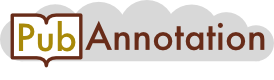
PMC:7116472 / 17639-24288
Annnotations
LitCovid-PubTator
{"project":"LitCovid-PubTator","denotations":[{"id":"251","span":{"begin":38,"end":46},"obj":"Species"},{"id":"252","span":{"begin":125,"end":133},"obj":"Species"},{"id":"253","span":{"begin":230,"end":238},"obj":"Species"},{"id":"254","span":{"begin":259,"end":267},"obj":"Species"},{"id":"255","span":{"begin":197,"end":206},"obj":"Disease"},{"id":"261","span":{"begin":1067,"end":1085},"obj":"Gene"},{"id":"262","span":{"begin":1012,"end":1018},"obj":"Chemical"},{"id":"263","span":{"begin":1051,"end":1055},"obj":"Chemical"},{"id":"264","span":{"begin":866,"end":875},"obj":"Disease"},{"id":"265","span":{"begin":1039,"end":1043},"obj":"Disease"},{"id":"267","span":{"begin":1672,"end":1681},"obj":"Disease"},{"id":"271","span":{"begin":1724,"end":1733},"obj":"Disease"},{"id":"272","span":{"begin":1771,"end":1776},"obj":"Disease"},{"id":"273","span":{"begin":1892,"end":1901},"obj":"Disease"},{"id":"279","span":{"begin":2136,"end":2144},"obj":"Species"},{"id":"280","span":{"begin":2245,"end":2253},"obj":"Species"},{"id":"281","span":{"begin":2348,"end":2356},"obj":"Species"},{"id":"282","span":{"begin":2377,"end":2385},"obj":"Species"},{"id":"283","span":{"begin":2317,"end":2326},"obj":"Disease"},{"id":"287","span":{"begin":2551,"end":2560},"obj":"Disease"},{"id":"288","span":{"begin":2763,"end":2772},"obj":"Disease"},{"id":"289","span":{"begin":2909,"end":2918},"obj":"Disease"},{"id":"293","span":{"begin":3073,"end":3082},"obj":"Disease"},{"id":"294","span":{"begin":3132,"end":3141},"obj":"Disease"},{"id":"295","span":{"begin":3425,"end":3434},"obj":"Disease"},{"id":"302","span":{"begin":3449,"end":3457},"obj":"Species"},{"id":"303","span":{"begin":3580,"end":3588},"obj":"Species"},{"id":"304","span":{"begin":3713,"end":3721},"obj":"Species"},{"id":"305","span":{"begin":3522,"end":3531},"obj":"Disease"},{"id":"306","span":{"begin":3648,"end":3657},"obj":"Disease"},{"id":"307","span":{"begin":3765,"end":3774},"obj":"Disease"},{"id":"316","span":{"begin":4263,"end":4271},"obj":"Species"},{"id":"317","span":{"begin":4065,"end":4074},"obj":"Disease"},{"id":"318","span":{"begin":4171,"end":4180},"obj":"Disease"},{"id":"319","span":{"begin":4296,"end":4305},"obj":"Disease"},{"id":"320","span":{"begin":4363,"end":4372},"obj":"Disease"},{"id":"321","span":{"begin":4573,"end":4581},"obj":"Disease"},{"id":"322","span":{"begin":4661,"end":4671},"obj":"Disease"},{"id":"323","span":{"begin":4762,"end":4771},"obj":"Disease"},{"id":"327","span":{"begin":4893,"end":4901},"obj":"Species"},{"id":"328","span":{"begin":5160,"end":5168},"obj":"Species"},{"id":"329","span":{"begin":5374,"end":5383},"obj":"Disease"},{"id":"331","span":{"begin":5966,"end":5975},"obj":"Disease"},{"id":"335","span":{"begin":6284,"end":6287},"obj":"Species"},{"id":"336","span":{"begin":6392,"end":6397},"obj":"Species"},{"id":"337","span":{"begin":6198,"end":6207},"obj":"Disease"}],"attributes":[{"id":"A251","pred":"tao:has_database_id","subj":"251","obj":"Tax:9606"},{"id":"A252","pred":"tao:has_database_id","subj":"252","obj":"Tax:9606"},{"id":"A253","pred":"tao:has_database_id","subj":"253","obj":"Tax:9606"},{"id":"A254","pred":"tao:has_database_id","subj":"254","obj":"Tax:9606"},{"id":"A255","pred":"tao:has_database_id","subj":"255","obj":"MESH:D003643"},{"id":"A261","pred":"tao:has_database_id","subj":"261","obj":"Gene:1401"},{"id":"A262","pred":"tao:has_database_id","subj":"262","obj":"MESH:D010100"},{"id":"A263","pred":"tao:has_database_id","subj":"263","obj":"MESH:D014508"},{"id":"A264","pred":"tao:has_database_id","subj":"264","obj":"MESH:D003643"},{"id":"A265","pred":"tao:has_database_id","subj":"265","obj":"MESH:D003128"},{"id":"A267","pred":"tao:has_database_id","subj":"267","obj":"MESH:D003643"},{"id":"A271","pred":"tao:has_database_id","subj":"271","obj":"MESH:D003643"},{"id":"A272","pred":"tao:has_database_id","subj":"272","obj":"MESH:D003643"},{"id":"A273","pred":"tao:has_database_id","subj":"273","obj":"MESH:D003643"},{"id":"A279","pred":"tao:has_database_id","subj":"279","obj":"Tax:9606"},{"id":"A280","pred":"tao:has_database_id","subj":"280","obj":"Tax:9606"},{"id":"A281","pred":"tao:has_database_id","subj":"281","obj":"Tax:9606"},{"id":"A282","pred":"tao:has_database_id","subj":"282","obj":"Tax:9606"},{"id":"A283","pred":"tao:has_database_id","subj":"283","obj":"MESH:D003643"},{"id":"A287","pred":"tao:has_database_id","subj":"287","obj":"MESH:D003643"},{"id":"A288","pred":"tao:has_database_id","subj":"288","obj":"MESH:D003643"},{"id":"A289","pred":"tao:has_database_id","subj":"289","obj":"MESH:D003643"},{"id":"A293","pred":"tao:has_database_id","subj":"293","obj":"MESH:D003643"},{"id":"A294","pred":"tao:has_database_id","subj":"294","obj":"MESH:D003643"},{"id":"A295","pred":"tao:has_database_id","subj":"295","obj":"MESH:D003643"},{"id":"A302","pred":"tao:has_database_id","subj":"302","obj":"Tax:9606"},{"id":"A303","pred":"tao:has_database_id","subj":"303","obj":"Tax:9606"},{"id":"A304","pred":"tao:has_database_id","subj":"304","obj":"Tax:9606"},{"id":"A305","pred":"tao:has_database_id","subj":"305","obj":"MESH:D003643"},{"id":"A306","pred":"tao:has_database_id","subj":"306","obj":"MESH:D003643"},{"id":"A307","pred":"tao:has_database_id","subj":"307","obj":"MESH:D003643"},{"id":"A316","pred":"tao:has_database_id","subj":"316","obj":"Tax:9606"},{"id":"A317","pred":"tao:has_database_id","subj":"317","obj":"MESH:D003643"},{"id":"A318","pred":"tao:has_database_id","subj":"318","obj":"MESH:D003643"},{"id":"A319","pred":"tao:has_database_id","subj":"319","obj":"MESH:D011014"},{"id":"A320","pred":"tao:has_database_id","subj":"320","obj":"MESH:D003643"},{"id":"A321","pred":"tao:has_database_id","subj":"321","obj":"MESH:C000657245"},{"id":"A322","pred":"tao:has_database_id","subj":"322","obj":"MESH:C000657245"},{"id":"A323","pred":"tao:has_database_id","subj":"323","obj":"MESH:D003643"},{"id":"A327","pred":"tao:has_database_id","subj":"327","obj":"Tax:9606"},{"id":"A328","pred":"tao:has_database_id","subj":"328","obj":"Tax:9606"},{"id":"A329","pred":"tao:has_database_id","subj":"329","obj":"MESH:D003643"},{"id":"A331","pred":"tao:has_database_id","subj":"331","obj":"MESH:D003643"},{"id":"A335","pred":"tao:has_database_id","subj":"335","obj":"Tax:9606"},{"id":"A336","pred":"tao:has_database_id","subj":"336","obj":"Tax:9606"},{"id":"A337","pred":"tao:has_database_id","subj":"337","obj":"MESH:D003643"}],"namespaces":[{"prefix":"Tax","uri":"https://www.ncbi.nlm.nih.gov/taxonomy/"},{"prefix":"MESH","uri":"https://id.nlm.nih.gov/mesh/"},{"prefix":"Gene","uri":"https://www.ncbi.nlm.nih.gov/gene/"},{"prefix":"CVCL","uri":"https://web.expasy.org/cellosaurus/CVCL_"}],"text":"Results\nWe collected data from 35 463 patients between 6 February 2020 and 20 May 2020 in the derivation cohort; 1275 (3.6%) patients had no outcome recorded and were considered alive. The overall mortality rate was 32.2% (11 426 patients). The median age of patients in the cohort was 73 years (interquartile range 59-83); 41.7% (14 741) were female and 76.0% (26 966) had at least one comorbidity. Table 1 shows demographic and clinical characteristics for the derivation and validation datasets.\n\nModel development\nWe identified 41 candidate predictor variables measured at hospital admission for model creation (fig 1, appendix 2). After the creation of a composite variable containing all seven individual comorbidities and the exclusion of 13 variables owing to high levels of missing values, 21 variables remained.\nWe identified eight important predictors of mortality by using generalised additive modelling with multiply imputed datasets: age, sex, number of comorbidities, respiratory rate, peripheral oxygen saturation, Glasgow coma scale, urea level, and C reactive protein (for variable selection process, see appendix 3). Given the need for a pragmatic score for use at the bedside, continuous variables were converted to factors with cut-off values chosen by using component smoothed functions (on linear predictor scale) from generalised additive modelling (appendix 4).\nOn entering variables into a penalised logistic regression model (least absolute shrinkage and selection operator), all variables were retained within the final model (appendix 5). We converted penalised regression coefficients into a prognostic index by using appropriate scaling (4C Mortality Score range 0-21 points; table 2).\nThe 4C Mortality Score showed good discrimination for death in hospital within the derivation cohort (table 3), with performance approaching that of the XGBoost model. The 4C Mortality Score showed good calibration (calibration intercept=0, slope=1, Brier score 0.170) across the range of risk and no adjustment to the model was required (appendix 11).\n\nModel validation\nThe validation cohort included data from 22 361 patients collected between 21 May 2020 and 29 June 2020 who had at least four weeks of follow-up; 743 (3.3%) patients had no outcome recorded and were considered alive. The overall mortality rate was 30.1% (6729 patients). The median age of patients in the cohort was 76 (interquartile range 60-85) years; 10 178 (45.6%) were female and 17 263 (77%) had at least one comorbidity (table 1).\nDiscrimination of the 4C Mortality Score in the validation cohort was similar to that of the XGBoost model (table 3). Calibration was also found to be excellent in the validation cohort: overall observed (30.1%) versus predicted (30.1%) mortality was equal (calibration-in-the-large=0) and calibration was excellent over the range of risk (slope=1, Brier score 0.171; fig 2). The 4C Mortality Score showed good performance in clinically relevant metrics across a range of cut-off values (table 4).\nFour risk groups were defined with corresponding mortality rates determined (table 5): low risk (0-3 score, mortality rate 1.2%), intermediate risk (4-8 score, 9.9%), high risk (9-14 score, 31.4%), and very high risk (≥15 score, 61.5%). Performance metrics showed a high sensitivity (99.7%) and negative predictive value (98.8%) for the low risk group, covering 7.4% of the cohort and a corresponding mortality rate of 1.2%.\nPatients in the intermediate risk group (score 4-8, n=4889, 21.9%) had a mortality rate of 9.9% (negative predictive value 90.1%). Patients in the high risk group (score 9-14, n=11 664, 52.2%) had a mortality rate of 31.4% (negative predictive value 68.6%), while patients scoring 15 or higher (n=4158, 18.6%) had a mortality rate of 61.5% (positive predictive value 61.5%). An interactive infographic is available at https://isaric4c.net/risk\n\nComparison with existing tools\nWe performed a systematic literature search and identified 15 risk stratification scores that could beapplied to these data.62228-40 The 4C Mortality Score compared well against these existing risk stratification scores in predicting in-hospital mortality (table 6, fig 3, upper panel). Risk stratification scores originally validated in patients with community acquired pneumonia (n=9) generally had higher discrimination for inhospital mortality in the validation cohort (eg, A-DROP (area under the receiver operating characteristic curve 0.74, 95% confidence interval 0.73 to 0.74) and E-CURB65 (0.76, 0.74 to 0.79)) than those developed within covid-19 cohorts (n=4: Surgisphere (0.63, 0.62 to 0.64), DL score (0.67, 0.66 to 0.68), COVID-GRAM (0.71, 0.68 to 0.74), and Xie score (0.73, 0.70 to 0.75)). Performance metrics for the 4C Mortality Score compared well against existing risk stratification scores at specified cut-off values (appendix 13).\nThe number of patients in whom risk stratification scores could be applied differed owing to certain variables not being available, either because of missingness or because they were not tested for or recorded in clinical practice. Seven scores could be applied to fewer than 2000 patients (\u003c10%) in the validation cohort owing to the requirement for biomarkers or physiological parameters that were not routinely captured (eg, lactate dehydrogenase). Decision curve analysis showed that the 4C Mortality Score had better clinical utility across a wide range of threshold risks compared with the best performing existing scores applicable to more than 50% of the validation cohort (A-DROP and CURB65; fig 3, lower panel).\n\nSensitivity analysis\nSensitivity analyses that used complete case data showed similar discrimination (appendix 7) and performance metrics (appendices 8 and 9) to analyses that used the imputed dataset. After stratification of the validation cohort into two geographical cohorts (validation north and south; appendix 14), discrimination remained similar for the 4C Mortality Score in the north subset (area under the receiver operating characteristic curve 0.77, 95% confidence interval 0.76 to 0.78) and south subset (0.76, 0.75 to 0.77; appendix 6).\nFinally, we checked discrimination of the 4C Mortality Score by sex and ethnic group (appendix 10). Discrimination was the same in men (area under the receiver operating characteristic curve 0.77, 95% confidence interval 0.76 to 0.78) and women (0.76, 0.75 to 0.77). Discrimination was better in all nonwhite ethnic groups compared with the white group: South Asian (0.82, 0.80 to 0.85), East Asian (0.85, 0.79 to 0.91), Black (0.83, 0.80 to 0.86), and other ethnic minority (0.81, 0.79 to 0.84)."}
LitCovid-sentences
{"project":"LitCovid-sentences","denotations":[{"id":"T118","span":{"begin":0,"end":7},"obj":"Sentence"},{"id":"T119","span":{"begin":8,"end":184},"obj":"Sentence"},{"id":"T120","span":{"begin":185,"end":240},"obj":"Sentence"},{"id":"T121","span":{"begin":241,"end":399},"obj":"Sentence"},{"id":"T122","span":{"begin":400,"end":498},"obj":"Sentence"},{"id":"T123","span":{"begin":500,"end":517},"obj":"Sentence"},{"id":"T124","span":{"begin":518,"end":635},"obj":"Sentence"},{"id":"T125","span":{"begin":636,"end":821},"obj":"Sentence"},{"id":"T126","span":{"begin":822,"end":1135},"obj":"Sentence"},{"id":"T127","span":{"begin":1136,"end":1386},"obj":"Sentence"},{"id":"T128","span":{"begin":1387,"end":1567},"obj":"Sentence"},{"id":"T129","span":{"begin":1568,"end":1716},"obj":"Sentence"},{"id":"T130","span":{"begin":1717,"end":1884},"obj":"Sentence"},{"id":"T131","span":{"begin":1885,"end":2069},"obj":"Sentence"},{"id":"T132","span":{"begin":2071,"end":2087},"obj":"Sentence"},{"id":"T133","span":{"begin":2088,"end":2304},"obj":"Sentence"},{"id":"T134","span":{"begin":2305,"end":2358},"obj":"Sentence"},{"id":"T135","span":{"begin":2359,"end":2525},"obj":"Sentence"},{"id":"T136","span":{"begin":2526,"end":2643},"obj":"Sentence"},{"id":"T137","span":{"begin":2644,"end":2901},"obj":"Sentence"},{"id":"T138","span":{"begin":2902,"end":3023},"obj":"Sentence"},{"id":"T139","span":{"begin":3024,"end":3260},"obj":"Sentence"},{"id":"T140","span":{"begin":3261,"end":3448},"obj":"Sentence"},{"id":"T141","span":{"begin":3449,"end":3579},"obj":"Sentence"},{"id":"T142","span":{"begin":3580,"end":3823},"obj":"Sentence"},{"id":"T143","span":{"begin":3824,"end":3892},"obj":"Sentence"},{"id":"T144","span":{"begin":3894,"end":3924},"obj":"Sentence"},{"id":"T145","span":{"begin":3925,"end":4211},"obj":"Sentence"},{"id":"T146","span":{"begin":4212,"end":4595},"obj":"Sentence"},{"id":"T147","span":{"begin":4596,"end":4730},"obj":"Sentence"},{"id":"T148","span":{"begin":4731,"end":4878},"obj":"Sentence"},{"id":"T149","span":{"begin":4879,"end":5110},"obj":"Sentence"},{"id":"T150","span":{"begin":5111,"end":5330},"obj":"Sentence"},{"id":"T151","span":{"begin":5331,"end":5600},"obj":"Sentence"},{"id":"T152","span":{"begin":5602,"end":5622},"obj":"Sentence"},{"id":"T153","span":{"begin":5623,"end":5803},"obj":"Sentence"},{"id":"T154","span":{"begin":5804,"end":6152},"obj":"Sentence"},{"id":"T155","span":{"begin":6153,"end":6252},"obj":"Sentence"},{"id":"T156","span":{"begin":6253,"end":6419},"obj":"Sentence"},{"id":"T157","span":{"begin":6420,"end":6506},"obj":"Sentence"},{"id":"T158","span":{"begin":6507,"end":6649},"obj":"Sentence"}],"namespaces":[{"prefix":"_base","uri":"http://pubannotation.org/ontology/tao.owl#"}],"text":"Results\nWe collected data from 35 463 patients between 6 February 2020 and 20 May 2020 in the derivation cohort; 1275 (3.6%) patients had no outcome recorded and were considered alive. The overall mortality rate was 32.2% (11 426 patients). The median age of patients in the cohort was 73 years (interquartile range 59-83); 41.7% (14 741) were female and 76.0% (26 966) had at least one comorbidity. Table 1 shows demographic and clinical characteristics for the derivation and validation datasets.\n\nModel development\nWe identified 41 candidate predictor variables measured at hospital admission for model creation (fig 1, appendix 2). After the creation of a composite variable containing all seven individual comorbidities and the exclusion of 13 variables owing to high levels of missing values, 21 variables remained.\nWe identified eight important predictors of mortality by using generalised additive modelling with multiply imputed datasets: age, sex, number of comorbidities, respiratory rate, peripheral oxygen saturation, Glasgow coma scale, urea level, and C reactive protein (for variable selection process, see appendix 3). Given the need for a pragmatic score for use at the bedside, continuous variables were converted to factors with cut-off values chosen by using component smoothed functions (on linear predictor scale) from generalised additive modelling (appendix 4).\nOn entering variables into a penalised logistic regression model (least absolute shrinkage and selection operator), all variables were retained within the final model (appendix 5). We converted penalised regression coefficients into a prognostic index by using appropriate scaling (4C Mortality Score range 0-21 points; table 2).\nThe 4C Mortality Score showed good discrimination for death in hospital within the derivation cohort (table 3), with performance approaching that of the XGBoost model. The 4C Mortality Score showed good calibration (calibration intercept=0, slope=1, Brier score 0.170) across the range of risk and no adjustment to the model was required (appendix 11).\n\nModel validation\nThe validation cohort included data from 22 361 patients collected between 21 May 2020 and 29 June 2020 who had at least four weeks of follow-up; 743 (3.3%) patients had no outcome recorded and were considered alive. The overall mortality rate was 30.1% (6729 patients). The median age of patients in the cohort was 76 (interquartile range 60-85) years; 10 178 (45.6%) were female and 17 263 (77%) had at least one comorbidity (table 1).\nDiscrimination of the 4C Mortality Score in the validation cohort was similar to that of the XGBoost model (table 3). Calibration was also found to be excellent in the validation cohort: overall observed (30.1%) versus predicted (30.1%) mortality was equal (calibration-in-the-large=0) and calibration was excellent over the range of risk (slope=1, Brier score 0.171; fig 2). The 4C Mortality Score showed good performance in clinically relevant metrics across a range of cut-off values (table 4).\nFour risk groups were defined with corresponding mortality rates determined (table 5): low risk (0-3 score, mortality rate 1.2%), intermediate risk (4-8 score, 9.9%), high risk (9-14 score, 31.4%), and very high risk (≥15 score, 61.5%). Performance metrics showed a high sensitivity (99.7%) and negative predictive value (98.8%) for the low risk group, covering 7.4% of the cohort and a corresponding mortality rate of 1.2%.\nPatients in the intermediate risk group (score 4-8, n=4889, 21.9%) had a mortality rate of 9.9% (negative predictive value 90.1%). Patients in the high risk group (score 9-14, n=11 664, 52.2%) had a mortality rate of 31.4% (negative predictive value 68.6%), while patients scoring 15 or higher (n=4158, 18.6%) had a mortality rate of 61.5% (positive predictive value 61.5%). An interactive infographic is available at https://isaric4c.net/risk\n\nComparison with existing tools\nWe performed a systematic literature search and identified 15 risk stratification scores that could beapplied to these data.62228-40 The 4C Mortality Score compared well against these existing risk stratification scores in predicting in-hospital mortality (table 6, fig 3, upper panel). Risk stratification scores originally validated in patients with community acquired pneumonia (n=9) generally had higher discrimination for inhospital mortality in the validation cohort (eg, A-DROP (area under the receiver operating characteristic curve 0.74, 95% confidence interval 0.73 to 0.74) and E-CURB65 (0.76, 0.74 to 0.79)) than those developed within covid-19 cohorts (n=4: Surgisphere (0.63, 0.62 to 0.64), DL score (0.67, 0.66 to 0.68), COVID-GRAM (0.71, 0.68 to 0.74), and Xie score (0.73, 0.70 to 0.75)). Performance metrics for the 4C Mortality Score compared well against existing risk stratification scores at specified cut-off values (appendix 13).\nThe number of patients in whom risk stratification scores could be applied differed owing to certain variables not being available, either because of missingness or because they were not tested for or recorded in clinical practice. Seven scores could be applied to fewer than 2000 patients (\u003c10%) in the validation cohort owing to the requirement for biomarkers or physiological parameters that were not routinely captured (eg, lactate dehydrogenase). Decision curve analysis showed that the 4C Mortality Score had better clinical utility across a wide range of threshold risks compared with the best performing existing scores applicable to more than 50% of the validation cohort (A-DROP and CURB65; fig 3, lower panel).\n\nSensitivity analysis\nSensitivity analyses that used complete case data showed similar discrimination (appendix 7) and performance metrics (appendices 8 and 9) to analyses that used the imputed dataset. After stratification of the validation cohort into two geographical cohorts (validation north and south; appendix 14), discrimination remained similar for the 4C Mortality Score in the north subset (area under the receiver operating characteristic curve 0.77, 95% confidence interval 0.76 to 0.78) and south subset (0.76, 0.75 to 0.77; appendix 6).\nFinally, we checked discrimination of the 4C Mortality Score by sex and ethnic group (appendix 10). Discrimination was the same in men (area under the receiver operating characteristic curve 0.77, 95% confidence interval 0.76 to 0.78) and women (0.76, 0.75 to 0.77). Discrimination was better in all nonwhite ethnic groups compared with the white group: South Asian (0.82, 0.80 to 0.85), East Asian (0.85, 0.79 to 0.91), Black (0.83, 0.80 to 0.86), and other ethnic minority (0.81, 0.79 to 0.84)."}
LitCovid-PD-HP
{"project":"LitCovid-PD-HP","denotations":[{"id":"T14","span":{"begin":1039,"end":1043},"obj":"Phenotype"},{"id":"T15","span":{"begin":4296,"end":4305},"obj":"Phenotype"}],"attributes":[{"id":"A14","pred":"hp_id","subj":"T14","obj":"http://purl.obolibrary.org/obo/HP_0001259"},{"id":"A15","pred":"hp_id","subj":"T15","obj":"http://purl.obolibrary.org/obo/HP_0002090"}],"text":"Results\nWe collected data from 35 463 patients between 6 February 2020 and 20 May 2020 in the derivation cohort; 1275 (3.6%) patients had no outcome recorded and were considered alive. The overall mortality rate was 32.2% (11 426 patients). The median age of patients in the cohort was 73 years (interquartile range 59-83); 41.7% (14 741) were female and 76.0% (26 966) had at least one comorbidity. Table 1 shows demographic and clinical characteristics for the derivation and validation datasets.\n\nModel development\nWe identified 41 candidate predictor variables measured at hospital admission for model creation (fig 1, appendix 2). After the creation of a composite variable containing all seven individual comorbidities and the exclusion of 13 variables owing to high levels of missing values, 21 variables remained.\nWe identified eight important predictors of mortality by using generalised additive modelling with multiply imputed datasets: age, sex, number of comorbidities, respiratory rate, peripheral oxygen saturation, Glasgow coma scale, urea level, and C reactive protein (for variable selection process, see appendix 3). Given the need for a pragmatic score for use at the bedside, continuous variables were converted to factors with cut-off values chosen by using component smoothed functions (on linear predictor scale) from generalised additive modelling (appendix 4).\nOn entering variables into a penalised logistic regression model (least absolute shrinkage and selection operator), all variables were retained within the final model (appendix 5). We converted penalised regression coefficients into a prognostic index by using appropriate scaling (4C Mortality Score range 0-21 points; table 2).\nThe 4C Mortality Score showed good discrimination for death in hospital within the derivation cohort (table 3), with performance approaching that of the XGBoost model. The 4C Mortality Score showed good calibration (calibration intercept=0, slope=1, Brier score 0.170) across the range of risk and no adjustment to the model was required (appendix 11).\n\nModel validation\nThe validation cohort included data from 22 361 patients collected between 21 May 2020 and 29 June 2020 who had at least four weeks of follow-up; 743 (3.3%) patients had no outcome recorded and were considered alive. The overall mortality rate was 30.1% (6729 patients). The median age of patients in the cohort was 76 (interquartile range 60-85) years; 10 178 (45.6%) were female and 17 263 (77%) had at least one comorbidity (table 1).\nDiscrimination of the 4C Mortality Score in the validation cohort was similar to that of the XGBoost model (table 3). Calibration was also found to be excellent in the validation cohort: overall observed (30.1%) versus predicted (30.1%) mortality was equal (calibration-in-the-large=0) and calibration was excellent over the range of risk (slope=1, Brier score 0.171; fig 2). The 4C Mortality Score showed good performance in clinically relevant metrics across a range of cut-off values (table 4).\nFour risk groups were defined with corresponding mortality rates determined (table 5): low risk (0-3 score, mortality rate 1.2%), intermediate risk (4-8 score, 9.9%), high risk (9-14 score, 31.4%), and very high risk (≥15 score, 61.5%). Performance metrics showed a high sensitivity (99.7%) and negative predictive value (98.8%) for the low risk group, covering 7.4% of the cohort and a corresponding mortality rate of 1.2%.\nPatients in the intermediate risk group (score 4-8, n=4889, 21.9%) had a mortality rate of 9.9% (negative predictive value 90.1%). Patients in the high risk group (score 9-14, n=11 664, 52.2%) had a mortality rate of 31.4% (negative predictive value 68.6%), while patients scoring 15 or higher (n=4158, 18.6%) had a mortality rate of 61.5% (positive predictive value 61.5%). An interactive infographic is available at https://isaric4c.net/risk\n\nComparison with existing tools\nWe performed a systematic literature search and identified 15 risk stratification scores that could beapplied to these data.62228-40 The 4C Mortality Score compared well against these existing risk stratification scores in predicting in-hospital mortality (table 6, fig 3, upper panel). Risk stratification scores originally validated in patients with community acquired pneumonia (n=9) generally had higher discrimination for inhospital mortality in the validation cohort (eg, A-DROP (area under the receiver operating characteristic curve 0.74, 95% confidence interval 0.73 to 0.74) and E-CURB65 (0.76, 0.74 to 0.79)) than those developed within covid-19 cohorts (n=4: Surgisphere (0.63, 0.62 to 0.64), DL score (0.67, 0.66 to 0.68), COVID-GRAM (0.71, 0.68 to 0.74), and Xie score (0.73, 0.70 to 0.75)). Performance metrics for the 4C Mortality Score compared well against existing risk stratification scores at specified cut-off values (appendix 13).\nThe number of patients in whom risk stratification scores could be applied differed owing to certain variables not being available, either because of missingness or because they were not tested for or recorded in clinical practice. Seven scores could be applied to fewer than 2000 patients (\u003c10%) in the validation cohort owing to the requirement for biomarkers or physiological parameters that were not routinely captured (eg, lactate dehydrogenase). Decision curve analysis showed that the 4C Mortality Score had better clinical utility across a wide range of threshold risks compared with the best performing existing scores applicable to more than 50% of the validation cohort (A-DROP and CURB65; fig 3, lower panel).\n\nSensitivity analysis\nSensitivity analyses that used complete case data showed similar discrimination (appendix 7) and performance metrics (appendices 8 and 9) to analyses that used the imputed dataset. After stratification of the validation cohort into two geographical cohorts (validation north and south; appendix 14), discrimination remained similar for the 4C Mortality Score in the north subset (area under the receiver operating characteristic curve 0.77, 95% confidence interval 0.76 to 0.78) and south subset (0.76, 0.75 to 0.77; appendix 6).\nFinally, we checked discrimination of the 4C Mortality Score by sex and ethnic group (appendix 10). Discrimination was the same in men (area under the receiver operating characteristic curve 0.77, 95% confidence interval 0.76 to 0.78) and women (0.76, 0.75 to 0.77). Discrimination was better in all nonwhite ethnic groups compared with the white group: South Asian (0.82, 0.80 to 0.85), East Asian (0.85, 0.79 to 0.91), Black (0.83, 0.80 to 0.86), and other ethnic minority (0.81, 0.79 to 0.84)."}