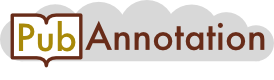
PMC:4307189 / 44222-49962
Annnotations
2_test
{"project":"2_test","denotations":[{"id":"25599599-19671800-14868172","span":{"begin":261,"end":263},"obj":"19671800"},{"id":"25599599-14993899-14868173","span":{"begin":1113,"end":1115},"obj":"14993899"},{"id":"25599599-19671800-14868174","span":{"begin":5376,"end":5378},"obj":"19671800"}],"text":"Validation of the framework using BT474 cell-line (GSE16179)\nTo further illustrate our method, we analysed a second dataset (GSE16179) containing gene expression profiles of breast cancer cell-line BT474 under two conditions (parental and lapatinib resistant) [16]. The reason for choosing this dataset was that it was obtained using a similar experimental design to the primary dataset GSE38376, but with an additional treatment condition using foretinib (GSK1363089) only and with combined drug use (lapatinib + foretinib). There were three samples per treatment condition. However, to adapt simply and be coherent with the previous experiment, we only considered expression values of parental conditions (3 samples with basal condition: GSM799168, GSM799169 and GSM799170; 3 samples with 1 μM of lapatinib treatment: GSM79917, GSM799172 and GSM799173), and the same conditions with lapatinib resistant cells (3 samples with basal condition: GSM799174, GSM799175 and GSM799176; 3 samples with 1 μM of lapatinib treatment: GSM799180, GSM799181 and GSM799182). Among the 375 cancer genes from Cancer Gene Census [23], there were 357 genes which had gene expression values. We identified 27,358 and 26,292 pair-wise gene-gene relationships (undirected edges) in resistant and parental networks by applying the thresholds 0.71 and 0.81, respectively. Bayesian inference of the p1-model parameters for an undirected network was applied to these two gene-gene relationship networks as before. Thereafter, among all 63,546 [= (357 ×356) ÷2] possibilities, we found 10,811 gene-pairs (Additional file 10: Table S9) with the same thresholds of odds ratio (≥10.0) as previously, but smaller posterior probability (≥0.15) of occurring in the resistant network. With this set of putative drug-resistant gene-pairs, we also observed the overlap of potential cross-talks of EGFR/ErbB with other signaling pathways using Reactome, KEGG and WikiPathway databases. We found 83 (72 distinct), 133 (87 distinct) and 277 (81 distinct) cross-talks between EGFR/ErbB and other signaling pathways from Reactome, KEGG and WikiPathway (Additional file 11: Table S10, Additional file 12: Table S11 and Additional file 13: Table S12), respectively. The numbers of signaling pathways that were involved in those EGFR/ErbB cross-talks were 10, 18 and 54, respectively. Among the 83, 133 and 277 cross-talks, we found 50 distinct gene-pairs in at least two of these sets. Table 3 shows the comparative findings between our primary dataset (SKBR3 cell-line, GSE38379) and our secondary dataset (BT474 cell-line, GSE16179). In Table 3, we show that some important signaling pathways that were involved in the EGFR/ErbB cross-talks (i.e. Notch, WNT, GPCR, IR/IGF1R, TGF- β signaling pathways) in our primary dataset, have some overlap with our secondary dataset.\nTable 3 Comparative results between primary dataset (SKBR3 cell-line, GSE38376) and validation dataset (BT474 cell-line, GSE16179)\nPathway name Found in Pathway source Found in Pathway source Common cross-talks in both Studies ¶\n(GSE38376) (GSE16179)\nNotch Signaling Reactome, Reactome, MAP2K4::NOTCH1\nKEGG, KEGG,\nWikiPathway WikiPathway\nGPCR signaling Reactome, Reactome, CBL::TSHR\nWikiPathway WikiPathway FGFR1::TSHR\nPDGFRA::GNAQ\nKIT::TSHR\nLCK::TSHR\nMDM2::TSHR\nPDGFRA::TSHR\nWNT Signaling Reactome, Reactome, AKT2::CCND2\nKEGG, KEGG, MAP2K4::CCND2\nWikiPathway WikiPathway MAP2K4::TP53\nMDM2::MAP2K4\nInsulin (IGF1R) Signaling Reactome, Reactome, MDM2::MAP2K4\nWikiPathway WikiPathway TP53::MAP2K4\nTGF- β Signaling Reactome, Reactome, MDM2::TFE3\nWikiPathway KEGG, TP53::TFE3\nWikiPathway\nMAPK signaling KEGG, KEGG, MDM2::MAP2K4\nWikiPathway WikiPathway\n¶These common cross-talks were found using the primary dataset (104, 188 and 299 cross-talks from Reactome, KEGG and WikiPathway databases, respectively) and validation datasets (83, 133 and 277 cross-talks from Reactome, KEGG and WikiPathway databases, respectively). Cross-talks mentioned with Bold face are those consistent with our hypothesis that both genes in the particular cross-talk are up-regulated in resistant conditions but down-regulated in parental conditions.\nThere were 78 genes involved in these sets of 83, 133 and 277 putative cross-talks. We performed a similar Netwalker analyses with these 78 genes as we did for the dataset GSE38376, and found 37 genes (involved in 86 cross-talks (Additional file 14: Table S13)) consistent with our hypothesis that both genes in a particular cross-talk should be up-regulated in resistant conditions but down-regulated in parental conditions. In Figure 4, the selected genes from the secondary dataset exhibit an even clearer pattern of up-regulation in resistant conditions than the selected genes from our primary dataset.\nFigure 4 Heatmap of genes in putative drug-resistant cross-talks in breast cancer cell-line: BT474 (GSE16179). Heatmap image of comparative gene expression changes of parental and resistant conditions in (A) all 78 genes in all 83, 133 and 277 putative drug-resistant cross-talks using signaling pathways from Reactome, KEGG and WikiPathway database, respectively, and (B) 37 selected genes based on their differential regulation. Here, for each gene, the expression value at each of the 4 conditions (2 parental conditions, and 2 resistant conditions) is the average value of 3 sample patients [16]. For each gene, these 4 expression values (each of them is the average of 3 samples) were transformed into z-scores (zero mean, unit standard deviation) and each z-score was normalized with the maximum absolute value of the z-scores across that particular gene. For both (A) and (B), red and green bars indicate up-regulation and down-regulation, respectively."}