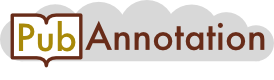
PMC:4331679 / 18250-23675
Annnotations
{"target":"https://pubannotation.org/docs/sourcedb/PMC/sourceid/4331679","sourcedb":"PMC","sourceid":"4331679","source_url":"https://www.ncbi.nlm.nih.gov/pmc/4331679","text":"Genetic algorithm\nWe applied the Genetic Algorithm to search for the network topologies that fit the real data well, in order to uncover the rewiring inside the tumour cells. There are several optimisation techniques available in the literature which can be used to search for the network topologies. These techniques include, for example, Simulated Annealing, Evolution Strategies, Genetic Algorithm, Evolutionary Programming etc. Simulated Annealing has population size of only one which represents current solution, thus the solution space is not as wide as GA. Similarly, Evolutionary Programming can only use mutation operator. By contrast, Genetic Algorithms have several solutions in the beginning and crossovers can be performed to generate the diversity in the population of network structures. While the probabilistic selection of GA helps us simulate the stochastic biochemical networks in better ways, Evolution Strategies uses deterministic selection [25,26]. After comparing these techniques, we decided to use Genetic Algorithm in our study. As a global search technique, GA is considered to be very robust as it makes very few assumptions about the problem under consideration [27].\nThe implementation of Genetic Algorithm assumes that the variables from problem can be represented in the form of binary strings typically known as Chromosomes. A feasible solution is also encoded in the form of a chromosome. The initial population for the Genetic Algorithm is a random set of chromosomes. These chromosomes are evaluated using an objective function. The chromosomes representing better solutions are given higher probability to reproduce offspring in next generation. Chromosomes with lower scores are removed from the solution set. The pseudocode for the Genetic algorithm used in our experiments is given in Table 1.\nTable 1 Genetic Algorithm for finding mutation in apoptotic network.\nINPUT: Objective function, Network Topology(NT) in the form of Adjacency vector of size n × n, reaction rate constants vector of size n × n, maximum number of generations Max num gen for the algorithm, biological data measurements\nOUTPUT: A vector consisting topology of best uncovered network, score vector S, for such uncovered networks\n1. NT0 ⇐ topology\n2. RC0 ⇐ rate constants\n3. Max_num_itr ⇐ total number of iteration allowed\n4. S0 ⇐ 0\n5. Derive Population P (randomly generated networks)\n6. For max_ num_gen times do:\n7. Derive rate equations for each network in P\n8. Solve each of the Rate equations\n9. Derive numerical solutions (time series data)\n10. Compare the simulation results with Yaffe's data using DTW Objective function and calculate the Score\n11. Select 50 best score\n12. Perform crossover among best selected networks to formulate next generation, total of 100 networks again. The crossover points are selected based on random numbers. The networks to be crossed over are selected randomly.\n13. Perform mutation for each bit with the probability of 0.01, for each of the hundred networks.\n14. Set new population P = mutated network from step 13\n15. Check for the convergence.\n16. If network not converged, go to step 5\n7. Output solution set. The network topology is represented by an adjacency matrix. For each edge between two nodes in the network there is a Boolean value of ' 1' in the matrix, and a ' 0' entry represents the absence of connection between the corresponding nodes. The adjacency matrix can be modified in Genetic Algorithm as follows [27]. We initially derive the chromosome (vector of 1 × 484) by concatenating the rows of the adjacency matrix of the network into one binary string. As such, the network topology of 22 nodes is represented as a chromosome i.e. a binary string of length 484. The topology of the basic network N1 was changed randomly using mutation and crossover. The crossover points were randomly selected uniformly from the range of [1:484]. Generally, the mutation rate for any particular bit is less than 1% [27]. In our implementation the mutation operator was applied to each of the bit in a chromosome (after performing crossover) with a low probability value of 0.01. Once the process of selection of chromosome, recombination and mutation is finished, the population of next generation is evaluated [27].\nTo compare the time series data, we used Dynamic Time Warping (DTW) [28,29]. DTW is widely used for comparing time series data, due to its strength to capture the variation in data that may vary owing to different speed. Hence, DTW can calculate the optimal match between two temporal data. The objective function of DTW, for comparing two time series datasets s and t is given as:\nD i , j = c o s t + m i n D i - 1 , j D [ i , j - 1 ] D i - 1 , j - 1 ,\nwhere cost = dist(s[i], t[j]), D is a matrix of size [0..n, 0..m], D[0, 0] = 0. Initially each entry of D[i, 0], for 0 \u003ci ≤ n, and D[0, j], for 0 \u003cj ≤ m, are set to infinity, i.e. a larger value which cannot be part of the data sequences. Here s[1..n] and t[1..m] are the two time series representing protein phosphorylation levels over time points to be compared, whereas s[i] and t[j] represents the data at time points i and j respectively. For any two numbers s[i] and t[j], dist(s[i], t[j]) represents the distance between the numbers, e.g. dist(s[i], t[j]) = |s[i] −t[j]|. The objective function aims to measure the goodness of fit between the simulation data and real data.","divisions":[{"label":"title","span":{"begin":0,"end":17}},{"label":"p","span":{"begin":18,"end":1198}},{"label":"p","span":{"begin":1199,"end":1835}},{"label":"table-wrap","span":{"begin":1836,"end":3169}},{"label":"label","span":{"begin":1836,"end":1843}},{"label":"caption","span":{"begin":1845,"end":1905}},{"label":"p","span":{"begin":1845,"end":1905}},{"label":"table","span":{"begin":1906,"end":3169}},{"label":"tr","span":{"begin":1906,"end":2136}},{"label":"td","span":{"begin":1906,"end":2136}},{"label":"tr","span":{"begin":2137,"end":2244}},{"label":"td","span":{"begin":2137,"end":2244}},{"label":"tr","span":{"begin":2245,"end":2262}},{"label":"td","span":{"begin":2245,"end":2262}},{"label":"tr","span":{"begin":2263,"end":2286}},{"label":"td","span":{"begin":2263,"end":2286}},{"label":"tr","span":{"begin":2287,"end":2337}},{"label":"td","span":{"begin":2287,"end":2337}},{"label":"tr","span":{"begin":2338,"end":2347}},{"label":"td","span":{"begin":2338,"end":2347}},{"label":"tr","span":{"begin":2348,"end":2400}},{"label":"td","span":{"begin":2348,"end":2400}},{"label":"tr","span":{"begin":2401,"end":2430}},{"label":"td","span":{"begin":2401,"end":2430}},{"label":"tr","span":{"begin":2431,"end":2477}},{"label":"td","span":{"begin":2431,"end":2477}},{"label":"tr","span":{"begin":2478,"end":2513}},{"label":"td","span":{"begin":2478,"end":2513}},{"label":"tr","span":{"begin":2514,"end":2562}},{"label":"td","span":{"begin":2514,"end":2562}},{"label":"tr","span":{"begin":2563,"end":2668}},{"label":"td","span":{"begin":2563,"end":2668}},{"label":"tr","span":{"begin":2669,"end":2693}},{"label":"td","span":{"begin":2669,"end":2693}},{"label":"tr","span":{"begin":2694,"end":2917}},{"label":"td","span":{"begin":2694,"end":2917}},{"label":"tr","span":{"begin":2918,"end":3015}},{"label":"td","span":{"begin":2918,"end":3015}},{"label":"tr","span":{"begin":3016,"end":3071}},{"label":"td","span":{"begin":3016,"end":3071}},{"label":"tr","span":{"begin":3072,"end":3102}},{"label":"td","span":{"begin":3072,"end":3102}},{"label":"tr","span":{"begin":3103,"end":3145}},{"label":"td","span":{"begin":3103,"end":3145}},{"label":"tr","span":{"begin":3146,"end":3169}},{"label":"td","span":{"begin":3146,"end":3169}},{"label":"p","span":{"begin":3170,"end":4278}},{"label":"p","span":{"begin":4279,"end":4660}},{"label":"p","span":{"begin":4661,"end":4744}}],"tracks":[]}