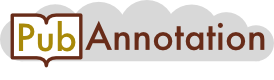
PMC:4979055 / 7249-22498
Annnotations
{"target":"https://pubannotation.org/docs/sourcedb/PMC/sourceid/4979055","sourcedb":"PMC","sourceid":"4979055","source_url":"https://www.ncbi.nlm.nih.gov/pmc/4979055","text":"3. Results and Discussion\nSome of the datasets had a very large number of differentially expressed genes. In the cases of E-GEOD-19188, E-GEOD-40725 and E-GEOD-50081 it was necessary to reduce the number of differentially expressed genes before running ReactomePA as the large number of genes had a negative effect on the algorithm finding any enriched pathways. In the cases of the E-GEOD-18842 and EG-GEOD-43458 datasets although there are a large number of differentially expressed genes these come from a small number of pathways, and so the pathway analysis could be carried out without the use of a cut-off. A plot of the adjusted p-value for the differential expression shows a long almost horizontal region before a sharp rise and then a steady linear increase (Figure 1).\nFigure 1 A plot of the adjusted p-values for the top scoring probes from the differential expression analysis between adenocarcinoma and squamous cell carcinoma in the E-GEOD-50081 dataset. From the graph the point where the adjusted p-value starts to rise from the horizontal is around 2000 probes and so this was used as a limit on the number of differentially expressed genes in the problem datasets, except in the case of the normal vs. small-cell carcinoma comparison in dataset E‑GEOD-40725 where a cut-off of 1500 was needed.\n\n3.1. The Effect of Normalization on the Number of Differentially Expressed Genes\nTable 2 shows the numbers of differentially expressed probes and genes for the different datasets. In some datasets there are multiple sub-groups and so these datasets have multiple comparisons in order to determine the variation in expression levels. It is also important to note that the number of genes identified by their EntrezID number is larger than the number of probes identified on the array. This is because there is a one-to-many relationship between the probes and EntrezID. This is far from ideal as this means that there are cross-gene effects in the array.\nFrom the table it is clear that the method used for normalization makes a difference to the number of differentially expressed genes and sometimes this can be quite large, amounting to over 1000 genes or about 20% of the total number. This could have a significant impact on any subsequent gene set enrichment analysis.\nThere is also a general trend to a larger number of differentially expressed genes in the larger datasets. This reflects the higher power (improved sensitivity) of larger datasets, and the much smaller E‑GEOD-6044 has a particularly small number of variable genes. However, the p-value distribution (Figure 1) also suggests that in the larger datasets noise is becoming an issue and that there are a large number of supposedly differentially expressed genes might be an artifact of those datasets. The E‑GEOD-18842 and E-GEOD-43458 datasets both perform very well in the subsequent pathway analysis compared to the datasets where it was necessary to introduce a cut-off to reduce the number of genes being considered. Both of those datasets contained only two sub-groups and the division of the data into further sub-groups unless these were part of the original experimental design is highly controversial and likely to result in an increased number of false positives. Only the E-GEOD-50081 dataset suggests that there are a large number of differentially expressed genes between adenocarcinoma and squamous cell carcinoma, but this dataset was specifically created in order to ask this question.\nmicroarrays-03-00212-t002_Table 2 Table 2 The number of differentially expressed probes and genes (EntrezIDs) between the two specified conditions for each of the datasets normalized using rma, gcrma and farms. In cases where the cut-off of 2000 probes was used the number of EntrezIDs are given for these cut-off values.\n\n3.2. Pathway Analysis\nA pathway enrichment analysis of the differentially expressed genes was carried out against the Reactome pathway database using the ReactomePA module. Like gene set enrichment analysis this produces a list of key terms, in this case pathways, and a p-value for the probability that the observed distribution of expression occurred by chance. The cut-off value for the pathway p-values was chosen at 0.05. An example of the effect of normalization on the pathway analysis is given in Figure 2.\nIn this case there are a large number of additional pathways after farms normalization, but the pathways identified by the rma normalized analysis are conserved in the farms normalized results. It is possible that in this case farms normalization produces a dataset that is more sensitive to changes in pathway regulation, but it is also possible that a number of these pathways will be false positives.\nFigure 2 Pathway Analysis for E-GEOD-43458, Normal-Adenocarcinoma. (left) After rma normalization; (right) After farms normalization.\n\n3.2.1. Pathways that Are Differentially Expressed between Normal Lung Tissue and Tumors\nTwo of the datasets E-GEOD-18842 and E-GEOD-19188 compare normal lung tissue to NSCLC or tumor tissue, respectively. Of these E-GEOD-18842 has a much better defined list of differentially expressed genes. Again this is going to partly be a result of the larger size of the dataset as this results in a much lower standard error in the expression levels of the comparison groups and an increase in power of the experiment, but noise is also a factor as the final list contains almost all of the genes on the array. A cut-off had to be used for E-GEOD-19188 and only the top 2000 genes were used for pathway analysis.\nFrom the E-GEOD-18842 the three different normalization methods produce a very similar pathway analysis. The rma and farms normalized data give identical pathway analysis and identify 16 significant pathways (Figure 3). The results for the gcrma normalized data contain 12 out of the 16 previously identified pathways: deposition of new CENPA-containing nucleosomes at the centromere; telomere maintenance; unwinding of DNA and nucleosome assembly are absent, and separation of sister chromatids; resolution of sister chromatid cohesion; E2F mediated regulation of DNA replication; mitotic metaphase and anaphase; mitotic anaphase; DNA strand elongation and G2/M checkpoints are added.\nThe results from E-GEOD-19188 are less definitive (Figure 4). From the rma and gcrma normalized data over 40 pathways are identified as significant. There is considerable overlap between these two pathway sets with 37 of the top 40 pathways being conserved between the two analyses. The farms normalized data only yields 29 significant pathways and some of those identified are notably different from the other results, especially the pathways associated with erythrocytes that are absent from the other pathway analyses (O2/CO2 exchange in erythrocytes, hemostasis, erythrocytes take up oxygen and release carbon dioxide, erythrocytes take up carbon dioxide and release oxygen). Associations between hemostasis, platelets and cancer were proposed in the 1980s, but these associations have only recently become the focus of renewed attention for pathway analysis [29,30,31].\nFigure 3 Pathway Analysis for E-GEOD-18842, Normal-NSCLC (left) After rma normalization; (right) After gcrma normalisation. The overlapping pathways between the E-GEOD-18842 and E-GEOD-19188 contain many of the usual suspects, such as the control of mitosis and control of the cell cycle including checkpoints. The E2F mediated regulation of DNA replication has also been identified as important in a number of different cancers, as this transcription factor regulates cyclin E [32]. Two other pathways of interest are the Polo-like kinase mediated events and the activation of ATR in response to replication stress. Polo‑like kinase (PLK1) is a DNA damage checkpoint and it has been a target for cancer therapy [33,34]. Ataxia-telangiectasia and Rad3-related protein (ATR) halts DNA replication when replication forks are stalled and need to be repaired. The absence of gene creates fragile sites in the chromosome. ATR has been targeted as a possible cancer preventative or in boosting the effectiveness of existing therapies [35,36].\nFigure 4 Pathway Analysis for E-GEOD-19188, Healthy-Tumor (left) After rma normalization; (right) After farms normalization.\n\n3.2.2. Pathways that Are Differentially Expressed between Normal Lung Tissue and Adenocarcinoma\nThree datasets have a comparison between normal lung tissue and adenocarcinoma: E-GEOD-6044, E-GEOD-40725 and E-GEOD-43458. Of these the E-GEOD-43458 dataset is the largest and it is the only one that was specifically targeted to adenocarcinoma.\nThe smallest of these datasets is E-GEOD-6044. This array also used the hgfocus gene array and not the more complete hgu133a array. From the rma and farms normalized data less than 10 pathways were identified and these were: biological oxidation; axon guidance; hemostasis; platelet activation, signaling and aggregation; platelet degranulation; translation of GLUT4 to the plasma membrane; response to elevated platelet cytosolic Ca2+; L1CAM interactions; phase 1-functionalisation of compounds; membrane trafficking and extracellular matrix organization. It is noteworthy that none of these pathways were amongst those identified as differences between the normal and tumor tissues and that they are not involved in cell cycle or DNA regulation. Phase 1-functionalisation is the processing and export of proteins from the endoplasmic reticulum and the reactions involve cytochrome P-450. Sugar transporters have previously been identified as having a role in cancer [37]. The L1 cell adhesion molecule L1CAM is associated with invasive tumors and metastasis [38,39]. After gcrma normalization pathway analysis identifies over 40 significant pathways (Supplementary Figure S1). These include the DNA regulation, mitotic pathways and cell cycle pathways previously identified in the normal-tumor comparisons. It also includes the meiosis pathways, negative regulation of rRNA expression and amyloids.\nThe E-GEOD-40275 dataset uses an exon array and so it can only be normalized using rma. The results of pathway analysis are very different from those for the E-GEOD-6044 dataset and the normal‑tumor analysis. These include a number of cancer specific pathways involving mutants and also a group of pathways involved in mRNA regulation and processing (Figure 5).\nFigure 5 Pathway Analysis for E-GEOD-40275, Normal-Adenocarcinoma. The E-GEOD-43458 dataset could only be normalized with rma and farms as gcrma is incompatible with the array type used. The results from the pathway analysis have been shown in Figure 2. As stated above there is a large difference in the number of pathways identified between the two analyses, with many more differentially expressed pathways after farms normalization. Many of them are previously identified pathways involved in cell cycle regulation and mitosis. Amyloids appear in both of the pathway analyses and they were identified in the gcrma normalized pathway analysis of E-GEOD-6044. Serum amyloid a protein has been known for some time to be a biomarker for lung cancer and nasopharyngeal cancer [40,41]. Signaling pathways including the Rho GTPases, Wnt and TCF are also present in the post-farms pathway analysis. These signaling pathways have previously been associated with cancer, although TCF has mainly been associated with colorectal cancers [42,43,44,45,46]. The L1CAM pathway is also identified as being significant in agreement with the results from E-GEOD-6044.\n\n3.2.3. Pathways that Are Differentially Expressed between Normal Lung Tissue and Squamous Cell Carcinoma\nTwo datasets are available to compare the transcriptomes of normal lung tissue and squamous cell carcinoma, E-GEOD-6044 and E-GEOD-40275. The E-GEOD-6044 data shows a similar profile to the normal-adenocarcinoma pathway profile for E-GEOD-40275 including the TCF dependent signaling in response to Wnt (Supplementary Figures S2–S4). There are also shared pathways with the normal-adenocarcinoma analysis of the same dataset. The results suggest that squamous cell carcinoma has an affect on a more diverse range of pathways than adenocarcinoma.\nThe E-GEOD-40275 normal-squamous cell carcinoma comparison identifies platelet associated pathways as well as RNA processing as the most important groups of differentiated pathways (Figure 6). These overlap with the pathways identified in the normal-adenocarcinoma comparison carried out with the same dataset and also the normal-adenocarcinoma pathway differences identified using the E-GEOD-6044 data.\nFigure 6 Pathway analysis for E-GEOD-40275, Normal-Squamous Cell Carcinoma.\n\n3.2.4. Pathways that Are Differentially Expressed between Adenocarcinoma and Squamous Cell Carcinoma\nFrom the previous analysis it is apparent that there is considerable overlap in the pathways that are differentially expressed in adenocarcinoma and squamous cell carcinoma. There are three datasets that allow direct comparison between the transcriptomes of the two NSCLC sub-types, E-GEOD-6044, E-GEOD-40275 and E-GEOD-50081 (this dataset was specifically created to compare gene expression between cancer sub-types). Table 2 shows that there are only a small number of differentially expressed genes between the two NSCLC sub-types except in the E-GEOD-50081 dataset. It is therefore slightly surprising that after pathway analysis this large number of genes reduces to 13 pathways: unwinding DNA; type I hemidesmosome assembly; telomere maintenance; SIRTS1 negatively regulates rRNA expression; packaging of telomere ends; nucleosome assembly; metabolism of porphyrins; heme degradation; glucuronidation; DNA strand elongation; Deposition of new CENPA-containing nucleosomes at the centromere; condensation of prophase chromosomes and chromosome maintenance.\nThe E-GEOD-6044 dataset has many fewer differentially expressed genes (although more than are differentially expressed between normal-adenocarcinoma and normal-squamous cell carcinoma). This produces a much more extensive list of pathways, with novel pathways involved in extra-cellular processes, such as extracellular matrix organization, cell-cell communication, cell-cell junction organization and immune system. The data however is very noisy and there is poor overlap between the results from pathway analysis after the three different normalization methods and so these results should be considered with some caution.\nFinally the E-GEOD-40275 also shows a small number of differentially expressed pathways. These agree with the extra-cellular pathways found in the E-GEOD-6044 results lending support to those findings, and also include new pathways involved in fatty acid triacylglycerol, and ketone body metabolism, as well as the metabolism of lipids and lipoproteins. There are also two pathways highlighted that are involved in the regulation of the peroxisome proliferator-activated receptor alpha (PPARA). There is some evidence that PPARA is associated with breast cancer but this is the first time it has been identified as involved in lung cancer although the gamma receptor has previously been identified and playing a role in inhibiting lung cancer cell growth [47,48].\n\n","divisions":[{"label":"Title","span":{"begin":0,"end":25}},{"label":"Figure caption","span":{"begin":781,"end":973}},{"label":"Section","span":{"begin":1318,"end":3816}},{"label":"Title","span":{"begin":1318,"end":1398}},{"label":"Table caption","span":{"begin":3491,"end":3815}},{"label":"Section","span":{"begin":3815,"end":15248}},{"label":"Title","span":{"begin":3815,"end":3836}},{"label":"Figure caption","span":{"begin":4734,"end":4870}},{"label":"Section","span":{"begin":4870,"end":8303}},{"label":"Title","span":{"begin":4870,"end":4957}},{"label":"Figure caption","span":{"begin":7135,"end":7261}},{"label":"Figure caption","span":{"begin":8175,"end":8302}},{"label":"Section","span":{"begin":8302,"end":11562}},{"label":"Title","span":{"begin":8302,"end":8397}},{"label":"Figure caption","span":{"begin":10407,"end":10476}},{"label":"Section","span":{"begin":11564,"end":12697}},{"label":"Title","span":{"begin":11564,"end":11668}},{"label":"Figure caption","span":{"begin":12618,"end":12696}},{"label":"Section","span":{"begin":12696,"end":15247}},{"label":"Title","span":{"begin":12696,"end":12796}}],"tracks":[{"project":"2_test","denotations":[{"id":"27600345-2667057-69480487","span":{"begin":7124,"end":7126},"obj":"2667057"},{"id":"27600345-22682129-69480488","span":{"begin":7127,"end":7129},"obj":"22682129"},{"id":"27600345-21071699-69480489","span":{"begin":7130,"end":7132},"obj":"21071699"},{"id":"27600345-10980711-69480490","span":{"begin":7851,"end":7853},"obj":"10980711"},{"id":"27600345-16557283-69480491","span":{"begin":7854,"end":7856},"obj":"16557283"},{"id":"27600345-17431119-69480492","span":{"begin":8167,"end":8169},"obj":"17431119"},{"id":"27600345-17406033-69480493","span":{"begin":8170,"end":8172},"obj":"17406033"},{"id":"27600345-20706540-69480494","span":{"begin":9613,"end":9615},"obj":"20706540"},{"id":"27600345-19144458-69480495","span":{"begin":9705,"end":9707},"obj":"19144458"},{"id":"27600345-21860410-69480496","span":{"begin":9708,"end":9710},"obj":"21860410"},{"id":"27600345-14734450-69480497","span":{"begin":11186,"end":11188},"obj":"14734450"},{"id":"27600345-3734116-69480498","span":{"begin":11189,"end":11191},"obj":"3734116"},{"id":"27600345-12635176-69480499","span":{"begin":11440,"end":11442},"obj":"12635176"},{"id":"27600345-10921899-69480500","span":{"begin":11443,"end":11445},"obj":"10921899"},{"id":"27600345-15829953-69480501","span":{"begin":11446,"end":11448},"obj":"15829953"},{"id":"27600345-11900454-69480502","span":{"begin":11449,"end":11451},"obj":"11900454"},{"id":"27600345-12408868-69480503","span":{"begin":11452,"end":11454},"obj":"12408868"},{"id":"27600345-18586686-69480504","span":{"begin":15240,"end":15242},"obj":"18586686"},{"id":"27600345-10753637-69480505","span":{"begin":15243,"end":15245},"obj":"10753637"}],"attributes":[{"subj":"27600345-2667057-69480487","pred":"source","obj":"2_test"},{"subj":"27600345-22682129-69480488","pred":"source","obj":"2_test"},{"subj":"27600345-21071699-69480489","pred":"source","obj":"2_test"},{"subj":"27600345-10980711-69480490","pred":"source","obj":"2_test"},{"subj":"27600345-16557283-69480491","pred":"source","obj":"2_test"},{"subj":"27600345-17431119-69480492","pred":"source","obj":"2_test"},{"subj":"27600345-17406033-69480493","pred":"source","obj":"2_test"},{"subj":"27600345-20706540-69480494","pred":"source","obj":"2_test"},{"subj":"27600345-19144458-69480495","pred":"source","obj":"2_test"},{"subj":"27600345-21860410-69480496","pred":"source","obj":"2_test"},{"subj":"27600345-14734450-69480497","pred":"source","obj":"2_test"},{"subj":"27600345-3734116-69480498","pred":"source","obj":"2_test"},{"subj":"27600345-12635176-69480499","pred":"source","obj":"2_test"},{"subj":"27600345-10921899-69480500","pred":"source","obj":"2_test"},{"subj":"27600345-15829953-69480501","pred":"source","obj":"2_test"},{"subj":"27600345-11900454-69480502","pred":"source","obj":"2_test"},{"subj":"27600345-12408868-69480503","pred":"source","obj":"2_test"},{"subj":"27600345-18586686-69480504","pred":"source","obj":"2_test"},{"subj":"27600345-10753637-69480505","pred":"source","obj":"2_test"}]}],"config":{"attribute types":[{"pred":"source","value type":"selection","values":[{"id":"2_test","color":"#a793ec","default":true}]}]}}