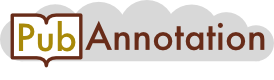
PMC:4620161 / 47986-50521
Annnotations
0_colil
{"project":"0_colil","denotations":[{"id":"26578853-16568421-358046","span":{"begin":1428,"end":1432},"obj":"16568421"},{"id":"26578853-24252847-358047","span":{"begin":1447,"end":1451},"obj":"24252847"},{"id":"26578853-15050587-358048","span":{"begin":2007,"end":2011},"obj":"15050587"},{"id":"26578853-22431587-358049","span":{"begin":2039,"end":2043},"obj":"22431587"}],"text":"There are many characteristics that could describe the HDR shape: onset latency, onset-to-peak, peak location, peak duration, magnitude or shape of the undershoot after the onset or during the recovery phase, and habituation or saturation effect. Because of the multiple facets of HDR shape, a lot of effects may well have gone undetected at both individual and group levels in most neuroimaging data analyses, and the failures to capture the shape nuances might have partially contributed to the poor reliability and reproducibility in the field. With a few exceptions, most analyses adopt FSM or ASM mainly for the simplicity of group analysis, as each condition or task is associated with one effect estimate, while other coefficients (e.g., time and dispersion derivatives in ASM) are a priori ignored. That is, activation detection intuitively focuses on the estimated magnitude around the activation peak while statistical inference on the whole HDR shape is generally considered a daunting hurdle. FSM may work well for situations such as a contrast between a condition and fixation. However, it would fail to detect shape subtleties such as prolonged plateau at the peak, slower or faster rise or fall, bigger or longer undershoot, or overall duration. Therefore, FSM through a presumed HDR (gamma variate in AFNI, canonical function in FSL and SPM) is very crude even in an experiment with a block design (Saad et al., 2006; Shan et al., 2013). ASM is an improvement over FSM; however, its flexibility is still limited. For instance, when one is interested in contrasting two conditions (or groups) or in investigating higher-order interactions, the three ASM basis functions may still not be enough in capturing the undershoot subtleties. In addition, characterizing the whole HDR curve with its peak value from ASM for group analysis may suffer from significant power loss, as demonstrated in our real experimental data. Response shapes can vary considerably over space (e.g., Handwerker et al., 2004; Gonzalez-Castillo et al., 2012; Badillo et al., 2013), and we believe it is important to model more accurately the HDRs at the individual level and test for shape rather just amplitude at the group level, particularly when detecting subtle differences between conditions or groups. The dominant adoption of FSM or ASM with a relatively rigid HDR shape reflects the daunting challenge in adopting ESM at the group level, and it is this challenge that motivated our exploration of various group analysis strategies with ESM."}
TEST0
{"project":"TEST0","denotations":[{"id":"26578853-167-175-358046","span":{"begin":1428,"end":1432},"obj":"[\"16568421\"]"},{"id":"26578853-186-194-358047","span":{"begin":1447,"end":1451},"obj":"[\"24252847\"]"},{"id":"26578853-75-83-358048","span":{"begin":2007,"end":2011},"obj":"[\"15050587\"]"},{"id":"26578853-107-115-358049","span":{"begin":2039,"end":2043},"obj":"[\"22431587\"]"}],"text":"There are many characteristics that could describe the HDR shape: onset latency, onset-to-peak, peak location, peak duration, magnitude or shape of the undershoot after the onset or during the recovery phase, and habituation or saturation effect. Because of the multiple facets of HDR shape, a lot of effects may well have gone undetected at both individual and group levels in most neuroimaging data analyses, and the failures to capture the shape nuances might have partially contributed to the poor reliability and reproducibility in the field. With a few exceptions, most analyses adopt FSM or ASM mainly for the simplicity of group analysis, as each condition or task is associated with one effect estimate, while other coefficients (e.g., time and dispersion derivatives in ASM) are a priori ignored. That is, activation detection intuitively focuses on the estimated magnitude around the activation peak while statistical inference on the whole HDR shape is generally considered a daunting hurdle. FSM may work well for situations such as a contrast between a condition and fixation. However, it would fail to detect shape subtleties such as prolonged plateau at the peak, slower or faster rise or fall, bigger or longer undershoot, or overall duration. Therefore, FSM through a presumed HDR (gamma variate in AFNI, canonical function in FSL and SPM) is very crude even in an experiment with a block design (Saad et al., 2006; Shan et al., 2013). ASM is an improvement over FSM; however, its flexibility is still limited. For instance, when one is interested in contrasting two conditions (or groups) or in investigating higher-order interactions, the three ASM basis functions may still not be enough in capturing the undershoot subtleties. In addition, characterizing the whole HDR curve with its peak value from ASM for group analysis may suffer from significant power loss, as demonstrated in our real experimental data. Response shapes can vary considerably over space (e.g., Handwerker et al., 2004; Gonzalez-Castillo et al., 2012; Badillo et al., 2013), and we believe it is important to model more accurately the HDRs at the individual level and test for shape rather just amplitude at the group level, particularly when detecting subtle differences between conditions or groups. The dominant adoption of FSM or ASM with a relatively rigid HDR shape reflects the daunting challenge in adopting ESM at the group level, and it is this challenge that motivated our exploration of various group analysis strategies with ESM."}
2_test
{"project":"2_test","denotations":[{"id":"26578853-16568421-38285035","span":{"begin":1428,"end":1432},"obj":"16568421"},{"id":"26578853-24252847-38285036","span":{"begin":1447,"end":1451},"obj":"24252847"},{"id":"26578853-15050587-38285037","span":{"begin":2007,"end":2011},"obj":"15050587"},{"id":"26578853-22431587-38285038","span":{"begin":2039,"end":2043},"obj":"22431587"}],"text":"There are many characteristics that could describe the HDR shape: onset latency, onset-to-peak, peak location, peak duration, magnitude or shape of the undershoot after the onset or during the recovery phase, and habituation or saturation effect. Because of the multiple facets of HDR shape, a lot of effects may well have gone undetected at both individual and group levels in most neuroimaging data analyses, and the failures to capture the shape nuances might have partially contributed to the poor reliability and reproducibility in the field. With a few exceptions, most analyses adopt FSM or ASM mainly for the simplicity of group analysis, as each condition or task is associated with one effect estimate, while other coefficients (e.g., time and dispersion derivatives in ASM) are a priori ignored. That is, activation detection intuitively focuses on the estimated magnitude around the activation peak while statistical inference on the whole HDR shape is generally considered a daunting hurdle. FSM may work well for situations such as a contrast between a condition and fixation. However, it would fail to detect shape subtleties such as prolonged plateau at the peak, slower or faster rise or fall, bigger or longer undershoot, or overall duration. Therefore, FSM through a presumed HDR (gamma variate in AFNI, canonical function in FSL and SPM) is very crude even in an experiment with a block design (Saad et al., 2006; Shan et al., 2013). ASM is an improvement over FSM; however, its flexibility is still limited. For instance, when one is interested in contrasting two conditions (or groups) or in investigating higher-order interactions, the three ASM basis functions may still not be enough in capturing the undershoot subtleties. In addition, characterizing the whole HDR curve with its peak value from ASM for group analysis may suffer from significant power loss, as demonstrated in our real experimental data. Response shapes can vary considerably over space (e.g., Handwerker et al., 2004; Gonzalez-Castillo et al., 2012; Badillo et al., 2013), and we believe it is important to model more accurately the HDRs at the individual level and test for shape rather just amplitude at the group level, particularly when detecting subtle differences between conditions or groups. The dominant adoption of FSM or ASM with a relatively rigid HDR shape reflects the daunting challenge in adopting ESM at the group level, and it is this challenge that motivated our exploration of various group analysis strategies with ESM."}