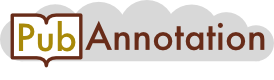
PMC:5003481 / 4541-5785
Annnotations
2_test
{"project":"2_test","denotations":[{"id":"27605182-22529925-69477431","span":{"begin":127,"end":129},"obj":"22529925"},{"id":"27605182-22050602-69477432","span":{"begin":195,"end":197},"obj":"22050602"},{"id":"27605182-20736237-69477433","span":{"begin":412,"end":414},"obj":"20736237"},{"id":"27605182-20736237-69477434","span":{"begin":790,"end":792},"obj":"20736237"}],"text":"2. Experimental Section\nMicroRNA expression profiles were downloaded from Gene Expression Omnibus [11] GSE32935 (aging heart; [12] Exiqon Mouse microRNA v11.0) and GSE35672 (iPS-cardiomyocytes; [13], Illumina Human v2 MicroRNA expression beadchip datasets). microRNA microarray data from ventricular maturation (MirVana v2.0, miRBase version 8.0) were already available to us, as described by Chinchilla et al. [14]. To minimize technical (non-biological) variability among arrays, each data group was independently log2 transformed and then normalized using the quantiles normalization function implemented in the Bioconductor limma package [15], with default parameters run in R software [16], and finally, each probe was Z-scored (). MicroRNA expression data in ventricular development [14], also Z-scored, was identically processed as describe above, but with an initial k-nearest neighbor(KNN) imputation of the densitometry values \u003c1 using the KNN algorithm implemented in the Bioconductor impute package [15] with default parameters. Hierarchical clustering (Euclidean distance and complete linkage), an unsupervised way of grouping samples based only on their gene expression similarities, was carried out using TM4 software suite [17]."}