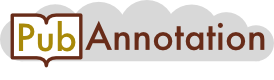
PMC:7143846 / 6803-9107
Annnotations
LitCovid-PubTator
Id | Subject | Object | Predicate | Lexical cue | tao:has_database_id |
---|---|---|---|---|---|
91 | 197-204 | Disease | denotes | anxiety | MESH:D001007 |
95 | 1252-1278 | Disease | denotes | deep learning technologies | MESH:D007859 |
96 | 1312-1319 | Disease | denotes | anxiety | MESH:D001007 |
97 | 1321-1331 | Disease | denotes | depression | MESH:D000275 |
101 | 1790-1797 | Disease | denotes | anxiety | MESH:D001007 |
102 | 1799-1809 | Disease | denotes | depression | MESH:D000275 |
103 | 2093-2101 | Disease | denotes | COVID-19 | MESH:C000657245 |
LitCovid-PD-MONDO
Id | Subject | Object | Predicate | Lexical cue | mondo_id |
---|---|---|---|---|---|
T30 | 197-204 | Disease | denotes | anxiety | http://purl.obolibrary.org/obo/MONDO_0005618|http://purl.obolibrary.org/obo/MONDO_0011918 |
T32 | 1312-1331 | Disease | denotes | anxiety, depression | http://purl.obolibrary.org/obo/MONDO_0041086 |
T33 | 1312-1319 | Disease | denotes | anxiety | http://purl.obolibrary.org/obo/MONDO_0005618|http://purl.obolibrary.org/obo/MONDO_0011918 |
T35 | 1321-1331 | Disease | denotes | depression | http://purl.obolibrary.org/obo/MONDO_0002050 |
T36 | 1790-1809 | Disease | denotes | anxiety, depression | http://purl.obolibrary.org/obo/MONDO_0041086 |
T37 | 1790-1797 | Disease | denotes | anxiety | http://purl.obolibrary.org/obo/MONDO_0005618|http://purl.obolibrary.org/obo/MONDO_0011918 |
T39 | 1799-1809 | Disease | denotes | depression | http://purl.obolibrary.org/obo/MONDO_0002050 |
T40 | 2093-2101 | Disease | denotes | COVID-19 | http://purl.obolibrary.org/obo/MONDO_0100096 |
LitCovid-PD-CLO
Id | Subject | Object | Predicate | Lexical cue |
---|---|---|---|---|
T35 | 487-489 | http://purl.obolibrary.org/obo/CLO_0050507 | denotes | 22 |
T36 | 748-755 | http://purl.obolibrary.org/obo/BFO_0000030 | denotes | objects |
T37 | 1135-1141 | http://purl.obolibrary.org/obo/CLO_0001658 | denotes | active |
T38 | 1581-1582 | http://purl.obolibrary.org/obo/CLO_0001020 | denotes | a |
T39 | 1668-1671 | http://purl.obolibrary.org/obo/CLO_0051582 | denotes | has |
T40 | 2150-2154 | http://purl.obolibrary.org/obo/UBERON_0000473 | denotes | test |
T41 | 2213-2215 | http://purl.obolibrary.org/obo/CLO_0050507 | denotes | 22 |
LitCovid-PD-CHEBI
Id | Subject | Object | Predicate | Lexical cue | chebi_id |
---|---|---|---|---|---|
T2 | 1520-1529 | Chemical | denotes | indicator | http://purl.obolibrary.org/obo/CHEBI_47867 |
LitCovid-PD-HP
Id | Subject | Object | Predicate | Lexical cue | hp_id |
---|---|---|---|---|---|
T7 | 197-204 | Phenotype | denotes | anxiety | http://purl.obolibrary.org/obo/HP_0000739 |
T8 | 1312-1319 | Phenotype | denotes | anxiety | http://purl.obolibrary.org/obo/HP_0000739 |
T9 | 1321-1331 | Phenotype | denotes | depression | http://purl.obolibrary.org/obo/HP_0000716 |
T10 | 1790-1797 | Phenotype | denotes | anxiety | http://purl.obolibrary.org/obo/HP_0000739 |
T11 | 1799-1809 | Phenotype | denotes | depression | http://purl.obolibrary.org/obo/HP_0000716 |
LitCovid-PD-GO-BP
Id | Subject | Object | Predicate | Lexical cue |
---|---|---|---|---|
T10 | 332-348 | http://purl.obolibrary.org/obo/GO_0048731 | denotes | system developed |
T11 | 515-527 | http://purl.obolibrary.org/obo/GO_0035282 | denotes | segmentation |
T12 | 600-612 | http://purl.obolibrary.org/obo/GO_0035282 | denotes | segmentation |
T13 | 1257-1265 | http://purl.obolibrary.org/obo/GO_0007612 | denotes | learning |
LitCovid-sentences
Id | Subject | Object | Predicate | Lexical cue |
---|---|---|---|---|
T51 | 0-4 | Sentence | denotes | 2.2. |
T52 | 5-55 | Sentence | denotes | Measurement of Psychological Traits and Procedures |
T53 | 56-309 | Sentence | denotes | In this study, we used Online Ecological Recognition (OER) [20], which referred to the automatic recognition of psychological profile (e.g., anxiety, well-being, etc.) by using predictive models [17,20,21] based on ecological behavioral data from Weibo. |
T54 | 310-574 | Sentence | denotes | We employed Text Mind system developed by the Computational Cyber Psychology Laboratory at the Institute of Psychology, Chinese Academy of Sciences to extract content features [22], including Chinese word segmentation tool [17], and psychoanalytic dictionary [23]. |
T55 | 575-905 | Sentence | denotes | We used the Chinese word segmentation tool to divide users’ original microblog content into words/phrases with linguistic annotations, such as verbs, nouns, adverbials, and objects [24], and then extracted psychologically meaningful categories through the simplified Chinese LIWC (Language Inquiry and Word Count) dictionary [23]. |
T56 | 906-975 | Sentence | denotes | These lexical features were data sources for word frequency analysis. |
T57 | 976-1154 | Sentence | denotes | After feature extraction, we used the psychological prediction model [25] obtained from the preliminary training to predict the psychological profile of these active Weibo users. |
T58 | 1155-1446 | Sentence | denotes | These predictive models are tools developed for online psychology research based on big data and deep learning technologies, including emotional indicators (anxiety, depression, indignation, and Oxford happiness), cognitive indicators (social risk judgment and life satisfaction), and so on. |
T59 | 1447-1541 | Sentence | denotes | Figure 1 portrays the procedure from feature extraction to psychological indicator prediction. |
T60 | 1542-1630 | Sentence | denotes | All the prediction models have reached a moderate correlation with questionnaire scores. |
T61 | 1631-1712 | Sentence | denotes | The feasibility of predictive models has been repeatedly demonstrated [26,27,28]. |
T62 | 1713-1978 | Sentence | denotes | We calculated word frequency, scores of negative emotional indicators (i.e., anxiety, depression, and indignation), positive emotional indicators (i.e., Oxford happiness), and cognitive indicators (i.e., social risk and life satisfaction) of the collected messages. |
T63 | 1979-2304 | Sentence | denotes | We then compared the differences of psychological characteristics before and after the declaration of outbreak of COVID-19 on 20 January, 2020 through the paired sample t-test by using SPSS (Statistical Product and Service Solutions) 22, which is published by IBM (International Business Machines Corporation), New York, USA. |
2_test
Id | Subject | Object | Predicate | Lexical cue |
---|---|---|---|---|
32204411-27322382-49451143 | 570-572 | 27322382 | denotes | 23 |
32204411-27322382-49451144 | 901-903 | 27322382 | denotes | 23 |
32204411-28059682-49451145 | 1708-1710 | 28059682 | denotes | 28 |
T70343 | 570-572 | 27322382 | denotes | 23 |
T61461 | 901-903 | 27322382 | denotes | 23 |
T27610 | 1708-1710 | 28059682 | denotes | 28 |