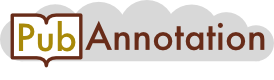
PMC:7210464 / 23926-27987
Annnotations
LitCovid-PD-FMA-UBERON
{"project":"LitCovid-PD-FMA-UBERON","denotations":[{"id":"T5","span":{"begin":1852,"end":1857},"obj":"Body_part"},{"id":"T6","span":{"begin":2031,"end":2039},"obj":"Body_part"},{"id":"T7","span":{"begin":2720,"end":2725},"obj":"Body_part"},{"id":"T8","span":{"begin":3678,"end":3686},"obj":"Body_part"},{"id":"T9","span":{"begin":4050,"end":4058},"obj":"Body_part"}],"attributes":[{"id":"A5","pred":"fma_id","subj":"T5","obj":"http://purl.org/sig/ont/fma/fma7490"},{"id":"A6","pred":"fma_id","subj":"T6","obj":"http://purl.org/sig/ont/fma/fma14542"},{"id":"A7","pred":"fma_id","subj":"T7","obj":"http://purl.org/sig/ont/fma/fma7490"},{"id":"A8","pred":"fma_id","subj":"T8","obj":"http://purl.org/sig/ont/fma/fma14542"},{"id":"A9","pred":"fma_id","subj":"T9","obj":"http://purl.org/sig/ont/fma/fma14542"}],"text":"Selection of instrumental variables\nThe transmission rate of COVID-19 may be affected by many environmental factors. Human-to-human transmission of COVID-19 is mostly through droplets and contacts (National Health Commission of the PRC 2020). Weather conditions such as rainfall, wind speed, and temperature may shape infections via their influences on social activities and virus transmissions. For instance, increased precipitation results in higher humidity, which may weaken virus transmissions (Lowen and Steel 2014). The virus may survive longer with lower temperature (Wang et al. 2020b; Puhani 2020). Greater wind speed and therefore ventilated air may decrease virus transmissions. In addition, increased rainfall and lower temperature may also reduce social activities. Newly confirmed COVID-19 cases typically arise from contracting the virus within 2 weeks in the past (e.g., World Health Organization 2020b). The extent of human-to-human transmission is determined by the number of people who have already contracted the virus and the environmental conditions within the next 2 weeks. Conditional on the number of people who are infectious and environmental conditions in the previous first and second weeks, it is plausible that weather conditions further in the past, i.e., in the previous third and fourth weeks, should not directly affect the number of current new cases. Based on the existing literature, we select weather characteristics as the instrumental variables, which include daily maximum temperature, precipitation, wind speed, and the interaction between precipitation and wind speed.\nWe then regress the endogenous variables on the instrumental variables, contemporaneous weather controls, city, date, and city by week fixed effects. Table 2 shows that F-tests on the coefficients of the instrumental variables all reject joint insignificance, which confirms that overall the selected instrumental variables are not weak. The coefficients of the first stage regressions are reported in Table 9 in the appendix.\nTable 2 First stage results\nJan 19–Feb 29 Jan 19–Feb 1 Feb 2–Feb 29\nOwn city\nAverage # new cases, 1-week lag F stat 11.41 4.02 17.28\np value 0.0000 0.0000 0.0000\nAverage # new cases, 2-week lag F stat 8.46 5.66 10.25\np value 0.0000 0.0000 0.0000\nAverage # new cases, previous 14 days F stat 18.37 7.72 21.69\np value 0.0000 0.0000 0.0000\nOther cities, inverse distance weighted\nAverage # new cases, 1-week lag F stat 19.10 36.29 17.58\np value 0.0000 0.0000 0.0000\nAverage # new cases, 2-week lag F stat 36.32 19.94 37.31\np value 0.0000 0.0000 0.0000\nAverage # new cases, previous 14 days F stat 47.08 33.45 46.22\np value 0.0000 0.0000 0.0000\nThis table reports the F-tests on the joint significance of the coefficients on the instrumental variables (IV) that are excluded from the estimation equations. Our IV include weekly averages of daily maximum temperature, precipitation, wind speed, and the interaction between precipitation and wind speed, during the preceding third and fourth weeks, and the averages of these variables in other cities weighted by the inverse of log distance. For each F statistic, the variable in the corresponding row is the dependent variable, and the time window in the corresponding column indicates the time span of the sample. Each regression also includes 1- and 2-week lags of these weather variables, weekly averages of new infections in the preceding first and second weeks in Wuhan which are interacted with the inverse log distance or the population flow, and city, date and city by week fixed effects. Coefficients on the instrumental variables for the full sample are reported in Table 15 in the appendix\nWe also need additional weather variables to instrument the adoption of public health measures at the city level. Since there is no theoretical guidance from the existing literature, we implement the Cluster-Lasso method of Belloni et al. (2016) and Ahrens et al. (2019) to select weather characteristics that have good predictive power. Details are displayed in Appendix A."}
LitCovid-PD-MONDO
{"project":"LitCovid-PD-MONDO","denotations":[{"id":"T68","span":{"begin":61,"end":69},"obj":"Disease"},{"id":"T69","span":{"begin":148,"end":156},"obj":"Disease"},{"id":"T70","span":{"begin":318,"end":328},"obj":"Disease"},{"id":"T71","span":{"begin":796,"end":804},"obj":"Disease"},{"id":"T72","span":{"begin":1142,"end":1152},"obj":"Disease"},{"id":"T73","span":{"begin":3401,"end":3414},"obj":"Disease"}],"attributes":[{"id":"A68","pred":"mondo_id","subj":"T68","obj":"http://purl.obolibrary.org/obo/MONDO_0100096"},{"id":"A69","pred":"mondo_id","subj":"T69","obj":"http://purl.obolibrary.org/obo/MONDO_0100096"},{"id":"A70","pred":"mondo_id","subj":"T70","obj":"http://purl.obolibrary.org/obo/MONDO_0005550"},{"id":"A71","pred":"mondo_id","subj":"T71","obj":"http://purl.obolibrary.org/obo/MONDO_0100096"},{"id":"A72","pred":"mondo_id","subj":"T72","obj":"http://purl.obolibrary.org/obo/MONDO_0005550"},{"id":"A73","pred":"mondo_id","subj":"T73","obj":"http://purl.obolibrary.org/obo/MONDO_0005550"}],"text":"Selection of instrumental variables\nThe transmission rate of COVID-19 may be affected by many environmental factors. Human-to-human transmission of COVID-19 is mostly through droplets and contacts (National Health Commission of the PRC 2020). Weather conditions such as rainfall, wind speed, and temperature may shape infections via their influences on social activities and virus transmissions. For instance, increased precipitation results in higher humidity, which may weaken virus transmissions (Lowen and Steel 2014). The virus may survive longer with lower temperature (Wang et al. 2020b; Puhani 2020). Greater wind speed and therefore ventilated air may decrease virus transmissions. In addition, increased rainfall and lower temperature may also reduce social activities. Newly confirmed COVID-19 cases typically arise from contracting the virus within 2 weeks in the past (e.g., World Health Organization 2020b). The extent of human-to-human transmission is determined by the number of people who have already contracted the virus and the environmental conditions within the next 2 weeks. Conditional on the number of people who are infectious and environmental conditions in the previous first and second weeks, it is plausible that weather conditions further in the past, i.e., in the previous third and fourth weeks, should not directly affect the number of current new cases. Based on the existing literature, we select weather characteristics as the instrumental variables, which include daily maximum temperature, precipitation, wind speed, and the interaction between precipitation and wind speed.\nWe then regress the endogenous variables on the instrumental variables, contemporaneous weather controls, city, date, and city by week fixed effects. Table 2 shows that F-tests on the coefficients of the instrumental variables all reject joint insignificance, which confirms that overall the selected instrumental variables are not weak. The coefficients of the first stage regressions are reported in Table 9 in the appendix.\nTable 2 First stage results\nJan 19–Feb 29 Jan 19–Feb 1 Feb 2–Feb 29\nOwn city\nAverage # new cases, 1-week lag F stat 11.41 4.02 17.28\np value 0.0000 0.0000 0.0000\nAverage # new cases, 2-week lag F stat 8.46 5.66 10.25\np value 0.0000 0.0000 0.0000\nAverage # new cases, previous 14 days F stat 18.37 7.72 21.69\np value 0.0000 0.0000 0.0000\nOther cities, inverse distance weighted\nAverage # new cases, 1-week lag F stat 19.10 36.29 17.58\np value 0.0000 0.0000 0.0000\nAverage # new cases, 2-week lag F stat 36.32 19.94 37.31\np value 0.0000 0.0000 0.0000\nAverage # new cases, previous 14 days F stat 47.08 33.45 46.22\np value 0.0000 0.0000 0.0000\nThis table reports the F-tests on the joint significance of the coefficients on the instrumental variables (IV) that are excluded from the estimation equations. Our IV include weekly averages of daily maximum temperature, precipitation, wind speed, and the interaction between precipitation and wind speed, during the preceding third and fourth weeks, and the averages of these variables in other cities weighted by the inverse of log distance. For each F statistic, the variable in the corresponding row is the dependent variable, and the time window in the corresponding column indicates the time span of the sample. Each regression also includes 1- and 2-week lags of these weather variables, weekly averages of new infections in the preceding first and second weeks in Wuhan which are interacted with the inverse log distance or the population flow, and city, date and city by week fixed effects. Coefficients on the instrumental variables for the full sample are reported in Table 15 in the appendix\nWe also need additional weather variables to instrument the adoption of public health measures at the city level. Since there is no theoretical guidance from the existing literature, we implement the Cluster-Lasso method of Belloni et al. (2016) and Ahrens et al. (2019) to select weather characteristics that have good predictive power. Details are displayed in Appendix A."}
LitCovid-PD-CLO
{"project":"LitCovid-PD-CLO","denotations":[{"id":"T97","span":{"begin":13,"end":25},"obj":"http://purl.obolibrary.org/obo/OBI_0000968"},{"id":"T98","span":{"begin":117,"end":122},"obj":"http://purl.obolibrary.org/obo/NCBITaxon_9606"},{"id":"T99","span":{"begin":126,"end":131},"obj":"http://purl.obolibrary.org/obo/NCBITaxon_9606"},{"id":"T100","span":{"begin":360,"end":370},"obj":"http://purl.obolibrary.org/obo/CLO_0001658"},{"id":"T101","span":{"begin":375,"end":380},"obj":"http://purl.obolibrary.org/obo/NCBITaxon_10239"},{"id":"T102","span":{"begin":479,"end":484},"obj":"http://purl.obolibrary.org/obo/NCBITaxon_10239"},{"id":"T103","span":{"begin":500,"end":505},"obj":"http://purl.obolibrary.org/obo/CLO_0007373"},{"id":"T104","span":{"begin":527,"end":532},"obj":"http://purl.obolibrary.org/obo/NCBITaxon_10239"},{"id":"T105","span":{"begin":670,"end":675},"obj":"http://purl.obolibrary.org/obo/NCBITaxon_10239"},{"id":"T106","span":{"begin":768,"end":778},"obj":"http://purl.obolibrary.org/obo/CLO_0001658"},{"id":"T107","span":{"begin":848,"end":853},"obj":"http://purl.obolibrary.org/obo/NCBITaxon_10239"},{"id":"T108","span":{"begin":901,"end":913},"obj":"http://purl.obolibrary.org/obo/OBI_0000245"},{"id":"T109","span":{"begin":936,"end":941},"obj":"http://purl.obolibrary.org/obo/NCBITaxon_9606"},{"id":"T110","span":{"begin":945,"end":950},"obj":"http://purl.obolibrary.org/obo/NCBITaxon_9606"},{"id":"T111","span":{"begin":1034,"end":1039},"obj":"http://purl.obolibrary.org/obo/NCBITaxon_10239"},{"id":"T112","span":{"begin":1464,"end":1476},"obj":"http://purl.obolibrary.org/obo/OBI_0000968"},{"id":"T113","span":{"begin":1662,"end":1674},"obj":"http://purl.obolibrary.org/obo/OBI_0000968"},{"id":"T114","span":{"begin":1785,"end":1790},"obj":"http://purl.obolibrary.org/obo/UBERON_0000473"},{"id":"T115","span":{"begin":1818,"end":1830},"obj":"http://purl.obolibrary.org/obo/OBI_0000968"},{"id":"T116","span":{"begin":1852,"end":1857},"obj":"http://purl.obolibrary.org/obo/UBERON_0000982"},{"id":"T117","span":{"begin":1852,"end":1857},"obj":"http://purl.obolibrary.org/obo/UBERON_0004905"},{"id":"T118","span":{"begin":1915,"end":1927},"obj":"http://purl.obolibrary.org/obo/OBI_0000968"},{"id":"T119","span":{"begin":2146,"end":2149},"obj":"http://purl.obolibrary.org/obo/CLO_0050236"},{"id":"T120","span":{"begin":2231,"end":2234},"obj":"http://purl.obolibrary.org/obo/CLO_0050236"},{"id":"T121","span":{"begin":2446,"end":2449},"obj":"http://purl.obolibrary.org/obo/CLO_0050236"},{"id":"T122","span":{"begin":2532,"end":2535},"obj":"http://purl.obolibrary.org/obo/CLO_0050236"},{"id":"T123","span":{"begin":2707,"end":2712},"obj":"http://purl.obolibrary.org/obo/UBERON_0000473"},{"id":"T124","span":{"begin":2720,"end":2725},"obj":"http://purl.obolibrary.org/obo/UBERON_0000982"},{"id":"T125","span":{"begin":2720,"end":2725},"obj":"http://purl.obolibrary.org/obo/UBERON_0004905"},{"id":"T126","span":{"begin":2766,"end":2778},"obj":"http://purl.obolibrary.org/obo/OBI_0000968"},{"id":"T127","span":{"begin":3603,"end":3615},"obj":"http://purl.obolibrary.org/obo/OBI_0000968"},{"id":"T128","span":{"begin":3732,"end":3742},"obj":"http://purl.obolibrary.org/obo/OBI_0000968"},{"id":"T129","span":{"begin":4059,"end":4060},"obj":"http://purl.obolibrary.org/obo/CLO_0001020"}],"text":"Selection of instrumental variables\nThe transmission rate of COVID-19 may be affected by many environmental factors. Human-to-human transmission of COVID-19 is mostly through droplets and contacts (National Health Commission of the PRC 2020). Weather conditions such as rainfall, wind speed, and temperature may shape infections via their influences on social activities and virus transmissions. For instance, increased precipitation results in higher humidity, which may weaken virus transmissions (Lowen and Steel 2014). The virus may survive longer with lower temperature (Wang et al. 2020b; Puhani 2020). Greater wind speed and therefore ventilated air may decrease virus transmissions. In addition, increased rainfall and lower temperature may also reduce social activities. Newly confirmed COVID-19 cases typically arise from contracting the virus within 2 weeks in the past (e.g., World Health Organization 2020b). The extent of human-to-human transmission is determined by the number of people who have already contracted the virus and the environmental conditions within the next 2 weeks. Conditional on the number of people who are infectious and environmental conditions in the previous first and second weeks, it is plausible that weather conditions further in the past, i.e., in the previous third and fourth weeks, should not directly affect the number of current new cases. Based on the existing literature, we select weather characteristics as the instrumental variables, which include daily maximum temperature, precipitation, wind speed, and the interaction between precipitation and wind speed.\nWe then regress the endogenous variables on the instrumental variables, contemporaneous weather controls, city, date, and city by week fixed effects. Table 2 shows that F-tests on the coefficients of the instrumental variables all reject joint insignificance, which confirms that overall the selected instrumental variables are not weak. The coefficients of the first stage regressions are reported in Table 9 in the appendix.\nTable 2 First stage results\nJan 19–Feb 29 Jan 19–Feb 1 Feb 2–Feb 29\nOwn city\nAverage # new cases, 1-week lag F stat 11.41 4.02 17.28\np value 0.0000 0.0000 0.0000\nAverage # new cases, 2-week lag F stat 8.46 5.66 10.25\np value 0.0000 0.0000 0.0000\nAverage # new cases, previous 14 days F stat 18.37 7.72 21.69\np value 0.0000 0.0000 0.0000\nOther cities, inverse distance weighted\nAverage # new cases, 1-week lag F stat 19.10 36.29 17.58\np value 0.0000 0.0000 0.0000\nAverage # new cases, 2-week lag F stat 36.32 19.94 37.31\np value 0.0000 0.0000 0.0000\nAverage # new cases, previous 14 days F stat 47.08 33.45 46.22\np value 0.0000 0.0000 0.0000\nThis table reports the F-tests on the joint significance of the coefficients on the instrumental variables (IV) that are excluded from the estimation equations. Our IV include weekly averages of daily maximum temperature, precipitation, wind speed, and the interaction between precipitation and wind speed, during the preceding third and fourth weeks, and the averages of these variables in other cities weighted by the inverse of log distance. For each F statistic, the variable in the corresponding row is the dependent variable, and the time window in the corresponding column indicates the time span of the sample. Each regression also includes 1- and 2-week lags of these weather variables, weekly averages of new infections in the preceding first and second weeks in Wuhan which are interacted with the inverse log distance or the population flow, and city, date and city by week fixed effects. Coefficients on the instrumental variables for the full sample are reported in Table 15 in the appendix\nWe also need additional weather variables to instrument the adoption of public health measures at the city level. Since there is no theoretical guidance from the existing literature, we implement the Cluster-Lasso method of Belloni et al. (2016) and Ahrens et al. (2019) to select weather characteristics that have good predictive power. Details are displayed in Appendix A."}
LitCovid-sentences
{"project":"LitCovid-sentences","denotations":[{"id":"T180","span":{"begin":0,"end":35},"obj":"Sentence"},{"id":"T181","span":{"begin":36,"end":116},"obj":"Sentence"},{"id":"T182","span":{"begin":117,"end":242},"obj":"Sentence"},{"id":"T183","span":{"begin":243,"end":395},"obj":"Sentence"},{"id":"T184","span":{"begin":396,"end":522},"obj":"Sentence"},{"id":"T185","span":{"begin":523,"end":587},"obj":"Sentence"},{"id":"T186","span":{"begin":588,"end":608},"obj":"Sentence"},{"id":"T187","span":{"begin":609,"end":690},"obj":"Sentence"},{"id":"T188","span":{"begin":691,"end":779},"obj":"Sentence"},{"id":"T189","span":{"begin":780,"end":921},"obj":"Sentence"},{"id":"T190","span":{"begin":922,"end":1097},"obj":"Sentence"},{"id":"T191","span":{"begin":1098,"end":1388},"obj":"Sentence"},{"id":"T192","span":{"begin":1389,"end":1613},"obj":"Sentence"},{"id":"T193","span":{"begin":1614,"end":1763},"obj":"Sentence"},{"id":"T194","span":{"begin":1764,"end":1951},"obj":"Sentence"},{"id":"T195","span":{"begin":1952,"end":2040},"obj":"Sentence"},{"id":"T196","span":{"begin":2041,"end":2068},"obj":"Sentence"},{"id":"T197","span":{"begin":2069,"end":2108},"obj":"Sentence"},{"id":"T198","span":{"begin":2109,"end":2117},"obj":"Sentence"},{"id":"T199","span":{"begin":2118,"end":2173},"obj":"Sentence"},{"id":"T200","span":{"begin":2174,"end":2202},"obj":"Sentence"},{"id":"T201","span":{"begin":2203,"end":2257},"obj":"Sentence"},{"id":"T202","span":{"begin":2258,"end":2286},"obj":"Sentence"},{"id":"T203","span":{"begin":2287,"end":2348},"obj":"Sentence"},{"id":"T204","span":{"begin":2349,"end":2377},"obj":"Sentence"},{"id":"T205","span":{"begin":2378,"end":2417},"obj":"Sentence"},{"id":"T206","span":{"begin":2418,"end":2474},"obj":"Sentence"},{"id":"T207","span":{"begin":2475,"end":2503},"obj":"Sentence"},{"id":"T208","span":{"begin":2504,"end":2560},"obj":"Sentence"},{"id":"T209","span":{"begin":2561,"end":2589},"obj":"Sentence"},{"id":"T210","span":{"begin":2590,"end":2652},"obj":"Sentence"},{"id":"T211","span":{"begin":2653,"end":2681},"obj":"Sentence"},{"id":"T212","span":{"begin":2682,"end":2842},"obj":"Sentence"},{"id":"T213","span":{"begin":2843,"end":3126},"obj":"Sentence"},{"id":"T214","span":{"begin":3127,"end":3300},"obj":"Sentence"},{"id":"T215","span":{"begin":3301,"end":3582},"obj":"Sentence"},{"id":"T216","span":{"begin":3583,"end":3686},"obj":"Sentence"},{"id":"T217","span":{"begin":3687,"end":3800},"obj":"Sentence"},{"id":"T218","span":{"begin":3801,"end":4024},"obj":"Sentence"},{"id":"T219","span":{"begin":4025,"end":4061},"obj":"Sentence"}],"namespaces":[{"prefix":"_base","uri":"http://pubannotation.org/ontology/tao.owl#"}],"text":"Selection of instrumental variables\nThe transmission rate of COVID-19 may be affected by many environmental factors. Human-to-human transmission of COVID-19 is mostly through droplets and contacts (National Health Commission of the PRC 2020). Weather conditions such as rainfall, wind speed, and temperature may shape infections via their influences on social activities and virus transmissions. For instance, increased precipitation results in higher humidity, which may weaken virus transmissions (Lowen and Steel 2014). The virus may survive longer with lower temperature (Wang et al. 2020b; Puhani 2020). Greater wind speed and therefore ventilated air may decrease virus transmissions. In addition, increased rainfall and lower temperature may also reduce social activities. Newly confirmed COVID-19 cases typically arise from contracting the virus within 2 weeks in the past (e.g., World Health Organization 2020b). The extent of human-to-human transmission is determined by the number of people who have already contracted the virus and the environmental conditions within the next 2 weeks. Conditional on the number of people who are infectious and environmental conditions in the previous first and second weeks, it is plausible that weather conditions further in the past, i.e., in the previous third and fourth weeks, should not directly affect the number of current new cases. Based on the existing literature, we select weather characteristics as the instrumental variables, which include daily maximum temperature, precipitation, wind speed, and the interaction between precipitation and wind speed.\nWe then regress the endogenous variables on the instrumental variables, contemporaneous weather controls, city, date, and city by week fixed effects. Table 2 shows that F-tests on the coefficients of the instrumental variables all reject joint insignificance, which confirms that overall the selected instrumental variables are not weak. The coefficients of the first stage regressions are reported in Table 9 in the appendix.\nTable 2 First stage results\nJan 19–Feb 29 Jan 19–Feb 1 Feb 2–Feb 29\nOwn city\nAverage # new cases, 1-week lag F stat 11.41 4.02 17.28\np value 0.0000 0.0000 0.0000\nAverage # new cases, 2-week lag F stat 8.46 5.66 10.25\np value 0.0000 0.0000 0.0000\nAverage # new cases, previous 14 days F stat 18.37 7.72 21.69\np value 0.0000 0.0000 0.0000\nOther cities, inverse distance weighted\nAverage # new cases, 1-week lag F stat 19.10 36.29 17.58\np value 0.0000 0.0000 0.0000\nAverage # new cases, 2-week lag F stat 36.32 19.94 37.31\np value 0.0000 0.0000 0.0000\nAverage # new cases, previous 14 days F stat 47.08 33.45 46.22\np value 0.0000 0.0000 0.0000\nThis table reports the F-tests on the joint significance of the coefficients on the instrumental variables (IV) that are excluded from the estimation equations. Our IV include weekly averages of daily maximum temperature, precipitation, wind speed, and the interaction between precipitation and wind speed, during the preceding third and fourth weeks, and the averages of these variables in other cities weighted by the inverse of log distance. For each F statistic, the variable in the corresponding row is the dependent variable, and the time window in the corresponding column indicates the time span of the sample. Each regression also includes 1- and 2-week lags of these weather variables, weekly averages of new infections in the preceding first and second weeks in Wuhan which are interacted with the inverse log distance or the population flow, and city, date and city by week fixed effects. Coefficients on the instrumental variables for the full sample are reported in Table 15 in the appendix\nWe also need additional weather variables to instrument the adoption of public health measures at the city level. Since there is no theoretical guidance from the existing literature, we implement the Cluster-Lasso method of Belloni et al. (2016) and Ahrens et al. (2019) to select weather characteristics that have good predictive power. Details are displayed in Appendix A."}
LitCovid-PubTator
{"project":"LitCovid-PubTator","denotations":[{"id":"186","span":{"begin":117,"end":122},"obj":"Species"},{"id":"187","span":{"begin":126,"end":131},"obj":"Species"},{"id":"188","span":{"begin":936,"end":941},"obj":"Species"},{"id":"189","span":{"begin":945,"end":950},"obj":"Species"},{"id":"190","span":{"begin":995,"end":1001},"obj":"Species"},{"id":"191","span":{"begin":1127,"end":1133},"obj":"Species"},{"id":"192","span":{"begin":61,"end":69},"obj":"Disease"},{"id":"193","span":{"begin":148,"end":156},"obj":"Disease"},{"id":"194","span":{"begin":318,"end":328},"obj":"Disease"},{"id":"195","span":{"begin":796,"end":804},"obj":"Disease"},{"id":"197","span":{"begin":2090,"end":2101},"obj":"Gene"},{"id":"199","span":{"begin":3401,"end":3411},"obj":"Disease"}],"attributes":[{"id":"A186","pred":"tao:has_database_id","subj":"186","obj":"Tax:9606"},{"id":"A187","pred":"tao:has_database_id","subj":"187","obj":"Tax:9606"},{"id":"A188","pred":"tao:has_database_id","subj":"188","obj":"Tax:9606"},{"id":"A189","pred":"tao:has_database_id","subj":"189","obj":"Tax:9606"},{"id":"A190","pred":"tao:has_database_id","subj":"190","obj":"Tax:9606"},{"id":"A191","pred":"tao:has_database_id","subj":"191","obj":"Tax:9606"},{"id":"A192","pred":"tao:has_database_id","subj":"192","obj":"MESH:C000657245"},{"id":"A193","pred":"tao:has_database_id","subj":"193","obj":"MESH:C000657245"},{"id":"A194","pred":"tao:has_database_id","subj":"194","obj":"MESH:D007239"},{"id":"A195","pred":"tao:has_database_id","subj":"195","obj":"MESH:C000657245"},{"id":"A197","pred":"tao:has_database_id","subj":"197","obj":"Gene:2233"},{"id":"A199","pred":"tao:has_database_id","subj":"199","obj":"MESH:D007239"}],"namespaces":[{"prefix":"Tax","uri":"https://www.ncbi.nlm.nih.gov/taxonomy/"},{"prefix":"MESH","uri":"https://id.nlm.nih.gov/mesh/"},{"prefix":"Gene","uri":"https://www.ncbi.nlm.nih.gov/gene/"},{"prefix":"CVCL","uri":"https://web.expasy.org/cellosaurus/CVCL_"}],"text":"Selection of instrumental variables\nThe transmission rate of COVID-19 may be affected by many environmental factors. Human-to-human transmission of COVID-19 is mostly through droplets and contacts (National Health Commission of the PRC 2020). Weather conditions such as rainfall, wind speed, and temperature may shape infections via their influences on social activities and virus transmissions. For instance, increased precipitation results in higher humidity, which may weaken virus transmissions (Lowen and Steel 2014). The virus may survive longer with lower temperature (Wang et al. 2020b; Puhani 2020). Greater wind speed and therefore ventilated air may decrease virus transmissions. In addition, increased rainfall and lower temperature may also reduce social activities. Newly confirmed COVID-19 cases typically arise from contracting the virus within 2 weeks in the past (e.g., World Health Organization 2020b). The extent of human-to-human transmission is determined by the number of people who have already contracted the virus and the environmental conditions within the next 2 weeks. Conditional on the number of people who are infectious and environmental conditions in the previous first and second weeks, it is plausible that weather conditions further in the past, i.e., in the previous third and fourth weeks, should not directly affect the number of current new cases. Based on the existing literature, we select weather characteristics as the instrumental variables, which include daily maximum temperature, precipitation, wind speed, and the interaction between precipitation and wind speed.\nWe then regress the endogenous variables on the instrumental variables, contemporaneous weather controls, city, date, and city by week fixed effects. Table 2 shows that F-tests on the coefficients of the instrumental variables all reject joint insignificance, which confirms that overall the selected instrumental variables are not weak. The coefficients of the first stage regressions are reported in Table 9 in the appendix.\nTable 2 First stage results\nJan 19–Feb 29 Jan 19–Feb 1 Feb 2–Feb 29\nOwn city\nAverage # new cases, 1-week lag F stat 11.41 4.02 17.28\np value 0.0000 0.0000 0.0000\nAverage # new cases, 2-week lag F stat 8.46 5.66 10.25\np value 0.0000 0.0000 0.0000\nAverage # new cases, previous 14 days F stat 18.37 7.72 21.69\np value 0.0000 0.0000 0.0000\nOther cities, inverse distance weighted\nAverage # new cases, 1-week lag F stat 19.10 36.29 17.58\np value 0.0000 0.0000 0.0000\nAverage # new cases, 2-week lag F stat 36.32 19.94 37.31\np value 0.0000 0.0000 0.0000\nAverage # new cases, previous 14 days F stat 47.08 33.45 46.22\np value 0.0000 0.0000 0.0000\nThis table reports the F-tests on the joint significance of the coefficients on the instrumental variables (IV) that are excluded from the estimation equations. Our IV include weekly averages of daily maximum temperature, precipitation, wind speed, and the interaction between precipitation and wind speed, during the preceding third and fourth weeks, and the averages of these variables in other cities weighted by the inverse of log distance. For each F statistic, the variable in the corresponding row is the dependent variable, and the time window in the corresponding column indicates the time span of the sample. Each regression also includes 1- and 2-week lags of these weather variables, weekly averages of new infections in the preceding first and second weeks in Wuhan which are interacted with the inverse log distance or the population flow, and city, date and city by week fixed effects. Coefficients on the instrumental variables for the full sample are reported in Table 15 in the appendix\nWe also need additional weather variables to instrument the adoption of public health measures at the city level. Since there is no theoretical guidance from the existing literature, we implement the Cluster-Lasso method of Belloni et al. (2016) and Ahrens et al. (2019) to select weather characteristics that have good predictive power. Details are displayed in Appendix A."}
2_test
{"project":"2_test","denotations":[{"id":"32395017-24789791-64435797","span":{"begin":516,"end":520},"obj":"24789791"}],"text":"Selection of instrumental variables\nThe transmission rate of COVID-19 may be affected by many environmental factors. Human-to-human transmission of COVID-19 is mostly through droplets and contacts (National Health Commission of the PRC 2020). Weather conditions such as rainfall, wind speed, and temperature may shape infections via their influences on social activities and virus transmissions. For instance, increased precipitation results in higher humidity, which may weaken virus transmissions (Lowen and Steel 2014). The virus may survive longer with lower temperature (Wang et al. 2020b; Puhani 2020). Greater wind speed and therefore ventilated air may decrease virus transmissions. In addition, increased rainfall and lower temperature may also reduce social activities. Newly confirmed COVID-19 cases typically arise from contracting the virus within 2 weeks in the past (e.g., World Health Organization 2020b). The extent of human-to-human transmission is determined by the number of people who have already contracted the virus and the environmental conditions within the next 2 weeks. Conditional on the number of people who are infectious and environmental conditions in the previous first and second weeks, it is plausible that weather conditions further in the past, i.e., in the previous third and fourth weeks, should not directly affect the number of current new cases. Based on the existing literature, we select weather characteristics as the instrumental variables, which include daily maximum temperature, precipitation, wind speed, and the interaction between precipitation and wind speed.\nWe then regress the endogenous variables on the instrumental variables, contemporaneous weather controls, city, date, and city by week fixed effects. Table 2 shows that F-tests on the coefficients of the instrumental variables all reject joint insignificance, which confirms that overall the selected instrumental variables are not weak. The coefficients of the first stage regressions are reported in Table 9 in the appendix.\nTable 2 First stage results\nJan 19–Feb 29 Jan 19–Feb 1 Feb 2–Feb 29\nOwn city\nAverage # new cases, 1-week lag F stat 11.41 4.02 17.28\np value 0.0000 0.0000 0.0000\nAverage # new cases, 2-week lag F stat 8.46 5.66 10.25\np value 0.0000 0.0000 0.0000\nAverage # new cases, previous 14 days F stat 18.37 7.72 21.69\np value 0.0000 0.0000 0.0000\nOther cities, inverse distance weighted\nAverage # new cases, 1-week lag F stat 19.10 36.29 17.58\np value 0.0000 0.0000 0.0000\nAverage # new cases, 2-week lag F stat 36.32 19.94 37.31\np value 0.0000 0.0000 0.0000\nAverage # new cases, previous 14 days F stat 47.08 33.45 46.22\np value 0.0000 0.0000 0.0000\nThis table reports the F-tests on the joint significance of the coefficients on the instrumental variables (IV) that are excluded from the estimation equations. Our IV include weekly averages of daily maximum temperature, precipitation, wind speed, and the interaction between precipitation and wind speed, during the preceding third and fourth weeks, and the averages of these variables in other cities weighted by the inverse of log distance. For each F statistic, the variable in the corresponding row is the dependent variable, and the time window in the corresponding column indicates the time span of the sample. Each regression also includes 1- and 2-week lags of these weather variables, weekly averages of new infections in the preceding first and second weeks in Wuhan which are interacted with the inverse log distance or the population flow, and city, date and city by week fixed effects. Coefficients on the instrumental variables for the full sample are reported in Table 15 in the appendix\nWe also need additional weather variables to instrument the adoption of public health measures at the city level. Since there is no theoretical guidance from the existing literature, we implement the Cluster-Lasso method of Belloni et al. (2016) and Ahrens et al. (2019) to select weather characteristics that have good predictive power. Details are displayed in Appendix A."}