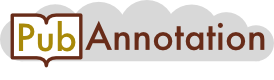
PMC:7210464 / 75447-78512
Annnotations
LitCovid-PD-MONDO
{"project":"LitCovid-PD-MONDO","denotations":[{"id":"T160","span":{"begin":64,"end":88},"obj":"Disease"},{"id":"T161","span":{"begin":291,"end":304},"obj":"Disease"},{"id":"T162","span":{"begin":453,"end":462},"obj":"Disease"},{"id":"T163","span":{"begin":523,"end":533},"obj":"Disease"},{"id":"T164","span":{"begin":579,"end":589},"obj":"Disease"},{"id":"T165","span":{"begin":698,"end":708},"obj":"Disease"},{"id":"T166","span":{"begin":780,"end":788},"obj":"Disease"},{"id":"T167","span":{"begin":1065,"end":1073},"obj":"Disease"},{"id":"T168","span":{"begin":1181,"end":1189},"obj":"Disease"},{"id":"T169","span":{"begin":1190,"end":1200},"obj":"Disease"},{"id":"T170","span":{"begin":1923,"end":1933},"obj":"Disease"},{"id":"T171","span":{"begin":2070,"end":2080},"obj":"Disease"},{"id":"T172","span":{"begin":2813,"end":2821},"obj":"Disease"},{"id":"T173","span":{"begin":2885,"end":2895},"obj":"Disease"}],"attributes":[{"id":"A160","pred":"mondo_id","subj":"T160","obj":"http://purl.obolibrary.org/obo/MONDO_0100096"},{"id":"A161","pred":"mondo_id","subj":"T161","obj":"http://purl.obolibrary.org/obo/MONDO_0005550"},{"id":"A162","pred":"mondo_id","subj":"T162","obj":"http://purl.obolibrary.org/obo/MONDO_0005550"},{"id":"A163","pred":"mondo_id","subj":"T163","obj":"http://purl.obolibrary.org/obo/MONDO_0005550"},{"id":"A164","pred":"mondo_id","subj":"T164","obj":"http://purl.obolibrary.org/obo/MONDO_0005550"},{"id":"A165","pred":"mondo_id","subj":"T165","obj":"http://purl.obolibrary.org/obo/MONDO_0005550"},{"id":"A166","pred":"mondo_id","subj":"T166","obj":"http://purl.obolibrary.org/obo/MONDO_0100096"},{"id":"A167","pred":"mondo_id","subj":"T167","obj":"http://purl.obolibrary.org/obo/MONDO_0100096"},{"id":"A168","pred":"mondo_id","subj":"T168","obj":"http://purl.obolibrary.org/obo/MONDO_0100096"},{"id":"A169","pred":"mondo_id","subj":"T169","obj":"http://purl.obolibrary.org/obo/MONDO_0005550"},{"id":"A170","pred":"mondo_id","subj":"T170","obj":"http://purl.obolibrary.org/obo/MONDO_0005550"},{"id":"A171","pred":"mondo_id","subj":"T171","obj":"http://purl.obolibrary.org/obo/MONDO_0005550"},{"id":"A172","pred":"mondo_id","subj":"T172","obj":"http://purl.obolibrary.org/obo/MONDO_0100096"},{"id":"A173","pred":"mondo_id","subj":"T173","obj":"http://purl.obolibrary.org/obo/MONDO_0005550"}],"text":"Conclusion\nThis paper examines the transmission dynamics of the coronavirus disease 2019 in China, considering both within- and between-city transmissions. Our sample is from January 19 to February 29 and covers key episodes such as the initial spread of the virus across China, the peak of infections in terms of domestic case counts, and the gradual containment of the virus in China. Changes in weather conditions induce exogenous variations in past infection rates, which allow us to identify the causal impact of past infections on new cases. The estimates suggest that the infectious effect of the existing cases is mostly observed within 1 week and people’s responses can break the chain of infections. Comparing estimates in two sub-samples, we observe that the spread of COVID-19 has been effectively contained by mid February, especially for cities outside Hubei province. Data on real-time population flows between cities have become available in recent years. We show that this new source of data is valuable in explaining between-city transmissions of COVID-19, even after controlling for traditional measures of geographic and economic proximity.\nBy April 5 of 2020, COVID-19 infections have been reported in more than 200 countries or territories and more than 64,700 people have died. Behind the grim statistics, more and more national and local governments are implementing countermeasures. Cross border travel restrictions are imposed in order to reduce the risk of case importation. In areas with risks of community transmissions, public health measures such as social distancing, mandatory quarantine, and city lockdown are implemented. In a series of counterfactual simulations, we find that based on the experience in China, preventing sustained community transmissions from taking hold in the first place has the largest impact, followed by restricting population flows from areas with high risks of infections. Local public health measures such as closed management of communities and family outdoor restrictions can further reduce the number of infections.\nA key limitation of the paper is that we are not able to disentangle the effects from each of the stringent measures taken, as within this 6-week sampling period, China enforced such a large number of densely timed policies to contain the virus spreading, often simultaneously in many cities. A second limitation is that shortly after the starting date of the official data release for confirmed infected cases throughout China, i.e., January 19, 2020, many stringent measures were implemented, which prevents researchers to compare the post treatment sub-sample with a pre treatment sub-sample during which no strict policies were enforced. Key knowledge gaps remain in the understanding of the epidemiological characteristics of COVID-19, such as individual risk factors for contracting the virus and infections from asymptotic cases. Data on the demographics and exposure history for those who have shown symptoms as well as those who have not will help facilitate these research."}
LitCovid-PD-CLO
{"project":"LitCovid-PD-CLO","denotations":[{"id":"T285","span":{"begin":259,"end":264},"obj":"http://purl.obolibrary.org/obo/NCBITaxon_10239"},{"id":"T286","span":{"begin":371,"end":376},"obj":"http://purl.obolibrary.org/obo/NCBITaxon_10239"},{"id":"T287","span":{"begin":789,"end":792},"obj":"http://purl.obolibrary.org/obo/CLO_0051582"},{"id":"T288","span":{"begin":1660,"end":1661},"obj":"http://purl.obolibrary.org/obo/CLO_0001020"},{"id":"T289","span":{"begin":1828,"end":1831},"obj":"http://purl.obolibrary.org/obo/CLO_0051582"},{"id":"T290","span":{"begin":2082,"end":2083},"obj":"http://purl.obolibrary.org/obo/CLO_0001020"},{"id":"T291","span":{"begin":2265,"end":2266},"obj":"http://purl.obolibrary.org/obo/CLO_0001020"},{"id":"T292","span":{"begin":2321,"end":2326},"obj":"http://purl.obolibrary.org/obo/NCBITaxon_10239"},{"id":"T293","span":{"begin":2375,"end":2376},"obj":"http://purl.obolibrary.org/obo/CLO_0001020"},{"id":"T294","span":{"begin":2650,"end":2651},"obj":"http://purl.obolibrary.org/obo/CLO_0001020"},{"id":"T295","span":{"begin":2875,"end":2880},"obj":"http://purl.obolibrary.org/obo/NCBITaxon_10239"}],"text":"Conclusion\nThis paper examines the transmission dynamics of the coronavirus disease 2019 in China, considering both within- and between-city transmissions. Our sample is from January 19 to February 29 and covers key episodes such as the initial spread of the virus across China, the peak of infections in terms of domestic case counts, and the gradual containment of the virus in China. Changes in weather conditions induce exogenous variations in past infection rates, which allow us to identify the causal impact of past infections on new cases. The estimates suggest that the infectious effect of the existing cases is mostly observed within 1 week and people’s responses can break the chain of infections. Comparing estimates in two sub-samples, we observe that the spread of COVID-19 has been effectively contained by mid February, especially for cities outside Hubei province. Data on real-time population flows between cities have become available in recent years. We show that this new source of data is valuable in explaining between-city transmissions of COVID-19, even after controlling for traditional measures of geographic and economic proximity.\nBy April 5 of 2020, COVID-19 infections have been reported in more than 200 countries or territories and more than 64,700 people have died. Behind the grim statistics, more and more national and local governments are implementing countermeasures. Cross border travel restrictions are imposed in order to reduce the risk of case importation. In areas with risks of community transmissions, public health measures such as social distancing, mandatory quarantine, and city lockdown are implemented. In a series of counterfactual simulations, we find that based on the experience in China, preventing sustained community transmissions from taking hold in the first place has the largest impact, followed by restricting population flows from areas with high risks of infections. Local public health measures such as closed management of communities and family outdoor restrictions can further reduce the number of infections.\nA key limitation of the paper is that we are not able to disentangle the effects from each of the stringent measures taken, as within this 6-week sampling period, China enforced such a large number of densely timed policies to contain the virus spreading, often simultaneously in many cities. A second limitation is that shortly after the starting date of the official data release for confirmed infected cases throughout China, i.e., January 19, 2020, many stringent measures were implemented, which prevents researchers to compare the post treatment sub-sample with a pre treatment sub-sample during which no strict policies were enforced. Key knowledge gaps remain in the understanding of the epidemiological characteristics of COVID-19, such as individual risk factors for contracting the virus and infections from asymptotic cases. Data on the demographics and exposure history for those who have shown symptoms as well as those who have not will help facilitate these research."}
LitCovid-sentences
{"project":"LitCovid-sentences","denotations":[{"id":"T676","span":{"begin":0,"end":10},"obj":"Sentence"},{"id":"T677","span":{"begin":11,"end":155},"obj":"Sentence"},{"id":"T678","span":{"begin":156,"end":386},"obj":"Sentence"},{"id":"T679","span":{"begin":387,"end":547},"obj":"Sentence"},{"id":"T680","span":{"begin":548,"end":709},"obj":"Sentence"},{"id":"T681","span":{"begin":710,"end":882},"obj":"Sentence"},{"id":"T682","span":{"begin":883,"end":971},"obj":"Sentence"},{"id":"T683","span":{"begin":972,"end":1160},"obj":"Sentence"},{"id":"T684","span":{"begin":1161,"end":1300},"obj":"Sentence"},{"id":"T685","span":{"begin":1301,"end":1407},"obj":"Sentence"},{"id":"T686","span":{"begin":1408,"end":1501},"obj":"Sentence"},{"id":"T687","span":{"begin":1502,"end":1656},"obj":"Sentence"},{"id":"T688","span":{"begin":1657,"end":1934},"obj":"Sentence"},{"id":"T689","span":{"begin":1935,"end":2081},"obj":"Sentence"},{"id":"T690","span":{"begin":2082,"end":2374},"obj":"Sentence"},{"id":"T691","span":{"begin":2375,"end":2723},"obj":"Sentence"},{"id":"T692","span":{"begin":2724,"end":2918},"obj":"Sentence"},{"id":"T693","span":{"begin":2919,"end":3065},"obj":"Sentence"}],"namespaces":[{"prefix":"_base","uri":"http://pubannotation.org/ontology/tao.owl#"}],"text":"Conclusion\nThis paper examines the transmission dynamics of the coronavirus disease 2019 in China, considering both within- and between-city transmissions. Our sample is from January 19 to February 29 and covers key episodes such as the initial spread of the virus across China, the peak of infections in terms of domestic case counts, and the gradual containment of the virus in China. Changes in weather conditions induce exogenous variations in past infection rates, which allow us to identify the causal impact of past infections on new cases. The estimates suggest that the infectious effect of the existing cases is mostly observed within 1 week and people’s responses can break the chain of infections. Comparing estimates in two sub-samples, we observe that the spread of COVID-19 has been effectively contained by mid February, especially for cities outside Hubei province. Data on real-time population flows between cities have become available in recent years. We show that this new source of data is valuable in explaining between-city transmissions of COVID-19, even after controlling for traditional measures of geographic and economic proximity.\nBy April 5 of 2020, COVID-19 infections have been reported in more than 200 countries or territories and more than 64,700 people have died. Behind the grim statistics, more and more national and local governments are implementing countermeasures. Cross border travel restrictions are imposed in order to reduce the risk of case importation. In areas with risks of community transmissions, public health measures such as social distancing, mandatory quarantine, and city lockdown are implemented. In a series of counterfactual simulations, we find that based on the experience in China, preventing sustained community transmissions from taking hold in the first place has the largest impact, followed by restricting population flows from areas with high risks of infections. Local public health measures such as closed management of communities and family outdoor restrictions can further reduce the number of infections.\nA key limitation of the paper is that we are not able to disentangle the effects from each of the stringent measures taken, as within this 6-week sampling period, China enforced such a large number of densely timed policies to contain the virus spreading, often simultaneously in many cities. A second limitation is that shortly after the starting date of the official data release for confirmed infected cases throughout China, i.e., January 19, 2020, many stringent measures were implemented, which prevents researchers to compare the post treatment sub-sample with a pre treatment sub-sample during which no strict policies were enforced. Key knowledge gaps remain in the understanding of the epidemiological characteristics of COVID-19, such as individual risk factors for contracting the virus and infections from asymptotic cases. Data on the demographics and exposure history for those who have shown symptoms as well as those who have not will help facilitate these research."}
LitCovid-PubTator
{"project":"LitCovid-PubTator","denotations":[{"id":"404","span":{"begin":656,"end":662},"obj":"Species"},{"id":"405","span":{"begin":64,"end":88},"obj":"Disease"},{"id":"406","span":{"begin":291,"end":301},"obj":"Disease"},{"id":"407","span":{"begin":453,"end":462},"obj":"Disease"},{"id":"408","span":{"begin":523,"end":533},"obj":"Disease"},{"id":"409","span":{"begin":698,"end":708},"obj":"Disease"},{"id":"410","span":{"begin":780,"end":788},"obj":"Disease"},{"id":"411","span":{"begin":1065,"end":1073},"obj":"Disease"},{"id":"418","span":{"begin":1283,"end":1289},"obj":"Species"},{"id":"419","span":{"begin":1181,"end":1189},"obj":"Disease"},{"id":"420","span":{"begin":1190,"end":1200},"obj":"Disease"},{"id":"421","span":{"begin":1295,"end":1299},"obj":"Disease"},{"id":"422","span":{"begin":1923,"end":1933},"obj":"Disease"},{"id":"423","span":{"begin":2070,"end":2080},"obj":"Disease"},{"id":"427","span":{"begin":2478,"end":2486},"obj":"Disease"},{"id":"428","span":{"begin":2813,"end":2821},"obj":"Disease"},{"id":"429","span":{"begin":2885,"end":2895},"obj":"Disease"}],"attributes":[{"id":"A404","pred":"tao:has_database_id","subj":"404","obj":"Tax:9606"},{"id":"A405","pred":"tao:has_database_id","subj":"405","obj":"MESH:C000657245"},{"id":"A406","pred":"tao:has_database_id","subj":"406","obj":"MESH:D007239"},{"id":"A407","pred":"tao:has_database_id","subj":"407","obj":"MESH:D007239"},{"id":"A408","pred":"tao:has_database_id","subj":"408","obj":"MESH:D007239"},{"id":"A409","pred":"tao:has_database_id","subj":"409","obj":"MESH:D007239"},{"id":"A410","pred":"tao:has_database_id","subj":"410","obj":"MESH:C000657245"},{"id":"A411","pred":"tao:has_database_id","subj":"411","obj":"MESH:C000657245"},{"id":"A418","pred":"tao:has_database_id","subj":"418","obj":"Tax:9606"},{"id":"A419","pred":"tao:has_database_id","subj":"419","obj":"MESH:C000657245"},{"id":"A420","pred":"tao:has_database_id","subj":"420","obj":"MESH:D007239"},{"id":"A421","pred":"tao:has_database_id","subj":"421","obj":"MESH:D003643"},{"id":"A422","pred":"tao:has_database_id","subj":"422","obj":"MESH:D007239"},{"id":"A423","pred":"tao:has_database_id","subj":"423","obj":"MESH:D007239"},{"id":"A427","pred":"tao:has_database_id","subj":"427","obj":"MESH:D007239"},{"id":"A428","pred":"tao:has_database_id","subj":"428","obj":"MESH:C000657245"},{"id":"A429","pred":"tao:has_database_id","subj":"429","obj":"MESH:D007239"}],"namespaces":[{"prefix":"Tax","uri":"https://www.ncbi.nlm.nih.gov/taxonomy/"},{"prefix":"MESH","uri":"https://id.nlm.nih.gov/mesh/"},{"prefix":"Gene","uri":"https://www.ncbi.nlm.nih.gov/gene/"},{"prefix":"CVCL","uri":"https://web.expasy.org/cellosaurus/CVCL_"}],"text":"Conclusion\nThis paper examines the transmission dynamics of the coronavirus disease 2019 in China, considering both within- and between-city transmissions. Our sample is from January 19 to February 29 and covers key episodes such as the initial spread of the virus across China, the peak of infections in terms of domestic case counts, and the gradual containment of the virus in China. Changes in weather conditions induce exogenous variations in past infection rates, which allow us to identify the causal impact of past infections on new cases. The estimates suggest that the infectious effect of the existing cases is mostly observed within 1 week and people’s responses can break the chain of infections. Comparing estimates in two sub-samples, we observe that the spread of COVID-19 has been effectively contained by mid February, especially for cities outside Hubei province. Data on real-time population flows between cities have become available in recent years. We show that this new source of data is valuable in explaining between-city transmissions of COVID-19, even after controlling for traditional measures of geographic and economic proximity.\nBy April 5 of 2020, COVID-19 infections have been reported in more than 200 countries or territories and more than 64,700 people have died. Behind the grim statistics, more and more national and local governments are implementing countermeasures. Cross border travel restrictions are imposed in order to reduce the risk of case importation. In areas with risks of community transmissions, public health measures such as social distancing, mandatory quarantine, and city lockdown are implemented. In a series of counterfactual simulations, we find that based on the experience in China, preventing sustained community transmissions from taking hold in the first place has the largest impact, followed by restricting population flows from areas with high risks of infections. Local public health measures such as closed management of communities and family outdoor restrictions can further reduce the number of infections.\nA key limitation of the paper is that we are not able to disentangle the effects from each of the stringent measures taken, as within this 6-week sampling period, China enforced such a large number of densely timed policies to contain the virus spreading, often simultaneously in many cities. A second limitation is that shortly after the starting date of the official data release for confirmed infected cases throughout China, i.e., January 19, 2020, many stringent measures were implemented, which prevents researchers to compare the post treatment sub-sample with a pre treatment sub-sample during which no strict policies were enforced. Key knowledge gaps remain in the understanding of the epidemiological characteristics of COVID-19, such as individual risk factors for contracting the virus and infections from asymptotic cases. Data on the demographics and exposure history for those who have shown symptoms as well as those who have not will help facilitate these research."}