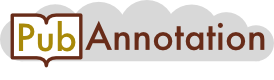
PMC:7160614 / 10074-11197
Annnotations
LitCovid-PubTator
{"project":"LitCovid-PubTator","denotations":[{"id":"175","span":{"begin":368,"end":376},"obj":"Disease"},{"id":"176","span":{"begin":385,"end":393},"obj":"Disease"},{"id":"177","span":{"begin":567,"end":575},"obj":"Disease"}],"attributes":[{"id":"A175","pred":"tao:has_database_id","subj":"175","obj":"MESH:C000657245"},{"id":"A176","pred":"tao:has_database_id","subj":"176","obj":"MESH:C000657245"},{"id":"A177","pred":"tao:has_database_id","subj":"177","obj":"MESH:C000657245"}],"namespaces":[{"prefix":"Tax","uri":"https://www.ncbi.nlm.nih.gov/taxonomy/"},{"prefix":"MESH","uri":"https://id.nlm.nih.gov/mesh/"},{"prefix":"Gene","uri":"https://www.ncbi.nlm.nih.gov/gene/"},{"prefix":"CVCL","uri":"https://web.expasy.org/cellosaurus/CVCL_"}],"text":"To obtain the most valuable clinical and radiological semantic features, statistical analysis, univariate analysis, and the least absolute shrinkage and selection operator (LASSO) method were performed. In statistical analysis, the chi-square test, the Kruskal-Wallis H test, and t test were utilized to compare the radiological semantic and clinical features between COVID-19 and non-COVID-19 groups. The features with p value smaller than 0.05 were selected. Then, univariate analysis was performed for clinical and radiological candidate features to determine the COVID-19 risk factors. The features with p value smaller than 0.05 in univariate analysis were also selected. The least absolute shrinkage and selection operator (LASSO) method [23] was utilized to select the most useful features with penalty parameter tuning that was conducted by 10-fold cross-validation based on minimum criteria. Diagnostic models were then constructed by multivariate logistic regression with the selected features. The flowchart of the feature selection process for these models was presented in the Supplementary Material (Fig. E2)."}
LitCovid-PD-MONDO
{"project":"LitCovid-PD-MONDO","denotations":[{"id":"T53","span":{"begin":368,"end":376},"obj":"Disease"},{"id":"T54","span":{"begin":385,"end":393},"obj":"Disease"},{"id":"T55","span":{"begin":567,"end":575},"obj":"Disease"}],"attributes":[{"id":"A53","pred":"mondo_id","subj":"T53","obj":"http://purl.obolibrary.org/obo/MONDO_0100096"},{"id":"A54","pred":"mondo_id","subj":"T54","obj":"http://purl.obolibrary.org/obo/MONDO_0100096"},{"id":"A55","pred":"mondo_id","subj":"T55","obj":"http://purl.obolibrary.org/obo/MONDO_0100096"}],"text":"To obtain the most valuable clinical and radiological semantic features, statistical analysis, univariate analysis, and the least absolute shrinkage and selection operator (LASSO) method were performed. In statistical analysis, the chi-square test, the Kruskal-Wallis H test, and t test were utilized to compare the radiological semantic and clinical features between COVID-19 and non-COVID-19 groups. The features with p value smaller than 0.05 were selected. Then, univariate analysis was performed for clinical and radiological candidate features to determine the COVID-19 risk factors. The features with p value smaller than 0.05 in univariate analysis were also selected. The least absolute shrinkage and selection operator (LASSO) method [23] was utilized to select the most useful features with penalty parameter tuning that was conducted by 10-fold cross-validation based on minimum criteria. Diagnostic models were then constructed by multivariate logistic regression with the selected features. The flowchart of the feature selection process for these models was presented in the Supplementary Material (Fig. E2)."}
LitCovid-PD-CLO
{"project":"LitCovid-PD-CLO","denotations":[{"id":"T68","span":{"begin":243,"end":247},"obj":"http://purl.obolibrary.org/obo/UBERON_0000473"},{"id":"T69","span":{"begin":270,"end":274},"obj":"http://purl.obolibrary.org/obo/UBERON_0000473"},{"id":"T70","span":{"begin":282,"end":286},"obj":"http://purl.obolibrary.org/obo/UBERON_0000473"},{"id":"T71","span":{"begin":1119,"end":1121},"obj":"http://purl.obolibrary.org/obo/CLO_0002860"}],"text":"To obtain the most valuable clinical and radiological semantic features, statistical analysis, univariate analysis, and the least absolute shrinkage and selection operator (LASSO) method were performed. In statistical analysis, the chi-square test, the Kruskal-Wallis H test, and t test were utilized to compare the radiological semantic and clinical features between COVID-19 and non-COVID-19 groups. The features with p value smaller than 0.05 were selected. Then, univariate analysis was performed for clinical and radiological candidate features to determine the COVID-19 risk factors. The features with p value smaller than 0.05 in univariate analysis were also selected. The least absolute shrinkage and selection operator (LASSO) method [23] was utilized to select the most useful features with penalty parameter tuning that was conducted by 10-fold cross-validation based on minimum criteria. Diagnostic models were then constructed by multivariate logistic regression with the selected features. The flowchart of the feature selection process for these models was presented in the Supplementary Material (Fig. E2)."}
0_colil
{"project":"0_colil","denotations":[{"id":"32300971-26037317-67437","span":{"begin":745,"end":747},"obj":"26037317"}],"text":"To obtain the most valuable clinical and radiological semantic features, statistical analysis, univariate analysis, and the least absolute shrinkage and selection operator (LASSO) method were performed. In statistical analysis, the chi-square test, the Kruskal-Wallis H test, and t test were utilized to compare the radiological semantic and clinical features between COVID-19 and non-COVID-19 groups. The features with p value smaller than 0.05 were selected. Then, univariate analysis was performed for clinical and radiological candidate features to determine the COVID-19 risk factors. The features with p value smaller than 0.05 in univariate analysis were also selected. The least absolute shrinkage and selection operator (LASSO) method [23] was utilized to select the most useful features with penalty parameter tuning that was conducted by 10-fold cross-validation based on minimum criteria. Diagnostic models were then constructed by multivariate logistic regression with the selected features. The flowchart of the feature selection process for these models was presented in the Supplementary Material (Fig. E2)."}
TEST0
{"project":"TEST0","denotations":[{"id":"32300971-68-74-67437","span":{"begin":745,"end":747},"obj":"[\"26037317\"]"}],"text":"To obtain the most valuable clinical and radiological semantic features, statistical analysis, univariate analysis, and the least absolute shrinkage and selection operator (LASSO) method were performed. In statistical analysis, the chi-square test, the Kruskal-Wallis H test, and t test were utilized to compare the radiological semantic and clinical features between COVID-19 and non-COVID-19 groups. The features with p value smaller than 0.05 were selected. Then, univariate analysis was performed for clinical and radiological candidate features to determine the COVID-19 risk factors. The features with p value smaller than 0.05 in univariate analysis were also selected. The least absolute shrinkage and selection operator (LASSO) method [23] was utilized to select the most useful features with penalty parameter tuning that was conducted by 10-fold cross-validation based on minimum criteria. Diagnostic models were then constructed by multivariate logistic regression with the selected features. The flowchart of the feature selection process for these models was presented in the Supplementary Material (Fig. E2)."}
2_test
{"project":"2_test","denotations":[{"id":"32300971-26037317-29373585","span":{"begin":745,"end":747},"obj":"26037317"}],"text":"To obtain the most valuable clinical and radiological semantic features, statistical analysis, univariate analysis, and the least absolute shrinkage and selection operator (LASSO) method were performed. In statistical analysis, the chi-square test, the Kruskal-Wallis H test, and t test were utilized to compare the radiological semantic and clinical features between COVID-19 and non-COVID-19 groups. The features with p value smaller than 0.05 were selected. Then, univariate analysis was performed for clinical and radiological candidate features to determine the COVID-19 risk factors. The features with p value smaller than 0.05 in univariate analysis were also selected. The least absolute shrinkage and selection operator (LASSO) method [23] was utilized to select the most useful features with penalty parameter tuning that was conducted by 10-fold cross-validation based on minimum criteria. Diagnostic models were then constructed by multivariate logistic regression with the selected features. The flowchart of the feature selection process for these models was presented in the Supplementary Material (Fig. E2)."}
LitCovid-sentences
{"project":"LitCovid-sentences","denotations":[{"id":"T83","span":{"begin":0,"end":202},"obj":"Sentence"},{"id":"T84","span":{"begin":203,"end":401},"obj":"Sentence"},{"id":"T85","span":{"begin":402,"end":460},"obj":"Sentence"},{"id":"T86","span":{"begin":461,"end":589},"obj":"Sentence"},{"id":"T87","span":{"begin":590,"end":676},"obj":"Sentence"},{"id":"T88","span":{"begin":677,"end":900},"obj":"Sentence"},{"id":"T89","span":{"begin":901,"end":1004},"obj":"Sentence"},{"id":"T90","span":{"begin":1005,"end":1123},"obj":"Sentence"}],"namespaces":[{"prefix":"_base","uri":"http://pubannotation.org/ontology/tao.owl#"}],"text":"To obtain the most valuable clinical and radiological semantic features, statistical analysis, univariate analysis, and the least absolute shrinkage and selection operator (LASSO) method were performed. In statistical analysis, the chi-square test, the Kruskal-Wallis H test, and t test were utilized to compare the radiological semantic and clinical features between COVID-19 and non-COVID-19 groups. The features with p value smaller than 0.05 were selected. Then, univariate analysis was performed for clinical and radiological candidate features to determine the COVID-19 risk factors. The features with p value smaller than 0.05 in univariate analysis were also selected. The least absolute shrinkage and selection operator (LASSO) method [23] was utilized to select the most useful features with penalty parameter tuning that was conducted by 10-fold cross-validation based on minimum criteria. Diagnostic models were then constructed by multivariate logistic regression with the selected features. The flowchart of the feature selection process for these models was presented in the Supplementary Material (Fig. E2)."}
MyTest
{"project":"MyTest","denotations":[{"id":"32300971-26037317-29373585","span":{"begin":745,"end":747},"obj":"26037317"}],"namespaces":[{"prefix":"_base","uri":"https://www.uniprot.org/uniprot/testbase"},{"prefix":"UniProtKB","uri":"https://www.uniprot.org/uniprot/"},{"prefix":"uniprot","uri":"https://www.uniprot.org/uniprotkb/"}],"text":"To obtain the most valuable clinical and radiological semantic features, statistical analysis, univariate analysis, and the least absolute shrinkage and selection operator (LASSO) method were performed. In statistical analysis, the chi-square test, the Kruskal-Wallis H test, and t test were utilized to compare the radiological semantic and clinical features between COVID-19 and non-COVID-19 groups. The features with p value smaller than 0.05 were selected. Then, univariate analysis was performed for clinical and radiological candidate features to determine the COVID-19 risk factors. The features with p value smaller than 0.05 in univariate analysis were also selected. The least absolute shrinkage and selection operator (LASSO) method [23] was utilized to select the most useful features with penalty parameter tuning that was conducted by 10-fold cross-validation based on minimum criteria. Diagnostic models were then constructed by multivariate logistic regression with the selected features. The flowchart of the feature selection process for these models was presented in the Supplementary Material (Fig. E2)."}