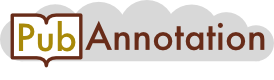
PMC:4331678 / 10447-11595
Annnotations
{"target":"https://pubannotation.org/docs/sourcedb/PMC/sourceid/4331678","sourcedb":"PMC","sourceid":"4331678","source_url":"https://www.ncbi.nlm.nih.gov/pmc/4331678","text":"MNet has a close relationship with multiple kernel learning, which is a popular topic in machine learning [26], and it's also widely applied in biological data mining [5,14,25]. Wang et al. [27] introduced a method called Optimal Multiple Graphs learning (OMG) to integrate multiple graphs into a composite one for graph-based semi-supervised learning. Shiga et al. [28] proposed a method called LIG. LIG first partitions each individual graph into several locally informative subgraphs via soft spectral clustering and then integrates these subgraphs into a composite one for graph-based classification. A protein can have several different functions and these functions are inter-correlated, thus protein function prediction from multiple data sources can also be transformed into a multi-label multiple kernel learning problem [3,25]. Multi-label multiple kernel learning methods often learn a composite kernel for each binary label and thus have a complexity linear to the number of labels. Bucak et al. [29] suggested a method called multiple kernel learning by stochastic approximation, whose complexity is sub-linear to the number of labels.","tracks":[{"project":"2_test","denotations":[{"id":"25707434-15130933-14839070","span":{"begin":168,"end":169},"obj":"15130933"}],"attributes":[{"subj":"25707434-15130933-14839070","pred":"source","obj":"2_test"}]}],"config":{"attribute types":[{"pred":"source","value type":"selection","values":[{"id":"2_test","color":"#93e1ec","default":true}]}]}}