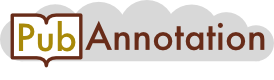
PMC:7160614 / 27991-29091
Annnotations
LitCovid-PubTator
{"project":"LitCovid-PubTator","denotations":[{"id":"361","span":{"begin":442,"end":444},"obj":"Chemical"},{"id":"362","span":{"begin":122,"end":130},"obj":"Disease"},{"id":"363","span":{"begin":377,"end":382},"obj":"Disease"},{"id":"364","span":{"begin":387,"end":394},"obj":"Disease"},{"id":"365","span":{"begin":571,"end":584},"obj":"Disease"}],"attributes":[{"id":"A362","pred":"tao:has_database_id","subj":"362","obj":"MESH:C000657245"},{"id":"A363","pred":"tao:has_database_id","subj":"363","obj":"MESH:D003371"},{"id":"A364","pred":"tao:has_database_id","subj":"364","obj":"MESH:D005221"},{"id":"A365","pred":"tao:has_database_id","subj":"365","obj":"MESH:D001943"}],"namespaces":[{"prefix":"Tax","uri":"https://www.ncbi.nlm.nih.gov/taxonomy/"},{"prefix":"MESH","uri":"https://id.nlm.nih.gov/mesh/"},{"prefix":"Gene","uri":"https://www.ncbi.nlm.nih.gov/gene/"},{"prefix":"CVCL","uri":"https://web.expasy.org/cellosaurus/CVCL_"}],"text":"To our knowledge, no diagnostic model based on imaging and clinical features alone has been proposed for the diagnosis of COVID-19. Our clinical and radiological semantic (CR) models consisted of the following features: total number of GGO with consolidation in the peripheral area, tree-in-bud, offending vessel augmentation in lesions, temperature, heart ratio, respiration, cough and fatigue, WBC count, and lymphocyte count category. The CR model outperformed the individual clinical and radiologic model. This result was in accordance with that in previous study in breast cancer, in which the model based on the combination of radiomics features and clinical features achieved a higher performance [24]. Compared with the radiomics-based model, the extraction of radiological semantic features can overcome the image discrepancy caused by different scanning parameters and/or different CT vendors. A previous study [28] also indicated that models based on semantic features determined by an experienced thoracic radiologist slightly outperformed models based on computed texture features alone."}
LitCovid-PD-FMA-UBERON
{"project":"LitCovid-PD-FMA-UBERON","denotations":[{"id":"T66","span":{"begin":351,"end":356},"obj":"Body_part"},{"id":"T67","span":{"begin":411,"end":421},"obj":"Body_part"},{"id":"T68","span":{"begin":571,"end":577},"obj":"Body_part"}],"attributes":[{"id":"A66","pred":"fma_id","subj":"T66","obj":"http://purl.org/sig/ont/fma/fma7088"},{"id":"A67","pred":"fma_id","subj":"T67","obj":"http://purl.org/sig/ont/fma/fma62863"},{"id":"A68","pred":"fma_id","subj":"T68","obj":"http://purl.org/sig/ont/fma/fma9601"}],"text":"To our knowledge, no diagnostic model based on imaging and clinical features alone has been proposed for the diagnosis of COVID-19. Our clinical and radiological semantic (CR) models consisted of the following features: total number of GGO with consolidation in the peripheral area, tree-in-bud, offending vessel augmentation in lesions, temperature, heart ratio, respiration, cough and fatigue, WBC count, and lymphocyte count category. The CR model outperformed the individual clinical and radiologic model. This result was in accordance with that in previous study in breast cancer, in which the model based on the combination of radiomics features and clinical features achieved a higher performance [24]. Compared with the radiomics-based model, the extraction of radiological semantic features can overcome the image discrepancy caused by different scanning parameters and/or different CT vendors. A previous study [28] also indicated that models based on semantic features determined by an experienced thoracic radiologist slightly outperformed models based on computed texture features alone."}
LitCovid-PD-UBERON
{"project":"LitCovid-PD-UBERON","denotations":[{"id":"T52","span":{"begin":306,"end":312},"obj":"Body_part"},{"id":"T53","span":{"begin":351,"end":356},"obj":"Body_part"},{"id":"T54","span":{"begin":571,"end":577},"obj":"Body_part"}],"attributes":[{"id":"A52","pred":"uberon_id","subj":"T52","obj":"http://purl.obolibrary.org/obo/UBERON_0000055"},{"id":"A53","pred":"uberon_id","subj":"T53","obj":"http://purl.obolibrary.org/obo/UBERON_0000948"},{"id":"A54","pred":"uberon_id","subj":"T54","obj":"http://purl.obolibrary.org/obo/UBERON_0000310"}],"text":"To our knowledge, no diagnostic model based on imaging and clinical features alone has been proposed for the diagnosis of COVID-19. Our clinical and radiological semantic (CR) models consisted of the following features: total number of GGO with consolidation in the peripheral area, tree-in-bud, offending vessel augmentation in lesions, temperature, heart ratio, respiration, cough and fatigue, WBC count, and lymphocyte count category. The CR model outperformed the individual clinical and radiologic model. This result was in accordance with that in previous study in breast cancer, in which the model based on the combination of radiomics features and clinical features achieved a higher performance [24]. Compared with the radiomics-based model, the extraction of radiological semantic features can overcome the image discrepancy caused by different scanning parameters and/or different CT vendors. A previous study [28] also indicated that models based on semantic features determined by an experienced thoracic radiologist slightly outperformed models based on computed texture features alone."}
LitCovid-PD-MONDO
{"project":"LitCovid-PD-MONDO","denotations":[{"id":"T114","span":{"begin":122,"end":130},"obj":"Disease"},{"id":"T115","span":{"begin":571,"end":584},"obj":"Disease"},{"id":"T116","span":{"begin":578,"end":584},"obj":"Disease"}],"attributes":[{"id":"A114","pred":"mondo_id","subj":"T114","obj":"http://purl.obolibrary.org/obo/MONDO_0100096"},{"id":"A115","pred":"mondo_id","subj":"T115","obj":"http://purl.obolibrary.org/obo/MONDO_0007254"},{"id":"A116","pred":"mondo_id","subj":"T116","obj":"http://purl.obolibrary.org/obo/MONDO_0004992"}],"text":"To our knowledge, no diagnostic model based on imaging and clinical features alone has been proposed for the diagnosis of COVID-19. Our clinical and radiological semantic (CR) models consisted of the following features: total number of GGO with consolidation in the peripheral area, tree-in-bud, offending vessel augmentation in lesions, temperature, heart ratio, respiration, cough and fatigue, WBC count, and lymphocyte count category. The CR model outperformed the individual clinical and radiologic model. This result was in accordance with that in previous study in breast cancer, in which the model based on the combination of radiomics features and clinical features achieved a higher performance [24]. Compared with the radiomics-based model, the extraction of radiological semantic features can overcome the image discrepancy caused by different scanning parameters and/or different CT vendors. A previous study [28] also indicated that models based on semantic features determined by an experienced thoracic radiologist slightly outperformed models based on computed texture features alone."}
LitCovid-PD-CLO
{"project":"LitCovid-PD-CLO","denotations":[{"id":"T175","span":{"begin":83,"end":86},"obj":"http://purl.obolibrary.org/obo/CLO_0051582"},{"id":"T176","span":{"begin":306,"end":312},"obj":"http://purl.obolibrary.org/obo/UBERON_0000055"},{"id":"T177","span":{"begin":351,"end":356},"obj":"http://purl.obolibrary.org/obo/UBERON_0000948"},{"id":"T178","span":{"begin":351,"end":356},"obj":"http://purl.obolibrary.org/obo/UBERON_0007100"},{"id":"T179","span":{"begin":351,"end":356},"obj":"http://purl.obolibrary.org/obo/UBERON_0015228"},{"id":"T180","span":{"begin":351,"end":356},"obj":"http://www.ebi.ac.uk/efo/EFO_0000815"},{"id":"T181","span":{"begin":571,"end":577},"obj":"http://purl.obolibrary.org/obo/UBERON_0000310"},{"id":"T182","span":{"begin":683,"end":684},"obj":"http://purl.obolibrary.org/obo/CLO_0001020"},{"id":"T183","span":{"begin":904,"end":905},"obj":"http://purl.obolibrary.org/obo/CLO_0001020"}],"text":"To our knowledge, no diagnostic model based on imaging and clinical features alone has been proposed for the diagnosis of COVID-19. Our clinical and radiological semantic (CR) models consisted of the following features: total number of GGO with consolidation in the peripheral area, tree-in-bud, offending vessel augmentation in lesions, temperature, heart ratio, respiration, cough and fatigue, WBC count, and lymphocyte count category. The CR model outperformed the individual clinical and radiologic model. This result was in accordance with that in previous study in breast cancer, in which the model based on the combination of radiomics features and clinical features achieved a higher performance [24]. Compared with the radiomics-based model, the extraction of radiological semantic features can overcome the image discrepancy caused by different scanning parameters and/or different CT vendors. A previous study [28] also indicated that models based on semantic features determined by an experienced thoracic radiologist slightly outperformed models based on computed texture features alone."}
LitCovid-PD-GO-BP
{"project":"LitCovid-PD-GO-BP","denotations":[{"id":"T14","span":{"begin":364,"end":375},"obj":"http://purl.obolibrary.org/obo/GO_0045333"},{"id":"T15","span":{"begin":364,"end":375},"obj":"http://purl.obolibrary.org/obo/GO_0007585"}],"text":"To our knowledge, no diagnostic model based on imaging and clinical features alone has been proposed for the diagnosis of COVID-19. Our clinical and radiological semantic (CR) models consisted of the following features: total number of GGO with consolidation in the peripheral area, tree-in-bud, offending vessel augmentation in lesions, temperature, heart ratio, respiration, cough and fatigue, WBC count, and lymphocyte count category. The CR model outperformed the individual clinical and radiologic model. This result was in accordance with that in previous study in breast cancer, in which the model based on the combination of radiomics features and clinical features achieved a higher performance [24]. Compared with the radiomics-based model, the extraction of radiological semantic features can overcome the image discrepancy caused by different scanning parameters and/or different CT vendors. A previous study [28] also indicated that models based on semantic features determined by an experienced thoracic radiologist slightly outperformed models based on computed texture features alone."}
LitCovid-PD-HP
{"project":"LitCovid-PD-HP","denotations":[{"id":"T40","span":{"begin":377,"end":382},"obj":"Phenotype"},{"id":"T41","span":{"begin":387,"end":394},"obj":"Phenotype"},{"id":"T42","span":{"begin":571,"end":584},"obj":"Phenotype"}],"attributes":[{"id":"A40","pred":"hp_id","subj":"T40","obj":"http://purl.obolibrary.org/obo/HP_0012735"},{"id":"A41","pred":"hp_id","subj":"T41","obj":"http://purl.obolibrary.org/obo/HP_0012378"},{"id":"A42","pred":"hp_id","subj":"T42","obj":"http://purl.obolibrary.org/obo/HP_0003002"}],"text":"To our knowledge, no diagnostic model based on imaging and clinical features alone has been proposed for the diagnosis of COVID-19. Our clinical and radiological semantic (CR) models consisted of the following features: total number of GGO with consolidation in the peripheral area, tree-in-bud, offending vessel augmentation in lesions, temperature, heart ratio, respiration, cough and fatigue, WBC count, and lymphocyte count category. The CR model outperformed the individual clinical and radiologic model. This result was in accordance with that in previous study in breast cancer, in which the model based on the combination of radiomics features and clinical features achieved a higher performance [24]. Compared with the radiomics-based model, the extraction of radiological semantic features can overcome the image discrepancy caused by different scanning parameters and/or different CT vendors. A previous study [28] also indicated that models based on semantic features determined by an experienced thoracic radiologist slightly outperformed models based on computed texture features alone."}
0_colil
{"project":"0_colil","denotations":[{"id":"32300971-30842125-67439","span":{"begin":705,"end":707},"obj":"30842125"},{"id":"32300971-31115618-67440","span":{"begin":922,"end":924},"obj":"31115618"}],"text":"To our knowledge, no diagnostic model based on imaging and clinical features alone has been proposed for the diagnosis of COVID-19. Our clinical and radiological semantic (CR) models consisted of the following features: total number of GGO with consolidation in the peripheral area, tree-in-bud, offending vessel augmentation in lesions, temperature, heart ratio, respiration, cough and fatigue, WBC count, and lymphocyte count category. The CR model outperformed the individual clinical and radiologic model. This result was in accordance with that in previous study in breast cancer, in which the model based on the combination of radiomics features and clinical features achieved a higher performance [24]. Compared with the radiomics-based model, the extraction of radiological semantic features can overcome the image discrepancy caused by different scanning parameters and/or different CT vendors. A previous study [28] also indicated that models based on semantic features determined by an experienced thoracic radiologist slightly outperformed models based on computed texture features alone."}
TEST0
{"project":"TEST0","denotations":[{"id":"32300971-195-201-67439","span":{"begin":705,"end":707},"obj":"[\"30842125\"]"},{"id":"32300971-18-24-67440","span":{"begin":922,"end":924},"obj":"[\"31115618\"]"}],"text":"To our knowledge, no diagnostic model based on imaging and clinical features alone has been proposed for the diagnosis of COVID-19. Our clinical and radiological semantic (CR) models consisted of the following features: total number of GGO with consolidation in the peripheral area, tree-in-bud, offending vessel augmentation in lesions, temperature, heart ratio, respiration, cough and fatigue, WBC count, and lymphocyte count category. The CR model outperformed the individual clinical and radiologic model. This result was in accordance with that in previous study in breast cancer, in which the model based on the combination of radiomics features and clinical features achieved a higher performance [24]. Compared with the radiomics-based model, the extraction of radiological semantic features can overcome the image discrepancy caused by different scanning parameters and/or different CT vendors. A previous study [28] also indicated that models based on semantic features determined by an experienced thoracic radiologist slightly outperformed models based on computed texture features alone."}
2_test
{"project":"2_test","denotations":[{"id":"32300971-30842125-29373587","span":{"begin":705,"end":707},"obj":"30842125"},{"id":"32300971-31115618-29373588","span":{"begin":922,"end":924},"obj":"31115618"}],"text":"To our knowledge, no diagnostic model based on imaging and clinical features alone has been proposed for the diagnosis of COVID-19. Our clinical and radiological semantic (CR) models consisted of the following features: total number of GGO with consolidation in the peripheral area, tree-in-bud, offending vessel augmentation in lesions, temperature, heart ratio, respiration, cough and fatigue, WBC count, and lymphocyte count category. The CR model outperformed the individual clinical and radiologic model. This result was in accordance with that in previous study in breast cancer, in which the model based on the combination of radiomics features and clinical features achieved a higher performance [24]. Compared with the radiomics-based model, the extraction of radiological semantic features can overcome the image discrepancy caused by different scanning parameters and/or different CT vendors. A previous study [28] also indicated that models based on semantic features determined by an experienced thoracic radiologist slightly outperformed models based on computed texture features alone."}
LitCovid-sentences
{"project":"LitCovid-sentences","denotations":[{"id":"T303","span":{"begin":0,"end":131},"obj":"Sentence"},{"id":"T304","span":{"begin":132,"end":437},"obj":"Sentence"},{"id":"T305","span":{"begin":438,"end":509},"obj":"Sentence"},{"id":"T306","span":{"begin":510,"end":709},"obj":"Sentence"},{"id":"T307","span":{"begin":710,"end":903},"obj":"Sentence"},{"id":"T308","span":{"begin":904,"end":1100},"obj":"Sentence"}],"namespaces":[{"prefix":"_base","uri":"http://pubannotation.org/ontology/tao.owl#"}],"text":"To our knowledge, no diagnostic model based on imaging and clinical features alone has been proposed for the diagnosis of COVID-19. Our clinical and radiological semantic (CR) models consisted of the following features: total number of GGO with consolidation in the peripheral area, tree-in-bud, offending vessel augmentation in lesions, temperature, heart ratio, respiration, cough and fatigue, WBC count, and lymphocyte count category. The CR model outperformed the individual clinical and radiologic model. This result was in accordance with that in previous study in breast cancer, in which the model based on the combination of radiomics features and clinical features achieved a higher performance [24]. Compared with the radiomics-based model, the extraction of radiological semantic features can overcome the image discrepancy caused by different scanning parameters and/or different CT vendors. A previous study [28] also indicated that models based on semantic features determined by an experienced thoracic radiologist slightly outperformed models based on computed texture features alone."}
MyTest
{"project":"MyTest","denotations":[{"id":"32300971-30842125-29373587","span":{"begin":705,"end":707},"obj":"30842125"},{"id":"32300971-31115618-29373588","span":{"begin":922,"end":924},"obj":"31115618"}],"namespaces":[{"prefix":"_base","uri":"https://www.uniprot.org/uniprot/testbase"},{"prefix":"UniProtKB","uri":"https://www.uniprot.org/uniprot/"},{"prefix":"uniprot","uri":"https://www.uniprot.org/uniprotkb/"}],"text":"To our knowledge, no diagnostic model based on imaging and clinical features alone has been proposed for the diagnosis of COVID-19. Our clinical and radiological semantic (CR) models consisted of the following features: total number of GGO with consolidation in the peripheral area, tree-in-bud, offending vessel augmentation in lesions, temperature, heart ratio, respiration, cough and fatigue, WBC count, and lymphocyte count category. The CR model outperformed the individual clinical and radiologic model. This result was in accordance with that in previous study in breast cancer, in which the model based on the combination of radiomics features and clinical features achieved a higher performance [24]. Compared with the radiomics-based model, the extraction of radiological semantic features can overcome the image discrepancy caused by different scanning parameters and/or different CT vendors. A previous study [28] also indicated that models based on semantic features determined by an experienced thoracic radiologist slightly outperformed models based on computed texture features alone."}