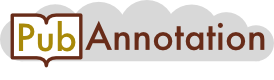
PMC:4636534 / 5954-7590
Annnotations
TEST0
{"project":"TEST0","denotations":[{"id":"26003789-150-156-67111","span":{"begin":348,"end":350},"obj":"[\"9891180\"]"},{"id":"26003789-154-160-67112","span":{"begin":352,"end":354},"obj":"[\"15968029\"]"},{"id":"26003789-129-135-67113","span":{"begin":984,"end":986},"obj":"[\"15858079\"]"},{"id":"26003789-50-56-67114","span":{"begin":1337,"end":1339},"obj":"[\"22990082\"]"}],"text":"Decision-analytic modelling\nWhile studies are being performed to inform decision makers about optimal DI tests, these studies often focus on the accuracy and short-term effects of the imaging test. However, for decision making it is also important to know how well a test can help to improve health outcome (e.g., survival and/or quality of life) [16, 21]. In addition, the societal impact may be relevant, including the cost-effectiveness or value for the money needed for a diagnostic test. It is possible to undertake trials that include such long-term consequences but these trials are often costly and practically challenging, due to the remoteness of the effects, requiring long follow-up periods and large sample sizes. Moreover, withholding a non-invasive imaging test that might provide useful diagnostic information may create ethical dilemmas.\nGiven these limitations, decision-analytic modelling is often used to synthesize data from trials with other available evidence [13]. Hence, the characteristics of a diagnostic test (e.g., sensitivity and specificity) can be linked with long-term patient outcomes. The results of these models tend to better fit the needs of decision makers.\nThe development and analysis of a decision-analytic model proceeds in a stepwise fashion. Comprehensive guidelines were recently published [22]. We present a six-step methodology for CEA in DI, including: (1) Defining the decision problem, (2) Choosing, and further developing the decision model, (3) Selecting input parameters, (4) Analysis and uncertainty analysis, (5) Interpretation of results , and (6) Transferability and validation."}
0_colil
{"project":"0_colil","denotations":[{"id":"26003789-9891180-67111","span":{"begin":348,"end":350},"obj":"9891180"},{"id":"26003789-15968029-67112","span":{"begin":352,"end":354},"obj":"15968029"},{"id":"26003789-15858079-67113","span":{"begin":984,"end":986},"obj":"15858079"},{"id":"26003789-22990082-67114","span":{"begin":1337,"end":1339},"obj":"22990082"}],"text":"Decision-analytic modelling\nWhile studies are being performed to inform decision makers about optimal DI tests, these studies often focus on the accuracy and short-term effects of the imaging test. However, for decision making it is also important to know how well a test can help to improve health outcome (e.g., survival and/or quality of life) [16, 21]. In addition, the societal impact may be relevant, including the cost-effectiveness or value for the money needed for a diagnostic test. It is possible to undertake trials that include such long-term consequences but these trials are often costly and practically challenging, due to the remoteness of the effects, requiring long follow-up periods and large sample sizes. Moreover, withholding a non-invasive imaging test that might provide useful diagnostic information may create ethical dilemmas.\nGiven these limitations, decision-analytic modelling is often used to synthesize data from trials with other available evidence [13]. Hence, the characteristics of a diagnostic test (e.g., sensitivity and specificity) can be linked with long-term patient outcomes. The results of these models tend to better fit the needs of decision makers.\nThe development and analysis of a decision-analytic model proceeds in a stepwise fashion. Comprehensive guidelines were recently published [22]. We present a six-step methodology for CEA in DI, including: (1) Defining the decision problem, (2) Choosing, and further developing the decision model, (3) Selecting input parameters, (4) Analysis and uncertainty analysis, (5) Interpretation of results , and (6) Transferability and validation."}
MyTest
{"project":"MyTest","denotations":[{"id":"26003789-9891180-29373354","span":{"begin":348,"end":350},"obj":"9891180"},{"id":"26003789-15968029-29373355","span":{"begin":352,"end":354},"obj":"15968029"},{"id":"26003789-15858079-29373356","span":{"begin":984,"end":986},"obj":"15858079"},{"id":"26003789-22990082-29373357","span":{"begin":1337,"end":1339},"obj":"22990082"}],"namespaces":[{"prefix":"_base","uri":"https://www.uniprot.org/uniprot/testbase"},{"prefix":"UniProtKB","uri":"https://www.uniprot.org/uniprot/"},{"prefix":"uniprot","uri":"https://www.uniprot.org/uniprotkb/"}],"text":"Decision-analytic modelling\nWhile studies are being performed to inform decision makers about optimal DI tests, these studies often focus on the accuracy and short-term effects of the imaging test. However, for decision making it is also important to know how well a test can help to improve health outcome (e.g., survival and/or quality of life) [16, 21]. In addition, the societal impact may be relevant, including the cost-effectiveness or value for the money needed for a diagnostic test. It is possible to undertake trials that include such long-term consequences but these trials are often costly and practically challenging, due to the remoteness of the effects, requiring long follow-up periods and large sample sizes. Moreover, withholding a non-invasive imaging test that might provide useful diagnostic information may create ethical dilemmas.\nGiven these limitations, decision-analytic modelling is often used to synthesize data from trials with other available evidence [13]. Hence, the characteristics of a diagnostic test (e.g., sensitivity and specificity) can be linked with long-term patient outcomes. The results of these models tend to better fit the needs of decision makers.\nThe development and analysis of a decision-analytic model proceeds in a stepwise fashion. Comprehensive guidelines were recently published [22]. We present a six-step methodology for CEA in DI, including: (1) Defining the decision problem, (2) Choosing, and further developing the decision model, (3) Selecting input parameters, (4) Analysis and uncertainty analysis, (5) Interpretation of results , and (6) Transferability and validation."}
2_test
{"project":"2_test","denotations":[{"id":"26003789-9891180-29373354","span":{"begin":348,"end":350},"obj":"9891180"},{"id":"26003789-15968029-29373355","span":{"begin":352,"end":354},"obj":"15968029"},{"id":"26003789-15858079-29373356","span":{"begin":984,"end":986},"obj":"15858079"},{"id":"26003789-22990082-29373357","span":{"begin":1337,"end":1339},"obj":"22990082"}],"text":"Decision-analytic modelling\nWhile studies are being performed to inform decision makers about optimal DI tests, these studies often focus on the accuracy and short-term effects of the imaging test. However, for decision making it is also important to know how well a test can help to improve health outcome (e.g., survival and/or quality of life) [16, 21]. In addition, the societal impact may be relevant, including the cost-effectiveness or value for the money needed for a diagnostic test. It is possible to undertake trials that include such long-term consequences but these trials are often costly and practically challenging, due to the remoteness of the effects, requiring long follow-up periods and large sample sizes. Moreover, withholding a non-invasive imaging test that might provide useful diagnostic information may create ethical dilemmas.\nGiven these limitations, decision-analytic modelling is often used to synthesize data from trials with other available evidence [13]. Hence, the characteristics of a diagnostic test (e.g., sensitivity and specificity) can be linked with long-term patient outcomes. The results of these models tend to better fit the needs of decision makers.\nThe development and analysis of a decision-analytic model proceeds in a stepwise fashion. Comprehensive guidelines were recently published [22]. We present a six-step methodology for CEA in DI, including: (1) Defining the decision problem, (2) Choosing, and further developing the decision model, (3) Selecting input parameters, (4) Analysis and uncertainty analysis, (5) Interpretation of results , and (6) Transferability and validation."}