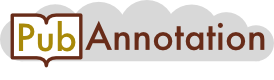
PMC:4331677 / 23618-26758
Annnotations
2_test
{"project":"2_test","denotations":[{"id":"25707321-7265238-14839525","span":{"begin":491,"end":493},"obj":"7265238"}],"text":"The role of global information in the binding\nBecause the intensity of intermolecular interaction decreases rapidly with the increasing distance, we can infer that local information may dominate the binding procedure. However, the local binding sites are inevitably influenced by the other part of target. We adopt the target sequence, obtained from the KEGG database [24], similarity score as global information. The sequence similarities are measured in nor-malized Smith-Waterman scores [25]. Kglo(t,t′)=SWt,t′/SW(t,t),SW(t′,t′) where t and t' are protein sequences, SW (·, ·) is the original Smith-Waterman s-core, and Kglo is a target global similarity matrix (global information). Finally, the global and local information are integrated by kernel trick, as follows:\n(11) K t a r ( s * 1 , s * 2 ) = λ K g l o ( t * 1 , t * 2 ) + 1 - λ K l o c ( s * 1 , s * 2 )\nwhere s∗1 and s∗2 are binding sites, t∗1 and t∗2 are target sequences corresponding to s∗1 and s∗2, and λ is the ratio of global information. After the introduction of global information, the kernels are no longer linear. We attempted to estimate the role of global information in the binding procedure by increasing the ratio λ. With the increase of global information (λ), the AUC score first increases, until λ = 0.3, then, the score reaches the maximum and further increasing of global information (λ) would result in the AUC score decreasing (Table 3). Although AUC score varies with λ, it only varies in a narrow range (from 0.916 to 0.922), which implies that the global information only has a limited influence on prediction accuracy.\nTable 3 Local-global trade-off\nλ 0.0 0.1 0.2 0.3 0.4 0.5\nAUC 0.916 0.919 0.920 0.922 0.919 0.918 Another approach to analyze the importance of global information is to measure the difference of the target kernel matrix (including global and local information, λ = 0.3) and the local kernel matrix (Figure 4). The left, middle and right panels of Figure 4 are the global, global-local (λ = 0.3) and local kernel matrices respectively. Figure 4 shows that a large area of the global kernel matrix is blue, which means that most values in the global kernel matrix are small. Comparing to global kernel matrix, the values in the local kernel matrix are much larger and the local kernel matrix determine the global-local kernel matrix. That would be the reason why the weight of global information is as high as 30%, while AUC score varies less than 1% (Table 3).\nFigure 4 Kernel matrix of global and local information. The left penal is the global kernel matrix; the middle penal is the local kernel matrix; the right penal is the global-local kernel matrix (λ = 0.3). The global-local kernel matrix and the local kernel matrix are similar, because the norm of global kernel matrix is small with regard to local kernel matrix. Based on the above facts, it is reasonable to infer that the fluctuation caused by global information is limited and local information dominates the binding predictive accuracy, which support our assumption that target-ligand binding is a local event."}