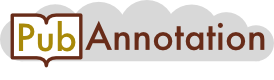
PMC:7033348 / 7542-8650
Annnotations
LitCovid-PD-MONDO
Id | Subject | Object | Predicate | Lexical cue | mondo_id |
---|---|---|---|---|---|
T10 | 189-199 | Disease | denotes | infectious | http://purl.obolibrary.org/obo/MONDO_0005550 |
T11 | 220-224 | Disease | denotes | SARS | http://purl.obolibrary.org/obo/MONDO_0005091 |
T12 | 226-231 | Disease | denotes | Ebola | http://purl.obolibrary.org/obo/MONDO_0005737 |
T13 | 242-251 | Disease | denotes | influenza | http://purl.obolibrary.org/obo/MONDO_0005812 |
T14 | 257-263 | Disease | denotes | dengue | http://purl.obolibrary.org/obo/MONDO_0005502 |
T15 | 851-855 | Disease | denotes | SARS | http://purl.obolibrary.org/obo/MONDO_0005091 |
LitCovid-PD-CLO
Id | Subject | Object | Predicate | Lexical cue |
---|---|---|---|---|
T38 | 155-156 | http://purl.obolibrary.org/obo/CLO_0001020 | denotes | a |
T39 | 309-313 | http://purl.obolibrary.org/obo/CLO_0001185 | denotes | 2018 |
T40 | 477-478 | http://purl.obolibrary.org/obo/CLO_0001020 | denotes | a |
T41 | 580-581 | http://purl.obolibrary.org/obo/CLO_0001020 | denotes | a |
T42 | 601-602 | http://purl.obolibrary.org/obo/CLO_0001020 | denotes | a |
T43 | 729-730 | http://purl.obolibrary.org/obo/CLO_0001020 | denotes | a |
T44 | 1032-1033 | http://purl.obolibrary.org/obo/CLO_0001020 | denotes | A |
LitCovid-PD-GO-BP
Id | Subject | Object | Predicate | Lexical cue |
---|---|---|---|---|
T5 | 365-371 | http://purl.obolibrary.org/obo/GO_0040007 | denotes | growth |
T6 | 412-418 | http://purl.obolibrary.org/obo/GO_0040007 | denotes | growth |
T7 | 456-462 | http://purl.obolibrary.org/obo/GO_0040007 | denotes | growth |
T8 | 490-496 | http://purl.obolibrary.org/obo/GO_0040007 | denotes | growth |
LitCovid-sentences
Id | Subject | Object | Predicate | Lexical cue |
---|---|---|---|---|
T44 | 0-6 | Sentence | denotes | Models |
T45 | 7-339 | Sentence | denotes | We generate short-term forecasts in real-time using three phenomenological models that have been previously used to derive short-term forecasts for a number of epidemics for several infectious diseases, including SARS, Ebola, pandemic influenza, and dengue (Chowell, Tariq, & Hyman, 2019; Pell et al., 2018; Wang, Wu, & Yang, 2012). |
T46 | 340-546 | Sentence | denotes | The generalized logistic growth model (GLM) extends the simple logistic growth model to accommodate sub-exponential growth dynamics with a scaling of growth parameter, p (Viboud, Simonsen, & Chowell, 2016). |
T47 | 547-712 | Sentence | denotes | The Richards model also includes a scaling parameter, a, to allow for deviation from the symmetric logistic curve (Chowell, 2017; Richards, 1959; Wang et al., 2012). |
T48 | 713-893 | Sentence | denotes | We also include a recently developed sub-epidemic wave model that supports complex epidemic trajectories, including multiple peaks (i.e., SARS in Singapore (Chowell et al., 2019)). |
T49 | 894-1031 | Sentence | denotes | In this approach, the observed reported curve is assumed to be the aggregate of multiple underlying sub-epidemics (Chowell et al., 2019). |
T50 | 1032-1108 | Sentence | denotes | A detailed description for each of the models is included in the Supplement. |
LitCovid-PubTator
Id | Subject | Object | Predicate | Lexical cue | tao:has_database_id |
---|---|---|---|---|---|
27 | 189-208 | Disease | denotes | infectious diseases | MESH:D003141 |
28 | 220-224 | Disease | denotes | SARS | MESH:D045169 |
29 | 851-855 | Disease | denotes | SARS | MESH:D045169 |
2_test
Id | Subject | Object | Predicate | Lexical cue |
---|---|---|---|---|
32110742-27913131-47437629 | 309-313 | 27913131 | denotes | 2018 |
32110742-22889641-47437630 | 333-337 | 22889641 | denotes | 2012 |
32110742-27266847-47437631 | 540-544 | 27266847 | denotes | 2016 |
32110742-22889641-47437632 | 706-710 | 22889641 | denotes | 2012 |
T48089 | 309-313 | 27913131 | denotes | 2018 |
T63529 | 333-337 | 22889641 | denotes | 2012 |
T61091 | 540-544 | 27266847 | denotes | 2016 |
T25904 | 706-710 | 22889641 | denotes | 2012 |