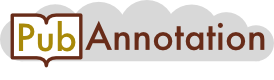
PMC:7210464 / 49205-54231
Annnotations
LitCovid-PD-MONDO
{"project":"LitCovid-PD-MONDO","denotations":[{"id":"T112","span":{"begin":1139,"end":1152},"obj":"Disease"},{"id":"T113","span":{"begin":1306,"end":1314},"obj":"Disease"},{"id":"T114","span":{"begin":1927,"end":1930},"obj":"Disease"},{"id":"T115","span":{"begin":1996,"end":1999},"obj":"Disease"},{"id":"T116","span":{"begin":2071,"end":2074},"obj":"Disease"},{"id":"T117","span":{"begin":2147,"end":2150},"obj":"Disease"},{"id":"T118","span":{"begin":2212,"end":2215},"obj":"Disease"},{"id":"T119","span":{"begin":2280,"end":2283},"obj":"Disease"},{"id":"T120","span":{"begin":4664,"end":4674},"obj":"Disease"}],"attributes":[{"id":"A112","pred":"mondo_id","subj":"T112","obj":"http://purl.obolibrary.org/obo/MONDO_0005550"},{"id":"A113","pred":"mondo_id","subj":"T113","obj":"http://purl.obolibrary.org/obo/MONDO_0100096"},{"id":"A114","pred":"mondo_id","subj":"T114","obj":"http://purl.obolibrary.org/obo/MONDO_0043678"},{"id":"A115","pred":"mondo_id","subj":"T115","obj":"http://purl.obolibrary.org/obo/MONDO_0043678"},{"id":"A116","pred":"mondo_id","subj":"T116","obj":"http://purl.obolibrary.org/obo/MONDO_0043678"},{"id":"A117","pred":"mondo_id","subj":"T117","obj":"http://purl.obolibrary.org/obo/MONDO_0043678"},{"id":"A118","pred":"mondo_id","subj":"T118","obj":"http://purl.obolibrary.org/obo/MONDO_0043678"},{"id":"A119","pred":"mondo_id","subj":"T119","obj":"http://purl.obolibrary.org/obo/MONDO_0043678"},{"id":"A120","pred":"mondo_id","subj":"T120","obj":"http://purl.obolibrary.org/obo/MONDO_0005550"}],"text":"Social and economic mediating factors\nWe also investigate the mediating impacts of some socioeconomic and environmental characteristics on the transmission rates (3). To ease the comparison between different moderators, we consider the mediating impacts on the influence of the average number of new cases in the past 2 weeks. Regarding own-city transmissions, we examine the mediating effects of population density, GDP per capita, number of doctors, and average temperature, wind speed, precipitation, and a dummy variable of adverse weather conditions. Regarding between-city transmissions, we consider the mediating effects of distance, difference in population density, and difference in GDP per capita since cities that are similar in density or economic development level may be more closely linked. We also include a measure of population flows from Wuhan. Table 6 reports the estimation results of the IV regressions. To ease the comparison across various moderators, for the mediating variables of within-city transmissions that are significant at 10%, we compute the changes in the variables so that the effect of new confirmed infections in the past 14 days on current new confirmed cases is reduced by 1 (columns (2) and (4)).\nTable 6 Social and economic factors mediating the transmission of COVID-19\n(1) (2) (3) (4)\nJan 19–Feb 1 Feb 2–Feb 29\nIV Coeff. IV Coeff.\nAverage # of new cases, previous 14 days\nOwn city − 0.251 0.672***\n(0.977) (0.219)\n× population density 0.000164 − 0.000202** + 495 per km2\n(0.000171) (8.91e-05)\n× per capita GDP 0.150*** − 66, 667 RMB 0.0102\n(0.0422) (0.0196)\n× # of doctors − 0.108* + 92, 593 0.0179\n(0.0622) (0.0236)\n× temperature 0.0849* − 11.78∘C − 0.00945\n(0.0438) (0.0126)\n× wind speed − 0.109 0.128\n(0.131) (0.114)\n× precipitation 0.965* − 1.04 mm 0.433* − 2.31 mm\n(0.555) (0.229)\n× adverse weather 0.0846 − 0.614*** + 163%\n(0.801) (0.208)\nOther cities 0.0356 − 0.00429\nwt. = inv. distance (0.0375) (0.00343)\nOther cities 0.00222 0.000192\nwt. = inv. density ratio (0.00147) (0.000891)\nOther cities 0.00232 0.00107\nwt. = inv. per capita GDP ratio (0.00497) (0.00165)\nWuhan − 0.165 − 0.00377\nwt. = inv. distance (0.150) (0.00981)\nWuhan − 0.00336 − 0.000849\nwt. = inv. density ratio (0.00435) (0.00111)\nWuhan − 0.440 − 0.0696\nwt. = inv. per capita GDP ratio (0.318) (0.0699)\nWuhan 0.00729*** 0.0125***\nwt. = population flow (0.00202) (0.00187)\nObservations 4032 8064\nNumber of cities 288 288\nWeather controls Yes Yes\nCity FE Yes Yes\nDate FE Yes Yes\nThe dependent variable is the number of daily new confirmed cases. The sample excludes cities in Hubei province. Columns (2) and (4) report the changes in the mediating variables that are needed to reduce the impact of new confirmed cases in the preceding 2 weeks by 1, using estimates with significance levels of at least 0.1 in columns (1) and (3), respectively. The endogenous variables include the average numbers of new cases in the own city and nearby cities in the preceding 14 days and their interactions with the mediating variables. Weekly averages of daily maximum temperature, precipitation, wind speed, the interaction between precipitation and wind speed, and the inverse log distance weighted sum of these variables in neighboring cities, during the preceding third and fourth weeks, are used as instrumental variables in the IV regressions. Additional instrumental variables are constructed by interacting them with the mediating variables. Weather controls include these variables in the preceding first and second weeks. Standard errors in parentheses are clustered by provinces\n*** p \u003c 0.01, ** p \u003c 0.05, * p \u003c 0.1\nIn the early phase of the epidemic (January 19 to February 1), cities with more medical resources, which are measured by the number of doctors, have lower transmission rates. One standard deviation increase in the number of doctors reduces the transmission rate by 0.12. Cities with higher GDP per capita have higher transmission rates, which can be ascribed to the increased social interactions as economic activities increase18. In the second sub-sample, these effects become insignificant probably because public health measures and inter-city resource sharing take effects. In fact, cities with higher population density have lower transmission rates in the second sub-sample. Regarding the environmental factors, we notice different significant mediating variables across the first and second sub-samples. The transmission rates are lower with adverse weather conditions, lower temperature, or less rain. Further research is needed to identify clear mechanisms. In addition, population flow from Wuhan still poses a risk of new infections for other cities even after we account for the above mediating effects on own-city transmission. This effect is robust to the inclusion of the proximity measures based on economic similarity and geographic proximity between Wuhan and other cities. Nevertheless, we do not find much evidence on between-city transmissions among cities other than Wuhan."}
LitCovid-PD-CLO
{"project":"LitCovid-PD-CLO","denotations":[{"id":"T209","span":{"begin":508,"end":509},"obj":"http://purl.obolibrary.org/obo/CLO_0001020"},{"id":"T210","span":{"begin":823,"end":824},"obj":"http://purl.obolibrary.org/obo/CLO_0001020"},{"id":"T211","span":{"begin":1324,"end":1329},"obj":"http://purl.obolibrary.org/obo/CLO_0001302"},{"id":"T212","span":{"begin":1870,"end":1873},"obj":"http://purl.obolibrary.org/obo/CLO_0001003"},{"id":"T213","span":{"begin":3308,"end":3320},"obj":"http://purl.obolibrary.org/obo/OBI_0000968"},{"id":"T214","span":{"begin":3365,"end":3377},"obj":"http://purl.obolibrary.org/obo/OBI_0000968"},{"id":"T215","span":{"begin":4039,"end":4049},"obj":"http://purl.obolibrary.org/obo/CLO_0001658"},{"id":"T216","span":{"begin":4650,"end":4651},"obj":"http://purl.obolibrary.org/obo/CLO_0001020"}],"text":"Social and economic mediating factors\nWe also investigate the mediating impacts of some socioeconomic and environmental characteristics on the transmission rates (3). To ease the comparison between different moderators, we consider the mediating impacts on the influence of the average number of new cases in the past 2 weeks. Regarding own-city transmissions, we examine the mediating effects of population density, GDP per capita, number of doctors, and average temperature, wind speed, precipitation, and a dummy variable of adverse weather conditions. Regarding between-city transmissions, we consider the mediating effects of distance, difference in population density, and difference in GDP per capita since cities that are similar in density or economic development level may be more closely linked. We also include a measure of population flows from Wuhan. Table 6 reports the estimation results of the IV regressions. To ease the comparison across various moderators, for the mediating variables of within-city transmissions that are significant at 10%, we compute the changes in the variables so that the effect of new confirmed infections in the past 14 days on current new confirmed cases is reduced by 1 (columns (2) and (4)).\nTable 6 Social and economic factors mediating the transmission of COVID-19\n(1) (2) (3) (4)\nJan 19–Feb 1 Feb 2–Feb 29\nIV Coeff. IV Coeff.\nAverage # of new cases, previous 14 days\nOwn city − 0.251 0.672***\n(0.977) (0.219)\n× population density 0.000164 − 0.000202** + 495 per km2\n(0.000171) (8.91e-05)\n× per capita GDP 0.150*** − 66, 667 RMB 0.0102\n(0.0422) (0.0196)\n× # of doctors − 0.108* + 92, 593 0.0179\n(0.0622) (0.0236)\n× temperature 0.0849* − 11.78∘C − 0.00945\n(0.0438) (0.0126)\n× wind speed − 0.109 0.128\n(0.131) (0.114)\n× precipitation 0.965* − 1.04 mm 0.433* − 2.31 mm\n(0.555) (0.229)\n× adverse weather 0.0846 − 0.614*** + 163%\n(0.801) (0.208)\nOther cities 0.0356 − 0.00429\nwt. = inv. distance (0.0375) (0.00343)\nOther cities 0.00222 0.000192\nwt. = inv. density ratio (0.00147) (0.000891)\nOther cities 0.00232 0.00107\nwt. = inv. per capita GDP ratio (0.00497) (0.00165)\nWuhan − 0.165 − 0.00377\nwt. = inv. distance (0.150) (0.00981)\nWuhan − 0.00336 − 0.000849\nwt. = inv. density ratio (0.00435) (0.00111)\nWuhan − 0.440 − 0.0696\nwt. = inv. per capita GDP ratio (0.318) (0.0699)\nWuhan 0.00729*** 0.0125***\nwt. = population flow (0.00202) (0.00187)\nObservations 4032 8064\nNumber of cities 288 288\nWeather controls Yes Yes\nCity FE Yes Yes\nDate FE Yes Yes\nThe dependent variable is the number of daily new confirmed cases. The sample excludes cities in Hubei province. Columns (2) and (4) report the changes in the mediating variables that are needed to reduce the impact of new confirmed cases in the preceding 2 weeks by 1, using estimates with significance levels of at least 0.1 in columns (1) and (3), respectively. The endogenous variables include the average numbers of new cases in the own city and nearby cities in the preceding 14 days and their interactions with the mediating variables. Weekly averages of daily maximum temperature, precipitation, wind speed, the interaction between precipitation and wind speed, and the inverse log distance weighted sum of these variables in neighboring cities, during the preceding third and fourth weeks, are used as instrumental variables in the IV regressions. Additional instrumental variables are constructed by interacting them with the mediating variables. Weather controls include these variables in the preceding first and second weeks. Standard errors in parentheses are clustered by provinces\n*** p \u003c 0.01, ** p \u003c 0.05, * p \u003c 0.1\nIn the early phase of the epidemic (January 19 to February 1), cities with more medical resources, which are measured by the number of doctors, have lower transmission rates. One standard deviation increase in the number of doctors reduces the transmission rate by 0.12. Cities with higher GDP per capita have higher transmission rates, which can be ascribed to the increased social interactions as economic activities increase18. In the second sub-sample, these effects become insignificant probably because public health measures and inter-city resource sharing take effects. In fact, cities with higher population density have lower transmission rates in the second sub-sample. Regarding the environmental factors, we notice different significant mediating variables across the first and second sub-samples. The transmission rates are lower with adverse weather conditions, lower temperature, or less rain. Further research is needed to identify clear mechanisms. In addition, population flow from Wuhan still poses a risk of new infections for other cities even after we account for the above mediating effects on own-city transmission. This effect is robust to the inclusion of the proximity measures based on economic similarity and geographic proximity between Wuhan and other cities. Nevertheless, we do not find much evidence on between-city transmissions among cities other than Wuhan."}
LitCovid-PD-HP
{"project":"LitCovid-PD-HP","denotations":[{"id":"T8","span":{"begin":4007,"end":4026},"obj":"Phenotype"},{"id":"T8","span":{"begin":4007,"end":4026},"obj":"Phenotype"}],"attributes":[{"id":"A8","pred":"hp_id","subj":"T8","obj":"http://purl.obolibrary.org/obo/HP_0008763"},{"id":"A8","pred":"hp_id","subj":"T8","obj":"http://purl.obolibrary.org/obo/HP_0008763"}],"text":"Social and economic mediating factors\nWe also investigate the mediating impacts of some socioeconomic and environmental characteristics on the transmission rates (3). To ease the comparison between different moderators, we consider the mediating impacts on the influence of the average number of new cases in the past 2 weeks. Regarding own-city transmissions, we examine the mediating effects of population density, GDP per capita, number of doctors, and average temperature, wind speed, precipitation, and a dummy variable of adverse weather conditions. Regarding between-city transmissions, we consider the mediating effects of distance, difference in population density, and difference in GDP per capita since cities that are similar in density or economic development level may be more closely linked. We also include a measure of population flows from Wuhan. Table 6 reports the estimation results of the IV regressions. To ease the comparison across various moderators, for the mediating variables of within-city transmissions that are significant at 10%, we compute the changes in the variables so that the effect of new confirmed infections in the past 14 days on current new confirmed cases is reduced by 1 (columns (2) and (4)).\nTable 6 Social and economic factors mediating the transmission of COVID-19\n(1) (2) (3) (4)\nJan 19–Feb 1 Feb 2–Feb 29\nIV Coeff. IV Coeff.\nAverage # of new cases, previous 14 days\nOwn city − 0.251 0.672***\n(0.977) (0.219)\n× population density 0.000164 − 0.000202** + 495 per km2\n(0.000171) (8.91e-05)\n× per capita GDP 0.150*** − 66, 667 RMB 0.0102\n(0.0422) (0.0196)\n× # of doctors − 0.108* + 92, 593 0.0179\n(0.0622) (0.0236)\n× temperature 0.0849* − 11.78∘C − 0.00945\n(0.0438) (0.0126)\n× wind speed − 0.109 0.128\n(0.131) (0.114)\n× precipitation 0.965* − 1.04 mm 0.433* − 2.31 mm\n(0.555) (0.229)\n× adverse weather 0.0846 − 0.614*** + 163%\n(0.801) (0.208)\nOther cities 0.0356 − 0.00429\nwt. = inv. distance (0.0375) (0.00343)\nOther cities 0.00222 0.000192\nwt. = inv. density ratio (0.00147) (0.000891)\nOther cities 0.00232 0.00107\nwt. = inv. per capita GDP ratio (0.00497) (0.00165)\nWuhan − 0.165 − 0.00377\nwt. = inv. distance (0.150) (0.00981)\nWuhan − 0.00336 − 0.000849\nwt. = inv. density ratio (0.00435) (0.00111)\nWuhan − 0.440 − 0.0696\nwt. = inv. per capita GDP ratio (0.318) (0.0699)\nWuhan 0.00729*** 0.0125***\nwt. = population flow (0.00202) (0.00187)\nObservations 4032 8064\nNumber of cities 288 288\nWeather controls Yes Yes\nCity FE Yes Yes\nDate FE Yes Yes\nThe dependent variable is the number of daily new confirmed cases. The sample excludes cities in Hubei province. Columns (2) and (4) report the changes in the mediating variables that are needed to reduce the impact of new confirmed cases in the preceding 2 weeks by 1, using estimates with significance levels of at least 0.1 in columns (1) and (3), respectively. The endogenous variables include the average numbers of new cases in the own city and nearby cities in the preceding 14 days and their interactions with the mediating variables. Weekly averages of daily maximum temperature, precipitation, wind speed, the interaction between precipitation and wind speed, and the inverse log distance weighted sum of these variables in neighboring cities, during the preceding third and fourth weeks, are used as instrumental variables in the IV regressions. Additional instrumental variables are constructed by interacting them with the mediating variables. Weather controls include these variables in the preceding first and second weeks. Standard errors in parentheses are clustered by provinces\n*** p \u003c 0.01, ** p \u003c 0.05, * p \u003c 0.1\nIn the early phase of the epidemic (January 19 to February 1), cities with more medical resources, which are measured by the number of doctors, have lower transmission rates. One standard deviation increase in the number of doctors reduces the transmission rate by 0.12. Cities with higher GDP per capita have higher transmission rates, which can be ascribed to the increased social interactions as economic activities increase18. In the second sub-sample, these effects become insignificant probably because public health measures and inter-city resource sharing take effects. In fact, cities with higher population density have lower transmission rates in the second sub-sample. Regarding the environmental factors, we notice different significant mediating variables across the first and second sub-samples. The transmission rates are lower with adverse weather conditions, lower temperature, or less rain. Further research is needed to identify clear mechanisms. In addition, population flow from Wuhan still poses a risk of new infections for other cities even after we account for the above mediating effects on own-city transmission. This effect is robust to the inclusion of the proximity measures based on economic similarity and geographic proximity between Wuhan and other cities. Nevertheless, we do not find much evidence on between-city transmissions among cities other than Wuhan."}
LitCovid-sentences
{"project":"LitCovid-sentences","denotations":[{"id":"T419","span":{"begin":0,"end":37},"obj":"Sentence"},{"id":"T420","span":{"begin":38,"end":166},"obj":"Sentence"},{"id":"T421","span":{"begin":167,"end":326},"obj":"Sentence"},{"id":"T422","span":{"begin":327,"end":555},"obj":"Sentence"},{"id":"T423","span":{"begin":556,"end":806},"obj":"Sentence"},{"id":"T424","span":{"begin":807,"end":864},"obj":"Sentence"},{"id":"T425","span":{"begin":865,"end":926},"obj":"Sentence"},{"id":"T426","span":{"begin":927,"end":1239},"obj":"Sentence"},{"id":"T427","span":{"begin":1240,"end":1314},"obj":"Sentence"},{"id":"T428","span":{"begin":1315,"end":1330},"obj":"Sentence"},{"id":"T429","span":{"begin":1331,"end":1356},"obj":"Sentence"},{"id":"T430","span":{"begin":1357,"end":1366},"obj":"Sentence"},{"id":"T431","span":{"begin":1367,"end":1376},"obj":"Sentence"},{"id":"T432","span":{"begin":1377,"end":1417},"obj":"Sentence"},{"id":"T433","span":{"begin":1418,"end":1443},"obj":"Sentence"},{"id":"T434","span":{"begin":1444,"end":1459},"obj":"Sentence"},{"id":"T435","span":{"begin":1460,"end":1516},"obj":"Sentence"},{"id":"T436","span":{"begin":1517,"end":1538},"obj":"Sentence"},{"id":"T437","span":{"begin":1539,"end":1585},"obj":"Sentence"},{"id":"T438","span":{"begin":1586,"end":1603},"obj":"Sentence"},{"id":"T439","span":{"begin":1604,"end":1644},"obj":"Sentence"},{"id":"T440","span":{"begin":1645,"end":1662},"obj":"Sentence"},{"id":"T441","span":{"begin":1663,"end":1704},"obj":"Sentence"},{"id":"T442","span":{"begin":1705,"end":1722},"obj":"Sentence"},{"id":"T443","span":{"begin":1723,"end":1749},"obj":"Sentence"},{"id":"T444","span":{"begin":1750,"end":1765},"obj":"Sentence"},{"id":"T445","span":{"begin":1766,"end":1815},"obj":"Sentence"},{"id":"T446","span":{"begin":1816,"end":1831},"obj":"Sentence"},{"id":"T447","span":{"begin":1832,"end":1874},"obj":"Sentence"},{"id":"T448","span":{"begin":1875,"end":1890},"obj":"Sentence"},{"id":"T449","span":{"begin":1891,"end":1920},"obj":"Sentence"},{"id":"T450","span":{"begin":1921,"end":1959},"obj":"Sentence"},{"id":"T451","span":{"begin":1960,"end":1989},"obj":"Sentence"},{"id":"T452","span":{"begin":1990,"end":2035},"obj":"Sentence"},{"id":"T453","span":{"begin":2036,"end":2064},"obj":"Sentence"},{"id":"T454","span":{"begin":2065,"end":2116},"obj":"Sentence"},{"id":"T455","span":{"begin":2117,"end":2140},"obj":"Sentence"},{"id":"T456","span":{"begin":2141,"end":2178},"obj":"Sentence"},{"id":"T457","span":{"begin":2179,"end":2205},"obj":"Sentence"},{"id":"T458","span":{"begin":2206,"end":2250},"obj":"Sentence"},{"id":"T459","span":{"begin":2251,"end":2273},"obj":"Sentence"},{"id":"T460","span":{"begin":2274,"end":2322},"obj":"Sentence"},{"id":"T461","span":{"begin":2323,"end":2349},"obj":"Sentence"},{"id":"T462","span":{"begin":2350,"end":2391},"obj":"Sentence"},{"id":"T463","span":{"begin":2392,"end":2414},"obj":"Sentence"},{"id":"T464","span":{"begin":2415,"end":2439},"obj":"Sentence"},{"id":"T465","span":{"begin":2440,"end":2464},"obj":"Sentence"},{"id":"T466","span":{"begin":2465,"end":2480},"obj":"Sentence"},{"id":"T467","span":{"begin":2481,"end":2496},"obj":"Sentence"},{"id":"T468","span":{"begin":2497,"end":2563},"obj":"Sentence"},{"id":"T469","span":{"begin":2564,"end":2609},"obj":"Sentence"},{"id":"T470","span":{"begin":2610,"end":2861},"obj":"Sentence"},{"id":"T471","span":{"begin":2862,"end":3039},"obj":"Sentence"},{"id":"T472","span":{"begin":3040,"end":3353},"obj":"Sentence"},{"id":"T473","span":{"begin":3354,"end":3453},"obj":"Sentence"},{"id":"T474","span":{"begin":3454,"end":3535},"obj":"Sentence"},{"id":"T475","span":{"begin":3536,"end":3593},"obj":"Sentence"},{"id":"T476","span":{"begin":3594,"end":3630},"obj":"Sentence"},{"id":"T477","span":{"begin":3631,"end":3805},"obj":"Sentence"},{"id":"T478","span":{"begin":3806,"end":3901},"obj":"Sentence"},{"id":"T479","span":{"begin":3902,"end":4061},"obj":"Sentence"},{"id":"T480","span":{"begin":4062,"end":4208},"obj":"Sentence"},{"id":"T481","span":{"begin":4209,"end":4311},"obj":"Sentence"},{"id":"T482","span":{"begin":4312,"end":4441},"obj":"Sentence"},{"id":"T483","span":{"begin":4442,"end":4540},"obj":"Sentence"},{"id":"T484","span":{"begin":4541,"end":4597},"obj":"Sentence"},{"id":"T485","span":{"begin":4598,"end":4771},"obj":"Sentence"},{"id":"T486","span":{"begin":4772,"end":4922},"obj":"Sentence"},{"id":"T487","span":{"begin":4923,"end":5026},"obj":"Sentence"}],"namespaces":[{"prefix":"_base","uri":"http://pubannotation.org/ontology/tao.owl#"}],"text":"Social and economic mediating factors\nWe also investigate the mediating impacts of some socioeconomic and environmental characteristics on the transmission rates (3). To ease the comparison between different moderators, we consider the mediating impacts on the influence of the average number of new cases in the past 2 weeks. Regarding own-city transmissions, we examine the mediating effects of population density, GDP per capita, number of doctors, and average temperature, wind speed, precipitation, and a dummy variable of adverse weather conditions. Regarding between-city transmissions, we consider the mediating effects of distance, difference in population density, and difference in GDP per capita since cities that are similar in density or economic development level may be more closely linked. We also include a measure of population flows from Wuhan. Table 6 reports the estimation results of the IV regressions. To ease the comparison across various moderators, for the mediating variables of within-city transmissions that are significant at 10%, we compute the changes in the variables so that the effect of new confirmed infections in the past 14 days on current new confirmed cases is reduced by 1 (columns (2) and (4)).\nTable 6 Social and economic factors mediating the transmission of COVID-19\n(1) (2) (3) (4)\nJan 19–Feb 1 Feb 2–Feb 29\nIV Coeff. IV Coeff.\nAverage # of new cases, previous 14 days\nOwn city − 0.251 0.672***\n(0.977) (0.219)\n× population density 0.000164 − 0.000202** + 495 per km2\n(0.000171) (8.91e-05)\n× per capita GDP 0.150*** − 66, 667 RMB 0.0102\n(0.0422) (0.0196)\n× # of doctors − 0.108* + 92, 593 0.0179\n(0.0622) (0.0236)\n× temperature 0.0849* − 11.78∘C − 0.00945\n(0.0438) (0.0126)\n× wind speed − 0.109 0.128\n(0.131) (0.114)\n× precipitation 0.965* − 1.04 mm 0.433* − 2.31 mm\n(0.555) (0.229)\n× adverse weather 0.0846 − 0.614*** + 163%\n(0.801) (0.208)\nOther cities 0.0356 − 0.00429\nwt. = inv. distance (0.0375) (0.00343)\nOther cities 0.00222 0.000192\nwt. = inv. density ratio (0.00147) (0.000891)\nOther cities 0.00232 0.00107\nwt. = inv. per capita GDP ratio (0.00497) (0.00165)\nWuhan − 0.165 − 0.00377\nwt. = inv. distance (0.150) (0.00981)\nWuhan − 0.00336 − 0.000849\nwt. = inv. density ratio (0.00435) (0.00111)\nWuhan − 0.440 − 0.0696\nwt. = inv. per capita GDP ratio (0.318) (0.0699)\nWuhan 0.00729*** 0.0125***\nwt. = population flow (0.00202) (0.00187)\nObservations 4032 8064\nNumber of cities 288 288\nWeather controls Yes Yes\nCity FE Yes Yes\nDate FE Yes Yes\nThe dependent variable is the number of daily new confirmed cases. The sample excludes cities in Hubei province. Columns (2) and (4) report the changes in the mediating variables that are needed to reduce the impact of new confirmed cases in the preceding 2 weeks by 1, using estimates with significance levels of at least 0.1 in columns (1) and (3), respectively. The endogenous variables include the average numbers of new cases in the own city and nearby cities in the preceding 14 days and their interactions with the mediating variables. Weekly averages of daily maximum temperature, precipitation, wind speed, the interaction between precipitation and wind speed, and the inverse log distance weighted sum of these variables in neighboring cities, during the preceding third and fourth weeks, are used as instrumental variables in the IV regressions. Additional instrumental variables are constructed by interacting them with the mediating variables. Weather controls include these variables in the preceding first and second weeks. Standard errors in parentheses are clustered by provinces\n*** p \u003c 0.01, ** p \u003c 0.05, * p \u003c 0.1\nIn the early phase of the epidemic (January 19 to February 1), cities with more medical resources, which are measured by the number of doctors, have lower transmission rates. One standard deviation increase in the number of doctors reduces the transmission rate by 0.12. Cities with higher GDP per capita have higher transmission rates, which can be ascribed to the increased social interactions as economic activities increase18. In the second sub-sample, these effects become insignificant probably because public health measures and inter-city resource sharing take effects. In fact, cities with higher population density have lower transmission rates in the second sub-sample. Regarding the environmental factors, we notice different significant mediating variables across the first and second sub-samples. The transmission rates are lower with adverse weather conditions, lower temperature, or less rain. Further research is needed to identify clear mechanisms. In addition, population flow from Wuhan still poses a risk of new infections for other cities even after we account for the above mediating effects on own-city transmission. This effect is robust to the inclusion of the proximity measures based on economic similarity and geographic proximity between Wuhan and other cities. Nevertheless, we do not find much evidence on between-city transmissions among cities other than Wuhan."}
LitCovid-PubTator
{"project":"LitCovid-PubTator","denotations":[{"id":"277","span":{"begin":1338,"end":1349},"obj":"Gene"},{"id":"278","span":{"begin":2087,"end":2090},"obj":"Chemical"},{"id":"279","span":{"begin":2296,"end":2299},"obj":"Chemical"},{"id":"281","span":{"begin":1306,"end":1314},"obj":"Disease"},{"id":"284","span":{"begin":693,"end":696},"obj":"Chemical"},{"id":"285","span":{"begin":1139,"end":1149},"obj":"Disease"},{"id":"288","span":{"begin":3921,"end":3924},"obj":"Chemical"},{"id":"289","span":{"begin":4664,"end":4674},"obj":"Disease"}],"attributes":[{"id":"A277","pred":"tao:has_database_id","subj":"277","obj":"Gene:2233"},{"id":"A278","pred":"tao:has_database_id","subj":"278","obj":"MESH:D006153"},{"id":"A279","pred":"tao:has_database_id","subj":"279","obj":"MESH:D006153"},{"id":"A281","pred":"tao:has_database_id","subj":"281","obj":"MESH:C000657245"},{"id":"A284","pred":"tao:has_database_id","subj":"284","obj":"MESH:D006153"},{"id":"A285","pred":"tao:has_database_id","subj":"285","obj":"MESH:D007239"},{"id":"A288","pred":"tao:has_database_id","subj":"288","obj":"MESH:D006153"},{"id":"A289","pred":"tao:has_database_id","subj":"289","obj":"MESH:D007239"}],"namespaces":[{"prefix":"Tax","uri":"https://www.ncbi.nlm.nih.gov/taxonomy/"},{"prefix":"MESH","uri":"https://id.nlm.nih.gov/mesh/"},{"prefix":"Gene","uri":"https://www.ncbi.nlm.nih.gov/gene/"},{"prefix":"CVCL","uri":"https://web.expasy.org/cellosaurus/CVCL_"}],"text":"Social and economic mediating factors\nWe also investigate the mediating impacts of some socioeconomic and environmental characteristics on the transmission rates (3). To ease the comparison between different moderators, we consider the mediating impacts on the influence of the average number of new cases in the past 2 weeks. Regarding own-city transmissions, we examine the mediating effects of population density, GDP per capita, number of doctors, and average temperature, wind speed, precipitation, and a dummy variable of adverse weather conditions. Regarding between-city transmissions, we consider the mediating effects of distance, difference in population density, and difference in GDP per capita since cities that are similar in density or economic development level may be more closely linked. We also include a measure of population flows from Wuhan. Table 6 reports the estimation results of the IV regressions. To ease the comparison across various moderators, for the mediating variables of within-city transmissions that are significant at 10%, we compute the changes in the variables so that the effect of new confirmed infections in the past 14 days on current new confirmed cases is reduced by 1 (columns (2) and (4)).\nTable 6 Social and economic factors mediating the transmission of COVID-19\n(1) (2) (3) (4)\nJan 19–Feb 1 Feb 2–Feb 29\nIV Coeff. IV Coeff.\nAverage # of new cases, previous 14 days\nOwn city − 0.251 0.672***\n(0.977) (0.219)\n× population density 0.000164 − 0.000202** + 495 per km2\n(0.000171) (8.91e-05)\n× per capita GDP 0.150*** − 66, 667 RMB 0.0102\n(0.0422) (0.0196)\n× # of doctors − 0.108* + 92, 593 0.0179\n(0.0622) (0.0236)\n× temperature 0.0849* − 11.78∘C − 0.00945\n(0.0438) (0.0126)\n× wind speed − 0.109 0.128\n(0.131) (0.114)\n× precipitation 0.965* − 1.04 mm 0.433* − 2.31 mm\n(0.555) (0.229)\n× adverse weather 0.0846 − 0.614*** + 163%\n(0.801) (0.208)\nOther cities 0.0356 − 0.00429\nwt. = inv. distance (0.0375) (0.00343)\nOther cities 0.00222 0.000192\nwt. = inv. density ratio (0.00147) (0.000891)\nOther cities 0.00232 0.00107\nwt. = inv. per capita GDP ratio (0.00497) (0.00165)\nWuhan − 0.165 − 0.00377\nwt. = inv. distance (0.150) (0.00981)\nWuhan − 0.00336 − 0.000849\nwt. = inv. density ratio (0.00435) (0.00111)\nWuhan − 0.440 − 0.0696\nwt. = inv. per capita GDP ratio (0.318) (0.0699)\nWuhan 0.00729*** 0.0125***\nwt. = population flow (0.00202) (0.00187)\nObservations 4032 8064\nNumber of cities 288 288\nWeather controls Yes Yes\nCity FE Yes Yes\nDate FE Yes Yes\nThe dependent variable is the number of daily new confirmed cases. The sample excludes cities in Hubei province. Columns (2) and (4) report the changes in the mediating variables that are needed to reduce the impact of new confirmed cases in the preceding 2 weeks by 1, using estimates with significance levels of at least 0.1 in columns (1) and (3), respectively. The endogenous variables include the average numbers of new cases in the own city and nearby cities in the preceding 14 days and their interactions with the mediating variables. Weekly averages of daily maximum temperature, precipitation, wind speed, the interaction between precipitation and wind speed, and the inverse log distance weighted sum of these variables in neighboring cities, during the preceding third and fourth weeks, are used as instrumental variables in the IV regressions. Additional instrumental variables are constructed by interacting them with the mediating variables. Weather controls include these variables in the preceding first and second weeks. Standard errors in parentheses are clustered by provinces\n*** p \u003c 0.01, ** p \u003c 0.05, * p \u003c 0.1\nIn the early phase of the epidemic (January 19 to February 1), cities with more medical resources, which are measured by the number of doctors, have lower transmission rates. One standard deviation increase in the number of doctors reduces the transmission rate by 0.12. Cities with higher GDP per capita have higher transmission rates, which can be ascribed to the increased social interactions as economic activities increase18. In the second sub-sample, these effects become insignificant probably because public health measures and inter-city resource sharing take effects. In fact, cities with higher population density have lower transmission rates in the second sub-sample. Regarding the environmental factors, we notice different significant mediating variables across the first and second sub-samples. The transmission rates are lower with adverse weather conditions, lower temperature, or less rain. Further research is needed to identify clear mechanisms. In addition, population flow from Wuhan still poses a risk of new infections for other cities even after we account for the above mediating effects on own-city transmission. This effect is robust to the inclusion of the proximity measures based on economic similarity and geographic proximity between Wuhan and other cities. Nevertheless, we do not find much evidence on between-city transmissions among cities other than Wuhan."}