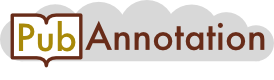
PMC:4620161 / 16202-18903
Annnotations
0_colil
{"project":"0_colil","denotations":[{"id":"26578853-24954281-358033","span":{"begin":73,"end":77},"obj":"24954281"},{"id":"26578853-7596267-358034","span":{"begin":1053,"end":1057},"obj":"7596267"},{"id":"26578853-1400644-358035","span":{"begin":1099,"end":1103},"obj":"1400644"},{"id":"26578853-8812068-358036","span":{"begin":1867,"end":1871},"obj":"8812068"},{"id":"26578853-22425670-358037","span":{"begin":2542,"end":2546},"obj":"22425670"}],"text":"Preview\nThis paper is a sequel to our previous exploration (Chen et al., 2014) of the multivariate modeling (MVM) approach for FMRI group analysis. The layout is as follows. First, we explore and review various hypothesis testing strategies at the group level when the HDR is estimated through multiple basis functions. Second, simulation data were generated to reveal how each methodology performs in terms of controllability for false positives and false negatives, and the performance of these methods was assessed when they were applied to the experimental dataset at both individual and group levels. Finally, we compare all the modeling methodologies for ASM and ESM as well as with and without dimension reduction. The modeling strategies and testing methods discussed here are all performed at the voxel level. Multiple testing correction can be applied in the conventional fashion by controlling the false positive rate (Benjamini and Hochberg, 1995) or the family-wise error through Monte Carlo simulations (3dClustSim in AFNI, Forman et al., 1995) or random field theory (Worsley et al., 1992).\nOur major contribution here is to demonstrate the importance of accounting for shape differences and to offer testing approaches at the group level within an MVM platform with the modeling flexibility that would not be available under the conventional GLM. Through our demonstration we propose that ESM should be adopted whenever appropriate or possible to identify the nuanced differences in HDR shape that would be difficult or unlikely to be revealed through FSM or ASM. Furthermore, we recommend that the investigator report the effect estimates such as the HDR curves to substantiate the results in addition to the statistical significance. The modeling framework and functionality are available in the program 3dMVM for public use in the AFNI suite (Cox, 1996).\nThroughout this article, regular italic letters (e.g., α) stand for scalars, boldfaced italic letters in lower (a) and upper (X) cases for column vectors and matrices respectively. The word multivariate is used here in the sense of treating the effect estimates from the same subject or from the levels of a within-subject factor as the instantiations of simultaneous response (or outcome) variables (e.g., the effect estimates for the HDR). This usage differs from the popular connotation in the FMRI field when the spatial structure (multiple voxels) is modeled as the simultaneous response variables, including such methods as multivariate pattern analysis (Haxby, 2012), independent component analysis, and machine learning methods such as support vector machines. Major acronyms used in the paper are listed in Appendix A."}
TEST0
{"project":"TEST0","denotations":[{"id":"26578853-65-73-358033","span":{"begin":73,"end":77},"obj":"[\"24954281\"]"},{"id":"26578853-234-242-358034","span":{"begin":1053,"end":1057},"obj":"[\"7596267\"]"},{"id":"26578853-234-242-358035","span":{"begin":1099,"end":1103},"obj":"[\"1400644\"]"},{"id":"26578853-115-123-358036","span":{"begin":1867,"end":1871},"obj":"[\"8812068\"]"},{"id":"26578853-226-234-358037","span":{"begin":2542,"end":2546},"obj":"[\"22425670\"]"}],"text":"Preview\nThis paper is a sequel to our previous exploration (Chen et al., 2014) of the multivariate modeling (MVM) approach for FMRI group analysis. The layout is as follows. First, we explore and review various hypothesis testing strategies at the group level when the HDR is estimated through multiple basis functions. Second, simulation data were generated to reveal how each methodology performs in terms of controllability for false positives and false negatives, and the performance of these methods was assessed when they were applied to the experimental dataset at both individual and group levels. Finally, we compare all the modeling methodologies for ASM and ESM as well as with and without dimension reduction. The modeling strategies and testing methods discussed here are all performed at the voxel level. Multiple testing correction can be applied in the conventional fashion by controlling the false positive rate (Benjamini and Hochberg, 1995) or the family-wise error through Monte Carlo simulations (3dClustSim in AFNI, Forman et al., 1995) or random field theory (Worsley et al., 1992).\nOur major contribution here is to demonstrate the importance of accounting for shape differences and to offer testing approaches at the group level within an MVM platform with the modeling flexibility that would not be available under the conventional GLM. Through our demonstration we propose that ESM should be adopted whenever appropriate or possible to identify the nuanced differences in HDR shape that would be difficult or unlikely to be revealed through FSM or ASM. Furthermore, we recommend that the investigator report the effect estimates such as the HDR curves to substantiate the results in addition to the statistical significance. The modeling framework and functionality are available in the program 3dMVM for public use in the AFNI suite (Cox, 1996).\nThroughout this article, regular italic letters (e.g., α) stand for scalars, boldfaced italic letters in lower (a) and upper (X) cases for column vectors and matrices respectively. The word multivariate is used here in the sense of treating the effect estimates from the same subject or from the levels of a within-subject factor as the instantiations of simultaneous response (or outcome) variables (e.g., the effect estimates for the HDR). This usage differs from the popular connotation in the FMRI field when the spatial structure (multiple voxels) is modeled as the simultaneous response variables, including such methods as multivariate pattern analysis (Haxby, 2012), independent component analysis, and machine learning methods such as support vector machines. Major acronyms used in the paper are listed in Appendix A."}
2_test
{"project":"2_test","denotations":[{"id":"26578853-24954281-38285022","span":{"begin":73,"end":77},"obj":"24954281"},{"id":"26578853-7596267-38285023","span":{"begin":1053,"end":1057},"obj":"7596267"},{"id":"26578853-1400644-38285024","span":{"begin":1099,"end":1103},"obj":"1400644"},{"id":"26578853-8812068-38285025","span":{"begin":1867,"end":1871},"obj":"8812068"},{"id":"26578853-22425670-38285026","span":{"begin":2542,"end":2546},"obj":"22425670"}],"text":"Preview\nThis paper is a sequel to our previous exploration (Chen et al., 2014) of the multivariate modeling (MVM) approach for FMRI group analysis. The layout is as follows. First, we explore and review various hypothesis testing strategies at the group level when the HDR is estimated through multiple basis functions. Second, simulation data were generated to reveal how each methodology performs in terms of controllability for false positives and false negatives, and the performance of these methods was assessed when they were applied to the experimental dataset at both individual and group levels. Finally, we compare all the modeling methodologies for ASM and ESM as well as with and without dimension reduction. The modeling strategies and testing methods discussed here are all performed at the voxel level. Multiple testing correction can be applied in the conventional fashion by controlling the false positive rate (Benjamini and Hochberg, 1995) or the family-wise error through Monte Carlo simulations (3dClustSim in AFNI, Forman et al., 1995) or random field theory (Worsley et al., 1992).\nOur major contribution here is to demonstrate the importance of accounting for shape differences and to offer testing approaches at the group level within an MVM platform with the modeling flexibility that would not be available under the conventional GLM. Through our demonstration we propose that ESM should be adopted whenever appropriate or possible to identify the nuanced differences in HDR shape that would be difficult or unlikely to be revealed through FSM or ASM. Furthermore, we recommend that the investigator report the effect estimates such as the HDR curves to substantiate the results in addition to the statistical significance. The modeling framework and functionality are available in the program 3dMVM for public use in the AFNI suite (Cox, 1996).\nThroughout this article, regular italic letters (e.g., α) stand for scalars, boldfaced italic letters in lower (a) and upper (X) cases for column vectors and matrices respectively. The word multivariate is used here in the sense of treating the effect estimates from the same subject or from the levels of a within-subject factor as the instantiations of simultaneous response (or outcome) variables (e.g., the effect estimates for the HDR). This usage differs from the popular connotation in the FMRI field when the spatial structure (multiple voxels) is modeled as the simultaneous response variables, including such methods as multivariate pattern analysis (Haxby, 2012), independent component analysis, and machine learning methods such as support vector machines. Major acronyms used in the paper are listed in Appendix A."}