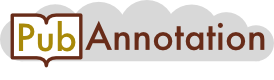
PMC:4845325 / 44516-45865
Annnotations
2_test
{"project":"2_test","denotations":[{"id":"27112350-22252388-72613531","span":{"begin":555,"end":557},"obj":"22252388"},{"id":"27112350-20179076-72613532","span":{"begin":1178,"end":1180},"obj":"20179076"}],"text":"Co-expression analysis was performed to identify proteins involved in AD pathogenesis that would inform us on more specific pathways than the broad ones implicated in the differential analysis above. Besides the mere difference in expression levels, proteins may differ in how they correlate with each other between disease and controls. We sought to discover protein networks that are changed between AD and Control by evaluating differential correlation matrices in an approach analogous to methods developed for analyzing genetic interaction profiles [78]. We thus created separate correlation matrices (Spearman’s rank correlation) in Matlab of all of the proteins measured for AD and Control signaling proteomes, respectively. Since cohort sizes were almost equal for AD and Controls we then calculated differential correlation profiles from these correlation matrices and used unsupervised clustering to identify 8 distinct clusters of proteins with highly similar differential correlation profiles (Fig. 4c, boxes). To demonstrate that differential co-expression data contains valuable biological information, we created semantic similarity scores for each protein pair [79]. Protein pairs with high differential co-expression profile correlation exhibited high semantic-similarity profile correlations as well (Additional file 1: Figure S5)."}