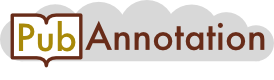
PMC:4331677 / 7882-19857
Annnotations
2_test
{"project":"2_test","denotations":[{"id":"25707321-21398668-14839511","span":{"begin":97,"end":99},"obj":"21398668"},{"id":"25707321-18676415-14839512","span":{"begin":806,"end":807},"obj":"18676415"},{"id":"25707321-17510168-14839513","span":{"begin":1795,"end":1797},"obj":"17510168"},{"id":"25707321-15319262-14839514","span":{"begin":2024,"end":2026},"obj":"15319262"},{"id":"25707321-17510168-14839515","span":{"begin":2027,"end":2029},"obj":"17510168"},{"id":"25707321-17510168-14839516","span":{"begin":2427,"end":2429},"obj":"17510168"},{"id":"25707321-15319262-14839517","span":{"begin":2988,"end":2990},"obj":"15319262"},{"id":"25707321-17510168-14839518","span":{"begin":2991,"end":2993},"obj":"17510168"},{"id":"25707321-20714286-14839519","span":{"begin":4849,"end":4851},"obj":"20714286"},{"id":"25707321-18676415-14839520","span":{"begin":9250,"end":9251},"obj":"18676415"},{"id":"25707321-19605421-14839521","span":{"begin":9252,"end":9254},"obj":"19605421"},{"id":"25707321-21506615-14839522","span":{"begin":9255,"end":9257},"obj":"21506615"},{"id":"25707321-21506615-14839523","span":{"begin":9854,"end":9856},"obj":"21506615"},{"id":"25707321-19605421-14839524","span":{"begin":9942,"end":9944},"obj":"19605421"}],"text":"Materials and methods\n\nData set\nWe extracted all human targets and ligands from sc-PDB database [16], which is an annotated archive of the druggable binding sites extracted from the Protein Data Bank [17]. In this work, we set the amino acid residues possessing at least one atom within eight angstroms around the ligand as binding sites. A target might possess more than one binding sites, and each binding site might interact with several ligands (Figure 2). After removing the redundance and checking the consistency, we got 836 targets and 2710 corresponding ligands. Among them, 782 single binding site targets interact with 1988 ligands to form 2561 interaction pairs, and other multiple binding sites targets interact with 722 ligands to form 854 interaction pairs. According to a published method [9], for each binding site, we generated as many negative site-ligand pairs as the known positive pairs by combining the site with randomly chosen ligands among the other sites' ligands (excluding those known to interact with the given target). Of course, this protocol may generate false negative data for some ligands could actually interact with the site whereas they have not been experimentally tested. Totally, 6830 site-ligand pairs include in our data set (Table 1).\nFigure 2 Illustration of the target-ligand binding. A target might possess more than one binding sites, and a binding site might bind with many ligands. The data set is organized in three levels: targets, sites and ligands.\nTable 1 Statistics of the data set\nTarget Ligand Pairs\nOne-site 782 1988 5122\nMulti-site 54 722 1708\nTotal 836 2710 6830\n\nRepresentation of targets' binding sites based on target dictionary\nIn this work, we made use of the target dictionary provided by Nagamine and Sakakibara [18] to represent the biding sites. The dictionary is organized as three layers: the amino acids, trimers and trimer clusters. In the first layer, each amino acid is first represented by 237 items of physical-chemical properties [19,18], such as residue volume, polarizability and solvation free energy. And then, a principal component analysis (PCA [20]) is applied to reduce the dimension. As a result, each amino acid is described by a 5-dimensional feature vector. In the second layer, twenty amino acids are permutated and combined into 4200 trimers. Each trimer αtri(α01, α11, α12) is mapped into a 5-dimensional vector space [18] as follows:\n(1) α t r i α 01 , α 11 , α 12 = α α 01 + α ( α 11 ) + α ( α 12 ) 4\nwhere α(α01), α(α11), α(α12) and αtri(α01, α11, α12) are the 5-dimensional vectors. α01 is the center (major) amino acid, α11 and α12 are the left and right amino acid (subordinate) respectively. There is no location difference between α11 and α12, that means αtri(α01, α11, α12) and αtri(α01, α12, α11) are equivalence. In the third layer, the hierarchical clustering (Ward's algorithm [21]) is used to cluster 4200 trimers into 199 clusters [19,18]. All clusters constitute the dictionary.\nTherefore, we first broke the binding site sequences into trimers. For example, the amino acid sequence NGMGN produces three trimers G(NM), M(GG) and G(MN). Since G(NM) and G(MN) are equivalence, we could combine them by adding a count. Then, we casted all the trimers into 199 clusters, and counted the occurring frequency of each cluster in every binding site. Finally, all cluster frequencies were normalized to unit L2 norm to obtain the feature vectors with 199 dimensions for the binding sites. For example, the sequence NGMGN can be represented as following:\n(2) B s ( N G M G N ) … c ( G ( N N ) , G ( M N ) , … ) … c ( M ( G G ) , M ( A G ) , … ) … = ( … 2 5 … 1 5 … )\nwhere Bs(·) denotes a binding site feature vector. c(·) denotes a cluster, for example, c(G(N N), G(M N),...) represent a cluster that contains G(N N), G(M N), etc. Because the trimers in the same cluster own similar chemical properties, the clusters can be viewed as chemical \"groups\", based on which the ligand binding sites are decomposed into fragments.\n\nGeneration of ligand dictionary and ligand representation\nRepresentation of chemical space involves two steps, defining a dictionary and de-scribing ligands as features. We have integrated sever data sources to make the dictionary (data shows in supplementary materials). In PubChem database, there are 881 predefined chemical substructures. We made some modification on the fin-gerprints to gear with our model. First, the single atoms and bonds were removed because they are not in the same structural level with trimers. Second, some sub-structures, such as benzene were removed; because they are too common to serve as a discriminately feature. Third, functional groups/fingerprints of molecular in Check-mol were integrated [22]. Finally, we generated a dictionary with 747 substructures. Based on the dictionary, each ligand was represented by a 747-dimensional binary vector whose element indicates the presence or absence of each substructure by 1 or 0.\n\nConstruction of fragment interaction model\nAs described above, all binding sites could be described as a matrix S = (s1, s2,⋯,sm)T, where m is the number of binding sites. si = (si1, si2,⋯,sip)T denotes the i-th binding site, where sik corresponds the k-th fragment of the i-th binding site and p is the number of site features/fragments. Meanwhile, all ligands could be described as a matrix L = (l1, l2,⋯,ln)T, where n is the number of ligands. lj = (lj1, lj2,⋯,ljq)T denotes the j-th ligand, where ljk indicates the presence or absence of ligand the k-th substructures of the j-th ligand and q is the number of ligand features. The interaction data set is denoted as D = {(i1, j1), (i2, j2),⋯,(ic, jc)}, where ik = 1, 2,⋯,m; jk = 1, 2,⋯,n; (ik, jk) is the k-th site-ligand pair and c is the number of site-ligand interaction pairs. sik and ljk are the site and ligand vectors in the k-th interaction pair respectively. y = (y1, y2,⋯,yc) denotes the labels of interaction pairs, where yk ∈ {1, −1} indicate the positive and negative interaction respectively. Because fragments of targets and ligands are in a physically interaction distance (within 8 angstrom), it is reasonable to assume that there exist inherent chemical interactions between target and ligand features, and the sum of feature interactions determinates the target-ligand interaction. Therefore, the proposed approach is called fragment interaction model (FIM, Figure 1) and it can be expressed as the following equation:\n(3) F ( s * , l * ) = s * T M l *\nwhere s∗ represents a binding site and l∗ represents a ligand. s∗ and l∗ might be unseen to the data set. M represents genomic and chemical spaces interaction matrix/network. If sign(F (s∗, l∗)) is 1, we predicted a positive interaction, otherwise we predicted a negative interaction (sign(·) is the sign function, return −1 and 1).\nIt is easy to solve parameters M in Equation 3 by logistic regression. However, it is inconvenient to expand to include more information. Therefore, mathematics transformation is conducted, and Equation 3 changes into Equation 4.\n(4) F ( s * , l * ) = w T ( s * ⊗ l * )\nwhere w is a vector vision of features interaction matrix M, and ⊗ denotes tensor product. Obviously, Equation 3 and Equation 4 are equivalent. For convenience, we denoted:\n(5) ψ ( s * , l * ) = s * ⊗ l *\nIt is easy to fit w through support vector machine (SVM). Based on the Lagrange dual theory, Equation 4 can be rewritten as its dual form on the data set.\n(6) F ( s * , l * ) = ∑ k = 1 c α k y k ψ ( s i k , l j k ) T ψ ( s * , l * )\nWhere αk is dual variable and yk is the label of the interaction pair (ik, jk) Equation 6 demonstrates that for a given site-ligand pair (s∗, l∗), it only relates with inner product of the support site-ligand pairs (αk ≠ 0). Therefore, we should only care 0 about the inner product of support site-ligand pairs and the site-ligand pair to predict.\n(7) ψ ( s * 1 , l * 1 ) T ψ ( s * 2 , l * 2 ) = ( s * 1 , ⊗ l * 1 ) T ( s * 2 ⊗ l * 2 ) = s * 1 T s * 2 l * 1 T l * 2\nwhere (s∗1, l∗1) and (s∗2, l∗2) are two pairs of site-ligands. Equation 7 is very important because it transforms tensor product into inner product. On one hand, we avoided calculating the tensor product, which always means large computing load. One the other hand, inner product facilitates us to design kernels and add more information by kernel trick. For convenience, we denoted:\n(8) K l o c ( s * 1 , s * 2 ) = s * 1 T s * 2 , K l i g ( l * 1 , l * 2 ) = l * 1 T l * 2\nThen Equation 7 can be changed as:\n(9) K l o c - l i g ( s * 1 , l * 1 ; s * 2 , l * 2 ) = K l o c ( s * 1 , s * 2 ) K l i g ( l * 1 , l * 2 )\nBecause we utilized linear kernel, therefore, the above Equations are invertible and the genomic and chemical interaction matrix M, on the data set, can be described as:\n(10) M = ∑ k = 1 c α k y k s i l j T\nAlthough Equation 7 have been mentioned in many papers [9,11,23], the kernels in those works were nonlinear and irreversible (because of kernel trick), thus we known little about how the genomic space interact with chemical space. In this paper, we adopted linear kernel without kernel trick, so that the genomic and chemical interaction matrix could be calculate through Equation 10, which rendered the model to be chemical interpretable.\n\nRepresentative methods for comparison\nIn order to evaluate the proposed method in this paper, we chosen three representative methods for comparison: chemical substructures and protein domains correlation model (CS-PD) [23], bipartite local model with neighbor-based interaction-profile inferring (BLM-NII) [11] and random forest (RF) [15].\n• CS-PD: Proteins were described by domains and ligands were represented by substructures in CS-PD model. Sparse canonical correspondence analysis (SCCA) algorithm was applied to recognize the physical-chemical factors between the domains and substructures. In prediction phase, the domain and substructure physical-chemical factors in a given target-ligand pair were added to generate a discriminant value. If the value was higher than a threshold, the target and ligand were predicted to interact with each other.\n• BLM-NII: On one hand, excluding target ti, make a list of all other known targets of ligand lj, as well as a separate list of the targets not known to be targeted ligand lj. The known targets were given a label +1 and the others a label −1. Then, look for a classification rule that tried to discriminate the +1-labeled data from the −1-labeled data using the available genomic sequence data for the targets. This rule was applied to predict the label of target ti and ligand lj. On the other hand, fixing the same target ti and excluding ligand lj, make a list of all other known ligands targeting ti, as well as a list of ligands not known to target ti. Similar with before, ligands known to target ti were given the label +1 and the others were given the label −1. We looked for a classification rule that tried to discriminate the +1-labeled data from the −1-labeled data, using the available chemical structure data for the ligands. This rule was also used to predict the label of target ti and ligand lj. At last, the two results were combined to generate a final label. For new targets or ligands, a neighbor-based interaction-profile inferring was applied to get an interaction profile.\n• RF: The targets were described as CTD (Composition-Transition-Distribution, [15]) features. The ligands were represented as fingerprints. Then, the two kinds of features were combined into a vector to generate data set. Finally, random forest (RF) was employed to predict interactions."}