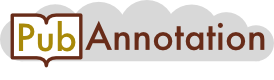
PMC:7073332 / 32589-38199
Annnotations
LitCovid-PubTator
{"project":"LitCovid-PubTator","denotations":[{"id":"1003","span":{"begin":5129,"end":5138},"obj":"Species"},{"id":"1004","span":{"begin":5139,"end":5149},"obj":"Species"},{"id":"1031","span":{"begin":1868,"end":1873},"obj":"Gene"},{"id":"1032","span":{"begin":930,"end":939},"obj":"Species"},{"id":"1033","span":{"begin":940,"end":950},"obj":"Species"},{"id":"1034","span":{"begin":1043,"end":1052},"obj":"Species"},{"id":"1035","span":{"begin":1053,"end":1063},"obj":"Species"},{"id":"1036","span":{"begin":1111,"end":1120},"obj":"Species"},{"id":"1037","span":{"begin":1121,"end":1131},"obj":"Species"},{"id":"1038","span":{"begin":1791,"end":1796},"obj":"Species"},{"id":"1039","span":{"begin":1803,"end":1816},"obj":"Species"},{"id":"1040","span":{"begin":2325,"end":2330},"obj":"Species"},{"id":"1041","span":{"begin":2413,"end":2422},"obj":"Species"},{"id":"1042","span":{"begin":2423,"end":2433},"obj":"Species"},{"id":"1043","span":{"begin":3014,"end":3023},"obj":"Species"},{"id":"1044","span":{"begin":3024,"end":3034},"obj":"Species"},{"id":"1045","span":{"begin":1695,"end":1705},"obj":"Chemical"},{"id":"1046","span":{"begin":1734,"end":1744},"obj":"Chemical"},{"id":"1047","span":{"begin":1877,"end":1887},"obj":"Chemical"},{"id":"1060","span":{"begin":3786,"end":3795},"obj":"Species"},{"id":"1061","span":{"begin":3796,"end":3806},"obj":"Species"},{"id":"1062","span":{"begin":4391,"end":4396},"obj":"Species"},{"id":"1063","span":{"begin":4500,"end":4504},"obj":"Species"},{"id":"1064","span":{"begin":4363,"end":4367},"obj":"Species"},{"id":"1065","span":{"begin":3464,"end":3474},"obj":"Chemical"},{"id":"1066","span":{"begin":3713,"end":3723},"obj":"Chemical"},{"id":"1067","span":{"begin":4720,"end":4730},"obj":"Chemical"},{"id":"1068","span":{"begin":3332,"end":3340},"obj":"Disease"},{"id":"1069","span":{"begin":3497,"end":3523},"obj":"Disease"},{"id":"1070","span":{"begin":3654,"end":3674},"obj":"Disease"},{"id":"1071","span":{"begin":3686,"end":3695},"obj":"Disease"}],"attributes":[{"id":"A1003","pred":"tao:has_database_id","subj":"1003","obj":"Tax:2697049"},{"id":"A1004","pred":"tao:has_database_id","subj":"1004","obj":"Tax:2697049"},{"id":"A1031","pred":"tao:has_database_id","subj":"1031","obj":"Gene:1974"},{"id":"A1032","pred":"tao:has_database_id","subj":"1032","obj":"Tax:2697049"},{"id":"A1033","pred":"tao:has_database_id","subj":"1033","obj":"Tax:2697049"},{"id":"A1034","pred":"tao:has_database_id","subj":"1034","obj":"Tax:2697049"},{"id":"A1035","pred":"tao:has_database_id","subj":"1035","obj":"Tax:2697049"},{"id":"A1036","pred":"tao:has_database_id","subj":"1036","obj":"Tax:2697049"},{"id":"A1037","pred":"tao:has_database_id","subj":"1037","obj":"Tax:2697049"},{"id":"A1038","pred":"tao:has_database_id","subj":"1038","obj":"Tax:1570291"},{"id":"A1039","pred":"tao:has_database_id","subj":"1039","obj":"Tax:11118"},{"id":"A1040","pred":"tao:has_database_id","subj":"1040","obj":"Tax:9606"},{"id":"A1041","pred":"tao:has_database_id","subj":"1041","obj":"Tax:2697049"},{"id":"A1042","pred":"tao:has_database_id","subj":"1042","obj":"Tax:2697049"},{"id":"A1043","pred":"tao:has_database_id","subj":"1043","obj":"Tax:2697049"},{"id":"A1044","pred":"tao:has_database_id","subj":"1044","obj":"Tax:2697049"},{"id":"A1045","pred":"tao:has_database_id","subj":"1045","obj":"MESH:C489897"},{"id":"A1047","pred":"tao:has_database_id","subj":"1047","obj":"MESH:C489897"},{"id":"A1060","pred":"tao:has_database_id","subj":"1060","obj":"Tax:2697049"},{"id":"A1061","pred":"tao:has_database_id","subj":"1061","obj":"Tax:2697049"},{"id":"A1062","pred":"tao:has_database_id","subj":"1062","obj":"Tax:9606"},{"id":"A1063","pred":"tao:has_database_id","subj":"1063","obj":"Tax:2697049"},{"id":"A1064","pred":"tao:has_database_id","subj":"1064","obj":"Tax:694448"},{"id":"A1065","pred":"tao:has_database_id","subj":"1065","obj":"MESH:D019804"},{"id":"A1066","pred":"tao:has_database_id","subj":"1066","obj":"MESH:D019804"},{"id":"A1067","pred":"tao:has_database_id","subj":"1067","obj":"MESH:C000606551"},{"id":"A1068","pred":"tao:has_database_id","subj":"1068","obj":"MESH:D064420"},{"id":"A1069","pred":"tao:has_database_id","subj":"1069","obj":"MESH:D015212"},{"id":"A1070","pred":"tao:has_database_id","subj":"1070","obj":"MESH:D008171"},{"id":"A1071","pred":"tao:has_database_id","subj":"1071","obj":"MESH:D011014"}],"namespaces":[{"prefix":"Tax","uri":"https://www.ncbi.nlm.nih.gov/taxonomy/"},{"prefix":"MESH","uri":"https://id.nlm.nih.gov/mesh/"},{"prefix":"Gene","uri":"https://www.ncbi.nlm.nih.gov/gene/"},{"prefix":"CVCL","uri":"https://web.expasy.org/cellosaurus/CVCL_"}],"text":"Discussion\nIn this study, we presented a network-based methodology for systematic identification of putative repurposable drugs and drug combinations for potential treatment of 2019-nCoV/SARS-CoV-2. Integration of drug–target networks, HCoV–host interactions, HCoV-induced transcriptome in human cell lines, and human protein–protein interactome network are essential for such identification. Based on comprehensive evaluation, we prioritized 16 candidate repurposable drugs (Fig. 5) and 3 potential drug combinations (Fig. 6) for targeting 2019-nCoV/SARS-CoV-2. However, although the majority of predictions have been validated by various literature data (Table 1), all network-predicted repurposable drugs and drug combinations must be validated in various 2019-nCoV/SARS-CoV-2 experimental assays64 and randomized clinical trials before being used in patients.\nWe acknowledge several limitations in the current study. Although 2019-nCoV/SARS-CoV-2 shared high nucleotide sequence identity with other HCoVs (Fig. 2), our predictions are not 2019-nCoV/SARS-CoV-2 specific by lack of the known host proteins on 2019-nCoV/SARS-CoV-2. We used a low binding affinity value of 10 μM as a threshold to define a physical drug–target interaction. However, a stronger binding affinity threshold (e.g., 1 μM) may be a more suitable cut-off in drug discovery, although it will generate a smaller drug–target network. Although sizeable efforts were made for assembling large scale, experimentally reported drug–target networks from publicly available databases, the network data may be incomplete and some drug–target interactions may be functional associations, instead of physical bindings. For example, Silvestrol, a natural product from the flavagline, was found to have antiviral activity against Ebola75 and Coronaviruses76. After adding its target, an RNA helicase enzyme EIF4A76, silvestrol was predicted to be significantly associated with HCoVs (Z = –1.24, P = 0.041) by network proximity analysis. To increase coverage of drug–target networks, we may use computational approaches to systematically predict the drug-target interactions further25,26. In addition, the collected virus–host interactions are far from completeness and the quality can be influenced by multiple factors, including different experimental assays and human cell line models. We may computationally predict a new virus–host interactome for 2019-nCoV/SARS-CoV-2 using sequence-based and structure-based approaches77. Drug targets representing nodes within cellular networks are often intrinsically coupled with both therapeutic and adverse profiles78, as drugs can inhibit or activate protein functions (including antagonists vs. agonists). The current systems pharmacology model cannot separate therapeutic (antiviral) effects from those predictions due to lack of detailed pharmacological effects of drug targets and unknown functional consequences of virus–host interactions. Comprehensive identification of the virus–host interactome for 2019-nCoV/SARS-CoV-2, with specific biological effects using functional genomics assays79,80, will significantly improve the accuracy of the proposed network-based methodologies further.\nOwing to a lack of the complete drug-target information (such as the molecular “promiscuity” of drugs), the dose–response and dose–toxicity effects for both repurposable drugs and drug combinations cannot be identified in the current network models. For example, Mesalazine, an approved drug for inflammatory bowel disease, is a top network-predicted repurposable drug associated with HCoVs (Fig. 5a). Yet, several clinical studies showed the potential pulmonary toxicities (including pneumonia) associated with mesalazine usage81,82. Integration of lung-specific gene expression23 of 2019-nCoV/SARS-CoV-2 host proteins and physiologically based pharmacokinetic modeling83 may reduce side effects of repurposable drugs or drug combinations. Preclinical studies are warranted to evaluate in vivo efficiency and side effects before clinical trials. Furthermore, we only limited to predict pairwise drug combinations based on our previous network-based framework28. However, we expect that our methodology remain to be a useful network-based tool for prediction of combining multiple drugs toward exploring network relationships of multiple drugs’ targets with the HCoV–host subnetwork in the human interactome. Finally, we aimed to systematically identify repurposable drugs by specifically targeting nCoV host proteins only. Thus, our current network models cannot predict repurposable drugs from the existing anti-virus drugs that target virus proteins only. Thus, combination of the existing anti-virus drugs (such as remdesivir64) with the network-predicted repurposable drugs (Fig. 5) or drug combinations (Fig. 6) may improve coverage of current network-based methodologies by utilizing multi-layer network framework16.\nIn conclusion, this study offers a powerful, integrative network-based systems pharmacology methodology for rapid identification of repurposable drugs and drug combinations for the potential treatment of 2019-nCoV/SARS-CoV-2. Our approach can minimize the translational gap between preclinical testing results and clinical outcomes, which is a significant problem in the rapid development of efficient treatment strategies for the emerging 2019-nCoV/SARS-CoV-2 outbreak. From a translational perspective, if broadly applied, the network tools developed here could help develop effective treatment strategies for other emerging viral infections and other human complex diseases as well."}
LitCovid-PD-FMA-UBERON
{"project":"LitCovid-PD-FMA-UBERON","denotations":[{"id":"T166","span":{"begin":296,"end":300},"obj":"Body_part"},{"id":"T167","span":{"begin":318,"end":325},"obj":"Body_part"},{"id":"T168","span":{"begin":326,"end":333},"obj":"Body_part"},{"id":"T169","span":{"begin":963,"end":973},"obj":"Body_part"},{"id":"T170","span":{"begin":1099,"end":1107},"obj":"Body_part"},{"id":"T171","span":{"begin":1848,"end":1851},"obj":"Body_part"},{"id":"T172","span":{"begin":2331,"end":2335},"obj":"Body_part"},{"id":"T173","span":{"begin":2657,"end":2664},"obj":"Body_part"},{"id":"T174","span":{"begin":3510,"end":3515},"obj":"Body_part"},{"id":"T175","span":{"begin":3751,"end":3755},"obj":"Body_part"},{"id":"T176","span":{"begin":3765,"end":3769},"obj":"Body_part"},{"id":"T177","span":{"begin":3812,"end":3820},"obj":"Body_part"},{"id":"T178","span":{"begin":4510,"end":4518},"obj":"Body_part"},{"id":"T179","span":{"begin":4645,"end":4653},"obj":"Body_part"}],"attributes":[{"id":"A166","pred":"fma_id","subj":"T166","obj":"http://purl.org/sig/ont/fma/fma68646"},{"id":"A167","pred":"fma_id","subj":"T167","obj":"http://purl.org/sig/ont/fma/fma67257"},{"id":"A168","pred":"fma_id","subj":"T168","obj":"http://purl.org/sig/ont/fma/fma67257"},{"id":"A169","pred":"fma_id","subj":"T169","obj":"http://purl.org/sig/ont/fma/fma82740"},{"id":"A170","pred":"fma_id","subj":"T170","obj":"http://purl.org/sig/ont/fma/fma67257"},{"id":"A171","pred":"fma_id","subj":"T171","obj":"http://purl.org/sig/ont/fma/fma67095"},{"id":"A172","pred":"fma_id","subj":"T172","obj":"http://purl.org/sig/ont/fma/fma68646"},{"id":"A173","pred":"fma_id","subj":"T173","obj":"http://purl.org/sig/ont/fma/fma67257"},{"id":"A174","pred":"fma_id","subj":"T174","obj":"http://purl.org/sig/ont/fma/fma7199"},{"id":"A175","pred":"fma_id","subj":"T175","obj":"http://purl.org/sig/ont/fma/fma7195"},{"id":"A176","pred":"fma_id","subj":"T176","obj":"http://purl.org/sig/ont/fma/fma74402"},{"id":"A177","pred":"fma_id","subj":"T177","obj":"http://purl.org/sig/ont/fma/fma67257"},{"id":"A178","pred":"fma_id","subj":"T178","obj":"http://purl.org/sig/ont/fma/fma67257"},{"id":"A179","pred":"fma_id","subj":"T179","obj":"http://purl.org/sig/ont/fma/fma67257"}],"text":"Discussion\nIn this study, we presented a network-based methodology for systematic identification of putative repurposable drugs and drug combinations for potential treatment of 2019-nCoV/SARS-CoV-2. Integration of drug–target networks, HCoV–host interactions, HCoV-induced transcriptome in human cell lines, and human protein–protein interactome network are essential for such identification. Based on comprehensive evaluation, we prioritized 16 candidate repurposable drugs (Fig. 5) and 3 potential drug combinations (Fig. 6) for targeting 2019-nCoV/SARS-CoV-2. However, although the majority of predictions have been validated by various literature data (Table 1), all network-predicted repurposable drugs and drug combinations must be validated in various 2019-nCoV/SARS-CoV-2 experimental assays64 and randomized clinical trials before being used in patients.\nWe acknowledge several limitations in the current study. Although 2019-nCoV/SARS-CoV-2 shared high nucleotide sequence identity with other HCoVs (Fig. 2), our predictions are not 2019-nCoV/SARS-CoV-2 specific by lack of the known host proteins on 2019-nCoV/SARS-CoV-2. We used a low binding affinity value of 10 μM as a threshold to define a physical drug–target interaction. However, a stronger binding affinity threshold (e.g., 1 μM) may be a more suitable cut-off in drug discovery, although it will generate a smaller drug–target network. Although sizeable efforts were made for assembling large scale, experimentally reported drug–target networks from publicly available databases, the network data may be incomplete and some drug–target interactions may be functional associations, instead of physical bindings. For example, Silvestrol, a natural product from the flavagline, was found to have antiviral activity against Ebola75 and Coronaviruses76. After adding its target, an RNA helicase enzyme EIF4A76, silvestrol was predicted to be significantly associated with HCoVs (Z = –1.24, P = 0.041) by network proximity analysis. To increase coverage of drug–target networks, we may use computational approaches to systematically predict the drug-target interactions further25,26. In addition, the collected virus–host interactions are far from completeness and the quality can be influenced by multiple factors, including different experimental assays and human cell line models. We may computationally predict a new virus–host interactome for 2019-nCoV/SARS-CoV-2 using sequence-based and structure-based approaches77. Drug targets representing nodes within cellular networks are often intrinsically coupled with both therapeutic and adverse profiles78, as drugs can inhibit or activate protein functions (including antagonists vs. agonists). The current systems pharmacology model cannot separate therapeutic (antiviral) effects from those predictions due to lack of detailed pharmacological effects of drug targets and unknown functional consequences of virus–host interactions. Comprehensive identification of the virus–host interactome for 2019-nCoV/SARS-CoV-2, with specific biological effects using functional genomics assays79,80, will significantly improve the accuracy of the proposed network-based methodologies further.\nOwing to a lack of the complete drug-target information (such as the molecular “promiscuity” of drugs), the dose–response and dose–toxicity effects for both repurposable drugs and drug combinations cannot be identified in the current network models. For example, Mesalazine, an approved drug for inflammatory bowel disease, is a top network-predicted repurposable drug associated with HCoVs (Fig. 5a). Yet, several clinical studies showed the potential pulmonary toxicities (including pneumonia) associated with mesalazine usage81,82. Integration of lung-specific gene expression23 of 2019-nCoV/SARS-CoV-2 host proteins and physiologically based pharmacokinetic modeling83 may reduce side effects of repurposable drugs or drug combinations. Preclinical studies are warranted to evaluate in vivo efficiency and side effects before clinical trials. Furthermore, we only limited to predict pairwise drug combinations based on our previous network-based framework28. However, we expect that our methodology remain to be a useful network-based tool for prediction of combining multiple drugs toward exploring network relationships of multiple drugs’ targets with the HCoV–host subnetwork in the human interactome. Finally, we aimed to systematically identify repurposable drugs by specifically targeting nCoV host proteins only. Thus, our current network models cannot predict repurposable drugs from the existing anti-virus drugs that target virus proteins only. Thus, combination of the existing anti-virus drugs (such as remdesivir64) with the network-predicted repurposable drugs (Fig. 5) or drug combinations (Fig. 6) may improve coverage of current network-based methodologies by utilizing multi-layer network framework16.\nIn conclusion, this study offers a powerful, integrative network-based systems pharmacology methodology for rapid identification of repurposable drugs and drug combinations for the potential treatment of 2019-nCoV/SARS-CoV-2. Our approach can minimize the translational gap between preclinical testing results and clinical outcomes, which is a significant problem in the rapid development of efficient treatment strategies for the emerging 2019-nCoV/SARS-CoV-2 outbreak. From a translational perspective, if broadly applied, the network tools developed here could help develop effective treatment strategies for other emerging viral infections and other human complex diseases as well."}
LitCovid-PD-UBERON
{"project":"LitCovid-PD-UBERON","denotations":[{"id":"T9","span":{"begin":1464,"end":1469},"obj":"Body_part"},{"id":"T10","span":{"begin":3751,"end":3755},"obj":"Body_part"}],"attributes":[{"id":"A9","pred":"uberon_id","subj":"T9","obj":"http://purl.obolibrary.org/obo/UBERON_0002542"},{"id":"A10","pred":"uberon_id","subj":"T10","obj":"http://purl.obolibrary.org/obo/UBERON_0002048"}],"text":"Discussion\nIn this study, we presented a network-based methodology for systematic identification of putative repurposable drugs and drug combinations for potential treatment of 2019-nCoV/SARS-CoV-2. Integration of drug–target networks, HCoV–host interactions, HCoV-induced transcriptome in human cell lines, and human protein–protein interactome network are essential for such identification. Based on comprehensive evaluation, we prioritized 16 candidate repurposable drugs (Fig. 5) and 3 potential drug combinations (Fig. 6) for targeting 2019-nCoV/SARS-CoV-2. However, although the majority of predictions have been validated by various literature data (Table 1), all network-predicted repurposable drugs and drug combinations must be validated in various 2019-nCoV/SARS-CoV-2 experimental assays64 and randomized clinical trials before being used in patients.\nWe acknowledge several limitations in the current study. Although 2019-nCoV/SARS-CoV-2 shared high nucleotide sequence identity with other HCoVs (Fig. 2), our predictions are not 2019-nCoV/SARS-CoV-2 specific by lack of the known host proteins on 2019-nCoV/SARS-CoV-2. We used a low binding affinity value of 10 μM as a threshold to define a physical drug–target interaction. However, a stronger binding affinity threshold (e.g., 1 μM) may be a more suitable cut-off in drug discovery, although it will generate a smaller drug–target network. Although sizeable efforts were made for assembling large scale, experimentally reported drug–target networks from publicly available databases, the network data may be incomplete and some drug–target interactions may be functional associations, instead of physical bindings. For example, Silvestrol, a natural product from the flavagline, was found to have antiviral activity against Ebola75 and Coronaviruses76. After adding its target, an RNA helicase enzyme EIF4A76, silvestrol was predicted to be significantly associated with HCoVs (Z = –1.24, P = 0.041) by network proximity analysis. To increase coverage of drug–target networks, we may use computational approaches to systematically predict the drug-target interactions further25,26. In addition, the collected virus–host interactions are far from completeness and the quality can be influenced by multiple factors, including different experimental assays and human cell line models. We may computationally predict a new virus–host interactome for 2019-nCoV/SARS-CoV-2 using sequence-based and structure-based approaches77. Drug targets representing nodes within cellular networks are often intrinsically coupled with both therapeutic and adverse profiles78, as drugs can inhibit or activate protein functions (including antagonists vs. agonists). The current systems pharmacology model cannot separate therapeutic (antiviral) effects from those predictions due to lack of detailed pharmacological effects of drug targets and unknown functional consequences of virus–host interactions. Comprehensive identification of the virus–host interactome for 2019-nCoV/SARS-CoV-2, with specific biological effects using functional genomics assays79,80, will significantly improve the accuracy of the proposed network-based methodologies further.\nOwing to a lack of the complete drug-target information (such as the molecular “promiscuity” of drugs), the dose–response and dose–toxicity effects for both repurposable drugs and drug combinations cannot be identified in the current network models. For example, Mesalazine, an approved drug for inflammatory bowel disease, is a top network-predicted repurposable drug associated with HCoVs (Fig. 5a). Yet, several clinical studies showed the potential pulmonary toxicities (including pneumonia) associated with mesalazine usage81,82. Integration of lung-specific gene expression23 of 2019-nCoV/SARS-CoV-2 host proteins and physiologically based pharmacokinetic modeling83 may reduce side effects of repurposable drugs or drug combinations. Preclinical studies are warranted to evaluate in vivo efficiency and side effects before clinical trials. Furthermore, we only limited to predict pairwise drug combinations based on our previous network-based framework28. However, we expect that our methodology remain to be a useful network-based tool for prediction of combining multiple drugs toward exploring network relationships of multiple drugs’ targets with the HCoV–host subnetwork in the human interactome. Finally, we aimed to systematically identify repurposable drugs by specifically targeting nCoV host proteins only. Thus, our current network models cannot predict repurposable drugs from the existing anti-virus drugs that target virus proteins only. Thus, combination of the existing anti-virus drugs (such as remdesivir64) with the network-predicted repurposable drugs (Fig. 5) or drug combinations (Fig. 6) may improve coverage of current network-based methodologies by utilizing multi-layer network framework16.\nIn conclusion, this study offers a powerful, integrative network-based systems pharmacology methodology for rapid identification of repurposable drugs and drug combinations for the potential treatment of 2019-nCoV/SARS-CoV-2. Our approach can minimize the translational gap between preclinical testing results and clinical outcomes, which is a significant problem in the rapid development of efficient treatment strategies for the emerging 2019-nCoV/SARS-CoV-2 outbreak. From a translational perspective, if broadly applied, the network tools developed here could help develop effective treatment strategies for other emerging viral infections and other human complex diseases as well."}
LitCovid-PD-MONDO
{"project":"LitCovid-PD-MONDO","denotations":[{"id":"T143","span":{"begin":187,"end":195},"obj":"Disease"},{"id":"T144","span":{"begin":551,"end":559},"obj":"Disease"},{"id":"T145","span":{"begin":769,"end":777},"obj":"Disease"},{"id":"T146","span":{"begin":940,"end":948},"obj":"Disease"},{"id":"T147","span":{"begin":1053,"end":1061},"obj":"Disease"},{"id":"T148","span":{"begin":1121,"end":1129},"obj":"Disease"},{"id":"T149","span":{"begin":2423,"end":2431},"obj":"Disease"},{"id":"T150","span":{"begin":3024,"end":3032},"obj":"Disease"},{"id":"T151","span":{"begin":3497,"end":3523},"obj":"Disease"},{"id":"T152","span":{"begin":3686,"end":3695},"obj":"Disease"},{"id":"T153","span":{"begin":3796,"end":3804},"obj":"Disease"},{"id":"T154","span":{"begin":5139,"end":5147},"obj":"Disease"},{"id":"T155","span":{"begin":5375,"end":5383},"obj":"Disease"},{"id":"T156","span":{"begin":5552,"end":5568},"obj":"Disease"}],"attributes":[{"id":"A143","pred":"mondo_id","subj":"T143","obj":"http://purl.obolibrary.org/obo/MONDO_0005091"},{"id":"A144","pred":"mondo_id","subj":"T144","obj":"http://purl.obolibrary.org/obo/MONDO_0005091"},{"id":"A145","pred":"mondo_id","subj":"T145","obj":"http://purl.obolibrary.org/obo/MONDO_0005091"},{"id":"A146","pred":"mondo_id","subj":"T146","obj":"http://purl.obolibrary.org/obo/MONDO_0005091"},{"id":"A147","pred":"mondo_id","subj":"T147","obj":"http://purl.obolibrary.org/obo/MONDO_0005091"},{"id":"A148","pred":"mondo_id","subj":"T148","obj":"http://purl.obolibrary.org/obo/MONDO_0005091"},{"id":"A149","pred":"mondo_id","subj":"T149","obj":"http://purl.obolibrary.org/obo/MONDO_0005091"},{"id":"A150","pred":"mondo_id","subj":"T150","obj":"http://purl.obolibrary.org/obo/MONDO_0005091"},{"id":"A151","pred":"mondo_id","subj":"T151","obj":"http://purl.obolibrary.org/obo/MONDO_0005265"},{"id":"A152","pred":"mondo_id","subj":"T152","obj":"http://purl.obolibrary.org/obo/MONDO_0005249"},{"id":"A153","pred":"mondo_id","subj":"T153","obj":"http://purl.obolibrary.org/obo/MONDO_0005091"},{"id":"A154","pred":"mondo_id","subj":"T154","obj":"http://purl.obolibrary.org/obo/MONDO_0005091"},{"id":"A155","pred":"mondo_id","subj":"T155","obj":"http://purl.obolibrary.org/obo/MONDO_0005091"},{"id":"A156","pred":"mondo_id","subj":"T156","obj":"http://purl.obolibrary.org/obo/MONDO_0005108"}],"text":"Discussion\nIn this study, we presented a network-based methodology for systematic identification of putative repurposable drugs and drug combinations for potential treatment of 2019-nCoV/SARS-CoV-2. Integration of drug–target networks, HCoV–host interactions, HCoV-induced transcriptome in human cell lines, and human protein–protein interactome network are essential for such identification. Based on comprehensive evaluation, we prioritized 16 candidate repurposable drugs (Fig. 5) and 3 potential drug combinations (Fig. 6) for targeting 2019-nCoV/SARS-CoV-2. However, although the majority of predictions have been validated by various literature data (Table 1), all network-predicted repurposable drugs and drug combinations must be validated in various 2019-nCoV/SARS-CoV-2 experimental assays64 and randomized clinical trials before being used in patients.\nWe acknowledge several limitations in the current study. Although 2019-nCoV/SARS-CoV-2 shared high nucleotide sequence identity with other HCoVs (Fig. 2), our predictions are not 2019-nCoV/SARS-CoV-2 specific by lack of the known host proteins on 2019-nCoV/SARS-CoV-2. We used a low binding affinity value of 10 μM as a threshold to define a physical drug–target interaction. However, a stronger binding affinity threshold (e.g., 1 μM) may be a more suitable cut-off in drug discovery, although it will generate a smaller drug–target network. Although sizeable efforts were made for assembling large scale, experimentally reported drug–target networks from publicly available databases, the network data may be incomplete and some drug–target interactions may be functional associations, instead of physical bindings. For example, Silvestrol, a natural product from the flavagline, was found to have antiviral activity against Ebola75 and Coronaviruses76. After adding its target, an RNA helicase enzyme EIF4A76, silvestrol was predicted to be significantly associated with HCoVs (Z = –1.24, P = 0.041) by network proximity analysis. To increase coverage of drug–target networks, we may use computational approaches to systematically predict the drug-target interactions further25,26. In addition, the collected virus–host interactions are far from completeness and the quality can be influenced by multiple factors, including different experimental assays and human cell line models. We may computationally predict a new virus–host interactome for 2019-nCoV/SARS-CoV-2 using sequence-based and structure-based approaches77. Drug targets representing nodes within cellular networks are often intrinsically coupled with both therapeutic and adverse profiles78, as drugs can inhibit or activate protein functions (including antagonists vs. agonists). The current systems pharmacology model cannot separate therapeutic (antiviral) effects from those predictions due to lack of detailed pharmacological effects of drug targets and unknown functional consequences of virus–host interactions. Comprehensive identification of the virus–host interactome for 2019-nCoV/SARS-CoV-2, with specific biological effects using functional genomics assays79,80, will significantly improve the accuracy of the proposed network-based methodologies further.\nOwing to a lack of the complete drug-target information (such as the molecular “promiscuity” of drugs), the dose–response and dose–toxicity effects for both repurposable drugs and drug combinations cannot be identified in the current network models. For example, Mesalazine, an approved drug for inflammatory bowel disease, is a top network-predicted repurposable drug associated with HCoVs (Fig. 5a). Yet, several clinical studies showed the potential pulmonary toxicities (including pneumonia) associated with mesalazine usage81,82. Integration of lung-specific gene expression23 of 2019-nCoV/SARS-CoV-2 host proteins and physiologically based pharmacokinetic modeling83 may reduce side effects of repurposable drugs or drug combinations. Preclinical studies are warranted to evaluate in vivo efficiency and side effects before clinical trials. Furthermore, we only limited to predict pairwise drug combinations based on our previous network-based framework28. However, we expect that our methodology remain to be a useful network-based tool for prediction of combining multiple drugs toward exploring network relationships of multiple drugs’ targets with the HCoV–host subnetwork in the human interactome. Finally, we aimed to systematically identify repurposable drugs by specifically targeting nCoV host proteins only. Thus, our current network models cannot predict repurposable drugs from the existing anti-virus drugs that target virus proteins only. Thus, combination of the existing anti-virus drugs (such as remdesivir64) with the network-predicted repurposable drugs (Fig. 5) or drug combinations (Fig. 6) may improve coverage of current network-based methodologies by utilizing multi-layer network framework16.\nIn conclusion, this study offers a powerful, integrative network-based systems pharmacology methodology for rapid identification of repurposable drugs and drug combinations for the potential treatment of 2019-nCoV/SARS-CoV-2. Our approach can minimize the translational gap between preclinical testing results and clinical outcomes, which is a significant problem in the rapid development of efficient treatment strategies for the emerging 2019-nCoV/SARS-CoV-2 outbreak. From a translational perspective, if broadly applied, the network tools developed here could help develop effective treatment strategies for other emerging viral infections and other human complex diseases as well."}
LitCovid-PD-CLO
{"project":"LitCovid-PD-CLO","denotations":[{"id":"T238","span":{"begin":39,"end":40},"obj":"http://purl.obolibrary.org/obo/CLO_0001020"},{"id":"T239","span":{"begin":290,"end":306},"obj":"http://www.ebi.ac.uk/cellline#human_cell_line"},{"id":"T240","span":{"begin":312,"end":325},"obj":"http://purl.obolibrary.org/obo/PR_000029067"},{"id":"T241","span":{"begin":1141,"end":1142},"obj":"http://purl.obolibrary.org/obo/CLO_0001020"},{"id":"T242","span":{"begin":1182,"end":1183},"obj":"http://purl.obolibrary.org/obo/CLO_0001020"},{"id":"T243","span":{"begin":1204,"end":1205},"obj":"http://purl.obolibrary.org/obo/CLO_0001020"},{"id":"T244","span":{"begin":1249,"end":1250},"obj":"http://purl.obolibrary.org/obo/CLO_0001020"},{"id":"T245","span":{"begin":1307,"end":1308},"obj":"http://purl.obolibrary.org/obo/CLO_0001020"},{"id":"T246","span":{"begin":1376,"end":1377},"obj":"http://purl.obolibrary.org/obo/CLO_0001020"},{"id":"T247","span":{"begin":1707,"end":1708},"obj":"http://purl.obolibrary.org/obo/CLO_0001020"},{"id":"T248","span":{"begin":1774,"end":1782},"obj":"http://purl.obolibrary.org/obo/CLO_0001658"},{"id":"T249","span":{"begin":2176,"end":2181},"obj":"http://purl.obolibrary.org/obo/NCBITaxon_10239"},{"id":"T250","span":{"begin":2325,"end":2340},"obj":"http://www.ebi.ac.uk/cellline#human_cell_line"},{"id":"T251","span":{"begin":2380,"end":2381},"obj":"http://purl.obolibrary.org/obo/CLO_0001020"},{"id":"T252","span":{"begin":2386,"end":2391},"obj":"http://purl.obolibrary.org/obo/NCBITaxon_10239"},{"id":"T253","span":{"begin":2648,"end":2656},"obj":"http://purl.obolibrary.org/obo/CLO_0001658"},{"id":"T254","span":{"begin":2926,"end":2931},"obj":"http://purl.obolibrary.org/obo/NCBITaxon_10239"},{"id":"T255","span":{"begin":2987,"end":2992},"obj":"http://purl.obolibrary.org/obo/NCBITaxon_10239"},{"id":"T256","span":{"begin":3210,"end":3211},"obj":"http://purl.obolibrary.org/obo/CLO_0001020"},{"id":"T257","span":{"begin":3528,"end":3529},"obj":"http://purl.obolibrary.org/obo/CLO_0001020"},{"id":"T258","span":{"begin":3751,"end":3755},"obj":"http://purl.obolibrary.org/obo/UBERON_0002048"},{"id":"T259","span":{"begin":3751,"end":3755},"obj":"http://www.ebi.ac.uk/efo/EFO_0000934"},{"id":"T260","span":{"begin":3765,"end":3769},"obj":"http://purl.obolibrary.org/obo/OGG_0000000002"},{"id":"T261","span":{"begin":4217,"end":4218},"obj":"http://purl.obolibrary.org/obo/CLO_0001020"},{"id":"T262","span":{"begin":4391,"end":4396},"obj":"http://purl.obolibrary.org/obo/NCBITaxon_9606"},{"id":"T263","span":{"begin":4615,"end":4620},"obj":"http://purl.obolibrary.org/obo/NCBITaxon_10239"},{"id":"T264","span":{"begin":4639,"end":4644},"obj":"http://purl.obolibrary.org/obo/NCBITaxon_10239"},{"id":"T265","span":{"begin":4699,"end":4704},"obj":"http://purl.obolibrary.org/obo/NCBITaxon_10239"},{"id":"T266","span":{"begin":4958,"end":4959},"obj":"http://purl.obolibrary.org/obo/CLO_0001020"},{"id":"T267","span":{"begin":5219,"end":5226},"obj":"http://purl.obolibrary.org/obo/UBERON_0000473"},{"id":"T268","span":{"begin":5267,"end":5268},"obj":"http://purl.obolibrary.org/obo/CLO_0001020"},{"id":"T269","span":{"begin":5401,"end":5402},"obj":"http://purl.obolibrary.org/obo/CLO_0001020"},{"id":"T270","span":{"begin":5579,"end":5584},"obj":"http://purl.obolibrary.org/obo/NCBITaxon_9606"}],"text":"Discussion\nIn this study, we presented a network-based methodology for systematic identification of putative repurposable drugs and drug combinations for potential treatment of 2019-nCoV/SARS-CoV-2. Integration of drug–target networks, HCoV–host interactions, HCoV-induced transcriptome in human cell lines, and human protein–protein interactome network are essential for such identification. Based on comprehensive evaluation, we prioritized 16 candidate repurposable drugs (Fig. 5) and 3 potential drug combinations (Fig. 6) for targeting 2019-nCoV/SARS-CoV-2. However, although the majority of predictions have been validated by various literature data (Table 1), all network-predicted repurposable drugs and drug combinations must be validated in various 2019-nCoV/SARS-CoV-2 experimental assays64 and randomized clinical trials before being used in patients.\nWe acknowledge several limitations in the current study. Although 2019-nCoV/SARS-CoV-2 shared high nucleotide sequence identity with other HCoVs (Fig. 2), our predictions are not 2019-nCoV/SARS-CoV-2 specific by lack of the known host proteins on 2019-nCoV/SARS-CoV-2. We used a low binding affinity value of 10 μM as a threshold to define a physical drug–target interaction. However, a stronger binding affinity threshold (e.g., 1 μM) may be a more suitable cut-off in drug discovery, although it will generate a smaller drug–target network. Although sizeable efforts were made for assembling large scale, experimentally reported drug–target networks from publicly available databases, the network data may be incomplete and some drug–target interactions may be functional associations, instead of physical bindings. For example, Silvestrol, a natural product from the flavagline, was found to have antiviral activity against Ebola75 and Coronaviruses76. After adding its target, an RNA helicase enzyme EIF4A76, silvestrol was predicted to be significantly associated with HCoVs (Z = –1.24, P = 0.041) by network proximity analysis. To increase coverage of drug–target networks, we may use computational approaches to systematically predict the drug-target interactions further25,26. In addition, the collected virus–host interactions are far from completeness and the quality can be influenced by multiple factors, including different experimental assays and human cell line models. We may computationally predict a new virus–host interactome for 2019-nCoV/SARS-CoV-2 using sequence-based and structure-based approaches77. Drug targets representing nodes within cellular networks are often intrinsically coupled with both therapeutic and adverse profiles78, as drugs can inhibit or activate protein functions (including antagonists vs. agonists). The current systems pharmacology model cannot separate therapeutic (antiviral) effects from those predictions due to lack of detailed pharmacological effects of drug targets and unknown functional consequences of virus–host interactions. Comprehensive identification of the virus–host interactome for 2019-nCoV/SARS-CoV-2, with specific biological effects using functional genomics assays79,80, will significantly improve the accuracy of the proposed network-based methodologies further.\nOwing to a lack of the complete drug-target information (such as the molecular “promiscuity” of drugs), the dose–response and dose–toxicity effects for both repurposable drugs and drug combinations cannot be identified in the current network models. For example, Mesalazine, an approved drug for inflammatory bowel disease, is a top network-predicted repurposable drug associated with HCoVs (Fig. 5a). Yet, several clinical studies showed the potential pulmonary toxicities (including pneumonia) associated with mesalazine usage81,82. Integration of lung-specific gene expression23 of 2019-nCoV/SARS-CoV-2 host proteins and physiologically based pharmacokinetic modeling83 may reduce side effects of repurposable drugs or drug combinations. Preclinical studies are warranted to evaluate in vivo efficiency and side effects before clinical trials. Furthermore, we only limited to predict pairwise drug combinations based on our previous network-based framework28. However, we expect that our methodology remain to be a useful network-based tool for prediction of combining multiple drugs toward exploring network relationships of multiple drugs’ targets with the HCoV–host subnetwork in the human interactome. Finally, we aimed to systematically identify repurposable drugs by specifically targeting nCoV host proteins only. Thus, our current network models cannot predict repurposable drugs from the existing anti-virus drugs that target virus proteins only. Thus, combination of the existing anti-virus drugs (such as remdesivir64) with the network-predicted repurposable drugs (Fig. 5) or drug combinations (Fig. 6) may improve coverage of current network-based methodologies by utilizing multi-layer network framework16.\nIn conclusion, this study offers a powerful, integrative network-based systems pharmacology methodology for rapid identification of repurposable drugs and drug combinations for the potential treatment of 2019-nCoV/SARS-CoV-2. Our approach can minimize the translational gap between preclinical testing results and clinical outcomes, which is a significant problem in the rapid development of efficient treatment strategies for the emerging 2019-nCoV/SARS-CoV-2 outbreak. From a translational perspective, if broadly applied, the network tools developed here could help develop effective treatment strategies for other emerging viral infections and other human complex diseases as well."}
LitCovid-PD-CHEBI
{"project":"LitCovid-PD-CHEBI","denotations":[{"id":"T380","span":{"begin":122,"end":127},"obj":"Chemical"},{"id":"T381","span":{"begin":132,"end":136},"obj":"Chemical"},{"id":"T382","span":{"begin":214,"end":218},"obj":"Chemical"},{"id":"T383","span":{"begin":318,"end":325},"obj":"Chemical"},{"id":"T384","span":{"begin":326,"end":333},"obj":"Chemical"},{"id":"T385","span":{"begin":469,"end":474},"obj":"Chemical"},{"id":"T386","span":{"begin":500,"end":504},"obj":"Chemical"},{"id":"T387","span":{"begin":702,"end":707},"obj":"Chemical"},{"id":"T388","span":{"begin":712,"end":716},"obj":"Chemical"},{"id":"T389","span":{"begin":963,"end":973},"obj":"Chemical"},{"id":"T390","span":{"begin":1099,"end":1107},"obj":"Chemical"},{"id":"T391","span":{"begin":1215,"end":1219},"obj":"Chemical"},{"id":"T392","span":{"begin":1334,"end":1338},"obj":"Chemical"},{"id":"T393","span":{"begin":1386,"end":1390},"obj":"Chemical"},{"id":"T394","span":{"begin":1495,"end":1499},"obj":"Chemical"},{"id":"T395","span":{"begin":1595,"end":1599},"obj":"Chemical"},{"id":"T396","span":{"begin":1734,"end":1744},"obj":"Chemical"},{"id":"T397","span":{"begin":1764,"end":1773},"obj":"Chemical"},{"id":"T398","span":{"begin":1877,"end":1887},"obj":"Chemical"},{"id":"T399","span":{"begin":2022,"end":2026},"obj":"Chemical"},{"id":"T400","span":{"begin":2110,"end":2114},"obj":"Chemical"},{"id":"T401","span":{"begin":2627,"end":2632},"obj":"Chemical"},{"id":"T402","span":{"begin":2657,"end":2664},"obj":"Chemical"},{"id":"T403","span":{"begin":2686,"end":2697},"obj":"Chemical"},{"id":"T404","span":{"begin":2702,"end":2710},"obj":"Chemical"},{"id":"T405","span":{"begin":2781,"end":2790},"obj":"Chemical"},{"id":"T406","span":{"begin":2874,"end":2878},"obj":"Chemical"},{"id":"T407","span":{"begin":3233,"end":3237},"obj":"Chemical"},{"id":"T408","span":{"begin":3297,"end":3302},"obj":"Chemical"},{"id":"T409","span":{"begin":3371,"end":3376},"obj":"Chemical"},{"id":"T410","span":{"begin":3381,"end":3385},"obj":"Chemical"},{"id":"T411","span":{"begin":3464,"end":3474},"obj":"Chemical"},{"id":"T412","span":{"begin":3488,"end":3492},"obj":"Chemical"},{"id":"T413","span":{"begin":3565,"end":3569},"obj":"Chemical"},{"id":"T414","span":{"begin":3713,"end":3723},"obj":"Chemical"},{"id":"T415","span":{"begin":3812,"end":3820},"obj":"Chemical"},{"id":"T416","span":{"begin":3914,"end":3919},"obj":"Chemical"},{"id":"T417","span":{"begin":3923,"end":3927},"obj":"Chemical"},{"id":"T418","span":{"begin":4097,"end":4101},"obj":"Chemical"},{"id":"T419","span":{"begin":4282,"end":4287},"obj":"Chemical"},{"id":"T420","span":{"begin":4339,"end":4344},"obj":"Chemical"},{"id":"T421","span":{"begin":4468,"end":4473},"obj":"Chemical"},{"id":"T422","span":{"begin":4510,"end":4518},"obj":"Chemical"},{"id":"T423","span":{"begin":4586,"end":4591},"obj":"Chemical"},{"id":"T424","span":{"begin":4621,"end":4626},"obj":"Chemical"},{"id":"T425","span":{"begin":4645,"end":4653},"obj":"Chemical"},{"id":"T426","span":{"begin":4705,"end":4710},"obj":"Chemical"},{"id":"T427","span":{"begin":4774,"end":4779},"obj":"Chemical"},{"id":"T428","span":{"begin":4792,"end":4796},"obj":"Chemical"},{"id":"T429","span":{"begin":5070,"end":5075},"obj":"Chemical"},{"id":"T430","span":{"begin":5080,"end":5084},"obj":"Chemical"}],"attributes":[{"id":"A380","pred":"chebi_id","subj":"T380","obj":"http://purl.obolibrary.org/obo/CHEBI_23888"},{"id":"A381","pred":"chebi_id","subj":"T381","obj":"http://purl.obolibrary.org/obo/CHEBI_23888"},{"id":"A382","pred":"chebi_id","subj":"T382","obj":"http://purl.obolibrary.org/obo/CHEBI_23888"},{"id":"A383","pred":"chebi_id","subj":"T383","obj":"http://purl.obolibrary.org/obo/CHEBI_36080"},{"id":"A384","pred":"chebi_id","subj":"T384","obj":"http://purl.obolibrary.org/obo/CHEBI_36080"},{"id":"A385","pred":"chebi_id","subj":"T385","obj":"http://purl.obolibrary.org/obo/CHEBI_23888"},{"id":"A386","pred":"chebi_id","subj":"T386","obj":"http://purl.obolibrary.org/obo/CHEBI_23888"},{"id":"A387","pred":"chebi_id","subj":"T387","obj":"http://purl.obolibrary.org/obo/CHEBI_23888"},{"id":"A388","pred":"chebi_id","subj":"T388","obj":"http://purl.obolibrary.org/obo/CHEBI_23888"},{"id":"A389","pred":"chebi_id","subj":"T389","obj":"http://purl.obolibrary.org/obo/CHEBI_36976"},{"id":"A390","pred":"chebi_id","subj":"T390","obj":"http://purl.obolibrary.org/obo/CHEBI_36080"},{"id":"A391","pred":"chebi_id","subj":"T391","obj":"http://purl.obolibrary.org/obo/CHEBI_23888"},{"id":"A392","pred":"chebi_id","subj":"T392","obj":"http://purl.obolibrary.org/obo/CHEBI_23888"},{"id":"A393","pred":"chebi_id","subj":"T393","obj":"http://purl.obolibrary.org/obo/CHEBI_23888"},{"id":"A394","pred":"chebi_id","subj":"T394","obj":"http://purl.obolibrary.org/obo/CHEBI_23888"},{"id":"A395","pred":"chebi_id","subj":"T395","obj":"http://purl.obolibrary.org/obo/CHEBI_23888"},{"id":"A396","pred":"chebi_id","subj":"T396","obj":"http://purl.obolibrary.org/obo/CHEBI_71504"},{"id":"A397","pred":"chebi_id","subj":"T397","obj":"http://purl.obolibrary.org/obo/CHEBI_22587"},{"id":"A398","pred":"chebi_id","subj":"T398","obj":"http://purl.obolibrary.org/obo/CHEBI_66484"},{"id":"A399","pred":"chebi_id","subj":"T399","obj":"http://purl.obolibrary.org/obo/CHEBI_23888"},{"id":"A400","pred":"chebi_id","subj":"T400","obj":"http://purl.obolibrary.org/obo/CHEBI_23888"},{"id":"A401","pred":"chebi_id","subj":"T401","obj":"http://purl.obolibrary.org/obo/CHEBI_23888"},{"id":"A402","pred":"chebi_id","subj":"T402","obj":"http://purl.obolibrary.org/obo/CHEBI_36080"},{"id":"A403","pred":"chebi_id","subj":"T403","obj":"http://purl.obolibrary.org/obo/CHEBI_48706"},{"id":"A404","pred":"chebi_id","subj":"T404","obj":"http://purl.obolibrary.org/obo/CHEBI_48705"},{"id":"A405","pred":"chebi_id","subj":"T405","obj":"http://purl.obolibrary.org/obo/CHEBI_22587"},{"id":"A406","pred":"chebi_id","subj":"T406","obj":"http://purl.obolibrary.org/obo/CHEBI_23888"},{"id":"A407","pred":"chebi_id","subj":"T407","obj":"http://purl.obolibrary.org/obo/CHEBI_23888"},{"id":"A408","pred":"chebi_id","subj":"T408","obj":"http://purl.obolibrary.org/obo/CHEBI_23888"},{"id":"A409","pred":"chebi_id","subj":"T409","obj":"http://purl.obolibrary.org/obo/CHEBI_23888"},{"id":"A410","pred":"chebi_id","subj":"T410","obj":"http://purl.obolibrary.org/obo/CHEBI_23888"},{"id":"A411","pred":"chebi_id","subj":"T411","obj":"http://purl.obolibrary.org/obo/CHEBI_6775"},{"id":"A412","pred":"chebi_id","subj":"T412","obj":"http://purl.obolibrary.org/obo/CHEBI_23888"},{"id":"A413","pred":"chebi_id","subj":"T413","obj":"http://purl.obolibrary.org/obo/CHEBI_23888"},{"id":"A414","pred":"chebi_id","subj":"T414","obj":"http://purl.obolibrary.org/obo/CHEBI_6775"},{"id":"A415","pred":"chebi_id","subj":"T415","obj":"http://purl.obolibrary.org/obo/CHEBI_36080"},{"id":"A416","pred":"chebi_id","subj":"T416","obj":"http://purl.obolibrary.org/obo/CHEBI_23888"},{"id":"A417","pred":"chebi_id","subj":"T417","obj":"http://purl.obolibrary.org/obo/CHEBI_23888"},{"id":"A418","pred":"chebi_id","subj":"T418","obj":"http://purl.obolibrary.org/obo/CHEBI_23888"},{"id":"A419","pred":"chebi_id","subj":"T419","obj":"http://purl.obolibrary.org/obo/CHEBI_23888"},{"id":"A420","pred":"chebi_id","subj":"T420","obj":"http://purl.obolibrary.org/obo/CHEBI_23888"},{"id":"A421","pred":"chebi_id","subj":"T421","obj":"http://purl.obolibrary.org/obo/CHEBI_23888"},{"id":"A422","pred":"chebi_id","subj":"T422","obj":"http://purl.obolibrary.org/obo/CHEBI_36080"},{"id":"A423","pred":"chebi_id","subj":"T423","obj":"http://purl.obolibrary.org/obo/CHEBI_23888"},{"id":"A424","pred":"chebi_id","subj":"T424","obj":"http://purl.obolibrary.org/obo/CHEBI_23888"},{"id":"A425","pred":"chebi_id","subj":"T425","obj":"http://purl.obolibrary.org/obo/CHEBI_36080"},{"id":"A426","pred":"chebi_id","subj":"T426","obj":"http://purl.obolibrary.org/obo/CHEBI_23888"},{"id":"A427","pred":"chebi_id","subj":"T427","obj":"http://purl.obolibrary.org/obo/CHEBI_23888"},{"id":"A428","pred":"chebi_id","subj":"T428","obj":"http://purl.obolibrary.org/obo/CHEBI_23888"},{"id":"A429","pred":"chebi_id","subj":"T429","obj":"http://purl.obolibrary.org/obo/CHEBI_23888"},{"id":"A430","pred":"chebi_id","subj":"T430","obj":"http://purl.obolibrary.org/obo/CHEBI_23888"}],"text":"Discussion\nIn this study, we presented a network-based methodology for systematic identification of putative repurposable drugs and drug combinations for potential treatment of 2019-nCoV/SARS-CoV-2. Integration of drug–target networks, HCoV–host interactions, HCoV-induced transcriptome in human cell lines, and human protein–protein interactome network are essential for such identification. Based on comprehensive evaluation, we prioritized 16 candidate repurposable drugs (Fig. 5) and 3 potential drug combinations (Fig. 6) for targeting 2019-nCoV/SARS-CoV-2. However, although the majority of predictions have been validated by various literature data (Table 1), all network-predicted repurposable drugs and drug combinations must be validated in various 2019-nCoV/SARS-CoV-2 experimental assays64 and randomized clinical trials before being used in patients.\nWe acknowledge several limitations in the current study. Although 2019-nCoV/SARS-CoV-2 shared high nucleotide sequence identity with other HCoVs (Fig. 2), our predictions are not 2019-nCoV/SARS-CoV-2 specific by lack of the known host proteins on 2019-nCoV/SARS-CoV-2. We used a low binding affinity value of 10 μM as a threshold to define a physical drug–target interaction. However, a stronger binding affinity threshold (e.g., 1 μM) may be a more suitable cut-off in drug discovery, although it will generate a smaller drug–target network. Although sizeable efforts were made for assembling large scale, experimentally reported drug–target networks from publicly available databases, the network data may be incomplete and some drug–target interactions may be functional associations, instead of physical bindings. For example, Silvestrol, a natural product from the flavagline, was found to have antiviral activity against Ebola75 and Coronaviruses76. After adding its target, an RNA helicase enzyme EIF4A76, silvestrol was predicted to be significantly associated with HCoVs (Z = –1.24, P = 0.041) by network proximity analysis. To increase coverage of drug–target networks, we may use computational approaches to systematically predict the drug-target interactions further25,26. In addition, the collected virus–host interactions are far from completeness and the quality can be influenced by multiple factors, including different experimental assays and human cell line models. We may computationally predict a new virus–host interactome for 2019-nCoV/SARS-CoV-2 using sequence-based and structure-based approaches77. Drug targets representing nodes within cellular networks are often intrinsically coupled with both therapeutic and adverse profiles78, as drugs can inhibit or activate protein functions (including antagonists vs. agonists). The current systems pharmacology model cannot separate therapeutic (antiviral) effects from those predictions due to lack of detailed pharmacological effects of drug targets and unknown functional consequences of virus–host interactions. Comprehensive identification of the virus–host interactome for 2019-nCoV/SARS-CoV-2, with specific biological effects using functional genomics assays79,80, will significantly improve the accuracy of the proposed network-based methodologies further.\nOwing to a lack of the complete drug-target information (such as the molecular “promiscuity” of drugs), the dose–response and dose–toxicity effects for both repurposable drugs and drug combinations cannot be identified in the current network models. For example, Mesalazine, an approved drug for inflammatory bowel disease, is a top network-predicted repurposable drug associated with HCoVs (Fig. 5a). Yet, several clinical studies showed the potential pulmonary toxicities (including pneumonia) associated with mesalazine usage81,82. Integration of lung-specific gene expression23 of 2019-nCoV/SARS-CoV-2 host proteins and physiologically based pharmacokinetic modeling83 may reduce side effects of repurposable drugs or drug combinations. Preclinical studies are warranted to evaluate in vivo efficiency and side effects before clinical trials. Furthermore, we only limited to predict pairwise drug combinations based on our previous network-based framework28. However, we expect that our methodology remain to be a useful network-based tool for prediction of combining multiple drugs toward exploring network relationships of multiple drugs’ targets with the HCoV–host subnetwork in the human interactome. Finally, we aimed to systematically identify repurposable drugs by specifically targeting nCoV host proteins only. Thus, our current network models cannot predict repurposable drugs from the existing anti-virus drugs that target virus proteins only. Thus, combination of the existing anti-virus drugs (such as remdesivir64) with the network-predicted repurposable drugs (Fig. 5) or drug combinations (Fig. 6) may improve coverage of current network-based methodologies by utilizing multi-layer network framework16.\nIn conclusion, this study offers a powerful, integrative network-based systems pharmacology methodology for rapid identification of repurposable drugs and drug combinations for the potential treatment of 2019-nCoV/SARS-CoV-2. Our approach can minimize the translational gap between preclinical testing results and clinical outcomes, which is a significant problem in the rapid development of efficient treatment strategies for the emerging 2019-nCoV/SARS-CoV-2 outbreak. From a translational perspective, if broadly applied, the network tools developed here could help develop effective treatment strategies for other emerging viral infections and other human complex diseases as well."}
LitCovid-PD-GO-BP
{"project":"LitCovid-PD-GO-BP","denotations":[{"id":"T55","span":{"begin":2176,"end":2199},"obj":"http://purl.obolibrary.org/obo/GO_0019048"},{"id":"T56","span":{"begin":2926,"end":2949},"obj":"http://purl.obolibrary.org/obo/GO_0019048"},{"id":"T57","span":{"begin":5181,"end":5194},"obj":"http://purl.obolibrary.org/obo/GO_0006412"},{"id":"T58","span":{"begin":5403,"end":5416},"obj":"http://purl.obolibrary.org/obo/GO_0006412"},{"id":"T59","span":{"begin":5552,"end":5568},"obj":"http://purl.obolibrary.org/obo/GO_0016032"}],"text":"Discussion\nIn this study, we presented a network-based methodology for systematic identification of putative repurposable drugs and drug combinations for potential treatment of 2019-nCoV/SARS-CoV-2. Integration of drug–target networks, HCoV–host interactions, HCoV-induced transcriptome in human cell lines, and human protein–protein interactome network are essential for such identification. Based on comprehensive evaluation, we prioritized 16 candidate repurposable drugs (Fig. 5) and 3 potential drug combinations (Fig. 6) for targeting 2019-nCoV/SARS-CoV-2. However, although the majority of predictions have been validated by various literature data (Table 1), all network-predicted repurposable drugs and drug combinations must be validated in various 2019-nCoV/SARS-CoV-2 experimental assays64 and randomized clinical trials before being used in patients.\nWe acknowledge several limitations in the current study. Although 2019-nCoV/SARS-CoV-2 shared high nucleotide sequence identity with other HCoVs (Fig. 2), our predictions are not 2019-nCoV/SARS-CoV-2 specific by lack of the known host proteins on 2019-nCoV/SARS-CoV-2. We used a low binding affinity value of 10 μM as a threshold to define a physical drug–target interaction. However, a stronger binding affinity threshold (e.g., 1 μM) may be a more suitable cut-off in drug discovery, although it will generate a smaller drug–target network. Although sizeable efforts were made for assembling large scale, experimentally reported drug–target networks from publicly available databases, the network data may be incomplete and some drug–target interactions may be functional associations, instead of physical bindings. For example, Silvestrol, a natural product from the flavagline, was found to have antiviral activity against Ebola75 and Coronaviruses76. After adding its target, an RNA helicase enzyme EIF4A76, silvestrol was predicted to be significantly associated with HCoVs (Z = –1.24, P = 0.041) by network proximity analysis. To increase coverage of drug–target networks, we may use computational approaches to systematically predict the drug-target interactions further25,26. In addition, the collected virus–host interactions are far from completeness and the quality can be influenced by multiple factors, including different experimental assays and human cell line models. We may computationally predict a new virus–host interactome for 2019-nCoV/SARS-CoV-2 using sequence-based and structure-based approaches77. Drug targets representing nodes within cellular networks are often intrinsically coupled with both therapeutic and adverse profiles78, as drugs can inhibit or activate protein functions (including antagonists vs. agonists). The current systems pharmacology model cannot separate therapeutic (antiviral) effects from those predictions due to lack of detailed pharmacological effects of drug targets and unknown functional consequences of virus–host interactions. Comprehensive identification of the virus–host interactome for 2019-nCoV/SARS-CoV-2, with specific biological effects using functional genomics assays79,80, will significantly improve the accuracy of the proposed network-based methodologies further.\nOwing to a lack of the complete drug-target information (such as the molecular “promiscuity” of drugs), the dose–response and dose–toxicity effects for both repurposable drugs and drug combinations cannot be identified in the current network models. For example, Mesalazine, an approved drug for inflammatory bowel disease, is a top network-predicted repurposable drug associated with HCoVs (Fig. 5a). Yet, several clinical studies showed the potential pulmonary toxicities (including pneumonia) associated with mesalazine usage81,82. Integration of lung-specific gene expression23 of 2019-nCoV/SARS-CoV-2 host proteins and physiologically based pharmacokinetic modeling83 may reduce side effects of repurposable drugs or drug combinations. Preclinical studies are warranted to evaluate in vivo efficiency and side effects before clinical trials. Furthermore, we only limited to predict pairwise drug combinations based on our previous network-based framework28. However, we expect that our methodology remain to be a useful network-based tool for prediction of combining multiple drugs toward exploring network relationships of multiple drugs’ targets with the HCoV–host subnetwork in the human interactome. Finally, we aimed to systematically identify repurposable drugs by specifically targeting nCoV host proteins only. Thus, our current network models cannot predict repurposable drugs from the existing anti-virus drugs that target virus proteins only. Thus, combination of the existing anti-virus drugs (such as remdesivir64) with the network-predicted repurposable drugs (Fig. 5) or drug combinations (Fig. 6) may improve coverage of current network-based methodologies by utilizing multi-layer network framework16.\nIn conclusion, this study offers a powerful, integrative network-based systems pharmacology methodology for rapid identification of repurposable drugs and drug combinations for the potential treatment of 2019-nCoV/SARS-CoV-2. Our approach can minimize the translational gap between preclinical testing results and clinical outcomes, which is a significant problem in the rapid development of efficient treatment strategies for the emerging 2019-nCoV/SARS-CoV-2 outbreak. From a translational perspective, if broadly applied, the network tools developed here could help develop effective treatment strategies for other emerging viral infections and other human complex diseases as well."}
LitCovid-PD-HP
{"project":"LitCovid-PD-HP","denotations":[{"id":"T25","span":{"begin":3497,"end":3523},"obj":"Phenotype"},{"id":"T26","span":{"begin":3686,"end":3695},"obj":"Phenotype"}],"attributes":[{"id":"A25","pred":"hp_id","subj":"T25","obj":"http://purl.obolibrary.org/obo/HP_0002037"},{"id":"A26","pred":"hp_id","subj":"T26","obj":"http://purl.obolibrary.org/obo/HP_0002090"}],"text":"Discussion\nIn this study, we presented a network-based methodology for systematic identification of putative repurposable drugs and drug combinations for potential treatment of 2019-nCoV/SARS-CoV-2. Integration of drug–target networks, HCoV–host interactions, HCoV-induced transcriptome in human cell lines, and human protein–protein interactome network are essential for such identification. Based on comprehensive evaluation, we prioritized 16 candidate repurposable drugs (Fig. 5) and 3 potential drug combinations (Fig. 6) for targeting 2019-nCoV/SARS-CoV-2. However, although the majority of predictions have been validated by various literature data (Table 1), all network-predicted repurposable drugs and drug combinations must be validated in various 2019-nCoV/SARS-CoV-2 experimental assays64 and randomized clinical trials before being used in patients.\nWe acknowledge several limitations in the current study. Although 2019-nCoV/SARS-CoV-2 shared high nucleotide sequence identity with other HCoVs (Fig. 2), our predictions are not 2019-nCoV/SARS-CoV-2 specific by lack of the known host proteins on 2019-nCoV/SARS-CoV-2. We used a low binding affinity value of 10 μM as a threshold to define a physical drug–target interaction. However, a stronger binding affinity threshold (e.g., 1 μM) may be a more suitable cut-off in drug discovery, although it will generate a smaller drug–target network. Although sizeable efforts were made for assembling large scale, experimentally reported drug–target networks from publicly available databases, the network data may be incomplete and some drug–target interactions may be functional associations, instead of physical bindings. For example, Silvestrol, a natural product from the flavagline, was found to have antiviral activity against Ebola75 and Coronaviruses76. After adding its target, an RNA helicase enzyme EIF4A76, silvestrol was predicted to be significantly associated with HCoVs (Z = –1.24, P = 0.041) by network proximity analysis. To increase coverage of drug–target networks, we may use computational approaches to systematically predict the drug-target interactions further25,26. In addition, the collected virus–host interactions are far from completeness and the quality can be influenced by multiple factors, including different experimental assays and human cell line models. We may computationally predict a new virus–host interactome for 2019-nCoV/SARS-CoV-2 using sequence-based and structure-based approaches77. Drug targets representing nodes within cellular networks are often intrinsically coupled with both therapeutic and adverse profiles78, as drugs can inhibit or activate protein functions (including antagonists vs. agonists). The current systems pharmacology model cannot separate therapeutic (antiviral) effects from those predictions due to lack of detailed pharmacological effects of drug targets and unknown functional consequences of virus–host interactions. Comprehensive identification of the virus–host interactome for 2019-nCoV/SARS-CoV-2, with specific biological effects using functional genomics assays79,80, will significantly improve the accuracy of the proposed network-based methodologies further.\nOwing to a lack of the complete drug-target information (such as the molecular “promiscuity” of drugs), the dose–response and dose–toxicity effects for both repurposable drugs and drug combinations cannot be identified in the current network models. For example, Mesalazine, an approved drug for inflammatory bowel disease, is a top network-predicted repurposable drug associated with HCoVs (Fig. 5a). Yet, several clinical studies showed the potential pulmonary toxicities (including pneumonia) associated with mesalazine usage81,82. Integration of lung-specific gene expression23 of 2019-nCoV/SARS-CoV-2 host proteins and physiologically based pharmacokinetic modeling83 may reduce side effects of repurposable drugs or drug combinations. Preclinical studies are warranted to evaluate in vivo efficiency and side effects before clinical trials. Furthermore, we only limited to predict pairwise drug combinations based on our previous network-based framework28. However, we expect that our methodology remain to be a useful network-based tool for prediction of combining multiple drugs toward exploring network relationships of multiple drugs’ targets with the HCoV–host subnetwork in the human interactome. Finally, we aimed to systematically identify repurposable drugs by specifically targeting nCoV host proteins only. Thus, our current network models cannot predict repurposable drugs from the existing anti-virus drugs that target virus proteins only. Thus, combination of the existing anti-virus drugs (such as remdesivir64) with the network-predicted repurposable drugs (Fig. 5) or drug combinations (Fig. 6) may improve coverage of current network-based methodologies by utilizing multi-layer network framework16.\nIn conclusion, this study offers a powerful, integrative network-based systems pharmacology methodology for rapid identification of repurposable drugs and drug combinations for the potential treatment of 2019-nCoV/SARS-CoV-2. Our approach can minimize the translational gap between preclinical testing results and clinical outcomes, which is a significant problem in the rapid development of efficient treatment strategies for the emerging 2019-nCoV/SARS-CoV-2 outbreak. From a translational perspective, if broadly applied, the network tools developed here could help develop effective treatment strategies for other emerging viral infections and other human complex diseases as well."}
LitCovid-sentences
{"project":"LitCovid-sentences","denotations":[{"id":"T203","span":{"begin":0,"end":10},"obj":"Sentence"},{"id":"T204","span":{"begin":11,"end":198},"obj":"Sentence"},{"id":"T205","span":{"begin":199,"end":392},"obj":"Sentence"},{"id":"T206","span":{"begin":393,"end":562},"obj":"Sentence"},{"id":"T207","span":{"begin":563,"end":863},"obj":"Sentence"},{"id":"T208","span":{"begin":864,"end":920},"obj":"Sentence"},{"id":"T209","span":{"begin":921,"end":1132},"obj":"Sentence"},{"id":"T210","span":{"begin":1133,"end":1239},"obj":"Sentence"},{"id":"T211","span":{"begin":1240,"end":1406},"obj":"Sentence"},{"id":"T212","span":{"begin":1407,"end":1681},"obj":"Sentence"},{"id":"T213","span":{"begin":1682,"end":1819},"obj":"Sentence"},{"id":"T214","span":{"begin":1820,"end":1997},"obj":"Sentence"},{"id":"T215","span":{"begin":1998,"end":2148},"obj":"Sentence"},{"id":"T216","span":{"begin":2149,"end":2348},"obj":"Sentence"},{"id":"T217","span":{"begin":2349,"end":2488},"obj":"Sentence"},{"id":"T218","span":{"begin":2489,"end":2712},"obj":"Sentence"},{"id":"T219","span":{"begin":2713,"end":2950},"obj":"Sentence"},{"id":"T220","span":{"begin":2951,"end":3200},"obj":"Sentence"},{"id":"T221","span":{"begin":3201,"end":3450},"obj":"Sentence"},{"id":"T222","span":{"begin":3451,"end":3602},"obj":"Sentence"},{"id":"T223","span":{"begin":3603,"end":3735},"obj":"Sentence"},{"id":"T224","span":{"begin":3736,"end":3941},"obj":"Sentence"},{"id":"T225","span":{"begin":3942,"end":4047},"obj":"Sentence"},{"id":"T226","span":{"begin":4048,"end":4163},"obj":"Sentence"},{"id":"T227","span":{"begin":4164,"end":4409},"obj":"Sentence"},{"id":"T228","span":{"begin":4410,"end":4524},"obj":"Sentence"},{"id":"T229","span":{"begin":4525,"end":4659},"obj":"Sentence"},{"id":"T230","span":{"begin":4660,"end":4924},"obj":"Sentence"},{"id":"T231","span":{"begin":4925,"end":5150},"obj":"Sentence"},{"id":"T232","span":{"begin":5151,"end":5395},"obj":"Sentence"},{"id":"T233","span":{"begin":5396,"end":5610},"obj":"Sentence"}],"namespaces":[{"prefix":"_base","uri":"http://pubannotation.org/ontology/tao.owl#"}],"text":"Discussion\nIn this study, we presented a network-based methodology for systematic identification of putative repurposable drugs and drug combinations for potential treatment of 2019-nCoV/SARS-CoV-2. Integration of drug–target networks, HCoV–host interactions, HCoV-induced transcriptome in human cell lines, and human protein–protein interactome network are essential for such identification. Based on comprehensive evaluation, we prioritized 16 candidate repurposable drugs (Fig. 5) and 3 potential drug combinations (Fig. 6) for targeting 2019-nCoV/SARS-CoV-2. However, although the majority of predictions have been validated by various literature data (Table 1), all network-predicted repurposable drugs and drug combinations must be validated in various 2019-nCoV/SARS-CoV-2 experimental assays64 and randomized clinical trials before being used in patients.\nWe acknowledge several limitations in the current study. Although 2019-nCoV/SARS-CoV-2 shared high nucleotide sequence identity with other HCoVs (Fig. 2), our predictions are not 2019-nCoV/SARS-CoV-2 specific by lack of the known host proteins on 2019-nCoV/SARS-CoV-2. We used a low binding affinity value of 10 μM as a threshold to define a physical drug–target interaction. However, a stronger binding affinity threshold (e.g., 1 μM) may be a more suitable cut-off in drug discovery, although it will generate a smaller drug–target network. Although sizeable efforts were made for assembling large scale, experimentally reported drug–target networks from publicly available databases, the network data may be incomplete and some drug–target interactions may be functional associations, instead of physical bindings. For example, Silvestrol, a natural product from the flavagline, was found to have antiviral activity against Ebola75 and Coronaviruses76. After adding its target, an RNA helicase enzyme EIF4A76, silvestrol was predicted to be significantly associated with HCoVs (Z = –1.24, P = 0.041) by network proximity analysis. To increase coverage of drug–target networks, we may use computational approaches to systematically predict the drug-target interactions further25,26. In addition, the collected virus–host interactions are far from completeness and the quality can be influenced by multiple factors, including different experimental assays and human cell line models. We may computationally predict a new virus–host interactome for 2019-nCoV/SARS-CoV-2 using sequence-based and structure-based approaches77. Drug targets representing nodes within cellular networks are often intrinsically coupled with both therapeutic and adverse profiles78, as drugs can inhibit or activate protein functions (including antagonists vs. agonists). The current systems pharmacology model cannot separate therapeutic (antiviral) effects from those predictions due to lack of detailed pharmacological effects of drug targets and unknown functional consequences of virus–host interactions. Comprehensive identification of the virus–host interactome for 2019-nCoV/SARS-CoV-2, with specific biological effects using functional genomics assays79,80, will significantly improve the accuracy of the proposed network-based methodologies further.\nOwing to a lack of the complete drug-target information (such as the molecular “promiscuity” of drugs), the dose–response and dose–toxicity effects for both repurposable drugs and drug combinations cannot be identified in the current network models. For example, Mesalazine, an approved drug for inflammatory bowel disease, is a top network-predicted repurposable drug associated with HCoVs (Fig. 5a). Yet, several clinical studies showed the potential pulmonary toxicities (including pneumonia) associated with mesalazine usage81,82. Integration of lung-specific gene expression23 of 2019-nCoV/SARS-CoV-2 host proteins and physiologically based pharmacokinetic modeling83 may reduce side effects of repurposable drugs or drug combinations. Preclinical studies are warranted to evaluate in vivo efficiency and side effects before clinical trials. Furthermore, we only limited to predict pairwise drug combinations based on our previous network-based framework28. However, we expect that our methodology remain to be a useful network-based tool for prediction of combining multiple drugs toward exploring network relationships of multiple drugs’ targets with the HCoV–host subnetwork in the human interactome. Finally, we aimed to systematically identify repurposable drugs by specifically targeting nCoV host proteins only. Thus, our current network models cannot predict repurposable drugs from the existing anti-virus drugs that target virus proteins only. Thus, combination of the existing anti-virus drugs (such as remdesivir64) with the network-predicted repurposable drugs (Fig. 5) or drug combinations (Fig. 6) may improve coverage of current network-based methodologies by utilizing multi-layer network framework16.\nIn conclusion, this study offers a powerful, integrative network-based systems pharmacology methodology for rapid identification of repurposable drugs and drug combinations for the potential treatment of 2019-nCoV/SARS-CoV-2. Our approach can minimize the translational gap between preclinical testing results and clinical outcomes, which is a significant problem in the rapid development of efficient treatment strategies for the emerging 2019-nCoV/SARS-CoV-2 outbreak. From a translational perspective, if broadly applied, the network tools developed here could help develop effective treatment strategies for other emerging viral infections and other human complex diseases as well."}
2_test
{"project":"2_test","denotations":[{"id":"32194980-32020029-19614235","span":{"begin":799,"end":801},"obj":"32020029"},{"id":"32194980-27864075-19614236","span":{"begin":1796,"end":1798},"obj":"27864075"},{"id":"32194980-29258862-19614237","span":{"begin":1816,"end":1818},"obj":"29258862"},{"id":"32194980-29258862-19614238","span":{"begin":1873,"end":1875},"obj":"29258862"},{"id":"32194980-29028879-19614239","span":{"begin":2485,"end":2487},"obj":"29028879"},{"id":"32194980-27146293-19614240","span":{"begin":2620,"end":2622},"obj":"27146293"},{"id":"32194980-24852294-19614241","span":{"begin":3101,"end":3103},"obj":"24852294"},{"id":"32194980-23667838-19614242","span":{"begin":3729,"end":3731},"obj":"23667838"},{"id":"32194980-28458997-19614243","span":{"begin":3732,"end":3734},"obj":"28458997"},{"id":"32194980-31375661-19614244","span":{"begin":3780,"end":3782},"obj":"31375661"},{"id":"32194980-29052226-19614245","span":{"begin":3871,"end":3873},"obj":"29052226"},{"id":"32194980-30867426-19614246","span":{"begin":4160,"end":4162},"obj":"30867426"},{"id":"32194980-32020029-19614247","span":{"begin":4730,"end":4732},"obj":"32020029"}],"text":"Discussion\nIn this study, we presented a network-based methodology for systematic identification of putative repurposable drugs and drug combinations for potential treatment of 2019-nCoV/SARS-CoV-2. Integration of drug–target networks, HCoV–host interactions, HCoV-induced transcriptome in human cell lines, and human protein–protein interactome network are essential for such identification. Based on comprehensive evaluation, we prioritized 16 candidate repurposable drugs (Fig. 5) and 3 potential drug combinations (Fig. 6) for targeting 2019-nCoV/SARS-CoV-2. However, although the majority of predictions have been validated by various literature data (Table 1), all network-predicted repurposable drugs and drug combinations must be validated in various 2019-nCoV/SARS-CoV-2 experimental assays64 and randomized clinical trials before being used in patients.\nWe acknowledge several limitations in the current study. Although 2019-nCoV/SARS-CoV-2 shared high nucleotide sequence identity with other HCoVs (Fig. 2), our predictions are not 2019-nCoV/SARS-CoV-2 specific by lack of the known host proteins on 2019-nCoV/SARS-CoV-2. We used a low binding affinity value of 10 μM as a threshold to define a physical drug–target interaction. However, a stronger binding affinity threshold (e.g., 1 μM) may be a more suitable cut-off in drug discovery, although it will generate a smaller drug–target network. Although sizeable efforts were made for assembling large scale, experimentally reported drug–target networks from publicly available databases, the network data may be incomplete and some drug–target interactions may be functional associations, instead of physical bindings. For example, Silvestrol, a natural product from the flavagline, was found to have antiviral activity against Ebola75 and Coronaviruses76. After adding its target, an RNA helicase enzyme EIF4A76, silvestrol was predicted to be significantly associated with HCoVs (Z = –1.24, P = 0.041) by network proximity analysis. To increase coverage of drug–target networks, we may use computational approaches to systematically predict the drug-target interactions further25,26. In addition, the collected virus–host interactions are far from completeness and the quality can be influenced by multiple factors, including different experimental assays and human cell line models. We may computationally predict a new virus–host interactome for 2019-nCoV/SARS-CoV-2 using sequence-based and structure-based approaches77. Drug targets representing nodes within cellular networks are often intrinsically coupled with both therapeutic and adverse profiles78, as drugs can inhibit or activate protein functions (including antagonists vs. agonists). The current systems pharmacology model cannot separate therapeutic (antiviral) effects from those predictions due to lack of detailed pharmacological effects of drug targets and unknown functional consequences of virus–host interactions. Comprehensive identification of the virus–host interactome for 2019-nCoV/SARS-CoV-2, with specific biological effects using functional genomics assays79,80, will significantly improve the accuracy of the proposed network-based methodologies further.\nOwing to a lack of the complete drug-target information (such as the molecular “promiscuity” of drugs), the dose–response and dose–toxicity effects for both repurposable drugs and drug combinations cannot be identified in the current network models. For example, Mesalazine, an approved drug for inflammatory bowel disease, is a top network-predicted repurposable drug associated with HCoVs (Fig. 5a). Yet, several clinical studies showed the potential pulmonary toxicities (including pneumonia) associated with mesalazine usage81,82. Integration of lung-specific gene expression23 of 2019-nCoV/SARS-CoV-2 host proteins and physiologically based pharmacokinetic modeling83 may reduce side effects of repurposable drugs or drug combinations. Preclinical studies are warranted to evaluate in vivo efficiency and side effects before clinical trials. Furthermore, we only limited to predict pairwise drug combinations based on our previous network-based framework28. However, we expect that our methodology remain to be a useful network-based tool for prediction of combining multiple drugs toward exploring network relationships of multiple drugs’ targets with the HCoV–host subnetwork in the human interactome. Finally, we aimed to systematically identify repurposable drugs by specifically targeting nCoV host proteins only. Thus, our current network models cannot predict repurposable drugs from the existing anti-virus drugs that target virus proteins only. Thus, combination of the existing anti-virus drugs (such as remdesivir64) with the network-predicted repurposable drugs (Fig. 5) or drug combinations (Fig. 6) may improve coverage of current network-based methodologies by utilizing multi-layer network framework16.\nIn conclusion, this study offers a powerful, integrative network-based systems pharmacology methodology for rapid identification of repurposable drugs and drug combinations for the potential treatment of 2019-nCoV/SARS-CoV-2. Our approach can minimize the translational gap between preclinical testing results and clinical outcomes, which is a significant problem in the rapid development of efficient treatment strategies for the emerging 2019-nCoV/SARS-CoV-2 outbreak. From a translational perspective, if broadly applied, the network tools developed here could help develop effective treatment strategies for other emerging viral infections and other human complex diseases as well."}