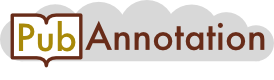
PMC:4996396 / 11910-22370
Annnotations
{"target":"https://pubannotation.org/docs/sourcedb/PMC/sourceid/4996396","sourcedb":"PMC","sourceid":"4996396","source_url":"https://www.ncbi.nlm.nih.gov/pmc/4996396","text":"2. Experimental Section\n\n2.1. Patient Cohort\nThe study population comprised 100 newly diagnosed and previously untreated lung cancer patients and 100 lung cancer-free control samples. All samples used in the present study were provided by the biobank of the Molecular Epidemiology group headed by Andrea Gsur at the Medical University, Department of Medicine I, Institute of Cancer Research, Vienna. Cases were recruited from two pulmonological centers in Vienna and Lower Austria. Regarding histological data, cases were further classified as 25 small-cell lung carcinoma (SCLC), 25 squamous cell carcinoma (SqLC), 25 large cell carcinoma (LCLC), and 25 adenocarcinoma (AdCa). Seventy-two hospital-based controls were derived from the Department of Orthopedics of the Medical University of Vienna. Only subjects with negative chest radiography and no history of malignant disease were selected as controls. Additionally, 28 controls were recruited from the colorectal cancer screening project “Burgenland Prevention Trial of Colorectal Cancer Disease with Immunological Testing” (B-PREDICT). These individuals underwent colonoscopy and did not show any pathological finding. All participants were Austrian Caucasians. Clinical characteristics of the samples are summarized in Table 1. \nBlood was collected using Vacuette BD vacutainer® tubes (Greiner Bio-One, Kremsmünster, Austria) with sodium heparin as anticoagulant. After collection, heparinized blood was centrifuged at 2000 × g for 10 minutes and plasma was removed from the cell pellet and stored at −80 °C. Written informed consent was obtained from all participants and research protocols were approved by the institutional review boards.\nmicroarrays-04-00162-t001_Table 1 Table 1 Clinical characteristics of the study population. a At time of diagnosis.\n\n2.2. Human IgG Isolation\nHuman immunoglobulin G (IgG) was isolated from plasma samples using the Melon®Gel Spin Plate Kit for IgG Screening (Thermo Scientific, Rockford, IL, USA) following the manufacturer’s instructions. IgG samples were stored over night at 4 °C until further processing. Concentration of purified IgG was measured spectrophotometrically at 280 nm on an Epoch Microplate Spectrophotometer (Biotek, Winooski, VT, USA). As blank sample Melon Gel Purification Buffer was used. Samples were measured in duplicates and arithmetic average was calculated. Pre-casted NuPAGE® 4%–12% Bis-Tris polyacrylamide gels (Novex®, Carlsbad, CA, USA) were used to verify IgG purity. The strongest band was observed at 150 kDa, which corresponds to the molecular weight of undegraded human IgG [44]. Integrity of all samples was confirmed.\n\n2.3. 16k Protein Microarray\nThe in-house printed 16k protein array comprised 5449 recombinantly expressed proteins derived from 15,417 cDNA clones, printed in duplicates on the microarray resulting in approximately 32,000 spots. An illustrated microarray design of the 16k array can be found in the supplementary section (Supplementary Figure S1). The cDNA clones are included in the UniPEx expression library purchased from ImaGenes GmbH (Berlin, Germany). Sequences included in this library comprise clones derived from human fetal brain, T-cells, lung, and colon protein expression libraries. The library consists of clones with two differently modified pQE vectors. Protein expression from clones carrying the pQE80LSN vector is induced by isopropyl-β-D-thiogalactopyranosid (IPTG). Clones with the pQE30NST vector inserted exhibit auto-induced protein expression. Both types of vectors include an N-terminal 6xHis-tag [45]. Protein expression and purification was conducted as described in Stempfer et al. [22]. To estimate purity of the UniPEx proteins after purification with Ni-NTA, protein eluates were run on SDS-PAGE using NuPAGE® Novex 4%–12% Bis-Tris gels (Supplementary Figure S2).\nSU8 epoxide-coated glass slides were printed with contact printing technology using a NanoPrintTM LM210 (Dynamic Devices, Wilmington, DE, USA) with 48 SMP2 Stealth Microarray Printing Pins (TeleChem ArrayIt Microarray Division, Sunnyvale, CA, USA). E. coli lysate in the concentrations 0.3, 0.4, and 0.5 mg mL−1 was used as positive control (n = 281), and elution buffer (50 mM Na2HPO4, 500 mM imidazole, 0.3 M NaCl, 0.01% SDS and 0.005% NaN3) was spotted as negative control (n = 82) onto the slides. Printed protein slides were stored at 4°C protected from light until processing.\n\n2.4. Protein Microarray Processing\nProtein microarrays were blocked for 30 minutes with DIG Easy Hyb solution (Roche, Basel, Switzerland), washed three times for 5 minutes with 1 × PBS + 0.1% Triton X buffer (PBS 10× pH 7.4 (Life Technologies, Carlsbad, CA, USA), Triton X-100 (Sigma-Aldrich, St. Louis, MO, USA) and rinsed with ddH2O. Slides were dried for 2 minutes by centrifugation at 900 rpm. Purified IgG samples were adjusted to a concentration of 0.4 μg μL−1 with Melon Gel Purification Buffer and were finally diluted 1:2 with 2 × PBS + 0.2% Triton X buffer containing 6% milk powder. 490 μL of the diluted samples were applied onto a single-chamber hybridization gasket slide (Agilent Technologies, Santa Clara, CA, USA) and the 16k protein slide was placed on top, allowing the spotted protein side of the slide to face the IgG sample. Samples were incubated on the 16k microarray slides for 4 hours under rotating conditions, 12 rpm at room temperature. Slides were washed three times with 1 × PBS + 0.1% Triton X wash buffer and rinsed with ddH2O. As detection antibody, Alexa Fluor® 647 Goat Anti-Human IgG (H+L) (Molecular Probes®, Carlsbad, CA, USA) was used, diluted 1:10,000 in PBS + 0.1% Triton X-100 + 3% milk powder. Incubation was done for 1 hour. Slides were again washed three times with 1 × PBS + 0.1% Triton X washing buffer, rinsed with ddH2O and spin-dried. \nMicroarray slides were scanned with an Agilent G2565CA Microarray Scanner System (Agilent Technologies, Santa Clara, CA, USA) using a helium-neon laser (633 nm, red dye channel). Slides were scanned with a resolution (pixel size) of 10 μm and sensitivity level of the red channel photomultiplier tube (PMT) was set to 70%. After scanning, images of slides were loaded into the GenePix® Pro 6.0 software (Molecular Devices, Sunnyvale, CA, USA) for subsequent data extraction. Median fluorescent intensity, corrected for local fluorescent background, for each IgG-probed protein spot was exported to a .gpr file for further statistical analysis.\n\n2.5. Data Processing\nStatistical analysis of the microarray data was performed using the R-based package BRB-ArrayTools, developed by Richard Simon [35]. Background-corrected median fluorescence values were loaded into the program and were log2-transformed. Furthermore, an average over replicate spots was calculated since all proteins were printed in duplicates resulting in a microarray comprising approximately 32,000 features. The intensity minimum threshold was set to a value of 100. If a feature was flagged in at least 50% of the arrays, it was excluded from analysis. \nData was normalized using different approaches and was further subjected to different statistical analyses. One method was quantile normalization, which equals distribution of values among different arrays. The second approach was data transformation using “Combating batch effects when combining batches of gene expression microarray data” (ComBat) in order to adjust data for batch effects [46]. For the application of ComBat, unnormalized data was used and missing values, which were due to flagging of low-quality features, were imputed. This was done using the package ‘impute’ for imputation of missing microarray data in R [47]. The third approach was distance weighted discrimination (DWD) which adjusts for systematic microarray data biases [32]. By means of using DWD, unnormalized data is loaded into the software but only two batches can be merged at one time. Therefore, a stepwise approach was applied for data adjustment. According to the PCA plot of the unnormalized data (Figure 3), two main groups of batches can be observed in the raw data. Therefore, the runs of each group were merged first before merging the two main groups in the last step. A similar approach was used by Benito and colleagues [32]. PCA [48] and PVCA [49] were used to investigate batch effects and underlying sources for variation.\nFurther statistical analysis was performed with BRB-ArrayTools. Mainly class prediction with complete leave-one-out cross-validation (LOOCV), which includes the steps of feature selection and classification model development, and class comparison were applied to different data subsets, which were pre-processed by different methods. Analysis was performed for all lung cancer cases versus all controls as well as for distinct histological types of lung cancer and their matched controls. Class comparison analysis was performed with a significance threshold of p \u003c 0.001, resulting in a list of significant differentially reactive antigens when comparing two sample groups. These lists were generated with unnormalized, quantile normalized, DWD-adjusted, and ComBat-adjusted data. Besides analysis of the sample groups, including all lung cancer samples versus all controls and the groups comprising only the histological subtype of lung cancer versus statistically matched controls, class comparison analysis was applied on the data of each histological subtype derived from single processing batches (run 1 to run 4). Moreover, cross-run class comparison was performed for each histological entity. The resulting lists of significant antigens were compared to each other for overlaps. \nClass prediction analysis was performed on the same data set including the analysis of all lung cancer cases versus all controls with quantile normalization and ComBat adjustment, as well as the analysis of distinct histological entities derived from multiple runs versus matched controls using quantile normalization, ComBat, and DWD adjustment. BRB-ArrayTools provides this supervised machine learning method with LOOCV. As feature selection methods, the greedy-pairs method [46] and the recursive feature elimination (RFE) method [50] were used. Methods used for model development were diagonal linear discriminant analysis, compound covariate predictor, nearest neighbor classification, nearest centroid classification, support vector machines and Bayesian compound covariate predictor [35].\n","divisions":[{"label":"Title","span":{"begin":0,"end":24}},{"label":"Section","span":{"begin":25,"end":1821}},{"label":"Title","span":{"begin":25,"end":44}},{"label":"Table caption","span":{"begin":1700,"end":1820}},{"label":"Section","span":{"begin":1820,"end":2658}},{"label":"Title","span":{"begin":1820,"end":1844}},{"label":"Section","span":{"begin":2660,"end":4438}},{"label":"Title","span":{"begin":2660,"end":2687}},{"label":"Section","span":{"begin":4440,"end":6471}},{"label":"Title","span":{"begin":4440,"end":4474}},{"label":"Section","span":{"begin":6472,"end":10459}},{"label":"Title","span":{"begin":6472,"end":6492}}],"tracks":[{"project":"2_test","denotations":[{"id":"27600218-11406581-69481427","span":{"begin":2614,"end":2616},"obj":"11406581"},{"id":"27600218-21372816-69481428","span":{"begin":3584,"end":3586},"obj":"21372816"},{"id":"27600218-21078204-69481429","span":{"begin":3672,"end":3674},"obj":"21078204"},{"id":"27600218-19455231-69481430","span":{"begin":6621,"end":6623},"obj":"19455231"},{"id":"27600218-14693816-69481431","span":{"begin":7802,"end":7804},"obj":"14693816"},{"id":"27600218-14693816-69481432","span":{"begin":8270,"end":8272},"obj":"14693816"},{"id":"27600218-19455231-69481433","span":{"begin":10455,"end":10457},"obj":"19455231"}],"attributes":[{"subj":"27600218-11406581-69481427","pred":"source","obj":"2_test"},{"subj":"27600218-21372816-69481428","pred":"source","obj":"2_test"},{"subj":"27600218-21078204-69481429","pred":"source","obj":"2_test"},{"subj":"27600218-19455231-69481430","pred":"source","obj":"2_test"},{"subj":"27600218-14693816-69481431","pred":"source","obj":"2_test"},{"subj":"27600218-14693816-69481432","pred":"source","obj":"2_test"},{"subj":"27600218-19455231-69481433","pred":"source","obj":"2_test"}]}],"config":{"attribute types":[{"pred":"source","value type":"selection","values":[{"id":"2_test","color":"#ece293","default":true}]}]}}