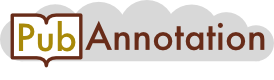
PMC:4331679 / 7151-23675
Annnotations
{"target":"https://pubannotation.org/docs/sourcedb/PMC/sourceid/4331679","sourcedb":"PMC","sourceid":"4331679","source_url":"https://www.ncbi.nlm.nih.gov/pmc/4331679","text":"Methods\nOur work is broadly organised in three interrelated parts. We initially constructed a basic apoptosis network (say N1) which is free of abnormal mutation and represents signalling pathways inside a healthy cell. We used the biological data for our studies based on the phosphorylation levels of signalling proteins and phenotypic responses taken from the biological experiments conducted in [5]. Hereafter, we call this data set as \"Yaffe's data\". We compared the simulation data from this network with the Yaffe's data using Genetic Algorithm and Dynamic Time Warping for DMSO treatment and derived a tumorigenic network (say N2). Then we modified N2 to fit with Yaffe's data to derive a drug sensitive network (say N3). N1 was constructed directly from literature; N2 consisted of edges added and deleted from N1 by Genetic Algorithm; N3 consisted of edges added and deleted from N2 by Genetic Algorithm.\n\nData\nThe Yaffe's data set contains six types of cellular phenotypic responses from three cancer cell lines, namely triple negative BT-20, Hormone sensitive MCF-7 and HER2 overexpressing MDA-MB-453. The six phenotypes are Apoptosis, Proliferation, G1, S, G2 and M. These phenotypic responses are linked with on 35 signalling proteins (only 32 available in the published data set). The signalling molecule data available contains 8 time points (0, 1, 2, 3, 4, 5, 6 and 7). The phenotypic responses were measured at five time points (0, 6, 7, 8 and 9), over 24 hours in the panel of breast cancer cell lines. The cell fates and signalling data are available for all the three cell lines. The measurements of the signalling and cell fate data have been done for six treatments namely DMSO, TAR, DOX, DT, T-D and D-T. The apoptosis level is reportedly high for the T-D treatment, meaning the cells are now less tumorigenic for this treatment and thereby more susceptible to death induced by DNA damage response pathways. The study in [5] employs several mathematical modelling approaches to relate signalling data to cell phenotypes. Principal component analysis (PCA) was used to identify co-variation between signals, whereas partial least-squares regression (PLSR) was used to identify co-variation between molecular signals and corresponding cellular phenotypic responses. In both PCA and PLSR modelling, the input vectors contained quantitative measurements of the signalling proteins.\n\nApoptosis network construction\nWe constructed a generic apoptosis network consisting of 22 signalling proteins including 18 proteins from the biological experiment conducted in [5]. The authors of [5] provided Variable Importance in Projection (VIP) scores for different selected proteins to demonstrate how the selected proteins were useful for predicting apoptosis of different cell lines. For example, proteins with VIP score \u003e 1 were important variables, proteins with VIP score \u003c 0.5 were unimportant variables. Since our goal is to test the goodness of fit of our model with Yaffe's data, we selected proteins that have been measured in the experiment. Also, our signalling network includes molecules based on the prior knowledge from several pathways playing critical roles in inducing cell death, which include DNA damage response and cell death pathways, stress response pathways, etc.\nThe network diagram is shown in Figure 1. We constructed connections between various signalling proteins in our basic apoptosis network using the existing literature. HER2 is a cell transmembrane receptor which plays a significant role in breast cancer by suppression of tumour growth. It provides growth signals in PI3KAKT and Ras-MAPK pathways. HER2 also suppresses p53-mediated apoptosis by upregulation of MDM2 by activation of AKT. p53 also participates in promoting apoptosis by sensing DNA damage and can upregulate pro-apoptotic Bcl-2 proteins (e.g. PUMA) as well as suppress IAPs (e.g. survivin) [6]. PUMA is a pro-apoptotic protein and plays crucial roles in apoptosis. Tumour suppressor gene p53 regulates the expression of PUMA. Once activated, PUMA frees the apoptosis regulator Bax and Bak owing to its high affinity with Bcl-2 oncogenic group of proteins. The freed proteins further transduce the apoptotic signals to the mitochondria. This causes the Caspases to be activated and the activated caspases eventually lead to the cell death [7]. Activation of pro-apoptotic BAX/Bak facilitates heme protein cytochrome c being released from the inner mitochondria membrane [8,9]. The cytochrome c further stimulates Casp9, which then stimulates Casp3 and Casp7, which eventually lead to apoptotic cell death [10]. Up-regulation of DAPK induces apoptosis by enhancing response of tumour suppressor p53 whereas down-regulation of DAPK reduces the response of p53 to several oncogenes including c-Myc and E2F-1. The activated form of DAPK further activates the Beclin-1 by phosphorylation. Beclin-1 activation is crucial for Autophagy and also for its crosstalk with apoptosis.\nFigure 1 Basic apoptosis network and various rewiring events. Basic apoptotic network contained all edges except the broken edges (green and blue). Edges represented by thick red colour were deleted by Genetic Algorithm while inferring N2 from N1. Broken edges represented by green colour were inserted by Genetic Algorithm while inferring N2 from N1. Edges represented by magenta colour were deleted by Genetic Algorithm while inferring N3 from N2. Broken edges represented by blue colour were inserted by Genetic Algorithm while inferring N3 from N2. Beclin-1 is an autophagy protein that interacts with Bcl-2, an anti-apoptotic protein, and they form a complex, to facilitate a switch from autophagy to apoptosis and vice-versa. The activity of Beclin-1, owing to the complex formation of Bcl-2:Beclin-1 complex, is suppressed by Bcl-2 protein [11,12]. STAT3 is an important gene due to its roles in regulating cell fate including cell survival and cell growth. Experimental results have shown that a decreased level of STAT3 leads to a reduced level of Bcl-2 [13]. Activation of STAT3 gene stimulates transcription of quite a few cell fate controlling genes, which include some of the members of Bcl-2 protein family [14]. The proteins Wee1 and Cdk1 (Cyclin-dependent kinase 1) play very important roles in regulating cell cycle. During mitosis, the size of a cell is controlled by the inhibition of Cdk1 by Wee1 which prevents the cell from going into mitosis. The suppression of Cdk1 by Wee1 is done by phosphorylation of Cdk1 [15]. Cdk1 in turn phosphorylates Bcl-2 and suppresses its activity [16]. The EGF receptor (EGFR) is a cell membrane protein receptor with high affinity for several specific proteins (ligands) including Epidermal Growth Factor (EGF). EGFR further activates many targets including Jun N-terminal kinases (JNKs). Mutation in EGFR may lead to its uncontrolled expression which can potentially cause uncontrolled cell growth, which is a hall mark of cancer [17]. The Bcl-2-associated death promoter (BAD), another member protein from Bcl-2 group of proteins, is highly pro-apoptotic. JNK can inhibit apoptosis by phosphorylating BAD protein [18]. Trimeric Tumor Necrosis Factor (TNF) activates multiple signalling pathways which in turn lead to apoptosis. TNF interacts with death domain (DD) protein TRADD. TRADD in turn has been shown to have high affinity for RIP [19]. Casp3 mediates cleavage of multiple proteins and causes DNA fragmentation which eventually leads to apoptosis [2].\n\nSimulation with ODE\nUsing the topology of the basic apoptosis network (Figure 1), constructed from the literature, we derived a computational model by generating rate equations for the network. The complete set of differential equations for the basic network N1 is given in Table 2. Concentration of each molecule is represented by enclosing the molecule name with square brackets (e.g. [Casp8]). Reaction rate constants are represented as rc1, rc2 etc. For example, based on the basic network N1 in Figure 1, the differential equations for the change of concentration of AKT and p53 were derived as:\nTable 2 Differential equations for the basic apoptosis network N1 (Figure 1)\nS.N. Rate of Change Differential equations\n1 d d t Casp 6 - [Casp6]·rc11 + [Casp9]·rc12\n2 d d t CDK 1 +[Wee1]·rc13 - [CDK1]·rc14\n3 d d t Wee 1 - [Wee1]·rc13\n4 d d t JNK - [JNK]·rc15 + [EGFR]·rc17\n5 d d t BID - [BID]·rc16 - [BID]·rc25\n6 d d t EGFR - [EGFR]·rc17 - [EGFR]·rc32;\n7 d d t PUMA - [PUMA]·rc19 + [p53]·rc27\n8 d d t Casp 3 - [Casp3]·rc1 + [XIAP]·rc2 + [SMAC]·rc4 + [Casp8]·rc7 - [Casp3]·rc8 +[Casp9]·rc9 +[Casp6]·rc11 + [MOMP]·rc30\n9 d d t Casp9 - [Casp9]·rc9 - [Casp9]·rc12 + [PUMA]·rc19 - [Casp9]·rc24 + [BCL2]·rc26\n10 d d t HER2 - [HER2]·rc20 - [HER2]·rc31\n11 d d t Casp8 - [Casp8]·rc3 + [RIP1]·rc6 - [Casp8]·rc7 + [Casp3]·rc8 + [BID]·rc25 +[EGFR]·rc\n12 d d t Apop +[Casp3]·rc1\n13 d d t XIAP - [XIAP]·rc2 + [SMAC]·rc21 + [Casp9]·rc24 + [HER2]·rc31\n14 d d t SMAC +[Casp8]·rc3 - [SMAC]·rc4 + [BCL2]·rc5 - [SMAC]·rc21 + [MOMP]·rc29\n15 d d t BCL2 - [BCL2]·rc5 + [p53]·rc10 + [CDK1]·rc14 + [JNK]·rc15 +[BID]·rc16 +[STAT3]·rc22 - [BCL2]·rc26\n16 d d t RIP1 - [RIP1]·rc6 + [TNFR]·rc23\n17 d d t P53 - [p53]·rc10 + [AKT]·rc18 - [p53]·rc27\n18 d d t AKT - [AKT]·rc18 + [HER2]·rc20\n19 d d t STAT3 - [STAT3]·rc22\n20 d d t TNFR - [TNFR]·rc23\n21 d d t DAPK 1 - [DAPK1]·rc28\n22 d d t MOMP +[DAPK1]·rc28 - [MOMP]·rc29 - [MOMP]·rc30 d d t A K T = - A K T ⋅ r c 18 + H E R 2 ⋅ r c 20 , d d t p 53 = - p 53 ⋅ r c 10 + A K T ⋅ | r c 18 - p 53 ⋅ r c 27 ,\nwhere rc18 is the binding rate constant from AKT to p53 and [AKT] is the concentration of the AKT molecule. Also, rc20 represents the binding rate constant for HER2 to AKT. Similarly, for p53, variables in the corresponding equation incorporate information for the molecular concentrations and the binding rate constants for AKT and p53.\nIn our study, the ODEs were solved using the standard Matlab library function ode45. This function can interpret the differential equations in the form of rate reactions. The ode45 accepts the initial values of the molecules and the time duration in the form of function parameters [20,21]. Using this procedure, we derived the rate equations and time course data for all twenty-two signalling proteins. The initial concentrations of the signalling molecules were set to dimensionless numeric value of one [22]. Some of the rate constants were taken directly from the literature. These include Casp9 \u0026 Casp3, Casp9 \u0026 XIAP, Casp8 \u0026 BID, Casp3 \u0026 XIAP, Casp3 \u0026Casp8, Casp3 \u0026 Apop, p53 \u0026 PUMA, Casp3 \u0026 Casp6 and XIAP \u0026 SMAC [4,23,24]. The remaining parameters were generated randomly but we verified them by Partial Least Square Regression (PLSR) test. We also performed sensitivity analysis of the model parameters by varying the model parameters to ensure overall robustness of the model to the small variations of parameters (see result section for PLSR test and sensitivity analysis).\n\nGenetic algorithm\nWe applied the Genetic Algorithm to search for the network topologies that fit the real data well, in order to uncover the rewiring inside the tumour cells. There are several optimisation techniques available in the literature which can be used to search for the network topologies. These techniques include, for example, Simulated Annealing, Evolution Strategies, Genetic Algorithm, Evolutionary Programming etc. Simulated Annealing has population size of only one which represents current solution, thus the solution space is not as wide as GA. Similarly, Evolutionary Programming can only use mutation operator. By contrast, Genetic Algorithms have several solutions in the beginning and crossovers can be performed to generate the diversity in the population of network structures. While the probabilistic selection of GA helps us simulate the stochastic biochemical networks in better ways, Evolution Strategies uses deterministic selection [25,26]. After comparing these techniques, we decided to use Genetic Algorithm in our study. As a global search technique, GA is considered to be very robust as it makes very few assumptions about the problem under consideration [27].\nThe implementation of Genetic Algorithm assumes that the variables from problem can be represented in the form of binary strings typically known as Chromosomes. A feasible solution is also encoded in the form of a chromosome. The initial population for the Genetic Algorithm is a random set of chromosomes. These chromosomes are evaluated using an objective function. The chromosomes representing better solutions are given higher probability to reproduce offspring in next generation. Chromosomes with lower scores are removed from the solution set. The pseudocode for the Genetic algorithm used in our experiments is given in Table 1.\nTable 1 Genetic Algorithm for finding mutation in apoptotic network.\nINPUT: Objective function, Network Topology(NT) in the form of Adjacency vector of size n × n, reaction rate constants vector of size n × n, maximum number of generations Max num gen for the algorithm, biological data measurements\nOUTPUT: A vector consisting topology of best uncovered network, score vector S, for such uncovered networks\n1. NT0 ⇐ topology\n2. RC0 ⇐ rate constants\n3. Max_num_itr ⇐ total number of iteration allowed\n4. S0 ⇐ 0\n5. Derive Population P (randomly generated networks)\n6. For max_ num_gen times do:\n7. Derive rate equations for each network in P\n8. Solve each of the Rate equations\n9. Derive numerical solutions (time series data)\n10. Compare the simulation results with Yaffe's data using DTW Objective function and calculate the Score\n11. Select 50 best score\n12. Perform crossover among best selected networks to formulate next generation, total of 100 networks again. The crossover points are selected based on random numbers. The networks to be crossed over are selected randomly.\n13. Perform mutation for each bit with the probability of 0.01, for each of the hundred networks.\n14. Set new population P = mutated network from step 13\n15. Check for the convergence.\n16. If network not converged, go to step 5\n7. Output solution set. The network topology is represented by an adjacency matrix. For each edge between two nodes in the network there is a Boolean value of ' 1' in the matrix, and a ' 0' entry represents the absence of connection between the corresponding nodes. The adjacency matrix can be modified in Genetic Algorithm as follows [27]. We initially derive the chromosome (vector of 1 × 484) by concatenating the rows of the adjacency matrix of the network into one binary string. As such, the network topology of 22 nodes is represented as a chromosome i.e. a binary string of length 484. The topology of the basic network N1 was changed randomly using mutation and crossover. The crossover points were randomly selected uniformly from the range of [1:484]. Generally, the mutation rate for any particular bit is less than 1% [27]. In our implementation the mutation operator was applied to each of the bit in a chromosome (after performing crossover) with a low probability value of 0.01. Once the process of selection of chromosome, recombination and mutation is finished, the population of next generation is evaluated [27].\nTo compare the time series data, we used Dynamic Time Warping (DTW) [28,29]. DTW is widely used for comparing time series data, due to its strength to capture the variation in data that may vary owing to different speed. Hence, DTW can calculate the optimal match between two temporal data. The objective function of DTW, for comparing two time series datasets s and t is given as:\nD i , j = c o s t + m i n D i - 1 , j D [ i , j - 1 ] D i - 1 , j - 1 ,\nwhere cost = dist(s[i], t[j]), D is a matrix of size [0..n, 0..m], D[0, 0] = 0. Initially each entry of D[i, 0], for 0 \u003ci ≤ n, and D[0, j], for 0 \u003cj ≤ m, are set to infinity, i.e. a larger value which cannot be part of the data sequences. Here s[1..n] and t[1..m] are the two time series representing protein phosphorylation levels over time points to be compared, whereas s[i] and t[j] represents the data at time points i and j respectively. For any two numbers s[i] and t[j], dist(s[i], t[j]) represents the distance between the numbers, e.g. dist(s[i], t[j]) = |s[i] −t[j]|. The objective function aims to measure the goodness of fit between the simulation data and real data.","divisions":[{"label":"title","span":{"begin":0,"end":7}},{"label":"p","span":{"begin":8,"end":914}},{"label":"sec","span":{"begin":916,"end":2401}},{"label":"title","span":{"begin":916,"end":920}},{"label":"p","span":{"begin":921,"end":2401}},{"label":"sec","span":{"begin":2403,"end":7501}},{"label":"title","span":{"begin":2403,"end":2433}},{"label":"p","span":{"begin":2434,"end":3297}},{"label":"p","span":{"begin":3298,"end":4983}},{"label":"figure","span":{"begin":4984,"end":5537}},{"label":"label","span":{"begin":4984,"end":4992}},{"label":"caption","span":{"begin":4994,"end":5537}},{"label":"p","span":{"begin":4994,"end":5537}},{"label":"p","span":{"begin":5538,"end":7501}},{"label":"sec","span":{"begin":7503,"end":11097}},{"label":"title","span":{"begin":7503,"end":7522}},{"label":"p","span":{"begin":7523,"end":8103}},{"label":"table-wrap","span":{"begin":8104,"end":9523}},{"label":"label","span":{"begin":8104,"end":8111}},{"label":"caption","span":{"begin":8113,"end":8181}},{"label":"p","span":{"begin":8113,"end":8181}},{"label":"table","span":{"begin":8182,"end":9523}},{"label":"tr","span":{"begin":8182,"end":8226}},{"label":"th","span":{"begin":8182,"end":8186}},{"label":"th","span":{"begin":8188,"end":8202}},{"label":"th","span":{"begin":8204,"end":8226}},{"label":"tr","span":{"begin":8227,"end":8277}},{"label":"td","span":{"begin":8227,"end":8228}},{"label":"td","span":{"begin":8230,"end":8246}},{"label":"td","span":{"begin":8248,"end":8277}},{"label":"tr","span":{"begin":8278,"end":8324}},{"label":"td","span":{"begin":8278,"end":8279}},{"label":"td","span":{"begin":8281,"end":8296}},{"label":"td","span":{"begin":8298,"end":8324}},{"label":"tr","span":{"begin":8325,"end":8358}},{"label":"td","span":{"begin":8325,"end":8326}},{"label":"td","span":{"begin":8328,"end":8343}},{"label":"td","span":{"begin":8345,"end":8358}},{"label":"tr","span":{"begin":8359,"end":8402}},{"label":"td","span":{"begin":8359,"end":8360}},{"label":"td","span":{"begin":8362,"end":8374}},{"label":"td","span":{"begin":8376,"end":8402}},{"label":"tr","span":{"begin":8403,"end":8445}},{"label":"td","span":{"begin":8403,"end":8404}},{"label":"td","span":{"begin":8406,"end":8418}},{"label":"td","span":{"begin":8420,"end":8445}},{"label":"tr","span":{"begin":8446,"end":8492}},{"label":"td","span":{"begin":8446,"end":8447}},{"label":"td","span":{"begin":8449,"end":8462}},{"label":"td","span":{"begin":8464,"end":8492}},{"label":"tr","span":{"begin":8493,"end":8537}},{"label":"td","span":{"begin":8493,"end":8494}},{"label":"td","span":{"begin":8496,"end":8509}},{"label":"td","span":{"begin":8511,"end":8537}},{"label":"tr","span":{"begin":8538,"end":8667}},{"label":"td","span":{"begin":8538,"end":8539}},{"label":"td","span":{"begin":8541,"end":8557}},{"label":"td","span":{"begin":8559,"end":8667}},{"label":"tr","span":{"begin":8668,"end":8758}},{"label":"td","span":{"begin":8668,"end":8669}},{"label":"td","span":{"begin":8671,"end":8685}},{"label":"td","span":{"begin":8687,"end":8758}},{"label":"tr","span":{"begin":8759,"end":8805}},{"label":"td","span":{"begin":8759,"end":8761}},{"label":"td","span":{"begin":8763,"end":8776}},{"label":"td","span":{"begin":8778,"end":8805}},{"label":"tr","span":{"begin":8806,"end":8904}},{"label":"td","span":{"begin":8806,"end":8808}},{"label":"td","span":{"begin":8810,"end":8824}},{"label":"td","span":{"begin":8826,"end":8904}},{"label":"tr","span":{"begin":8905,"end":8936}},{"label":"td","span":{"begin":8905,"end":8907}},{"label":"td","span":{"begin":8909,"end":8922}},{"label":"td","span":{"begin":8924,"end":8936}},{"label":"tr","span":{"begin":8937,"end":9011}},{"label":"td","span":{"begin":8937,"end":8939}},{"label":"td","span":{"begin":8941,"end":8954}},{"label":"td","span":{"begin":8956,"end":9011}},{"label":"tr","span":{"begin":9012,"end":9097}},{"label":"td","span":{"begin":9012,"end":9014}},{"label":"td","span":{"begin":9016,"end":9029}},{"label":"td","span":{"begin":9031,"end":9097}},{"label":"tr","span":{"begin":9098,"end":9209}},{"label":"td","span":{"begin":9098,"end":9100}},{"label":"td","span":{"begin":9102,"end":9115}},{"label":"td","span":{"begin":9117,"end":9209}},{"label":"tr","span":{"begin":9210,"end":9255}},{"label":"td","span":{"begin":9210,"end":9212}},{"label":"td","span":{"begin":9214,"end":9227}},{"label":"td","span":{"begin":9229,"end":9255}},{"label":"tr","span":{"begin":9256,"end":9312}},{"label":"td","span":{"begin":9256,"end":9258}},{"label":"td","span":{"begin":9260,"end":9272}},{"label":"td","span":{"begin":9274,"end":9312}},{"label":"tr","span":{"begin":9313,"end":9357}},{"label":"td","span":{"begin":9313,"end":9315}},{"label":"td","span":{"begin":9317,"end":9329}},{"label":"td","span":{"begin":9331,"end":9357}},{"label":"tr","span":{"begin":9358,"end":9392}},{"label":"td","span":{"begin":9358,"end":9360}},{"label":"td","span":{"begin":9362,"end":9376}},{"label":"td","span":{"begin":9378,"end":9392}},{"label":"tr","span":{"begin":9393,"end":9425}},{"label":"td","span":{"begin":9393,"end":9395}},{"label":"td","span":{"begin":9397,"end":9410}},{"label":"td","span":{"begin":9412,"end":9425}},{"label":"tr","span":{"begin":9426,"end":9462}},{"label":"td","span":{"begin":9426,"end":9428}},{"label":"td","span":{"begin":9430,"end":9446}},{"label":"td","span":{"begin":9448,"end":9462}},{"label":"tr","span":{"begin":9463,"end":9523}},{"label":"td","span":{"begin":9463,"end":9465}},{"label":"td","span":{"begin":9467,"end":9480}},{"label":"td","span":{"begin":9482,"end":9523}},{"label":"p","span":{"begin":9524,"end":9674}},{"label":"p","span":{"begin":9675,"end":10012}},{"label":"p","span":{"begin":10013,"end":11097}},{"label":"title","span":{"begin":11099,"end":11116}},{"label":"p","span":{"begin":11117,"end":12297}},{"label":"p","span":{"begin":12298,"end":12934}},{"label":"table-wrap","span":{"begin":12935,"end":14268}},{"label":"label","span":{"begin":12935,"end":12942}},{"label":"caption","span":{"begin":12944,"end":13004}},{"label":"p","span":{"begin":12944,"end":13004}},{"label":"table","span":{"begin":13005,"end":14268}},{"label":"tr","span":{"begin":13005,"end":13235}},{"label":"td","span":{"begin":13005,"end":13235}},{"label":"tr","span":{"begin":13236,"end":13343}},{"label":"td","span":{"begin":13236,"end":13343}},{"label":"tr","span":{"begin":13344,"end":13361}},{"label":"td","span":{"begin":13344,"end":13361}},{"label":"tr","span":{"begin":13362,"end":13385}},{"label":"td","span":{"begin":13362,"end":13385}},{"label":"tr","span":{"begin":13386,"end":13436}},{"label":"td","span":{"begin":13386,"end":13436}},{"label":"tr","span":{"begin":13437,"end":13446}},{"label":"td","span":{"begin":13437,"end":13446}},{"label":"tr","span":{"begin":13447,"end":13499}},{"label":"td","span":{"begin":13447,"end":13499}},{"label":"tr","span":{"begin":13500,"end":13529}},{"label":"td","span":{"begin":13500,"end":13529}},{"label":"tr","span":{"begin":13530,"end":13576}},{"label":"td","span":{"begin":13530,"end":13576}},{"label":"tr","span":{"begin":13577,"end":13612}},{"label":"td","span":{"begin":13577,"end":13612}},{"label":"tr","span":{"begin":13613,"end":13661}},{"label":"td","span":{"begin":13613,"end":13661}},{"label":"tr","span":{"begin":13662,"end":13767}},{"label":"td","span":{"begin":13662,"end":13767}},{"label":"tr","span":{"begin":13768,"end":13792}},{"label":"td","span":{"begin":13768,"end":13792}},{"label":"tr","span":{"begin":13793,"end":14016}},{"label":"td","span":{"begin":13793,"end":14016}},{"label":"tr","span":{"begin":14017,"end":14114}},{"label":"td","span":{"begin":14017,"end":14114}},{"label":"tr","span":{"begin":14115,"end":14170}},{"label":"td","span":{"begin":14115,"end":14170}},{"label":"tr","span":{"begin":14171,"end":14201}},{"label":"td","span":{"begin":14171,"end":14201}},{"label":"tr","span":{"begin":14202,"end":14244}},{"label":"td","span":{"begin":14202,"end":14244}},{"label":"tr","span":{"begin":14245,"end":14268}},{"label":"td","span":{"begin":14245,"end":14268}},{"label":"p","span":{"begin":14269,"end":15377}},{"label":"p","span":{"begin":15378,"end":15759}},{"label":"p","span":{"begin":15760,"end":15843}}],"tracks":[{"project":"2_test","denotations":[{"id":"25707537-22579283-14870713","span":{"begin":400,"end":401},"obj":"22579283"},{"id":"25707537-22579283-14870714","span":{"begin":1946,"end":1947},"obj":"22579283"},{"id":"25707537-22579283-14870715","span":{"begin":2581,"end":2582},"obj":"22579283"},{"id":"25707537-22579283-14870716","span":{"begin":2601,"end":2602},"obj":"22579283"},{"id":"25707537-19641508-14870717","span":{"begin":4352,"end":4353},"obj":"19641508"},{"id":"25707537-16874066-14870718","span":{"begin":4485,"end":4486},"obj":"16874066"},{"id":"25707537-12052897-14870719","span":{"begin":4618,"end":4620},"obj":"12052897"},{"id":"25707537-19180116-14870720","span":{"begin":5833,"end":5835},"obj":"19180116"},{"id":"25707537-22485198-14870721","span":{"begin":5836,"end":5838},"obj":"22485198"},{"id":"25707537-22504301-14870722","span":{"begin":6207,"end":6209},"obj":"22504301"},{"id":"25707537-7626804-14870723","span":{"begin":6519,"end":6521},"obj":"7626804"},{"id":"25707537-11350724-14870724","span":{"begin":6972,"end":6974},"obj":"11350724"},{"id":"25707537-14967141-14870725","span":{"begin":7156,"end":7158},"obj":"14967141"},{"id":"25707537-16940186-14870726","span":{"begin":7382,"end":7384},"obj":"16940186"},{"id":"25707537-20221256-14870727","span":{"begin":7498,"end":7499},"obj":"20221256"},{"id":"25707537-19619456-14870728","span":{"begin":10520,"end":10522},"obj":"19619456"},{"id":"25707537-22967854-14870729","span":{"begin":10734,"end":10735},"obj":"22967854"},{"id":"25707537-18406323-14870730","span":{"begin":10739,"end":10741},"obj":"18406323"}],"attributes":[{"subj":"25707537-22579283-14870713","pred":"source","obj":"2_test"},{"subj":"25707537-22579283-14870714","pred":"source","obj":"2_test"},{"subj":"25707537-22579283-14870715","pred":"source","obj":"2_test"},{"subj":"25707537-22579283-14870716","pred":"source","obj":"2_test"},{"subj":"25707537-19641508-14870717","pred":"source","obj":"2_test"},{"subj":"25707537-16874066-14870718","pred":"source","obj":"2_test"},{"subj":"25707537-12052897-14870719","pred":"source","obj":"2_test"},{"subj":"25707537-19180116-14870720","pred":"source","obj":"2_test"},{"subj":"25707537-22485198-14870721","pred":"source","obj":"2_test"},{"subj":"25707537-22504301-14870722","pred":"source","obj":"2_test"},{"subj":"25707537-7626804-14870723","pred":"source","obj":"2_test"},{"subj":"25707537-11350724-14870724","pred":"source","obj":"2_test"},{"subj":"25707537-14967141-14870725","pred":"source","obj":"2_test"},{"subj":"25707537-16940186-14870726","pred":"source","obj":"2_test"},{"subj":"25707537-20221256-14870727","pred":"source","obj":"2_test"},{"subj":"25707537-19619456-14870728","pred":"source","obj":"2_test"},{"subj":"25707537-22967854-14870729","pred":"source","obj":"2_test"},{"subj":"25707537-18406323-14870730","pred":"source","obj":"2_test"}]}],"config":{"attribute types":[{"pred":"source","value type":"selection","values":[{"id":"2_test","color":"#9393ec","default":true}]}]}}