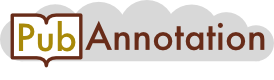
PMC:4845325 / 16163-17333
Annnotations
2_test
{"project":"2_test","denotations":[{"id":"27112350-21816131-72613480","span":{"begin":327,"end":329},"obj":"21816131"},{"id":"27112350-22206010-72613481","span":{"begin":431,"end":433},"obj":"22206010"},{"id":"27112350-11259398-72613482","span":{"begin":527,"end":529},"obj":"11259398"},{"id":"27112350-21912454-72613483","span":{"begin":584,"end":586},"obj":"21912454"},{"id":"27112350-7901004-72613484","span":{"begin":643,"end":645},"obj":"7901004"}],"text":"Exploring the “Regulation of growth” cluster in more detail, we observed several sub-clusters that partially aligned with known biological relationships, often bridging regulatory pathways together (Fig. 4d). For example APOE deficient mice are prone to atherosclerosis, but C3 modulation of lipid metabolism can protect them [15], while VEGFC is a marker for advanced atherosclerosis and hypercholesterolemia in the same animals [16]. TGFβ and interferons (IFN) have antagonizing relationships in the control of inflammation [17], while interferons reduce ghrelin (GHRL) expression [18], and Follistatin-like 1 (FSTL1) is controlled by TGFβ [19]. We also noticed that numerous proteins were directly or indirectly associated with or regulated by TGFβ/GDF/BMP signaling (e.g., TGFBR1, FSTL1, THBS1, MMP11, GREM1, GDF3, GDF5, GDF9), making that pathway a lead candidate to test for its involvement in AD. These data suggest that co-expression analysis can be used to identify clusters of proteins and regulatory pathways that are linked through similar co-expression profiles, potentially indicating pathways that are affected by or affecting AD pathology simultaneously."}