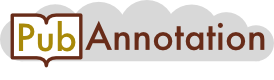
PMC:7210464 / 45729-47080
Annnotations
LitCovid-PD-MONDO
{"project":"LitCovid-PD-MONDO","denotations":[{"id":"T104","span":{"begin":418,"end":426},"obj":"Disease"}],"attributes":[{"id":"A104","pred":"mondo_id","subj":"T104","obj":"http://purl.obolibrary.org/obo/MONDO_0100096"}],"text":"While our basic reproduction number estimate (R0) is within the range of estimates in the literature and is close to its median, five features may distinguish our estimates from some of the existing epidemiological estimates. First, our instrumental variable approach helps isolate the causal effect of virus transmissions from other confounded factors; second, our estimate is based on an extended time period of the COVID-19 pandemic (until the end of February 2020) that may mitigate potential biases in the literature that relies on a shorter sampling period within 1–28 January 2020; third, our modeling makes minimum assumptions of virus transmissions, such as imposing fewer restrictions on the relationship between the unobserved determinants of new cases and the number of cases in the past; fourth, our model simultaneously considers comprehensive factors that may affect virus transmissions, including multiple policy instruments (such as closed management of communities and shelter-at-home order), population flow, within- and between-city transmissions, economic and demographic conditions, weather patterns, and preparedness of health care system. Fifth, our study uses spatially disaggregated data that cover China (except its Hubei province), while some other studies examine Wuhan city, Hubei province, China as a whole, or overseas."}
LitCovid-PD-CLO
{"project":"LitCovid-PD-CLO","denotations":[{"id":"T196","span":{"begin":237,"end":249},"obj":"http://purl.obolibrary.org/obo/OBI_0000968"},{"id":"T197","span":{"begin":303,"end":308},"obj":"http://purl.obolibrary.org/obo/NCBITaxon_10239"},{"id":"T198","span":{"begin":537,"end":538},"obj":"http://purl.obolibrary.org/obo/CLO_0001020"},{"id":"T199","span":{"begin":638,"end":643},"obj":"http://purl.obolibrary.org/obo/NCBITaxon_10239"},{"id":"T200","span":{"begin":882,"end":887},"obj":"http://purl.obolibrary.org/obo/NCBITaxon_10239"},{"id":"T201","span":{"begin":929,"end":940},"obj":"http://purl.obolibrary.org/obo/OBI_0000968"},{"id":"T202","span":{"begin":1330,"end":1331},"obj":"http://purl.obolibrary.org/obo/CLO_0001020"}],"text":"While our basic reproduction number estimate (R0) is within the range of estimates in the literature and is close to its median, five features may distinguish our estimates from some of the existing epidemiological estimates. First, our instrumental variable approach helps isolate the causal effect of virus transmissions from other confounded factors; second, our estimate is based on an extended time period of the COVID-19 pandemic (until the end of February 2020) that may mitigate potential biases in the literature that relies on a shorter sampling period within 1–28 January 2020; third, our modeling makes minimum assumptions of virus transmissions, such as imposing fewer restrictions on the relationship between the unobserved determinants of new cases and the number of cases in the past; fourth, our model simultaneously considers comprehensive factors that may affect virus transmissions, including multiple policy instruments (such as closed management of communities and shelter-at-home order), population flow, within- and between-city transmissions, economic and demographic conditions, weather patterns, and preparedness of health care system. Fifth, our study uses spatially disaggregated data that cover China (except its Hubei province), while some other studies examine Wuhan city, Hubei province, China as a whole, or overseas."}
LitCovid-PD-GO-BP
{"project":"LitCovid-PD-GO-BP","denotations":[{"id":"T8","span":{"begin":16,"end":28},"obj":"http://purl.obolibrary.org/obo/GO_0000003"}],"text":"While our basic reproduction number estimate (R0) is within the range of estimates in the literature and is close to its median, five features may distinguish our estimates from some of the existing epidemiological estimates. First, our instrumental variable approach helps isolate the causal effect of virus transmissions from other confounded factors; second, our estimate is based on an extended time period of the COVID-19 pandemic (until the end of February 2020) that may mitigate potential biases in the literature that relies on a shorter sampling period within 1–28 January 2020; third, our modeling makes minimum assumptions of virus transmissions, such as imposing fewer restrictions on the relationship between the unobserved determinants of new cases and the number of cases in the past; fourth, our model simultaneously considers comprehensive factors that may affect virus transmissions, including multiple policy instruments (such as closed management of communities and shelter-at-home order), population flow, within- and between-city transmissions, economic and demographic conditions, weather patterns, and preparedness of health care system. Fifth, our study uses spatially disaggregated data that cover China (except its Hubei province), while some other studies examine Wuhan city, Hubei province, China as a whole, or overseas."}
LitCovid-sentences
{"project":"LitCovid-sentences","denotations":[{"id":"T404","span":{"begin":0,"end":225},"obj":"Sentence"},{"id":"T405","span":{"begin":226,"end":1162},"obj":"Sentence"},{"id":"T406","span":{"begin":1163,"end":1351},"obj":"Sentence"}],"namespaces":[{"prefix":"_base","uri":"http://pubannotation.org/ontology/tao.owl#"}],"text":"While our basic reproduction number estimate (R0) is within the range of estimates in the literature and is close to its median, five features may distinguish our estimates from some of the existing epidemiological estimates. First, our instrumental variable approach helps isolate the causal effect of virus transmissions from other confounded factors; second, our estimate is based on an extended time period of the COVID-19 pandemic (until the end of February 2020) that may mitigate potential biases in the literature that relies on a shorter sampling period within 1–28 January 2020; third, our modeling makes minimum assumptions of virus transmissions, such as imposing fewer restrictions on the relationship between the unobserved determinants of new cases and the number of cases in the past; fourth, our model simultaneously considers comprehensive factors that may affect virus transmissions, including multiple policy instruments (such as closed management of communities and shelter-at-home order), population flow, within- and between-city transmissions, economic and demographic conditions, weather patterns, and preparedness of health care system. Fifth, our study uses spatially disaggregated data that cover China (except its Hubei province), while some other studies examine Wuhan city, Hubei province, China as a whole, or overseas."}
LitCovid-PubTator
{"project":"LitCovid-PubTator","denotations":[{"id":"253","span":{"begin":418,"end":426},"obj":"Disease"}],"attributes":[{"id":"A253","pred":"tao:has_database_id","subj":"253","obj":"MESH:C000657245"}],"namespaces":[{"prefix":"Tax","uri":"https://www.ncbi.nlm.nih.gov/taxonomy/"},{"prefix":"MESH","uri":"https://id.nlm.nih.gov/mesh/"},{"prefix":"Gene","uri":"https://www.ncbi.nlm.nih.gov/gene/"},{"prefix":"CVCL","uri":"https://web.expasy.org/cellosaurus/CVCL_"}],"text":"While our basic reproduction number estimate (R0) is within the range of estimates in the literature and is close to its median, five features may distinguish our estimates from some of the existing epidemiological estimates. First, our instrumental variable approach helps isolate the causal effect of virus transmissions from other confounded factors; second, our estimate is based on an extended time period of the COVID-19 pandemic (until the end of February 2020) that may mitigate potential biases in the literature that relies on a shorter sampling period within 1–28 January 2020; third, our modeling makes minimum assumptions of virus transmissions, such as imposing fewer restrictions on the relationship between the unobserved determinants of new cases and the number of cases in the past; fourth, our model simultaneously considers comprehensive factors that may affect virus transmissions, including multiple policy instruments (such as closed management of communities and shelter-at-home order), population flow, within- and between-city transmissions, economic and demographic conditions, weather patterns, and preparedness of health care system. Fifth, our study uses spatially disaggregated data that cover China (except its Hubei province), while some other studies examine Wuhan city, Hubei province, China as a whole, or overseas."}