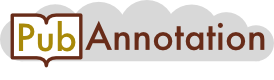
PMC:4572492 / 26814-32840
Annnotations
2_test
{"project":"2_test","denotations":[{"id":"26140448-24057835-2053719","span":{"begin":1094,"end":1096},"obj":"24057835"},{"id":"26140448-20967843-2053719","span":{"begin":1094,"end":1096},"obj":"20967843"},{"id":"26140448-10893422-2053720","span":{"begin":1941,"end":1943},"obj":"10893422"},{"id":"26140448-21054167-2053721","span":{"begin":2201,"end":2203},"obj":"21054167"},{"id":"26140448-19538313-2053721","span":{"begin":2201,"end":2203},"obj":"19538313"},{"id":"26140448-24482837-2053722","span":{"begin":3085,"end":3087},"obj":"24482837"},{"id":"26140448-23910463-2053722","span":{"begin":3085,"end":3087},"obj":"23910463"},{"id":"26140448-20942902-2053722","span":{"begin":3085,"end":3087},"obj":"20942902"},{"id":"26140448-25152454-2053722","span":{"begin":3085,"end":3087},"obj":"25152454"},{"id":"26140448-25279928-2053722","span":{"begin":3085,"end":3087},"obj":"25279928"},{"id":"26140448-25152454-2053723","span":{"begin":3461,"end":3463},"obj":"25152454"},{"id":"26140448-25279928-2053723","span":{"begin":3461,"end":3463},"obj":"25279928"},{"id":"26140448-24482837-2053724","span":{"begin":3562,"end":3564},"obj":"24482837"},{"id":"26140448-24123198-2053725","span":{"begin":4105,"end":4107},"obj":"24123198"}],"text":"Discussion\nInteractions between genes in gene-set and pathway analyses are assumed to exist, but they are, in general, not accounted for in current genetic analysis methods. Here we proposed a joint location-scale (mean-variance) testing method to account for these relationships as well as main effects, while avoiding explicit specification of the contributing interactions. We used this method to identify the apical membrane constituent gene set as contributing to CF lung disease and replicated this association in an independent sample of similarly ascertained CF-affected subjects. Further, we illustrated how the gene-set association notably benefits from consideration of phenotypic variance differences that might have been induced by GxG and/or GxE relationships. This apical gene-set association was not initially detected via the classical location-only testing as used in a typical GWAS and provides insight into interacting genes and exposure variables affecting lung function.\nSNPs in SLC9A3 have been reported to associate with CF lung disease in two candidate gene studies.19,29 However, a broader role for the SLC9A3/SLC9A3R2/EZR complex was not recognized. The JLS-Fisher test ranked these players as three of the top five contributing apical plasma membrane genes. Variation at SLC9A3R2 or EZR loci and possibly also SLC9A3R1 given its paralogous function (and supporting evidence in the French sample) could be expected to influence CF lung disease severity, presumably by affecting changes in net SLC9A3 activity. For example, modest changes in levels of either SLC9A3R2 or EZR could alter functional apical membrane SLC9A3 levels, just as SLC9A3 locus variants might alter the amount of SLC9A3 transcripts. It should also be noted that the SLC9A3 regulators, with their proximal localization at the apical membrane, could contribute a tethering function for a number of other apical membrane proteins, including CFTR.31 Given loss of function in CF, SLC9A3 regulators might not be mediating their effects via CFTR; however, it is possible that some of the remaining significant apical membrane components, beyond SLC9A3, utilize the EZR and/or the SLC9A3 regulator scaffold.32,33\nWe demonstrated the potential advantage of using the JLS framework over the conventional location-only or scale-only approach in practice. As proof of principle, we used examples from an association study of a complex trait (glycemic control in type 1 diabetes) and a modifier study of a Mendelian disease (lung function in CF). Both of these datasets provided examples of a genetic effect on a quantitative phenotype that varied based on another variable (i.e., treatment regimen and age, respectively). Our results showed that if the interacting exposure(s) were not known or available, the JLS method could have improved power over conventional approaches in detecting the association.\nRecent literature cautions the investigation and interpretation of significant differences in phenotypic variability across genotypes as well as observed GxE and GxG interaction effects.17,34–37 First, variance heterogeneity across genotypes can result from a mean-variance relationship induced by an inappropriate choice of measurement scale for the phenotype under investigation. Second, dependence between a causal locus and an exposure (G or E) can create synthetic interactions between the exposure and other tagged SNPs in incomplete LD with the causal locus.36,37 Consequently, we might observe phenotypic variance heterogeneity across genotypes of a tagged SNP.17 In these scenarios, however, because there is a true underlying genotype-phenotype relationship (main effect of G), it does not matter whether we detect this association through a location or a scale test, or both; the goal of our approach is to leverage complex genetic architecture to efficiently identify the associated G for follow-up investigation of causal interactors.\nChallenges in understanding and being able to replicate original findings, particularly in the GxG, GxE, gene-set, and pathway analysis setting, is well documented.38 Given the potential heterogeneity between the Canadian discovery sample and the French replication sample (e.g., clinical treatment, climate, and air quality), interaction models could differ between the two samples. Therefore, even if the exposure variables were precisely measured, a significant multivariate interaction effect found in the discovery sample may not be observed in the replication sample. The proposed method allows different exposure variables between genes and between samples, as long as the corresponding underlying phenotype-genotype association mechanism results in phenotypic mean and/or variance differences between genotypes of the variants of interest. A step-by-step guide for application of the JLS framework, and a discussion of its advantages compared to alternative approaches, is included as additional considerations in Appendix A.\nIn conclusion, we have provided a robust joint location-scale (JLS) testing framework for the detection of single-variant, gene-set, or pathway associations involving either main or interaction effects, or both. This methodology applies to analysis of a quantitative trait, regardless of the biological interpretation of the chosen scale of phenotypic measurement. Application of the JLS approach identified the SLC9A3 regulatory complex as an important contributor to CF lung disease, which was completely missed in previous analyses of the same data. The JLS association testing method should be considered for future gene-set, pathway, and whole-genome association scans. Further, JLS should be employed to re-examine datasets previously analyzed via the conventional location-only or scale-only testing approaches, for complex traits or complex secondary phenotypes of Mendelian diseases. The method will help researchers pinpoint susceptibility loci for additional analysis toward the understanding of the genetic architecture of a complex trait."}