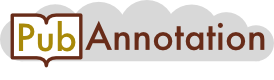
PMC:4331678 / 45070-46359
Annnotations
2_test
{"project":"2_test","denotations":[{"id":"25707434-23353650-14839094","span":{"begin":665,"end":666},"obj":"23353650"},{"id":"25707434-22522134-14839095","span":{"begin":669,"end":671},"obj":"22522134"}],"text":"Conclusions\nIn this paper, we study how to integrate multiple functional networks for accurate protein function prediction and propose a method called MNet. MNet unifies the optimization of a composite network and the optimization of a predictor on the composite network in a single objective. An extensive empirical study shows that MNet can predict protein functions more accurately than related competitive methods, and it's also less affected by the parameter selection issue.\nProtein functions are rather difficult to predict, i.e., proteins are often multifunctional and promiscuous, and the functional annotations of proteins are incomplete and error prone [2,3,21]. There are many avenues for future improvements for protein function prediction. For example, incorporating pathway information, evolutional knowledge, and reducing the noisy in the individual networks before the integration. In addition, an input network can have some high quality subnetworks and low quality subnetworks; it is promising to design algorithms to discover and differentiate these subnetworks, and to integrate the high quality subnetworks to enhance the prediction accuracy, and to discard the low quality ones (or assign very small weights to these subnetworks) to reduce their destructive effects."}