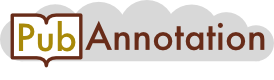
PMC:4620161 / 47975-66338
Annnotations
0_colil
{"project":"0_colil","denotations":[{"id":"26578853-16568421-358046","span":{"begin":1439,"end":1443},"obj":"16568421"},{"id":"26578853-24252847-358047","span":{"begin":1458,"end":1462},"obj":"24252847"},{"id":"26578853-15050587-358048","span":{"begin":2018,"end":2022},"obj":"15050587"},{"id":"26578853-22431587-358049","span":{"begin":2050,"end":2054},"obj":"22431587"},{"id":"26578853-18550763-358050","span":{"begin":3994,"end":3998},"obj":"18550763"},{"id":"26578853-24954281-358051","span":{"begin":4359,"end":4363},"obj":"24954281"},{"id":"26578853-25204545-358052","span":{"begin":5478,"end":5482},"obj":"25204545"},{"id":"26578853-16568421-358053","span":{"begin":6120,"end":6124},"obj":"16568421"},{"id":"26578853-25182535-358054","span":{"begin":6710,"end":6714},"obj":"25182535"},{"id":"26578853-24954281-358055","span":{"begin":7483,"end":7487},"obj":"24954281"},{"id":"26578853-11771969-358056","span":{"begin":8504,"end":8508},"obj":"11771969"},{"id":"26578853-15050594-358057","span":{"begin":8527,"end":8531},"obj":"15050594"},{"id":"26578853-22245637-358058","span":{"begin":8546,"end":8550},"obj":"22245637"},{"id":"26578853-15110015-358059","span":{"begin":11074,"end":11078},"obj":"15110015"},{"id":"26578853-16125978-358060","span":{"begin":11100,"end":11104},"obj":"16125978"},{"id":"26578853-19913625-358061","span":{"begin":11124,"end":11128},"obj":"19913625"},{"id":"26578853-22227535-358062","span":{"begin":11262,"end":11266},"obj":"22227535"},{"id":"26578853-16125978-358063","span":{"begin":11350,"end":11354},"obj":"16125978"},{"id":"26578853-15110015-358064","span":{"begin":11535,"end":11539},"obj":"15110015"},{"id":"26578853-14614810-358065","span":{"begin":12867,"end":12871},"obj":"14614810"},{"id":"26578853-17690338-358066","span":{"begin":12889,"end":12893},"obj":"17690338"},{"id":"26578853-24198784-358067","span":{"begin":12917,"end":12921},"obj":"24198784"},{"id":"26578853-16767087-358068","span":{"begin":13166,"end":13170},"obj":"16767087"},{"id":"26578853-17292627-358069","span":{"begin":13186,"end":13190},"obj":"17292627"},{"id":"26578853-18245043-358070","span":{"begin":13207,"end":13211},"obj":"18245043"},{"id":"26578853-12029136-358071","span":{"begin":13397,"end":13401},"obj":"12029136"},{"id":"26578853-18245043-358072","span":{"begin":13418,"end":13422},"obj":"18245043"},{"id":"26578853-24215974-358073","span":{"begin":13440,"end":13444},"obj":"24215974"},{"id":"26578853-18245043-358074","span":{"begin":13531,"end":13535},"obj":"18245043"},{"id":"26578853-19084070-358075","span":{"begin":13947,"end":13951},"obj":"19084070"},{"id":"26578853-19084070-358076","span":{"begin":14533,"end":14537},"obj":"19084070"},{"id":"26578853-22982104-358077","span":{"begin":14892,"end":14896},"obj":"22982104"},{"id":"26578853-23473935-358078","span":{"begin":15442,"end":15446},"obj":"23473935"},{"id":"26578853-24793829-358079","span":{"begin":16451,"end":16455},"obj":"24793829"},{"id":"26578853-16281292-358080","span":{"begin":17081,"end":17085},"obj":"16281292"},{"id":"26578853-14552578-358081","span":{"begin":17350,"end":17354},"obj":"14552578"},{"id":"26578853-24793829-358082","span":{"begin":17425,"end":17429},"obj":"24793829"},{"id":"26578853-25304775-358083","span":{"begin":17660,"end":17664},"obj":"25304775"},{"id":"26578853-24793829-358084","span":{"begin":17723,"end":17727},"obj":"24793829"},{"id":"26578853-23096056-358085","span":{"begin":18044,"end":18048},"obj":"23096056"}],"text":"Discussion\nThere are many characteristics that could describe the HDR shape: onset latency, onset-to-peak, peak location, peak duration, magnitude or shape of the undershoot after the onset or during the recovery phase, and habituation or saturation effect. Because of the multiple facets of HDR shape, a lot of effects may well have gone undetected at both individual and group levels in most neuroimaging data analyses, and the failures to capture the shape nuances might have partially contributed to the poor reliability and reproducibility in the field. With a few exceptions, most analyses adopt FSM or ASM mainly for the simplicity of group analysis, as each condition or task is associated with one effect estimate, while other coefficients (e.g., time and dispersion derivatives in ASM) are a priori ignored. That is, activation detection intuitively focuses on the estimated magnitude around the activation peak while statistical inference on the whole HDR shape is generally considered a daunting hurdle. FSM may work well for situations such as a contrast between a condition and fixation. However, it would fail to detect shape subtleties such as prolonged plateau at the peak, slower or faster rise or fall, bigger or longer undershoot, or overall duration. Therefore, FSM through a presumed HDR (gamma variate in AFNI, canonical function in FSL and SPM) is very crude even in an experiment with a block design (Saad et al., 2006; Shan et al., 2013). ASM is an improvement over FSM; however, its flexibility is still limited. For instance, when one is interested in contrasting two conditions (or groups) or in investigating higher-order interactions, the three ASM basis functions may still not be enough in capturing the undershoot subtleties. In addition, characterizing the whole HDR curve with its peak value from ASM for group analysis may suffer from significant power loss, as demonstrated in our real experimental data. Response shapes can vary considerably over space (e.g., Handwerker et al., 2004; Gonzalez-Castillo et al., 2012; Badillo et al., 2013), and we believe it is important to model more accurately the HDRs at the individual level and test for shape rather just amplitude at the group level, particularly when detecting subtle differences between conditions or groups. The dominant adoption of FSM or ASM with a relatively rigid HDR shape reflects the daunting challenge in adopting ESM at the group level, and it is this challenge that motivated our exploration of various group analysis strategies with ESM.\n\nOverview of the testing methodologies\nAmong all the testing strategies for ESM (Table 1), MVT and LME maintain an accurate characterization for the hypothesis. In contrast, the dimensional reduction methods AUC, L2D, and EXC (XUV and XMV) project the original space of the alternative hypothesis from ℝm to ℝ1, ℝ1, and ℝm−1, respectively. Any dimensional reduction usually translates to information loss or geometrical distortion. Based on the results from our simulations and real data applications, we believe that the major testing methods for ESM are LME, XUV, MVT, XMV, and AUC, which all have the proper controllability for FPR. If sample size is not an issue in FMRI studies, MVT (e.g., hypothesis 2a or 2b) would be the most accurate approach in terms of hypothesis characterization. However, in practice the number of subjects is usually not large enough for MVT due to resource limitations (e.g., financial cost, time, and manpower), leading to an underpowered performance of MVT as shown in our simulations and real data. Among all the workaround methods through dimensional reduction, XUV has the least hypothesis distortion and the lowest power loss. With one group of subjects and no other explanatory variables present, XUV surpasses MVT, XMV, and AUC in power. However, with an accurate representation of the hypothesis, LME is slightly more efficient than XUV, and should be considered as the first choice (e.g., Alvarez et al., 2008). For all other situations, LME modeling is not feasible due to the constraint of variable parameterization, and we opt for the workaround methods through dimensional reduction, among which AUC is insensitive to subtle shape differences while XMV mostly underperforms unless when the temporal correlation is relatively high (e.g., 0.65 or higher; Chen et al., 2014). XUV achieves the best balance between dimensional reduction and statistical power. However, as XUV tests for parallelism, not exactly the same as the accurate representation characterized in MVT, it may fail in detecting the situation where the HDR profiles are roughly parallel. To compensate for the occasions when XUV fails, other dimensional reduction methods (MVT, AUC, XMV) may offer some complementary detection power.\nIn light of the discussion here, we strongly encourage the adoption of the ESM approach to achieving two goals: detecting activations and estimating the hemodynamics by characterizing the HDR shape. In addition to the large power gain at both individual and group levels, ESM provides the estimated HDR shape information at the group level, providing an extra layer of validation about the effect veracity through the graphical display of the familiar HDR shape, and alleviating the misconceptions and malpractices prevalent in statistical analysis (e.g., P-hacking, graphical presentation of statistic values instead of effect estimates, overuse of statistical significance; Motulsky, 2014). The HDR profile information from ESM offers a precious boost especially when a cluster fails to survive the typical stringent thresholding for multiple testing correction but still reaches the significance level of 0.05 at the voxel level. Such a reassuring support of ESM is unavailable from the alternatives of FSM and ASM, with which typically the investigator would be only able to report the peak HDR magnitude or statistic values at a region.\nOur recommendation of adopting ESM not only applies to event-related experiments, but also are adaptable to modeling the attenuation or habituation effect in block designs (Saad et al., 2006). In addition, this approximation modeling methodology of XUV assisted with MVT, AUC, and XMV has been applied to DTI data in which the simultaneous variables (white matter network groups such as corpus callosum, corona radiata, left and right hemispheric projection fibers, left and right hemispheric association fibers) were modeled by multiple explanatory variables (e.g., sex, age, behavioral measures) for each response variable such as fractional anisotropy, axial diffusivity, mean diffusivity, radial diffusivity, T1 relaxation time, proton density, and volume (Taylor et al., 2015).\nThe proposed modeling strategies have been implemented into the open-source program 3dMVM in AFNI, which offers the investigator all the testing results in the output including XUV and the auxiliary approaches (MVT, XMV, and AUC). MVT for the components from ESM presents a unique challenge when one or more within-subject factors are included in the model, and we offer a testing strategy that still fits in the MVM framework (Appendix B). As an alternative, these tests could be conducted in the traditional univariate GLM except for the two multivariate methods, MVT and XMV. In other words, some of the testing methods (MVT and XMV) are truly multivariate, while others (XUV, AUV, and L2D) are essentially univariate. However, as we demonstrated in Chen et al. (2014), these univariate tests are sometimes difficult to perform under the univariate framework, as shown by the implementation challenges faced by some of the neuroimaging packages. Instead, these univariate tests can be more conveniently formulated under the MVM platform by treating the levels of each within subject factor as simultaneous variables in (1) and then constructing the univariate testing statistics through a conversion process. For example, those univariate tests presented in Figure 2 cannot be performed under the univariate GLM framework due to the incorporation of a covariate (age) in the presence of two within subject factors (Condition and HDR effects). It is in this sense that we frame our discussion here under the MVM perspective.\n\nLimitations of the ESM approach\nIt is noteworthy that the reliability information from the individual subject analysis is not considered at the group level with the modeling methods discussed here, unlike the mixed-effect multilevel analysis (Worsley et al., 2002; Woolrich et al., 2004; Chen et al., 2012). In addition, the number of basis functions monotonically increases among FSM, ASM, and ESM, therefore it is expected that the goodness of fit at the individual subject analysis level improves across the three methods. On the other hand, as each condition is characterized through multiple (e.g., ≥7) basis functions in ESM, a reliable estimation of the HDR curve at the individual level pays a price through the lower degrees of freedom and requires enough (e.g., 20 or more) trials per condition, and may encounter the risk of numerical instability due to high correlations or even multicollinearity among the regressors. These latter issues can be exacerbated by poor stimulus timing designs. In addition, the typical regression analysis at the individual level assumes the linearity of HDR across trials. Although available (e.g., 3dNLfim in AFNI), a non-linear approach is usually difficult to handle and still requires some extent of prior information about the HDR shape. Furthermore, the ESM approach is generally considered to be susceptible to noise or effects unrelated to the effects of interest (e.g., head motion, physiological confounds). In other words, the confounding effects may leak into the HDR estimation through over-fitting. However, the false positives from the potential over-fitting at the individual level is less a concern at the group level for the following reasons: a) the likelihood is reduced unless most subjects systematically have similar or same confounding effects; b) cluster-based inferences may reduce the risk of false positives; and most importantly c) examination of the estimated HDR profiles offer an extra safeguard to filter out the potential false positives.\n\nComparisons with other modeling approaches\nSome (not all) of the dimensional reduction methods for ESM discussed here have been sporadically and individually applied to real data in the literature. For example, a popular practice with ASM is to solely focus on the coefficient of the principal basis function (e.g., canonical curve in SPM) with other coefficients (e.g., time and dispersion derivatives) being a priori abandoned. As our results with real data showed, the investigator may fail to detect most activations when the effect lies in the HDR shape nuances but not the peak. One suggestion for ASM was to extend the definition of amplitude in (6) to the L2-distance by including either the effect for the time derivative (7) or the effects for both time and dispersion derivatives (8) (Calhoun et al., 2004; Worsley and Taylor, 2006; Steffener et al., 2010). A similar approach was to express the effect estimates from the first two basis functions of ASM as a complex number (Wang et al., 2012). However, the potential issues with L2D or its analogs (e.g., Worsley and Taylor, 2006) are the following. a) The definition of amplitude extension in (7) and (8) is under the premise that all the three basis functions are orthogonal with each other (Calhoun et al., 2004). However, only the first two basis functions are orthogonal with each other, but not the third one. b) The second and third basis functions are not normalized; that is, they are not scaled to have a maximum value of 1, unlike the first basis function. In addition, the three effect estimates have different dimensions: the first is of percent signal change while the other two of percent signal change by the unit of time. Therefore, it is difficult to render a physically meaning interpretation with the L2D measures. c) All the effect estimates including negative values are folded into a positive L2D measure, which cannot be differentiated among those effect estimates on the same circle or sphere (see Table 1). In addition, it may lead to the violation of the Gaussian distribution assumption, as illustrated in the poor controllability of FPR (Figure 1). d) Their power performance is not satisfactory (Figures 1, 2). As an alternative, MVT or LME through the hypothesis (2a) or (2b) on the two or three effect estimates from ASM, as shown in Figure 2A, provides a more accurate characterization because it allows for different units or dimensions across the effects.\nSimilarly for ESM, two dimensional reduction methods have separately been adopted in data analyses. For example, AUC was employed in Beauchamp et al. (2003), Greene et al. (2007), and McGregor et al. (2013). Although not explicitly stated, XUV was used in several real applications to identify the HDR effect under a condition through the main effect (or one-way interaction) of the ESM components in a one-way within-subject ANOVA (Weissman et al., 2006; Geier et al., 2007; Church et al., 2008), to detect the group or condition differences in the overall HDR shape through the group-by-component or condition-by-component interaction in a two-way ANOVA (e.g., Schlaggar et al., 2002; Church et al., 2008; Shuster et al., 2014), and to explore the three-way group-by-task-by-component interaction (Church et al., 2008). However, two limitations were not addressed in those analyses: the potential identification failure of XUV (Table 1 and Voxel 2 in Figure 2), and the limited applicability of univariate GLM.\nSome comparisons were performed in terms of amplitude, peak latency, and duration in the estimated HDR among various modeling methods (e.g., FSM, L2D, ESM, a nonlinear model, and inverse logit model; Lindquist et al., 2009). The inverse logit model was deemed the best among the candidates in both simulations and real data, and slightly more powerful than ESM. However, the comparisons were not optimal. First, the dimensional reduction from the HDR shape in ℝm to the three quantities (amplitude, delay, and duration) in ℝ3 might be compromised in power when detecting the shape subtleties—this point can be highly dependent on the experiment. Secondly, the reliability for the estimation of the three characteristics was suboptimal. For example, the lackluster performance of ESM in Lindquist et al. (2009) might be caused by the inaccurate amplitude based on the first local peak because such an approach could be misleading especially when more than one local peak occurs. Lastly, the final group analyses were still focused on the amplitude with the Student's t-test, an effective dimensional reduction from ℝm to ℝ1.\nA multivariate approach (Zhang et al., 2012) was previously proposed, analogous to our method except for the following differences. It was demonstrated among the voxels within only five structurally pre-defined regions; smoothing the estimated HDR from each subject by a Gaussian kernel and imposing regularization on the smoothed HDR were performed to improve the temporal continuities of the HDR; and group analysis was run through multivariate testing of one-sample or pair-wise comparisons among conditions, equivalent to MVT (2a or 2b) discussed here. Another approach (Zhang et al., 2013) assumed that the HDR under each condition would only vary in amplitude and latency across subjects; that is, the HDR shape was presumed same across all subjects. Specifically, the HDR curve for each condition was characterized at the group level by two parameters: one was of interest (amplitude) and the other of no interest (delay). In addition, the HDR shape (fixed across subjects) was modeled by cubic splines plus their time derivatives. Once the amplitude was estimated for each subject in a one-tier model that incorporated both within- and across-subject variances, a second round of group analysis was performed only on the amplitudes (ignoring the delay) through typical one-sample or paired t-test to make inference about a condition or contrast. The approach was demonstrated among the voxels within only three structurally predefined regions.\nRecently, a hierarchical approach was proposed for ESM through integrating both individual and group levels into one model (Degras and Lindquist, 2014) in which the HDR curves were captured through multiple higher-order B-spline functions. Even though only demonstrated on one slice of data, the approach is appealing because the variability at both levels is accounted for. However, the current implementation in Matlab is hindered by the following constraints or limitations. a) Spatial parcellation based on anatomical structure was required to determine the temporal correlation structure in the noise component. More applicable approaches would be based on a priori regions that are functionally parcellated through, for example, hierarchical clustering (Thirion et al., 2006; Ji, 2010), joint parcellation detection-estimation (Badillo et al., 2014), consensus clustering (Badillo et al., 2013), k-means clustering (Ji, 2010), etc. (b) The HDR shape may vary across different stimulus conditions under some scenarios (e.g., Ciuciu et al., 2003), and a presumption of the same shape HDR as in Degras and Lindquist (2014) may decrease the detection power when the shape subtleties are of interest. The same HDR assumption is reasonable under other circumstances and has proven sufficient for encoding or decoding the brain activity (Pedregosa et al., 2015). c) Final statistical inference in Degras and Lindquist (2014) through an asymptotic t-test was still based on the scaling factors of the same HDR curve shared by all conditions, a dimensional reduction approach from ℝm to ℝ1. An alternative approach is the incorporation of both individual and group levels in a mixed-effects model under the Bayesian framework (Chaari et al., 2013; Badillo et al., 2014). Applied at a priori regions that are functionally parcellated, this jointed detection and estimation method may render a robust procedure less sensitive to outliers than the conventional two-tier methods under the assumption that all the voxels share the same HDR within a region or parcel."}
TEST0
{"project":"TEST0","denotations":[{"id":"26578853-167-175-358046","span":{"begin":1439,"end":1443},"obj":"[\"16568421\"]"},{"id":"26578853-186-194-358047","span":{"begin":1458,"end":1462},"obj":"[\"24252847\"]"},{"id":"26578853-75-83-358048","span":{"begin":2018,"end":2022},"obj":"[\"15050587\"]"},{"id":"26578853-107-115-358049","span":{"begin":2050,"end":2054},"obj":"[\"22431587\"]"},{"id":"26578853-169-177-358050","span":{"begin":3994,"end":3998},"obj":"[\"18550763\"]"},{"id":"26578853-232-240-358051","span":{"begin":4359,"end":4363},"obj":"[\"24954281\"]"},{"id":"26578853-234-242-358052","span":{"begin":5478,"end":5482},"obj":"[\"25204545\"]"},{"id":"26578853-186-194-358053","span":{"begin":6120,"end":6124},"obj":"[\"16568421\"]"},{"id":"26578853-226-234-358054","span":{"begin":6710,"end":6714},"obj":"[\"25182535\"]"},{"id":"26578853-44-52-358055","span":{"begin":7483,"end":7487},"obj":"[\"24954281\"]"},{"id":"26578853-227-235-358056","span":{"begin":8504,"end":8508},"obj":"[\"11771969\"]"},{"id":"26578853-233-241-358057","span":{"begin":8527,"end":8531},"obj":"[\"15050594\"]"},{"id":"26578853-231-239-358058","span":{"begin":8546,"end":8550},"obj":"[\"22245637\"]"},{"id":"26578853-227-235-358059","span":{"begin":11074,"end":11078},"obj":"[\"15110015\"]"},{"id":"26578853-234-242-358060","span":{"begin":11100,"end":11104},"obj":"[\"16125978\"]"},{"id":"26578853-225-233-358061","span":{"begin":11124,"end":11128},"obj":"[\"19913625\"]"},{"id":"26578853-131-139-358062","span":{"begin":11262,"end":11266},"obj":"[\"22227535\"]"},{"id":"26578853-81-89-358063","span":{"begin":11350,"end":11354},"obj":"[\"16125978\"]"},{"id":"26578853-160-168-358064","span":{"begin":11535,"end":11539},"obj":"[\"15110015\"]"},{"id":"26578853-51-59-358065","span":{"begin":12867,"end":12871},"obj":"[\"14614810\"]"},{"id":"26578853-73-81-358066","span":{"begin":12889,"end":12893},"obj":"[\"17690338\"]"},{"id":"26578853-101-109-358067","span":{"begin":12917,"end":12921},"obj":"[\"24198784\"]"},{"id":"26578853-233-241-358068","span":{"begin":13166,"end":13170},"obj":"[\"16767087\"]"},{"id":"26578853-230-238-358069","span":{"begin":13186,"end":13190},"obj":"[\"17292627\"]"},{"id":"26578853-235-243-358070","span":{"begin":13207,"end":13211},"obj":"[\"18245043\"]"},{"id":"26578853-231-239-358071","span":{"begin":13397,"end":13401},"obj":"[\"12029136\"]"},{"id":"26578853-232-240-358072","span":{"begin":13418,"end":13422},"obj":"[\"18245043\"]"},{"id":"26578853-233-241-358073","span":{"begin":13440,"end":13444},"obj":"[\"24215974\"]"},{"id":"26578853-235-243-358074","span":{"begin":13531,"end":13535},"obj":"[\"18245043\"]"},{"id":"26578853-218-226-358075","span":{"begin":13947,"end":13951},"obj":"[\"19084070\"]"},{"id":"26578853-68-76-358076","span":{"begin":14533,"end":14537},"obj":"[\"19084070\"]"},{"id":"26578853-39-47-358077","span":{"begin":14892,"end":14896},"obj":"[\"22982104\"]"},{"id":"26578853-32-40-358078","span":{"begin":15442,"end":15446},"obj":"[\"23473935\"]"},{"id":"26578853-146-154-358079","span":{"begin":16451,"end":16455},"obj":"[\"24793829\"]"},{"id":"26578853-159-167-358080","span":{"begin":17081,"end":17085},"obj":"[\"16281292\"]"},{"id":"26578853-107-115-358081","span":{"begin":17350,"end":17354},"obj":"[\"14552578\"]"},{"id":"26578853-182-190-358082","span":{"begin":17425,"end":17429},"obj":"[\"24793829\"]"},{"id":"26578853-153-161-358083","span":{"begin":17660,"end":17664},"obj":"[\"25304775\"]"},{"id":"26578853-56-64-358084","span":{"begin":17723,"end":17727},"obj":"[\"24793829\"]"},{"id":"26578853-151-159-358085","span":{"begin":18044,"end":18048},"obj":"[\"23096056\"]"}],"text":"Discussion\nThere are many characteristics that could describe the HDR shape: onset latency, onset-to-peak, peak location, peak duration, magnitude or shape of the undershoot after the onset or during the recovery phase, and habituation or saturation effect. Because of the multiple facets of HDR shape, a lot of effects may well have gone undetected at both individual and group levels in most neuroimaging data analyses, and the failures to capture the shape nuances might have partially contributed to the poor reliability and reproducibility in the field. With a few exceptions, most analyses adopt FSM or ASM mainly for the simplicity of group analysis, as each condition or task is associated with one effect estimate, while other coefficients (e.g., time and dispersion derivatives in ASM) are a priori ignored. That is, activation detection intuitively focuses on the estimated magnitude around the activation peak while statistical inference on the whole HDR shape is generally considered a daunting hurdle. FSM may work well for situations such as a contrast between a condition and fixation. However, it would fail to detect shape subtleties such as prolonged plateau at the peak, slower or faster rise or fall, bigger or longer undershoot, or overall duration. Therefore, FSM through a presumed HDR (gamma variate in AFNI, canonical function in FSL and SPM) is very crude even in an experiment with a block design (Saad et al., 2006; Shan et al., 2013). ASM is an improvement over FSM; however, its flexibility is still limited. For instance, when one is interested in contrasting two conditions (or groups) or in investigating higher-order interactions, the three ASM basis functions may still not be enough in capturing the undershoot subtleties. In addition, characterizing the whole HDR curve with its peak value from ASM for group analysis may suffer from significant power loss, as demonstrated in our real experimental data. Response shapes can vary considerably over space (e.g., Handwerker et al., 2004; Gonzalez-Castillo et al., 2012; Badillo et al., 2013), and we believe it is important to model more accurately the HDRs at the individual level and test for shape rather just amplitude at the group level, particularly when detecting subtle differences between conditions or groups. The dominant adoption of FSM or ASM with a relatively rigid HDR shape reflects the daunting challenge in adopting ESM at the group level, and it is this challenge that motivated our exploration of various group analysis strategies with ESM.\n\nOverview of the testing methodologies\nAmong all the testing strategies for ESM (Table 1), MVT and LME maintain an accurate characterization for the hypothesis. In contrast, the dimensional reduction methods AUC, L2D, and EXC (XUV and XMV) project the original space of the alternative hypothesis from ℝm to ℝ1, ℝ1, and ℝm−1, respectively. Any dimensional reduction usually translates to information loss or geometrical distortion. Based on the results from our simulations and real data applications, we believe that the major testing methods for ESM are LME, XUV, MVT, XMV, and AUC, which all have the proper controllability for FPR. If sample size is not an issue in FMRI studies, MVT (e.g., hypothesis 2a or 2b) would be the most accurate approach in terms of hypothesis characterization. However, in practice the number of subjects is usually not large enough for MVT due to resource limitations (e.g., financial cost, time, and manpower), leading to an underpowered performance of MVT as shown in our simulations and real data. Among all the workaround methods through dimensional reduction, XUV has the least hypothesis distortion and the lowest power loss. With one group of subjects and no other explanatory variables present, XUV surpasses MVT, XMV, and AUC in power. However, with an accurate representation of the hypothesis, LME is slightly more efficient than XUV, and should be considered as the first choice (e.g., Alvarez et al., 2008). For all other situations, LME modeling is not feasible due to the constraint of variable parameterization, and we opt for the workaround methods through dimensional reduction, among which AUC is insensitive to subtle shape differences while XMV mostly underperforms unless when the temporal correlation is relatively high (e.g., 0.65 or higher; Chen et al., 2014). XUV achieves the best balance between dimensional reduction and statistical power. However, as XUV tests for parallelism, not exactly the same as the accurate representation characterized in MVT, it may fail in detecting the situation where the HDR profiles are roughly parallel. To compensate for the occasions when XUV fails, other dimensional reduction methods (MVT, AUC, XMV) may offer some complementary detection power.\nIn light of the discussion here, we strongly encourage the adoption of the ESM approach to achieving two goals: detecting activations and estimating the hemodynamics by characterizing the HDR shape. In addition to the large power gain at both individual and group levels, ESM provides the estimated HDR shape information at the group level, providing an extra layer of validation about the effect veracity through the graphical display of the familiar HDR shape, and alleviating the misconceptions and malpractices prevalent in statistical analysis (e.g., P-hacking, graphical presentation of statistic values instead of effect estimates, overuse of statistical significance; Motulsky, 2014). The HDR profile information from ESM offers a precious boost especially when a cluster fails to survive the typical stringent thresholding for multiple testing correction but still reaches the significance level of 0.05 at the voxel level. Such a reassuring support of ESM is unavailable from the alternatives of FSM and ASM, with which typically the investigator would be only able to report the peak HDR magnitude or statistic values at a region.\nOur recommendation of adopting ESM not only applies to event-related experiments, but also are adaptable to modeling the attenuation or habituation effect in block designs (Saad et al., 2006). In addition, this approximation modeling methodology of XUV assisted with MVT, AUC, and XMV has been applied to DTI data in which the simultaneous variables (white matter network groups such as corpus callosum, corona radiata, left and right hemispheric projection fibers, left and right hemispheric association fibers) were modeled by multiple explanatory variables (e.g., sex, age, behavioral measures) for each response variable such as fractional anisotropy, axial diffusivity, mean diffusivity, radial diffusivity, T1 relaxation time, proton density, and volume (Taylor et al., 2015).\nThe proposed modeling strategies have been implemented into the open-source program 3dMVM in AFNI, which offers the investigator all the testing results in the output including XUV and the auxiliary approaches (MVT, XMV, and AUC). MVT for the components from ESM presents a unique challenge when one or more within-subject factors are included in the model, and we offer a testing strategy that still fits in the MVM framework (Appendix B). As an alternative, these tests could be conducted in the traditional univariate GLM except for the two multivariate methods, MVT and XMV. In other words, some of the testing methods (MVT and XMV) are truly multivariate, while others (XUV, AUV, and L2D) are essentially univariate. However, as we demonstrated in Chen et al. (2014), these univariate tests are sometimes difficult to perform under the univariate framework, as shown by the implementation challenges faced by some of the neuroimaging packages. Instead, these univariate tests can be more conveniently formulated under the MVM platform by treating the levels of each within subject factor as simultaneous variables in (1) and then constructing the univariate testing statistics through a conversion process. For example, those univariate tests presented in Figure 2 cannot be performed under the univariate GLM framework due to the incorporation of a covariate (age) in the presence of two within subject factors (Condition and HDR effects). It is in this sense that we frame our discussion here under the MVM perspective.\n\nLimitations of the ESM approach\nIt is noteworthy that the reliability information from the individual subject analysis is not considered at the group level with the modeling methods discussed here, unlike the mixed-effect multilevel analysis (Worsley et al., 2002; Woolrich et al., 2004; Chen et al., 2012). In addition, the number of basis functions monotonically increases among FSM, ASM, and ESM, therefore it is expected that the goodness of fit at the individual subject analysis level improves across the three methods. On the other hand, as each condition is characterized through multiple (e.g., ≥7) basis functions in ESM, a reliable estimation of the HDR curve at the individual level pays a price through the lower degrees of freedom and requires enough (e.g., 20 or more) trials per condition, and may encounter the risk of numerical instability due to high correlations or even multicollinearity among the regressors. These latter issues can be exacerbated by poor stimulus timing designs. In addition, the typical regression analysis at the individual level assumes the linearity of HDR across trials. Although available (e.g., 3dNLfim in AFNI), a non-linear approach is usually difficult to handle and still requires some extent of prior information about the HDR shape. Furthermore, the ESM approach is generally considered to be susceptible to noise or effects unrelated to the effects of interest (e.g., head motion, physiological confounds). In other words, the confounding effects may leak into the HDR estimation through over-fitting. However, the false positives from the potential over-fitting at the individual level is less a concern at the group level for the following reasons: a) the likelihood is reduced unless most subjects systematically have similar or same confounding effects; b) cluster-based inferences may reduce the risk of false positives; and most importantly c) examination of the estimated HDR profiles offer an extra safeguard to filter out the potential false positives.\n\nComparisons with other modeling approaches\nSome (not all) of the dimensional reduction methods for ESM discussed here have been sporadically and individually applied to real data in the literature. For example, a popular practice with ASM is to solely focus on the coefficient of the principal basis function (e.g., canonical curve in SPM) with other coefficients (e.g., time and dispersion derivatives) being a priori abandoned. As our results with real data showed, the investigator may fail to detect most activations when the effect lies in the HDR shape nuances but not the peak. One suggestion for ASM was to extend the definition of amplitude in (6) to the L2-distance by including either the effect for the time derivative (7) or the effects for both time and dispersion derivatives (8) (Calhoun et al., 2004; Worsley and Taylor, 2006; Steffener et al., 2010). A similar approach was to express the effect estimates from the first two basis functions of ASM as a complex number (Wang et al., 2012). However, the potential issues with L2D or its analogs (e.g., Worsley and Taylor, 2006) are the following. a) The definition of amplitude extension in (7) and (8) is under the premise that all the three basis functions are orthogonal with each other (Calhoun et al., 2004). However, only the first two basis functions are orthogonal with each other, but not the third one. b) The second and third basis functions are not normalized; that is, they are not scaled to have a maximum value of 1, unlike the first basis function. In addition, the three effect estimates have different dimensions: the first is of percent signal change while the other two of percent signal change by the unit of time. Therefore, it is difficult to render a physically meaning interpretation with the L2D measures. c) All the effect estimates including negative values are folded into a positive L2D measure, which cannot be differentiated among those effect estimates on the same circle or sphere (see Table 1). In addition, it may lead to the violation of the Gaussian distribution assumption, as illustrated in the poor controllability of FPR (Figure 1). d) Their power performance is not satisfactory (Figures 1, 2). As an alternative, MVT or LME through the hypothesis (2a) or (2b) on the two or three effect estimates from ASM, as shown in Figure 2A, provides a more accurate characterization because it allows for different units or dimensions across the effects.\nSimilarly for ESM, two dimensional reduction methods have separately been adopted in data analyses. For example, AUC was employed in Beauchamp et al. (2003), Greene et al. (2007), and McGregor et al. (2013). Although not explicitly stated, XUV was used in several real applications to identify the HDR effect under a condition through the main effect (or one-way interaction) of the ESM components in a one-way within-subject ANOVA (Weissman et al., 2006; Geier et al., 2007; Church et al., 2008), to detect the group or condition differences in the overall HDR shape through the group-by-component or condition-by-component interaction in a two-way ANOVA (e.g., Schlaggar et al., 2002; Church et al., 2008; Shuster et al., 2014), and to explore the three-way group-by-task-by-component interaction (Church et al., 2008). However, two limitations were not addressed in those analyses: the potential identification failure of XUV (Table 1 and Voxel 2 in Figure 2), and the limited applicability of univariate GLM.\nSome comparisons were performed in terms of amplitude, peak latency, and duration in the estimated HDR among various modeling methods (e.g., FSM, L2D, ESM, a nonlinear model, and inverse logit model; Lindquist et al., 2009). The inverse logit model was deemed the best among the candidates in both simulations and real data, and slightly more powerful than ESM. However, the comparisons were not optimal. First, the dimensional reduction from the HDR shape in ℝm to the three quantities (amplitude, delay, and duration) in ℝ3 might be compromised in power when detecting the shape subtleties—this point can be highly dependent on the experiment. Secondly, the reliability for the estimation of the three characteristics was suboptimal. For example, the lackluster performance of ESM in Lindquist et al. (2009) might be caused by the inaccurate amplitude based on the first local peak because such an approach could be misleading especially when more than one local peak occurs. Lastly, the final group analyses were still focused on the amplitude with the Student's t-test, an effective dimensional reduction from ℝm to ℝ1.\nA multivariate approach (Zhang et al., 2012) was previously proposed, analogous to our method except for the following differences. It was demonstrated among the voxels within only five structurally pre-defined regions; smoothing the estimated HDR from each subject by a Gaussian kernel and imposing regularization on the smoothed HDR were performed to improve the temporal continuities of the HDR; and group analysis was run through multivariate testing of one-sample or pair-wise comparisons among conditions, equivalent to MVT (2a or 2b) discussed here. Another approach (Zhang et al., 2013) assumed that the HDR under each condition would only vary in amplitude and latency across subjects; that is, the HDR shape was presumed same across all subjects. Specifically, the HDR curve for each condition was characterized at the group level by two parameters: one was of interest (amplitude) and the other of no interest (delay). In addition, the HDR shape (fixed across subjects) was modeled by cubic splines plus their time derivatives. Once the amplitude was estimated for each subject in a one-tier model that incorporated both within- and across-subject variances, a second round of group analysis was performed only on the amplitudes (ignoring the delay) through typical one-sample or paired t-test to make inference about a condition or contrast. The approach was demonstrated among the voxels within only three structurally predefined regions.\nRecently, a hierarchical approach was proposed for ESM through integrating both individual and group levels into one model (Degras and Lindquist, 2014) in which the HDR curves were captured through multiple higher-order B-spline functions. Even though only demonstrated on one slice of data, the approach is appealing because the variability at both levels is accounted for. However, the current implementation in Matlab is hindered by the following constraints or limitations. a) Spatial parcellation based on anatomical structure was required to determine the temporal correlation structure in the noise component. More applicable approaches would be based on a priori regions that are functionally parcellated through, for example, hierarchical clustering (Thirion et al., 2006; Ji, 2010), joint parcellation detection-estimation (Badillo et al., 2014), consensus clustering (Badillo et al., 2013), k-means clustering (Ji, 2010), etc. (b) The HDR shape may vary across different stimulus conditions under some scenarios (e.g., Ciuciu et al., 2003), and a presumption of the same shape HDR as in Degras and Lindquist (2014) may decrease the detection power when the shape subtleties are of interest. The same HDR assumption is reasonable under other circumstances and has proven sufficient for encoding or decoding the brain activity (Pedregosa et al., 2015). c) Final statistical inference in Degras and Lindquist (2014) through an asymptotic t-test was still based on the scaling factors of the same HDR curve shared by all conditions, a dimensional reduction approach from ℝm to ℝ1. An alternative approach is the incorporation of both individual and group levels in a mixed-effects model under the Bayesian framework (Chaari et al., 2013; Badillo et al., 2014). Applied at a priori regions that are functionally parcellated, this jointed detection and estimation method may render a robust procedure less sensitive to outliers than the conventional two-tier methods under the assumption that all the voxels share the same HDR within a region or parcel."}
2_test
{"project":"2_test","denotations":[{"id":"26578853-16568421-38285035","span":{"begin":1439,"end":1443},"obj":"16568421"},{"id":"26578853-24252847-38285036","span":{"begin":1458,"end":1462},"obj":"24252847"},{"id":"26578853-15050587-38285037","span":{"begin":2018,"end":2022},"obj":"15050587"},{"id":"26578853-22431587-38285038","span":{"begin":2050,"end":2054},"obj":"22431587"},{"id":"26578853-18550763-38285039","span":{"begin":3994,"end":3998},"obj":"18550763"},{"id":"26578853-24954281-38285040","span":{"begin":4359,"end":4363},"obj":"24954281"},{"id":"26578853-25204545-38285041","span":{"begin":5478,"end":5482},"obj":"25204545"},{"id":"26578853-16568421-38285042","span":{"begin":6120,"end":6124},"obj":"16568421"},{"id":"26578853-25182535-38285043","span":{"begin":6710,"end":6714},"obj":"25182535"},{"id":"26578853-24954281-38285044","span":{"begin":7483,"end":7487},"obj":"24954281"},{"id":"26578853-11771969-38285045","span":{"begin":8504,"end":8508},"obj":"11771969"},{"id":"26578853-15050594-38285046","span":{"begin":8527,"end":8531},"obj":"15050594"},{"id":"26578853-22245637-38285047","span":{"begin":8546,"end":8550},"obj":"22245637"},{"id":"26578853-15110015-38285048","span":{"begin":11074,"end":11078},"obj":"15110015"},{"id":"26578853-16125978-38285049","span":{"begin":11100,"end":11104},"obj":"16125978"},{"id":"26578853-19913625-38285050","span":{"begin":11124,"end":11128},"obj":"19913625"},{"id":"26578853-22227535-38285051","span":{"begin":11262,"end":11266},"obj":"22227535"},{"id":"26578853-16125978-38285052","span":{"begin":11350,"end":11354},"obj":"16125978"},{"id":"26578853-15110015-38285053","span":{"begin":11535,"end":11539},"obj":"15110015"},{"id":"26578853-14614810-38285054","span":{"begin":12867,"end":12871},"obj":"14614810"},{"id":"26578853-17690338-38285055","span":{"begin":12889,"end":12893},"obj":"17690338"},{"id":"26578853-24198784-38285056","span":{"begin":12917,"end":12921},"obj":"24198784"},{"id":"26578853-16767087-38285057","span":{"begin":13166,"end":13170},"obj":"16767087"},{"id":"26578853-17292627-38285058","span":{"begin":13186,"end":13190},"obj":"17292627"},{"id":"26578853-18245043-38285059","span":{"begin":13207,"end":13211},"obj":"18245043"},{"id":"26578853-12029136-38285060","span":{"begin":13397,"end":13401},"obj":"12029136"},{"id":"26578853-18245043-38285061","span":{"begin":13418,"end":13422},"obj":"18245043"},{"id":"26578853-24215974-38285062","span":{"begin":13440,"end":13444},"obj":"24215974"},{"id":"26578853-18245043-38285063","span":{"begin":13531,"end":13535},"obj":"18245043"},{"id":"26578853-19084070-38285064","span":{"begin":13947,"end":13951},"obj":"19084070"},{"id":"26578853-19084070-38285065","span":{"begin":14533,"end":14537},"obj":"19084070"},{"id":"26578853-22982104-38285066","span":{"begin":14892,"end":14896},"obj":"22982104"},{"id":"26578853-23473935-38285067","span":{"begin":15442,"end":15446},"obj":"23473935"},{"id":"26578853-24793829-38285068","span":{"begin":16451,"end":16455},"obj":"24793829"},{"id":"26578853-16281292-38285069","span":{"begin":17081,"end":17085},"obj":"16281292"},{"id":"26578853-14552578-38285070","span":{"begin":17350,"end":17354},"obj":"14552578"},{"id":"26578853-24793829-38285071","span":{"begin":17425,"end":17429},"obj":"24793829"},{"id":"26578853-25304775-38285072","span":{"begin":17660,"end":17664},"obj":"25304775"},{"id":"26578853-24793829-38285073","span":{"begin":17723,"end":17727},"obj":"24793829"},{"id":"26578853-23096056-38285074","span":{"begin":18044,"end":18048},"obj":"23096056"}],"text":"Discussion\nThere are many characteristics that could describe the HDR shape: onset latency, onset-to-peak, peak location, peak duration, magnitude or shape of the undershoot after the onset or during the recovery phase, and habituation or saturation effect. Because of the multiple facets of HDR shape, a lot of effects may well have gone undetected at both individual and group levels in most neuroimaging data analyses, and the failures to capture the shape nuances might have partially contributed to the poor reliability and reproducibility in the field. With a few exceptions, most analyses adopt FSM or ASM mainly for the simplicity of group analysis, as each condition or task is associated with one effect estimate, while other coefficients (e.g., time and dispersion derivatives in ASM) are a priori ignored. That is, activation detection intuitively focuses on the estimated magnitude around the activation peak while statistical inference on the whole HDR shape is generally considered a daunting hurdle. FSM may work well for situations such as a contrast between a condition and fixation. However, it would fail to detect shape subtleties such as prolonged plateau at the peak, slower or faster rise or fall, bigger or longer undershoot, or overall duration. Therefore, FSM through a presumed HDR (gamma variate in AFNI, canonical function in FSL and SPM) is very crude even in an experiment with a block design (Saad et al., 2006; Shan et al., 2013). ASM is an improvement over FSM; however, its flexibility is still limited. For instance, when one is interested in contrasting two conditions (or groups) or in investigating higher-order interactions, the three ASM basis functions may still not be enough in capturing the undershoot subtleties. In addition, characterizing the whole HDR curve with its peak value from ASM for group analysis may suffer from significant power loss, as demonstrated in our real experimental data. Response shapes can vary considerably over space (e.g., Handwerker et al., 2004; Gonzalez-Castillo et al., 2012; Badillo et al., 2013), and we believe it is important to model more accurately the HDRs at the individual level and test for shape rather just amplitude at the group level, particularly when detecting subtle differences between conditions or groups. The dominant adoption of FSM or ASM with a relatively rigid HDR shape reflects the daunting challenge in adopting ESM at the group level, and it is this challenge that motivated our exploration of various group analysis strategies with ESM.\n\nOverview of the testing methodologies\nAmong all the testing strategies for ESM (Table 1), MVT and LME maintain an accurate characterization for the hypothesis. In contrast, the dimensional reduction methods AUC, L2D, and EXC (XUV and XMV) project the original space of the alternative hypothesis from ℝm to ℝ1, ℝ1, and ℝm−1, respectively. Any dimensional reduction usually translates to information loss or geometrical distortion. Based on the results from our simulations and real data applications, we believe that the major testing methods for ESM are LME, XUV, MVT, XMV, and AUC, which all have the proper controllability for FPR. If sample size is not an issue in FMRI studies, MVT (e.g., hypothesis 2a or 2b) would be the most accurate approach in terms of hypothesis characterization. However, in practice the number of subjects is usually not large enough for MVT due to resource limitations (e.g., financial cost, time, and manpower), leading to an underpowered performance of MVT as shown in our simulations and real data. Among all the workaround methods through dimensional reduction, XUV has the least hypothesis distortion and the lowest power loss. With one group of subjects and no other explanatory variables present, XUV surpasses MVT, XMV, and AUC in power. However, with an accurate representation of the hypothesis, LME is slightly more efficient than XUV, and should be considered as the first choice (e.g., Alvarez et al., 2008). For all other situations, LME modeling is not feasible due to the constraint of variable parameterization, and we opt for the workaround methods through dimensional reduction, among which AUC is insensitive to subtle shape differences while XMV mostly underperforms unless when the temporal correlation is relatively high (e.g., 0.65 or higher; Chen et al., 2014). XUV achieves the best balance between dimensional reduction and statistical power. However, as XUV tests for parallelism, not exactly the same as the accurate representation characterized in MVT, it may fail in detecting the situation where the HDR profiles are roughly parallel. To compensate for the occasions when XUV fails, other dimensional reduction methods (MVT, AUC, XMV) may offer some complementary detection power.\nIn light of the discussion here, we strongly encourage the adoption of the ESM approach to achieving two goals: detecting activations and estimating the hemodynamics by characterizing the HDR shape. In addition to the large power gain at both individual and group levels, ESM provides the estimated HDR shape information at the group level, providing an extra layer of validation about the effect veracity through the graphical display of the familiar HDR shape, and alleviating the misconceptions and malpractices prevalent in statistical analysis (e.g., P-hacking, graphical presentation of statistic values instead of effect estimates, overuse of statistical significance; Motulsky, 2014). The HDR profile information from ESM offers a precious boost especially when a cluster fails to survive the typical stringent thresholding for multiple testing correction but still reaches the significance level of 0.05 at the voxel level. Such a reassuring support of ESM is unavailable from the alternatives of FSM and ASM, with which typically the investigator would be only able to report the peak HDR magnitude or statistic values at a region.\nOur recommendation of adopting ESM not only applies to event-related experiments, but also are adaptable to modeling the attenuation or habituation effect in block designs (Saad et al., 2006). In addition, this approximation modeling methodology of XUV assisted with MVT, AUC, and XMV has been applied to DTI data in which the simultaneous variables (white matter network groups such as corpus callosum, corona radiata, left and right hemispheric projection fibers, left and right hemispheric association fibers) were modeled by multiple explanatory variables (e.g., sex, age, behavioral measures) for each response variable such as fractional anisotropy, axial diffusivity, mean diffusivity, radial diffusivity, T1 relaxation time, proton density, and volume (Taylor et al., 2015).\nThe proposed modeling strategies have been implemented into the open-source program 3dMVM in AFNI, which offers the investigator all the testing results in the output including XUV and the auxiliary approaches (MVT, XMV, and AUC). MVT for the components from ESM presents a unique challenge when one or more within-subject factors are included in the model, and we offer a testing strategy that still fits in the MVM framework (Appendix B). As an alternative, these tests could be conducted in the traditional univariate GLM except for the two multivariate methods, MVT and XMV. In other words, some of the testing methods (MVT and XMV) are truly multivariate, while others (XUV, AUV, and L2D) are essentially univariate. However, as we demonstrated in Chen et al. (2014), these univariate tests are sometimes difficult to perform under the univariate framework, as shown by the implementation challenges faced by some of the neuroimaging packages. Instead, these univariate tests can be more conveniently formulated under the MVM platform by treating the levels of each within subject factor as simultaneous variables in (1) and then constructing the univariate testing statistics through a conversion process. For example, those univariate tests presented in Figure 2 cannot be performed under the univariate GLM framework due to the incorporation of a covariate (age) in the presence of two within subject factors (Condition and HDR effects). It is in this sense that we frame our discussion here under the MVM perspective.\n\nLimitations of the ESM approach\nIt is noteworthy that the reliability information from the individual subject analysis is not considered at the group level with the modeling methods discussed here, unlike the mixed-effect multilevel analysis (Worsley et al., 2002; Woolrich et al., 2004; Chen et al., 2012). In addition, the number of basis functions monotonically increases among FSM, ASM, and ESM, therefore it is expected that the goodness of fit at the individual subject analysis level improves across the three methods. On the other hand, as each condition is characterized through multiple (e.g., ≥7) basis functions in ESM, a reliable estimation of the HDR curve at the individual level pays a price through the lower degrees of freedom and requires enough (e.g., 20 or more) trials per condition, and may encounter the risk of numerical instability due to high correlations or even multicollinearity among the regressors. These latter issues can be exacerbated by poor stimulus timing designs. In addition, the typical regression analysis at the individual level assumes the linearity of HDR across trials. Although available (e.g., 3dNLfim in AFNI), a non-linear approach is usually difficult to handle and still requires some extent of prior information about the HDR shape. Furthermore, the ESM approach is generally considered to be susceptible to noise or effects unrelated to the effects of interest (e.g., head motion, physiological confounds). In other words, the confounding effects may leak into the HDR estimation through over-fitting. However, the false positives from the potential over-fitting at the individual level is less a concern at the group level for the following reasons: a) the likelihood is reduced unless most subjects systematically have similar or same confounding effects; b) cluster-based inferences may reduce the risk of false positives; and most importantly c) examination of the estimated HDR profiles offer an extra safeguard to filter out the potential false positives.\n\nComparisons with other modeling approaches\nSome (not all) of the dimensional reduction methods for ESM discussed here have been sporadically and individually applied to real data in the literature. For example, a popular practice with ASM is to solely focus on the coefficient of the principal basis function (e.g., canonical curve in SPM) with other coefficients (e.g., time and dispersion derivatives) being a priori abandoned. As our results with real data showed, the investigator may fail to detect most activations when the effect lies in the HDR shape nuances but not the peak. One suggestion for ASM was to extend the definition of amplitude in (6) to the L2-distance by including either the effect for the time derivative (7) or the effects for both time and dispersion derivatives (8) (Calhoun et al., 2004; Worsley and Taylor, 2006; Steffener et al., 2010). A similar approach was to express the effect estimates from the first two basis functions of ASM as a complex number (Wang et al., 2012). However, the potential issues with L2D or its analogs (e.g., Worsley and Taylor, 2006) are the following. a) The definition of amplitude extension in (7) and (8) is under the premise that all the three basis functions are orthogonal with each other (Calhoun et al., 2004). However, only the first two basis functions are orthogonal with each other, but not the third one. b) The second and third basis functions are not normalized; that is, they are not scaled to have a maximum value of 1, unlike the first basis function. In addition, the three effect estimates have different dimensions: the first is of percent signal change while the other two of percent signal change by the unit of time. Therefore, it is difficult to render a physically meaning interpretation with the L2D measures. c) All the effect estimates including negative values are folded into a positive L2D measure, which cannot be differentiated among those effect estimates on the same circle or sphere (see Table 1). In addition, it may lead to the violation of the Gaussian distribution assumption, as illustrated in the poor controllability of FPR (Figure 1). d) Their power performance is not satisfactory (Figures 1, 2). As an alternative, MVT or LME through the hypothesis (2a) or (2b) on the two or three effect estimates from ASM, as shown in Figure 2A, provides a more accurate characterization because it allows for different units or dimensions across the effects.\nSimilarly for ESM, two dimensional reduction methods have separately been adopted in data analyses. For example, AUC was employed in Beauchamp et al. (2003), Greene et al. (2007), and McGregor et al. (2013). Although not explicitly stated, XUV was used in several real applications to identify the HDR effect under a condition through the main effect (or one-way interaction) of the ESM components in a one-way within-subject ANOVA (Weissman et al., 2006; Geier et al., 2007; Church et al., 2008), to detect the group or condition differences in the overall HDR shape through the group-by-component or condition-by-component interaction in a two-way ANOVA (e.g., Schlaggar et al., 2002; Church et al., 2008; Shuster et al., 2014), and to explore the three-way group-by-task-by-component interaction (Church et al., 2008). However, two limitations were not addressed in those analyses: the potential identification failure of XUV (Table 1 and Voxel 2 in Figure 2), and the limited applicability of univariate GLM.\nSome comparisons were performed in terms of amplitude, peak latency, and duration in the estimated HDR among various modeling methods (e.g., FSM, L2D, ESM, a nonlinear model, and inverse logit model; Lindquist et al., 2009). The inverse logit model was deemed the best among the candidates in both simulations and real data, and slightly more powerful than ESM. However, the comparisons were not optimal. First, the dimensional reduction from the HDR shape in ℝm to the three quantities (amplitude, delay, and duration) in ℝ3 might be compromised in power when detecting the shape subtleties—this point can be highly dependent on the experiment. Secondly, the reliability for the estimation of the three characteristics was suboptimal. For example, the lackluster performance of ESM in Lindquist et al. (2009) might be caused by the inaccurate amplitude based on the first local peak because such an approach could be misleading especially when more than one local peak occurs. Lastly, the final group analyses were still focused on the amplitude with the Student's t-test, an effective dimensional reduction from ℝm to ℝ1.\nA multivariate approach (Zhang et al., 2012) was previously proposed, analogous to our method except for the following differences. It was demonstrated among the voxels within only five structurally pre-defined regions; smoothing the estimated HDR from each subject by a Gaussian kernel and imposing regularization on the smoothed HDR were performed to improve the temporal continuities of the HDR; and group analysis was run through multivariate testing of one-sample or pair-wise comparisons among conditions, equivalent to MVT (2a or 2b) discussed here. Another approach (Zhang et al., 2013) assumed that the HDR under each condition would only vary in amplitude and latency across subjects; that is, the HDR shape was presumed same across all subjects. Specifically, the HDR curve for each condition was characterized at the group level by two parameters: one was of interest (amplitude) and the other of no interest (delay). In addition, the HDR shape (fixed across subjects) was modeled by cubic splines plus their time derivatives. Once the amplitude was estimated for each subject in a one-tier model that incorporated both within- and across-subject variances, a second round of group analysis was performed only on the amplitudes (ignoring the delay) through typical one-sample or paired t-test to make inference about a condition or contrast. The approach was demonstrated among the voxels within only three structurally predefined regions.\nRecently, a hierarchical approach was proposed for ESM through integrating both individual and group levels into one model (Degras and Lindquist, 2014) in which the HDR curves were captured through multiple higher-order B-spline functions. Even though only demonstrated on one slice of data, the approach is appealing because the variability at both levels is accounted for. However, the current implementation in Matlab is hindered by the following constraints or limitations. a) Spatial parcellation based on anatomical structure was required to determine the temporal correlation structure in the noise component. More applicable approaches would be based on a priori regions that are functionally parcellated through, for example, hierarchical clustering (Thirion et al., 2006; Ji, 2010), joint parcellation detection-estimation (Badillo et al., 2014), consensus clustering (Badillo et al., 2013), k-means clustering (Ji, 2010), etc. (b) The HDR shape may vary across different stimulus conditions under some scenarios (e.g., Ciuciu et al., 2003), and a presumption of the same shape HDR as in Degras and Lindquist (2014) may decrease the detection power when the shape subtleties are of interest. The same HDR assumption is reasonable under other circumstances and has proven sufficient for encoding or decoding the brain activity (Pedregosa et al., 2015). c) Final statistical inference in Degras and Lindquist (2014) through an asymptotic t-test was still based on the scaling factors of the same HDR curve shared by all conditions, a dimensional reduction approach from ℝm to ℝ1. An alternative approach is the incorporation of both individual and group levels in a mixed-effects model under the Bayesian framework (Chaari et al., 2013; Badillo et al., 2014). Applied at a priori regions that are functionally parcellated, this jointed detection and estimation method may render a robust procedure less sensitive to outliers than the conventional two-tier methods under the assumption that all the voxels share the same HDR within a region or parcel."}