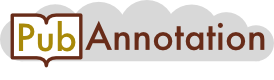
PMC:4620161 / 50523-56218
Annnotations
{"target":"http://pubannotation.org/docs/sourcedb/PMC/sourceid/4620161","sourcedb":"PMC","sourceid":"4620161","source_url":"https://www.ncbi.nlm.nih.gov/pmc/4620161","text":"Overview of the testing methodologies\nAmong all the testing strategies for ESM (Table 1), MVT and LME maintain an accurate characterization for the hypothesis. In contrast, the dimensional reduction methods AUC, L2D, and EXC (XUV and XMV) project the original space of the alternative hypothesis from ℝm to ℝ1, ℝ1, and ℝm−1, respectively. Any dimensional reduction usually translates to information loss or geometrical distortion. Based on the results from our simulations and real data applications, we believe that the major testing methods for ESM are LME, XUV, MVT, XMV, and AUC, which all have the proper controllability for FPR. If sample size is not an issue in FMRI studies, MVT (e.g., hypothesis 2a or 2b) would be the most accurate approach in terms of hypothesis characterization. However, in practice the number of subjects is usually not large enough for MVT due to resource limitations (e.g., financial cost, time, and manpower), leading to an underpowered performance of MVT as shown in our simulations and real data. Among all the workaround methods through dimensional reduction, XUV has the least hypothesis distortion and the lowest power loss. With one group of subjects and no other explanatory variables present, XUV surpasses MVT, XMV, and AUC in power. However, with an accurate representation of the hypothesis, LME is slightly more efficient than XUV, and should be considered as the first choice (e.g., Alvarez et al., 2008). For all other situations, LME modeling is not feasible due to the constraint of variable parameterization, and we opt for the workaround methods through dimensional reduction, among which AUC is insensitive to subtle shape differences while XMV mostly underperforms unless when the temporal correlation is relatively high (e.g., 0.65 or higher; Chen et al., 2014). XUV achieves the best balance between dimensional reduction and statistical power. However, as XUV tests for parallelism, not exactly the same as the accurate representation characterized in MVT, it may fail in detecting the situation where the HDR profiles are roughly parallel. To compensate for the occasions when XUV fails, other dimensional reduction methods (MVT, AUC, XMV) may offer some complementary detection power.\nIn light of the discussion here, we strongly encourage the adoption of the ESM approach to achieving two goals: detecting activations and estimating the hemodynamics by characterizing the HDR shape. In addition to the large power gain at both individual and group levels, ESM provides the estimated HDR shape information at the group level, providing an extra layer of validation about the effect veracity through the graphical display of the familiar HDR shape, and alleviating the misconceptions and malpractices prevalent in statistical analysis (e.g., P-hacking, graphical presentation of statistic values instead of effect estimates, overuse of statistical significance; Motulsky, 2014). The HDR profile information from ESM offers a precious boost especially when a cluster fails to survive the typical stringent thresholding for multiple testing correction but still reaches the significance level of 0.05 at the voxel level. Such a reassuring support of ESM is unavailable from the alternatives of FSM and ASM, with which typically the investigator would be only able to report the peak HDR magnitude or statistic values at a region.\nOur recommendation of adopting ESM not only applies to event-related experiments, but also are adaptable to modeling the attenuation or habituation effect in block designs (Saad et al., 2006). In addition, this approximation modeling methodology of XUV assisted with MVT, AUC, and XMV has been applied to DTI data in which the simultaneous variables (white matter network groups such as corpus callosum, corona radiata, left and right hemispheric projection fibers, left and right hemispheric association fibers) were modeled by multiple explanatory variables (e.g., sex, age, behavioral measures) for each response variable such as fractional anisotropy, axial diffusivity, mean diffusivity, radial diffusivity, T1 relaxation time, proton density, and volume (Taylor et al., 2015).\nThe proposed modeling strategies have been implemented into the open-source program 3dMVM in AFNI, which offers the investigator all the testing results in the output including XUV and the auxiliary approaches (MVT, XMV, and AUC). MVT for the components from ESM presents a unique challenge when one or more within-subject factors are included in the model, and we offer a testing strategy that still fits in the MVM framework (Appendix B). As an alternative, these tests could be conducted in the traditional univariate GLM except for the two multivariate methods, MVT and XMV. In other words, some of the testing methods (MVT and XMV) are truly multivariate, while others (XUV, AUV, and L2D) are essentially univariate. However, as we demonstrated in Chen et al. (2014), these univariate tests are sometimes difficult to perform under the univariate framework, as shown by the implementation challenges faced by some of the neuroimaging packages. Instead, these univariate tests can be more conveniently formulated under the MVM platform by treating the levels of each within subject factor as simultaneous variables in (1) and then constructing the univariate testing statistics through a conversion process. For example, those univariate tests presented in Figure 2 cannot be performed under the univariate GLM framework due to the incorporation of a covariate (age) in the presence of two within subject factors (Condition and HDR effects). It is in this sense that we frame our discussion here under the MVM perspective.","divisions":[{"label":"title","span":{"begin":0,"end":37}},{"label":"p","span":{"begin":38,"end":2243}},{"label":"p","span":{"begin":2244,"end":3385}},{"label":"p","span":{"begin":3386,"end":4168}}],"tracks":[{"project":"0_colil","denotations":[{"id":"26578853-18550763-358050","span":{"begin":1446,"end":1450},"obj":"18550763"},{"id":"26578853-24954281-358051","span":{"begin":1811,"end":1815},"obj":"24954281"},{"id":"26578853-25204545-358052","span":{"begin":2930,"end":2934},"obj":"25204545"},{"id":"26578853-16568421-358053","span":{"begin":3572,"end":3576},"obj":"16568421"},{"id":"26578853-25182535-358054","span":{"begin":4162,"end":4166},"obj":"25182535"},{"id":"26578853-24954281-358055","span":{"begin":4935,"end":4939},"obj":"24954281"}],"attributes":[{"subj":"26578853-18550763-358050","pred":"source","obj":"0_colil"},{"subj":"26578853-24954281-358051","pred":"source","obj":"0_colil"},{"subj":"26578853-25204545-358052","pred":"source","obj":"0_colil"},{"subj":"26578853-16568421-358053","pred":"source","obj":"0_colil"},{"subj":"26578853-25182535-358054","pred":"source","obj":"0_colil"},{"subj":"26578853-24954281-358055","pred":"source","obj":"0_colil"}]},{"project":"TEST0","denotations":[{"id":"26578853-169-177-358050","span":{"begin":1446,"end":1450},"obj":"[\"18550763\"]"},{"id":"26578853-232-240-358051","span":{"begin":1811,"end":1815},"obj":"[\"24954281\"]"},{"id":"26578853-234-242-358052","span":{"begin":2930,"end":2934},"obj":"[\"25204545\"]"},{"id":"26578853-186-194-358053","span":{"begin":3572,"end":3576},"obj":"[\"16568421\"]"},{"id":"26578853-226-234-358054","span":{"begin":4162,"end":4166},"obj":"[\"25182535\"]"},{"id":"26578853-44-52-358055","span":{"begin":4935,"end":4939},"obj":"[\"24954281\"]"}],"attributes":[{"subj":"26578853-169-177-358050","pred":"source","obj":"TEST0"},{"subj":"26578853-232-240-358051","pred":"source","obj":"TEST0"},{"subj":"26578853-234-242-358052","pred":"source","obj":"TEST0"},{"subj":"26578853-186-194-358053","pred":"source","obj":"TEST0"},{"subj":"26578853-226-234-358054","pred":"source","obj":"TEST0"},{"subj":"26578853-44-52-358055","pred":"source","obj":"TEST0"}]},{"project":"2_test","denotations":[{"id":"26578853-18550763-38285039","span":{"begin":1446,"end":1450},"obj":"18550763"},{"id":"26578853-24954281-38285040","span":{"begin":1811,"end":1815},"obj":"24954281"},{"id":"26578853-25204545-38285041","span":{"begin":2930,"end":2934},"obj":"25204545"},{"id":"26578853-16568421-38285042","span":{"begin":3572,"end":3576},"obj":"16568421"},{"id":"26578853-25182535-38285043","span":{"begin":4162,"end":4166},"obj":"25182535"},{"id":"26578853-24954281-38285044","span":{"begin":4935,"end":4939},"obj":"24954281"}],"attributes":[{"subj":"26578853-18550763-38285039","pred":"source","obj":"2_test"},{"subj":"26578853-24954281-38285040","pred":"source","obj":"2_test"},{"subj":"26578853-25204545-38285041","pred":"source","obj":"2_test"},{"subj":"26578853-16568421-38285042","pred":"source","obj":"2_test"},{"subj":"26578853-25182535-38285043","pred":"source","obj":"2_test"},{"subj":"26578853-24954281-38285044","pred":"source","obj":"2_test"}]}],"config":{"attribute types":[{"pred":"source","value type":"selection","values":[{"id":"0_colil","color":"#cbec93","default":true},{"id":"TEST0","color":"#ec93e5"},{"id":"2_test","color":"#93ecd8"}]}]}}