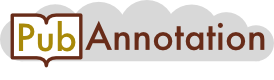
PMC:4331676 / 19102-21288
Annnotations
{"target":"https://pubannotation.org/docs/sourcedb/PMC/sourceid/4331676","sourcedb":"PMC","sourceid":"4331676","source_url":"https://www.ncbi.nlm.nih.gov/pmc/4331676","text":"The selection of LG and features\nTo evaluate the PSSM-DT method, we analyzed the impact of parameter LG on the predictive performance of the proposed model. The predictive results of SVM-PSSM-DT with different values of LG on the benchmark dataset by using five-fold cross validation is shown in Figure 1. As can be seen from the Figure, the value of LG has modest impact on both the ACC and MCC metrics. The ACC firstly increases to a maximum value and then slightly goes down as the LG value increases. So we can conclude that SVM-PSSM-DT achieves the best performance when LG = 5, which mean that the dimension of the feature space applied in this work is 2000. Therefore, the parameter LG was set as 5 for the following experiments.\nFigure 1 The performance of SVM-PSSM-DT with different LG. LG is a parameter in the present method SVM-PSSM-DT. The average ACC and MCC values were used to evaluate the impact of different LG values on the performance of SVM-PSSM-DT. The results were got by testing the model on the benchmark dataset by five-fold-cross-validation. In this study, we proposed three protein representations, including PSSM-DT, PSSM-SDT and PSSM-DDT. Table 1 lists predictive results of the three proposed protein representation according to jackknife validation on benchmark dataset. As a result, the predictor using PSSM-DT yields the highest ACC of 79.96%, MCC of 0.622 and AUC of 86.50%. So in the following experiments, the PSSM-DT based representation was applied to encode the features from PSSM profile.\nTable 1 Results on benchmark dataset of different features through jackknife validation.\nMethods Acc(%) MCC SN(%) SP(%)\nPSSM-DDTa 79.72 0.607 81.33 78.18\nPSSM-SDTb 74.79 0.512 77.147 74.93\nPSSM-DTc 79.96 0.622 81.91 78.00\nPSSM-DT can extract two kinds protein features, called PSSM-DDT and PSSM-SDT respectively. And PSSM-DT represents the combination of PSSM-DDT and PSSM-SDT. The results were got by testing the models on benchmark dataset through jackknife validation.\nathe predictor using PSSM-DDT as protein representation\nbthe predictor using PSSM-SDT as protein representation\ncthe predictor using PSSM-DT as protein representation","divisions":[{"label":"title","span":{"begin":0,"end":32}},{"label":"p","span":{"begin":33,"end":736}},{"label":"figure","span":{"begin":737,"end":1069}},{"label":"label","span":{"begin":737,"end":745}},{"label":"caption","span":{"begin":747,"end":1069}},{"label":"p","span":{"begin":747,"end":1069}},{"label":"p","span":{"begin":1070,"end":1530}},{"label":"label","span":{"begin":1531,"end":1538}},{"label":"caption","span":{"begin":1540,"end":1620}},{"label":"p","span":{"begin":1540,"end":1620}},{"label":"table","span":{"begin":1621,"end":1769}},{"label":"tr","span":{"begin":1621,"end":1655}},{"label":"th","span":{"begin":1621,"end":1628}},{"label":"th","span":{"begin":1630,"end":1636}},{"label":"th","span":{"begin":1638,"end":1641}},{"label":"th","span":{"begin":1643,"end":1648}},{"label":"th","span":{"begin":1650,"end":1655}},{"label":"tr","span":{"begin":1656,"end":1693}},{"label":"td","span":{"begin":1656,"end":1665}},{"label":"td","span":{"begin":1667,"end":1672}},{"label":"td","span":{"begin":1674,"end":1679}},{"label":"td","span":{"begin":1681,"end":1686}},{"label":"td","span":{"begin":1688,"end":1693}},{"label":"tr","span":{"begin":1694,"end":1732}},{"label":"td","span":{"begin":1694,"end":1703}},{"label":"td","span":{"begin":1705,"end":1710}},{"label":"td","span":{"begin":1712,"end":1717}},{"label":"td","span":{"begin":1719,"end":1725}},{"label":"td","span":{"begin":1727,"end":1732}},{"label":"tr","span":{"begin":1733,"end":1769}},{"label":"td","span":{"begin":1733,"end":1741}},{"label":"td","span":{"begin":1743,"end":1748}},{"label":"td","span":{"begin":1750,"end":1755}},{"label":"td","span":{"begin":1757,"end":1762}},{"label":"td","span":{"begin":1764,"end":1769}},{"label":"p","span":{"begin":1770,"end":2019}},{"label":"p","span":{"begin":2020,"end":2075}},{"label":"p","span":{"begin":2076,"end":2131}}],"tracks":[]}