of the virus depends on both the number of infectious people and the weather conditions, the coefficients in the first stage regressions do not have structural interpretations. The Wald tests on the joint significance of the excluded instruments are conducted and their F statistics are reported. The excluded instruments have good predictive power.\nThe implementation of local public health measures is likely correlated with the extent of the virus spread, so weather conditions that affect virus transmissions may also affect the likelihood that the policy is adopted. The influence of weather conditions on policy adoption may be complicated, so we use the Cluster-Lasso method of Belloni et al. (2016) to select the weather variables that have good predictive power on the adoption of closed management of communities or family outdoor restrictions. Let dct be the dummy variable of the adoption of the local public health measure, i.e., dct = 1 if the policy is in place in city c at day t. qct is a vector of candidate weather variables, including weekly averages of daily mean temperature, maximum temperature, minimum temperature, dew point, station-level pressure, sea-level pressure, visibility, wind speed, maximum wind speed, snow depth, precipitation, dummy for adverse weather conditions, squared terms of these variables, and interactions among them. First, city and day fixed effects are removed. d¨ct=dct−1n∑cdct−1T∑tdct+1nT∑ctdct and q¨ct is defined similarly. The Cluster-Lasso method solves the following minimization problem: 1nT∑ctd¨ct−q¨ct′b2+λnT∑kϕk|bk|.λ and ϕ are penalty parameters. A larger penalty value forces more coefficients to zero. The penalty parameters are picked using the theoretical result of Belloni et al. (2016). The estimation uses the Stata package by Ahrens et al. (2019). Table 10 lists the selected weather variables, which are used as the instruments in Table 8.\nTable 10 Variables selected\nDependent variable: closed management of communities\nDew point 1-week lag\nDiurnal temperature range 1-week lag\nDew point 2-week lag\nSea-level pressure 2-week lag\nDew point 3-week lag\nVisibility 4-week lag\nPrecipitation 4-week lag\nDependent variable: family outdoor restrictions\nStation pressure 1-week lag\nDummy for adverse weather conditions such as fog, rain, and drizzle 1-week lag\nMaximum temperature 2-week lag\nSea-level pressure 2-week lag\nAverage temperature 3-week lag\nMinimum temperature 3-week lag\nVisibility 3-week lag\nThis table shows the weather variables selected by lassopack (Ahrens et al. 2019), which implements the Cluster-Lasso method of Belloni et al. (2016). City and date fixed effects are included. Candidate variables include weekly averages of daily mean temperature, maximum temperature, minimum temperature, dew point, station-level pressure, sea-level pressure, visibility, wind speed, maximum wind speed, snow depth, precipitation, dummy for adverse weather conditions, squared terms of these variables, and interactions among them"}
LitCovid-PubTator
{"project":"LitCovid-PubTator","denotations":[{"id":"436","span":{"begin":1439,"end":1444},"obj":"Gene"},{"id":"437","span":{"begin":1450,"end":1455},"obj":"Gene"},{"id":"438","span":{"begin":2817,"end":2822},"obj":"Gene"},{"id":"439","span":{"begin":1428,"end":1433},"obj":"Gene"},{"id":"443","span":{"begin":198,"end":203},"obj":"Species"},{"id":"444","span":{"begin":395,"end":403},"obj":"Disease"},{"id":"445","span":{"begin":601,"end":609},"obj":"Disease"},{"id":"447","span":{"begin":3746,"end":3752},"obj":"Species"},{"id":"449","span":{"begin":5984,"end":5987},"obj":"Gene"},{"id":"451","span":{"begin":4635,"end":4642},"obj":"Gene"}],"attributes":[{"id":"A436","pred":"tao:has_database_id","subj":"436","obj":"Gene:388372"},{"id":"A437","pred":"tao:has_database_id","subj":"437","obj":"Gene:10578"},{"id":"A438","pred":"tao:has_database_id","subj":"438","obj":"Gene:3902"},{"id":"A439","pred":"tao:has_database_id","subj":"439","obj":"Gene:10578"},{"id":"A443","pred":"tao:has_database_id","subj":"443","obj":"Tax:9606"},{"id":"A444","pred":"tao:has_database_id","subj":"444","obj":"MESH:C000657245"},{"id":"A445","pred":"tao:has_database_id","subj":"445","obj":"MESH:C000657245"},{"id":"A447","pred":"tao:has_database_id","subj":"447","obj":"Tax:9606"},{"id":"A449","pred":"tao:has_database_id","subj":"449","obj":"Gene:161882"},{"id":"A451","pred":"tao:has_database_id","subj":"451","obj":"Gene:4891"}],"namespaces":[{"prefix":"Tax","uri":"https://www.ncbi.nlm.nih.gov/taxonomy/"},{"prefix":"MESH","uri":"https://id.nlm.nih.gov/mesh/"},{"prefix":"Gene","uri":"https://www.ncbi.nlm.nih.gov/gene/"},{"prefix":"CVCL","uri":"https://web.expasy.org/cellosaurus/CVCL_"}],"text":"Appendix A. First stage regressions\nWeather conditions affect disease transmissions either directly if the virus can more easily survive and spread in certain environment, or indirectly by changing human behavior. Table 9 reports results of the first stage of the IV regressions (Table 4) using the full sample. In columns (1) and (2), the dependent variables are the numbers of newly confirmed COVID-19 cases in the own city in the preceding first and second weeks, respectively. In columns (3) and (4), the dependent variables are the sum of inverse log distance weighted numbers of newly confirmed COVID-19 cases in other cities in the preceding first and second weeks, respectively. These are the endogenous variables in the IV regressions. The weather variables in the preceding first and second weeks are included in the control variables. The weather variables in the preceding third and fourth weeks are the excluded instruments, and their coefficients are reported in the table. Because the variables are averages in 7-day moving windows, the error term may be serially correlated, and we include city by week fixed effects. Also included in the control variables are the average numbers of new cases in Wuhan in the preceding first and second weeks, interacted with the inverse log distance or the population flow.\nTable 9 First stage regressions\nDependent variable Average # of new cases\nOwn city Other cities\n1-week lag 2-week lag 1-week lag 2-week lag\n(1) (2) (3) (4)\nOwn City\nMaximum temperature, 3-week lag 0.200*** − 0.0431 0.564 − 2.022***\n(0.0579) (0.0503) (0.424) (0.417)\nPrecipitation, 3-week lag − 0.685 − 0.865* 4.516 − 1.998\n(0.552) (0.480) (4.045) (3.982)\nWind speed, 3-week lag 0.508** 0.299 − 0.827 3.247*\n(0.256) (0.223) (1.878) (1.849)\nPrecipitation × wind speed, 3-week lag − 0.412** 0.122 − 1.129 − 2.091\n(0.199) (0.173) (1.460) (1.437)\nMaximum temperature, 4-week lag 0.162*** 0.125** 1.379*** 1.181***\n(0.0560) (0.0487) (0.410) (0.404)\nPrecipitation, 4-week lag 0.0250 − 0.503 2.667 8.952***\n(0.440) (0.383) (3.224) (3.174)\nWind speed, 4-week lag 0.179 0.214 − 1.839 1.658\n(0.199) (0.173) (1.458) (1.435)\nPrecipitation × wind speed, 4-week lag − 0.354** − 0.0270 1.107 − 2.159**\n(0.145) (0.126) (1.059) (1.043)\nOther cities, weight = inverse distance\nMaximum temperature, 3-week lag − 0.0809*** − 0.00633 0.0520 1.152***\n(0.0203) (0.0176) (0.149) (0.146)\nPrecipitation, 3-week lag 4.366*** − 2.370*** 17.99*** − 72.68***\n(0.639) (0.556) (4.684) (4.611)\nWind speed, 3-week lag 0.326*** − 0.222** − 1.456 − 11.02***\n(0.126) (0.110) (0.926) (0.912)\nPrecipitation × wind speed, 3-week lag − 1.780*** 0.724*** − 6.750*** 27.73***\n(0.227) (0.197) (1.663) (1.637)\nMaximum temperature, 4-week lag − 0.0929*** − 0.0346* − 0.518*** 0.0407\n(0.0220) (0.0191) (0.161) (0.159)\nPrecipitation, 4-week lag 3.357*** − 0.578 46.57*** − 25.31***\n(0.504) (0.438) (3.691) (3.633)\nWind speed, 4-week lag 0.499*** 0.214** 4.660*** − 4.639***\n(0.107) (0.0934) (0.787) (0.774)\nPrecipitation × wind speed, 4-week lag − 1.358*** − 0.0416 − 17.26*** 8.967***\n(0.178) (0.155) (1.303) (1.282)\nF statistic 11.41 8.46 19.10 36.32\np value 0.0000 0.0000 0.0000 0.0000\nObservations 12,768 12,768 12,768 12,768\nNumber of cities 304 304 304 304\n# cases in Wuhan Yes Yes Yes Yes\nContemporaneous weather controls Yes Yes Yes Yes\nCity FE Yes Yes Yes Yes\nDate FE Yes Yes Yes Yes\nCity by week FE Yes Yes Yes Yes\nThis table shows the results of the first stage IV regressions. The weather variables are weekly averages of daily weather readings. Coefficients of the weather variables which are used as excluded instrumental variables are reported. *** p \u003c 0.01, ** p \u003c 0.05, * p \u003c 0.1\nBecause the spread of the virus depends on both the number of infectious people and the weather conditions, the coefficients in the first stage regressions do not have structural interpretations. The Wald tests on the joint significance of the excluded instruments are conducted and their F statistics are reported. The excluded instruments have good predictive power.\nThe implementation of local public health measures is likely correlated with the extent of the virus spread, so weather conditions that affect virus transmissions may also affect the likelihood that the policy is adopted. The influence of weather conditions on policy adoption may be complicated, so we use the Cluster-Lasso method of Belloni et al. (2016) to select the weather variables that have good predictive power on the adoption of closed management of communities or family outdoor restrictions. Let dct be the dummy variable of the adoption of the local public health measure, i.e., dct = 1 if the policy is in place in city c at day t. qct is a vector of candidate weather variables, including weekly averages of daily mean temperature, maximum temperature, minimum temperature, dew point, station-level pressure, sea-level pressure, visibility, wind speed, maximum wind speed, snow depth, precipitation, dummy for adverse weather conditions, squared terms of these variables, and interactions among them. First, city and day fixed effects are removed. d¨ct=dct−1n∑cdct−1T∑tdct+1nT∑ctdct and q¨ct is defined similarly. The Cluster-Lasso method solves the following minimization problem: 1nT∑ctd¨ct−q¨ct′b2+λnT∑kϕk|bk|.λ and ϕ are penalty parameters. A larger penalty value forces more coefficients to zero. The penalty parameters are picked using the theoretical result of Belloni et al. (2016). The estimation uses the Stata package by Ahrens et al. (2019). Table 10 lists the selected weather variables, which are used as the instruments in Table 8.\nTable 10 Variables selected\nDependent variable: closed management of communities\nDew point 1-week lag\nDiurnal temperature range 1-week lag\nDew point 2-week lag\nSea-level pressure 2-week lag\nDew point 3-week lag\nVisibility 4-week lag\nPrecipitation 4-week lag\nDependent variable: family outdoor restrictions\nStation pressure 1-week lag\nDummy for adverse weather conditions such as fog, rain, and drizzle 1-week lag\nMaximum temperature 2-week lag\nSea-level pressure 2-week lag\nAverage temperature 3-week lag\nMinimum temperature 3-week lag\nVisibility 3-week lag\nThis table shows the weather variables selected by lassopack (Ahrens et al. 2019), which implements the Cluster-Lasso method of Belloni et al. (2016). City and date fixed effects are included. Candidate variables include weekly averages of daily mean temperature, maximum temperature, minimum temperature, dew point, station-level pressure, sea-level pressure, visibility, wind speed, maximum wind speed, snow depth, precipitation, dummy for adverse weather conditions, squared terms of these variables, and interactions among them"}