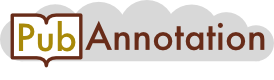
PMC:4979051 / 13114-18836
Annnotations
2_test
{"project":"2_test","denotations":[{"id":"27600352-15308542-69481368","span":{"begin":352,"end":353},"obj":"15308542"},{"id":"27600352-16585533-69481369","span":{"begin":354,"end":355},"obj":"16585533"},{"id":"27600352-20676074-69481370","span":{"begin":463,"end":465},"obj":"20676074"},{"id":"27600352-16585533-69481371","span":{"begin":582,"end":583},"obj":"16585533"},{"id":"27600352-11731795-69481372","span":{"begin":2172,"end":2174},"obj":"11731795"},{"id":"27600352-10521349-69481373","span":{"begin":2327,"end":2329},"obj":"10521349"},{"id":"27600352-20676074-69481374","span":{"begin":2449,"end":2451},"obj":"20676074"}],"text":"3.2. Robustness\nIn addition to having a good sensitivity, a good method for selecting the differentially expressed genes (DEG) should be robust, i.e., the lists of DEG generated by different samples should share a good fraction of genes. The lack of agreement between those lists is a well-known issue in microarray analysis, mainly in cancer studies [1,2]. Furthermore, a good reliability of the selected DEG correlates positively with the class predictability [25]. In order to assess the robustness of a method, we used a natural similarity measure introduced by Ein-Dor et al. [2], which is the fraction of genes shared by two lists of DEG obtained from different samples using a given method. More specifically, in order to estimate the robustness of the methods, we generated 100 training samples by taking a subset of n experiments chosen randomly. For each training sample we chose the Ntop=100 most significant genes obtained by the given method. Then, we compared the fraction of shared genes (fa,b) between the training samples a and b. The average of the fraction of shared genes over all combinations of two training samples (f=〈fa,b〉a≠b) is the figure of merit with which we represent and evaluate the robustness. Therefore, the closer is f to 1, the more robust is the method.\nFigure 1 ROC curve comparing the performance (sensitivity) of different ranking methodologies in identifying differentially expressed genes in spike-in data. (A) Comparison of our approach (median t-values) with different preprocessing methods used with t-test as ranking method to select the differentially expressed genes. (B) Comparison of our approach (median t-values) with the best performance of other ranking methods: t-test, SAM and LIMMA. The best performance of t-test, SAM and LIMMA is obtained when using RMA as a preprocessing algorithm. We calculated the average overlap for leukemia, breast cancer and multiple myeloma datasets for different sample sizes. The leukemia dataset consists of 24 samples of acute lymphoblastic leukemia (ALL) patients, 28 samples of acute myelogenous leukemia (AML) patients and 20 samples of mixed-lineage leukemia (MLL) patients [26]. We chose only the samples of leukemia type ALL and AML because these two types can be clearly distinguished based solely on gene expression profiles [27]. The Breast Cancer and Multiple Myeloma datasets were obtained from the MicroArray Quality Control (MAQC) consortium [25]. The Breast Cancer dataset can be divided according to two endpoints, pre-operative treatment response (pCR, pathologic complete response) and estrogen receptor (ER). The Multiple Myeloma dataset can be divided according to overall survival milestone outcome (OS-MO) and event free survival milestone outcome (EFS-MO), see Table A2 in the Appendix.\nWe compared the robustness of our methodology with different ranking methods: t-test, SAM and LIMMA, Figure 2, Figure 3 and Figure 4. The best performance of t-test, SAM and LIMMA was obtained using RMA as the preprocessing algorithm. Our approach (median t-value) shows a significantly higher overlap for the Leukemia and Multiple Myeloma datasets, Figure 2 and Figure 3, respectively. In the case of the Breast Cancer dataset, our approach shows a superior performance to the pre-operative treatment response (pCR, pathologic complete response) endpoint, but an inferior performance to the estrogen receptor (ER) endpoint, Figure 4. These results suggest that part of the lack of robustness in microarrays analysis is due to errors incorporated in the preprocessing steps, therefore explaining the significant gain of robustness of our methodology. However, we point out that for Breast Cancer and Multiple Myeloma, the levels of robustness for some analysis is still very low, suggesting a large biological heterogeneity among the samples.\nFigure 2 Average of the fraction of genes shared by two lists of differentially expressed genes (overlap) as a function of the sample size using the Leukemia dataset. Each list of differentially expressed genes is composed by the top 100 genes chosen according to different ranking methods, i.e., t-test, SAM and LIMMA (preprocessed by the RMA method), and our approach (median t-value) which does not require a preprocessing algorithm. The average value of the overlap between the lists is calculated over 100 lists chosen randomly.\nFigure 3 Average of the fraction of genes shared by two lists of differentially expressed genes (overlap) as a function of the sample size using the Multiple Myeloma dataset divided according to (A) Overall Survival Milestone Outcome (OS-MO) and (B) Event Free Survival Milestone Outcome (EFS-MO). Each list of differentially expressed genes is composed by the top 100 genes chosen according to different ranking methods, i.e., t-test, SAM and LIMMA (preprocessed by the RMA method), and our approach (median t-value) which does not require a preprocessing algorithm. The average value of the overlap between the lists is calculated over 100 lists chosen randomly.\nFigure 4 Average of the fraction of genes shared by two lists of differentially expressed genes (overlap) as a function of the sample size using the Breast Cancer dataset divided according to (A) pre-operative treatment response (pCR, pathologic complete response) and (B) estrogen receptor (ER) endpoint. Each list of differentially expressed genes is composed by the top 100 genes chosen according to different ranking methods, i.e., t-test, SAM and LIMMA (preprocessed by the RMA method), and our approach (median t-value) which does not require a preprocessing algorithm. The average value of the overlap between the lists is calculated over 100 lists chosen randomly.\n\n4"}