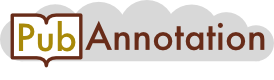
PMC:4636534 / 18790-21478
Annnotations
TEST0
{"project":"TEST0","denotations":[{"id":"26003789-90-96-67125","span":{"begin":269,"end":271},"obj":"[\"22990087\"]"},{"id":"26003789-97-103-67126","span":{"begin":1352,"end":1354},"obj":"[\"22990087\"]"}],"text":"Analysis of values and uncertainty\nCost-effectiveness modelling serves two purposes. First, it serves as an estimation of expected costs and outcomes for medical decision-making. Secondly, it assesses the uncertainties around these estimates and its range of validity [36].\nThe expected costs and outcomes can be obtained by multiplying the probabilities with their relevant costs and health outcomes. This results in average expected values per patient, for costs as well as health outcome. Specific software (e.g., TreeAgePro, TreeAge Software, Williamstown, MA, U.S.) can be used for this purpose, but Markov models and decision trees are also often analyzed using standard PC software (Microsoft Excel). The quality of estimates derived from the model directly depends on the quality of the input parameters. In general, the input parameters are desired to be as evidence-based as possible. However, model input parameters are surrounded by uncertainties. Parameter uncertainty reflects the uncertainty of the model input parameters because we do not know the precise values. This can be a result of multiple and conflicting or low quality studies, or merely from variation in the data as reflected in standard deviations surrounding a mean estimate. Parameter uncertainty can be addressed by a deterministic or probabilistic sensitivity analysis [36]. In a deterministic sensitivity analysis the mean values of the input parameters are altered to assess impact on total cost and outcome of the model. Even in the case when input parameters of the model might not be available, hypothetical values and borders can be set in the model to determine hypothetical estimates.\nAlternatively, parameter uncertainty can be addressed by means of a probabilistic sensitivity analysis. Each uncertain model input parameter is assigned a distribution, based on the mean and standard deviation/error as found in the literature. Examples of distributions surrounding the input parameters can be found in Table 1. In the probabilistic sensitivity analysis, different samples are taken from these distributions. For each sample, the hypothetical cohort runs through the model based on these sampled probabilities, and costs and outcome are derived. This results in a range of estimates of costs and outcome, representing the uncertainty in the result of the cost-effectiveness estimation. This range in outcome is usually expressed as 95 % Confidence Intervals (CI), based on the probabilistic analysis. In our example, the expected costs per patient are € 15,266 (95 % CI € 14,072–16,440) and expected QALYs 1.30 (95 % CI 1.00–1.61), if all patients were to receive PET-CT at three months follow-up."}
0_colil
{"project":"0_colil","denotations":[{"id":"26003789-22990087-67125","span":{"begin":269,"end":271},"obj":"22990087"},{"id":"26003789-22990087-67126","span":{"begin":1352,"end":1354},"obj":"22990087"}],"text":"Analysis of values and uncertainty\nCost-effectiveness modelling serves two purposes. First, it serves as an estimation of expected costs and outcomes for medical decision-making. Secondly, it assesses the uncertainties around these estimates and its range of validity [36].\nThe expected costs and outcomes can be obtained by multiplying the probabilities with their relevant costs and health outcomes. This results in average expected values per patient, for costs as well as health outcome. Specific software (e.g., TreeAgePro, TreeAge Software, Williamstown, MA, U.S.) can be used for this purpose, but Markov models and decision trees are also often analyzed using standard PC software (Microsoft Excel). The quality of estimates derived from the model directly depends on the quality of the input parameters. In general, the input parameters are desired to be as evidence-based as possible. However, model input parameters are surrounded by uncertainties. Parameter uncertainty reflects the uncertainty of the model input parameters because we do not know the precise values. This can be a result of multiple and conflicting or low quality studies, or merely from variation in the data as reflected in standard deviations surrounding a mean estimate. Parameter uncertainty can be addressed by a deterministic or probabilistic sensitivity analysis [36]. In a deterministic sensitivity analysis the mean values of the input parameters are altered to assess impact on total cost and outcome of the model. Even in the case when input parameters of the model might not be available, hypothetical values and borders can be set in the model to determine hypothetical estimates.\nAlternatively, parameter uncertainty can be addressed by means of a probabilistic sensitivity analysis. Each uncertain model input parameter is assigned a distribution, based on the mean and standard deviation/error as found in the literature. Examples of distributions surrounding the input parameters can be found in Table 1. In the probabilistic sensitivity analysis, different samples are taken from these distributions. For each sample, the hypothetical cohort runs through the model based on these sampled probabilities, and costs and outcome are derived. This results in a range of estimates of costs and outcome, representing the uncertainty in the result of the cost-effectiveness estimation. This range in outcome is usually expressed as 95 % Confidence Intervals (CI), based on the probabilistic analysis. In our example, the expected costs per patient are € 15,266 (95 % CI € 14,072–16,440) and expected QALYs 1.30 (95 % CI 1.00–1.61), if all patients were to receive PET-CT at three months follow-up."}
MyTest
{"project":"MyTest","denotations":[{"id":"26003789-22990087-29373368","span":{"begin":269,"end":271},"obj":"22990087"},{"id":"26003789-22990087-29373369","span":{"begin":1352,"end":1354},"obj":"22990087"}],"namespaces":[{"prefix":"_base","uri":"https://www.uniprot.org/uniprot/testbase"},{"prefix":"UniProtKB","uri":"https://www.uniprot.org/uniprot/"},{"prefix":"uniprot","uri":"https://www.uniprot.org/uniprotkb/"}],"text":"Analysis of values and uncertainty\nCost-effectiveness modelling serves two purposes. First, it serves as an estimation of expected costs and outcomes for medical decision-making. Secondly, it assesses the uncertainties around these estimates and its range of validity [36].\nThe expected costs and outcomes can be obtained by multiplying the probabilities with their relevant costs and health outcomes. This results in average expected values per patient, for costs as well as health outcome. Specific software (e.g., TreeAgePro, TreeAge Software, Williamstown, MA, U.S.) can be used for this purpose, but Markov models and decision trees are also often analyzed using standard PC software (Microsoft Excel). The quality of estimates derived from the model directly depends on the quality of the input parameters. In general, the input parameters are desired to be as evidence-based as possible. However, model input parameters are surrounded by uncertainties. Parameter uncertainty reflects the uncertainty of the model input parameters because we do not know the precise values. This can be a result of multiple and conflicting or low quality studies, or merely from variation in the data as reflected in standard deviations surrounding a mean estimate. Parameter uncertainty can be addressed by a deterministic or probabilistic sensitivity analysis [36]. In a deterministic sensitivity analysis the mean values of the input parameters are altered to assess impact on total cost and outcome of the model. Even in the case when input parameters of the model might not be available, hypothetical values and borders can be set in the model to determine hypothetical estimates.\nAlternatively, parameter uncertainty can be addressed by means of a probabilistic sensitivity analysis. Each uncertain model input parameter is assigned a distribution, based on the mean and standard deviation/error as found in the literature. Examples of distributions surrounding the input parameters can be found in Table 1. In the probabilistic sensitivity analysis, different samples are taken from these distributions. For each sample, the hypothetical cohort runs through the model based on these sampled probabilities, and costs and outcome are derived. This results in a range of estimates of costs and outcome, representing the uncertainty in the result of the cost-effectiveness estimation. This range in outcome is usually expressed as 95 % Confidence Intervals (CI), based on the probabilistic analysis. In our example, the expected costs per patient are € 15,266 (95 % CI € 14,072–16,440) and expected QALYs 1.30 (95 % CI 1.00–1.61), if all patients were to receive PET-CT at three months follow-up."}
2_test
{"project":"2_test","denotations":[{"id":"26003789-22990087-29373368","span":{"begin":269,"end":271},"obj":"22990087"},{"id":"26003789-22990087-29373369","span":{"begin":1352,"end":1354},"obj":"22990087"}],"text":"Analysis of values and uncertainty\nCost-effectiveness modelling serves two purposes. First, it serves as an estimation of expected costs and outcomes for medical decision-making. Secondly, it assesses the uncertainties around these estimates and its range of validity [36].\nThe expected costs and outcomes can be obtained by multiplying the probabilities with their relevant costs and health outcomes. This results in average expected values per patient, for costs as well as health outcome. Specific software (e.g., TreeAgePro, TreeAge Software, Williamstown, MA, U.S.) can be used for this purpose, but Markov models and decision trees are also often analyzed using standard PC software (Microsoft Excel). The quality of estimates derived from the model directly depends on the quality of the input parameters. In general, the input parameters are desired to be as evidence-based as possible. However, model input parameters are surrounded by uncertainties. Parameter uncertainty reflects the uncertainty of the model input parameters because we do not know the precise values. This can be a result of multiple and conflicting or low quality studies, or merely from variation in the data as reflected in standard deviations surrounding a mean estimate. Parameter uncertainty can be addressed by a deterministic or probabilistic sensitivity analysis [36]. In a deterministic sensitivity analysis the mean values of the input parameters are altered to assess impact on total cost and outcome of the model. Even in the case when input parameters of the model might not be available, hypothetical values and borders can be set in the model to determine hypothetical estimates.\nAlternatively, parameter uncertainty can be addressed by means of a probabilistic sensitivity analysis. Each uncertain model input parameter is assigned a distribution, based on the mean and standard deviation/error as found in the literature. Examples of distributions surrounding the input parameters can be found in Table 1. In the probabilistic sensitivity analysis, different samples are taken from these distributions. For each sample, the hypothetical cohort runs through the model based on these sampled probabilities, and costs and outcome are derived. This results in a range of estimates of costs and outcome, representing the uncertainty in the result of the cost-effectiveness estimation. This range in outcome is usually expressed as 95 % Confidence Intervals (CI), based on the probabilistic analysis. In our example, the expected costs per patient are € 15,266 (95 % CI € 14,072–16,440) and expected QALYs 1.30 (95 % CI 1.00–1.61), if all patients were to receive PET-CT at three months follow-up."}