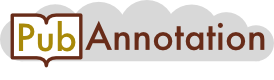
PMC:4996406 / 46014-47587
Annnotations
{"target":"https://pubannotation.org/docs/sourcedb/PMC/sourceid/4996406","sourcedb":"PMC","sourceid":"4996406","source_url":"https://www.ncbi.nlm.nih.gov/pmc/4996406","text":"8. Conclusions\nIn this paper, we proposed a synthetic kinome array data generator to synthesize datasets for which correct analysis results are known. The proposed synthetic data generator allows the user to control the level of noise in generation of a synthesized kinome array using the fold-change threshold parameter and the significance level parameter. The proposed method also relies on actual intensity measurements from kinome microarray experiments to preserve subtle characteristics of the original kinome microarray data. Histograms and statistical tests of actual arrays and synthesized arrays indicate that the synthesized arrays follow the same distribution as that of actual kinome arrays. The utility of the algorithm was demonstrated by evaluating Log2 and VSN normalization methods.\nIn order to compare these methods, we considered their effects on improving the results of downstream data analysis, which is the main goal of all normalization and preprocessing methods. More specifically, since the main purpose of kinome microarray experiments is to classify differentially and non-differentially phosphorylated peptides, we used sensitivity, specificity, precision, and accuracy as performance measures for peptide classification. The statistical data analysis indicated the superiority of VSN over Log2 method for the accuracy, sensitivity, and precision performance measures. Although we used a quantitative approach for comparing variance stabilization methods, this result is in accordance with other research in the transcriptional DNA community.","divisions":[{"label":"title","span":{"begin":0,"end":14}},{"label":"p","span":{"begin":15,"end":801}}],"tracks":[]}