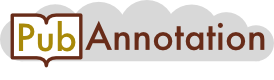
PMC:5118429 / 2380-6013
Annnotations
2_test
{"project":"2_test","denotations":[{"id":"27920797-26472703-33226412","span":{"begin":370,"end":374},"obj":"26472703"},{"id":"27920797-22178851-33226413","span":{"begin":519,"end":523},"obj":"22178851"},{"id":"27920797-17709780-33226414","span":{"begin":777,"end":781},"obj":"17709780"},{"id":"27920797-23115522-33226415","span":{"begin":1063,"end":1067},"obj":"23115522"},{"id":"27920797-26194229-33226416","span":{"begin":1335,"end":1339},"obj":"26194229"},{"id":"27920797-26499328-33226417","span":{"begin":1523,"end":1527},"obj":"26499328"},{"id":"27920797-25757012-33226418","span":{"begin":1547,"end":1551},"obj":"25757012"},{"id":"27920797-26360524-33226419","span":{"begin":1592,"end":1596},"obj":"26360524"},{"id":"27920797-25888203-33226422","span":{"begin":1671,"end":1675},"obj":"25888203"},{"id":"27920797-19700728-33226423","span":{"begin":1878,"end":1882},"obj":"19700728"},{"id":"27920797-20105297-33226424","span":{"begin":1906,"end":1910},"obj":"20105297"},{"id":"27920797-11290733-33226425","span":{"begin":2257,"end":2261},"obj":"11290733"},{"id":"27920797-22624567-33226426","span":{"begin":2855,"end":2859},"obj":"22624567"},{"id":"27920797-24755649-33226428","span":{"begin":3186,"end":3190},"obj":"24755649"}],"text":"Introduction\nFillet yield (FY, %), the ratio between the separable muscle of the fish and the harvest weight, is an economically important trait in rainbow trout aquaculture that reflects production efficiency with a consequent large impact on the returns (Kause et al., 2002). On a per-unit basis, the fillet price is three times that of the whole fish (Bugeon et al., 2010) and a recent global survey ranked FY among the six most important traits for genetic improvement in rainbow trout aquaculture (Sae-Lim et al., 2012). Despite that, FY has not received much attention in breeding programs because it is a lethally-measured trait, costly to measure, and difficult to select on, thus limiting the genetic progress in traditional selective breeding programs (Kause et al., 2007).\nTo overcome these phenotyping constraints and to increase the accuracy of selection, the use of high-density single nucleotide polymorphism (SNP) genotyping platforms and novel statistical models can identify SNPs associated with the trait for use in genomic selection (Dekkers, 2012). Several genome-wide association studies (GWAS) that match genetic variants, with or without pedigree records, with the observed phenotype have identified significant loci associated with economically important traits in livestock (Kadarmideen, 2014; Sharma et al., 2015). The aquaculture research community is following this trend, and interesting examples are available for growth and disease resistance traits in Atlantic Salmon (e.g., Correa et al., 2015; Gutierrez et al., 2015), rainbow trout (e.g., Kocmarek et al., 2015; Liu et al., 2015b; Palti et al., 2015b), and catfish (e.g., Geng et al., 2015).\nSingle-step genomic best linear unbiased prediction (ssGBLUP) and Bayesian variable selection methods are available for genomic predictions using dense SNP arrays. The ssGBLUP method (Misztal et al., 2009; Christensen and Lund, 2010) jointly incorporates all available genotypic, phenotypic, and pedigree data in the genomic predictions, but the method's assumption of an infinitesimal model is violated for traits affected by major genes. In contrast, Bayesian variable selection methods assume a prior distribution of the variance associated with each locus (Meuwissen et al., 2001), but are limited in that only data from genotyped animals are used in the analyses. Despite the limitations on data, the Bayesian variable selection method has resulted in greater accuracy of predicted genetic merit compared to ssGBLUP for traits known to have QTL segregating with moderate or large effect like bacterial cold water disease resistance in rainbow trout (Vallejo et al., submitted manuscript). The ssGBLUP method has been extended to a weighted ssGBLUP (wssGBLUP) that emulates the Bayesian variable selection method by allowing for unequal variances across loci (Wang et al., 2012).\nIn rainbow trout, the recent development of the 57K SNP Axiom® Trout Genotyping Array (Palti et al., 2015a; Affymetrix, Santa Clara, CA), a new genetic linkage map (published here) developed using 47,939 markers included in the 57K SNP Axiom® Trout Genotyping Array, and the release of a reference genome (Berthelot et al., 2014) have provided tools for studying FY using GWAS. The objectives of this study were to conduct a GWAS for FY and other carcass and body weight traits in a rainbow trout population developed at the National Center for Cool and Cold Water Aquaculture (NCCCWA) and selectively bred for improved growth performance, and to visualize gene networks and functional categories enriched among the putative genes detected by GWAS for the studied traits."}
MyTest
{"project":"MyTest","denotations":[{"id":"27920797-26472703-33226412","span":{"begin":370,"end":374},"obj":"26472703"},{"id":"27920797-22178851-33226413","span":{"begin":519,"end":523},"obj":"22178851"},{"id":"27920797-17709780-33226414","span":{"begin":777,"end":781},"obj":"17709780"},{"id":"27920797-23115522-33226415","span":{"begin":1063,"end":1067},"obj":"23115522"},{"id":"27920797-26194229-33226416","span":{"begin":1335,"end":1339},"obj":"26194229"},{"id":"27920797-26499328-33226417","span":{"begin":1523,"end":1527},"obj":"26499328"},{"id":"27920797-25757012-33226418","span":{"begin":1547,"end":1551},"obj":"25757012"},{"id":"27920797-26360524-33226419","span":{"begin":1592,"end":1596},"obj":"26360524"},{"id":"27920797-25888203-33226422","span":{"begin":1671,"end":1675},"obj":"25888203"},{"id":"27920797-19700728-33226423","span":{"begin":1878,"end":1882},"obj":"19700728"},{"id":"27920797-20105297-33226424","span":{"begin":1906,"end":1910},"obj":"20105297"},{"id":"27920797-11290733-33226425","span":{"begin":2257,"end":2261},"obj":"11290733"},{"id":"27920797-22624567-33226426","span":{"begin":2855,"end":2859},"obj":"22624567"},{"id":"27920797-24755649-33226428","span":{"begin":3186,"end":3190},"obj":"24755649"}],"namespaces":[{"prefix":"_base","uri":"https://www.uniprot.org/uniprot/testbase"},{"prefix":"UniProtKB","uri":"https://www.uniprot.org/uniprot/"},{"prefix":"uniprot","uri":"https://www.uniprot.org/uniprotkb/"}],"text":"Introduction\nFillet yield (FY, %), the ratio between the separable muscle of the fish and the harvest weight, is an economically important trait in rainbow trout aquaculture that reflects production efficiency with a consequent large impact on the returns (Kause et al., 2002). On a per-unit basis, the fillet price is three times that of the whole fish (Bugeon et al., 2010) and a recent global survey ranked FY among the six most important traits for genetic improvement in rainbow trout aquaculture (Sae-Lim et al., 2012). Despite that, FY has not received much attention in breeding programs because it is a lethally-measured trait, costly to measure, and difficult to select on, thus limiting the genetic progress in traditional selective breeding programs (Kause et al., 2007).\nTo overcome these phenotyping constraints and to increase the accuracy of selection, the use of high-density single nucleotide polymorphism (SNP) genotyping platforms and novel statistical models can identify SNPs associated with the trait for use in genomic selection (Dekkers, 2012). Several genome-wide association studies (GWAS) that match genetic variants, with or without pedigree records, with the observed phenotype have identified significant loci associated with economically important traits in livestock (Kadarmideen, 2014; Sharma et al., 2015). The aquaculture research community is following this trend, and interesting examples are available for growth and disease resistance traits in Atlantic Salmon (e.g., Correa et al., 2015; Gutierrez et al., 2015), rainbow trout (e.g., Kocmarek et al., 2015; Liu et al., 2015b; Palti et al., 2015b), and catfish (e.g., Geng et al., 2015).\nSingle-step genomic best linear unbiased prediction (ssGBLUP) and Bayesian variable selection methods are available for genomic predictions using dense SNP arrays. The ssGBLUP method (Misztal et al., 2009; Christensen and Lund, 2010) jointly incorporates all available genotypic, phenotypic, and pedigree data in the genomic predictions, but the method's assumption of an infinitesimal model is violated for traits affected by major genes. In contrast, Bayesian variable selection methods assume a prior distribution of the variance associated with each locus (Meuwissen et al., 2001), but are limited in that only data from genotyped animals are used in the analyses. Despite the limitations on data, the Bayesian variable selection method has resulted in greater accuracy of predicted genetic merit compared to ssGBLUP for traits known to have QTL segregating with moderate or large effect like bacterial cold water disease resistance in rainbow trout (Vallejo et al., submitted manuscript). The ssGBLUP method has been extended to a weighted ssGBLUP (wssGBLUP) that emulates the Bayesian variable selection method by allowing for unequal variances across loci (Wang et al., 2012).\nIn rainbow trout, the recent development of the 57K SNP Axiom® Trout Genotyping Array (Palti et al., 2015a; Affymetrix, Santa Clara, CA), a new genetic linkage map (published here) developed using 47,939 markers included in the 57K SNP Axiom® Trout Genotyping Array, and the release of a reference genome (Berthelot et al., 2014) have provided tools for studying FY using GWAS. The objectives of this study were to conduct a GWAS for FY and other carcass and body weight traits in a rainbow trout population developed at the National Center for Cool and Cold Water Aquaculture (NCCCWA) and selectively bred for improved growth performance, and to visualize gene networks and functional categories enriched among the putative genes detected by GWAS for the studied traits."}