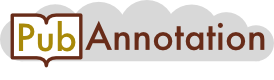
PMC:4331678 / 6620-8609
Annnotations
2_test
{"project":"2_test","denotations":[{"id":"25707434-18037900-14839064","span":{"begin":209,"end":211},"obj":"18037900"},{"id":"25707434-22522134-14839065","span":{"begin":227,"end":229},"obj":"22522134"},{"id":"25707434-23572412-14839066","span":{"begin":248,"end":250},"obj":"23572412"},{"id":"25707434-12015889-14839067","span":{"begin":672,"end":674},"obj":"12015889"},{"id":"25707434-15130933-14839068","span":{"begin":1337,"end":1338},"obj":"15130933"}],"text":"Different proteomic data sources (i.e., protein sequences, PPI networks and protein domains) often capture proteins' properties in different aspects, and have different correlations with different GO terms [1,20]. Yang et al. [21] and Teng et al. [22] observed that the GO term similarities have different correlations with different proteomic data sources. Therefore, integrating these data sources can often enable a more comprehensive view of proteins and their functions. Recently, several studies have observed a significant improvement in protein function prediction when multiple heterogenous biological data sources are integrated. To name a few, Pavlidis et al. [23] integrated heterogeneous data sources in three different ways: (i) early integration concatenates all feature vectors from different data sources of a protein into a single feature vector; (ii) intermediate integration computes the functional association network for each data set separately and then combines them; (iii) late integration trains a support vector machine (SVM) on each network (or kernel) and then combines the resulting discriminant values. Their study revealed that different data sources have different qualities, and setting different weights for different networks can enhance the accuracy of protein function prediction. Lanckriet et al. [5] proposed a semi-definite programming based SVM method to get the optimal weights on individual networks. Tsuda et al. [14] constructed an optimal combination of weights on individual networks using convex optimization. Mostafavi et al. [15] determined the optimal function-specific composite network by solving a linear regression problem. These methods constructed a composite network for each functional label. Since there are often more than hundreds of functional labels, and these labels are highly unbalanced and inter-correlated, these algorithms are often confronted with the over-fitting problem and require massive computational resources."}