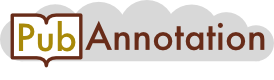
PMC:4331677 / 4972-6477
Annnotations
2_test
{"project":"2_test","denotations":[{"id":"25707321-19708682-14839504","span":{"begin":260,"end":261},"obj":"19708682"},{"id":"25707321-18676415-14839505","span":{"begin":410,"end":411},"obj":"18676415"},{"id":"25707321-21893517-14839506","span":{"begin":603,"end":605},"obj":"21893517"},{"id":"25707321-19605421-14839507","span":{"begin":761,"end":763},"obj":"19605421"},{"id":"25707321-20840733-14839508","span":{"begin":842,"end":844},"obj":"20840733"},{"id":"25707321-21893517-14839509","span":{"begin":845,"end":847},"obj":"21893517"},{"id":"25707321-23162055-14839510","span":{"begin":848,"end":850},"obj":"23162055"}],"text":"Recently, some methods considering both the target and ligand information have been proven to be promising in drug design and discovery. Jacob et al. applied the EC Number (Enzyme Commission number) and PubChem fingerprints (a set of molecular substructures) [8] to represent targets and ligands respectively, and proposed a pairwise support vector machine (pSVM) method to predict target-ligand interactions [9]. Laarhoven et al. described the targets and ligands by sequences and compound 3D structures respectively, and introduced the target-ligand interaction network to build the prediction model [10]. Bleakley et al. proposed Bipartite Local Model (BLM), which integrated the ligand-based and target-based methods to generate a comprehensive prediction [11]. BLM has been further studied by Xia et al., Laarhoven et al. and Mei et al.[12,10,13]. The BLM shows a very good predictive ability, however, it cannot deal with the situation that both the ligand and target are unseen in the training set. Yamanishi et al. represented the genome space with sequences and target profiles, and the chemical space with compound 3D structures and ligand profiles, and then generated a uniform \"pharmacological space\" to build the prediction model [2]. Cheng et al. applied the mass distribution property from physics on the target-ligand network to predict the target-ligand interactions [14]. Cao et al. integrated the genome and chemical space into random forests to obtain a better predictive ability [15]."}