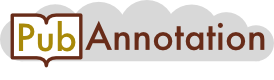
PMC:4996411 / 15560-18256
Annnotations
2_test
{"project":"2_test","denotations":[{"id":"27600238-19914823-69479219","span":{"begin":1206,"end":1208},"obj":"19914823"},{"id":"27600238-17768165-69479220","span":{"begin":1209,"end":1211},"obj":"17768165"},{"id":"27600238-21901597-69479221","span":{"begin":1234,"end":1236},"obj":"21901597"},{"id":"27600238-19176552-69479222","span":{"begin":1362,"end":1364},"obj":"19176552"},{"id":"27600238-17599930-69479223","span":{"begin":2184,"end":2186},"obj":"17599930"},{"id":"27600238-22467912-69479224","span":{"begin":2344,"end":2346},"obj":"22467912"}],"text":"3.2. Protein Quantification\nTwo principally different mathematical approaches are employed to infer numeric values that reflect the expression level of proteins assessed by RPPA: parametric and non-parametric models (Figure 2).\nFigure 2 Parametric and non-parametric approaches for protein quantification in RPPA data sets. While parametric approaches employ pre-defined functions to describe the relationship between measured expression levels and protein concentration, non-parametric approaches are comparably more data-adaptive and use e.g., protein-specific response curves. Such statistical models contain so-called parameters that can either describe certain experimental characteristics, e.g., background noise or saturation level, and are defined before fitting the model to the data or are not defined initially. Thus, parametric models employ certain assumptions regarding the function used to describe the relation between the observed expression levels and the unobserved and yet unknown protein concentration. On the contrary, non-parametric models do not imply such a predefined form of the model, so that they are highly data-adaptive.\nParametric approaches have employed linear models [11,32] or sigmoidal curves [12,28] to determine a response curve, the relationship between the observed signal and the protein concentration. Zhang et al. [23] used the Sips model, which is similar to a logistic model, to determine the relationship between signals in successive dilution steps. Zhang and colleagues argued that the response curve depends on factors such as the target protein concentration and exposure time during signal detection, specific or non-specific interactions of reporter molecules with proteins of a particular sample or of the slide matrix. While the impact of experimental factors might be difficult to quantify and to incorporate into a statistical model, the serial dilution curve method allows the determination of experimentally meaningful model parameters that can be optimized further.\nHowever, non-parametric methods seem to prevail due to their flexibility and their robust results. Non-parametric examples include the model of Hu et al. [16] which was based on the assumption that protein expression equals a non-parametric monotonically increasing function. The regularized approach by Li et al. [31] proposed an estimation of protein levels based on individual non-aggregated dilution series replicates to account for within-sample or within-group variability. Non-parametric parameters are more difficult to interpret but do not impose prior assumptions on experimental factors such as the reaction kinetics of the RPPA signal detection procedure."}