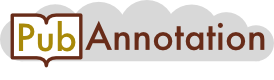
PMC:4157143 / 19704-33812
Annnotations
{"target":"https://pubannotation.org/docs/sourcedb/PMC/sourceid/4157143","sourcedb":"PMC","sourceid":"4157143","source_url":"https://www.ncbi.nlm.nih.gov/pmc/4157143","text":"Results\nWe set out to develop an improved understanding of the impact and interpretability of rare noncoding variants. Our approach involved combining high-quality genomes and transcriptomes within a single large family to identify cis-eQTLs and compare these to cis-eQTLs discovered in a large population sample. Through the use of RNA-seq data, we were also able to conduct comparable analyses for alternative splicing and ASE. Our analyses focused on the enrichment of rare and potentially regulatory variants in large-effect eQTLs and sQTLs in the family, and we sought to identify the properties of genes that exhibit such effects. Furthermore, we investigated the degree to which family transcriptome data enable the detection of noncoding annotation relevant to interpreting rare noncoding variants genome-wide.\n\nFamily Transcriptome Sequencing Identifies Large-Effect cis-eQTLs\nWe hypothesized that rare variants acting either alone or in combination with common variants can cause an eQTL to exhibit a larger effect size in the family than in the population. To identify such cases, we applied a ranking scheme in which we compared gene-expression cis-eQTLs between the family and the population to find genes that exhibited larger effect sizes within the family (see Material and Methods). At CI \u003e 0.95 (or empirical p value \u003c 0.05), we found that 319 (including both paternal and maternal β measurements) of the 7,341 genes we tested had effect sizes exceeding that of the best population cis-eQTL SNP (false-discovery rate [FDR] = 7,341 × 0.05 × 2 / 319 \u003e 1; Figure 1A). Using comparisons of β, we did not find more relatively large-effect eQTLs than we would expect by chance; however, we identified that this FDR is likely overconservative primarily because of differences in noise between the family and population (see Figures S17–S19), and we therefore also discuss less conservative estimates of FDR (see Figures S17–S19). It is important to note that FDR here measures whether there are more large effects in the family than in the population; however, ranking relative effect sizes by empirical p values is biologically meaningful whether there is an excess or a depletion. Such relative effects overlap (to a degree) genes measured only by absolute effect size in the family; for instance, when comparing genes at the 95% percentile for absolute β versus relative β, we observed an overlap of 52% (Figure S12). However, we chose to use in all subsequent analyses the ranking of genes according to their relative effect sizes instead of absolute effect sizes because we hypothesized that the former might better inform family-specific effects. By instead measuring fit (R2), we identified 577 cis-eQTLs that had a better fit in the family than the best population-level cis-eQTL variant (CI \u003e 0.95; FDR = 7,341 × 0.05 / 577 = 63%; Figure S10). Among those genes that exhibited the largest effect sizes and fits in the family (both at a CI \u003e 0.95), there was a significant overlap of 36.4% (Figure S11). To exclude the possibility of technical factors underlying effect-size differences, we repeated the analysis by using different quantification pipelines (Figures S13 and S14), population discovery-panel sizes (Table S6), and alternative methods for choosing the best SNP (Figure S16); we observed no significant difference in the discovery set of large-effect genes or on further downstream analyses (see Material and Methods).\nWe also identified genes that exhibited larger ASE effects in the family than in the population. We found that 223 of the 1,777 genes we tested had larger ASE effect sizes in the family (CI \u003e 0.95, FDR = 1,777 × 0.05 / 223 = 40%; Figure 1B; Figure S25). We expected that on an individual basis, the family and population would actually have the same distribution of ASE effect sizes (no excess of large effects, FDR = 1). We controlled for some initially observed excess in the family by matching read depths via downsampling; however, this did not address all the excess in the family, and unknown factors still remained. We expected that any excess, however, would only add noise to subsequent rare-variant enrichment analyses, and we further validated large ASE effects by using evidence from IBD siblings (Figure S25). In addition, we applied ASE to support discoveries of cis-eQTLs in the family; by stratifying their degree of effect size relative to those in the population, we detected a proportionally increased enrichment of detectable ASE (significant ASE sites defined as allelic imbalance \u003e 0.05, binomial test p value \u003c 0.05; Figure S21). This relationship supports a potential regulatory role of rare variants because it indicates that large-effect cis-eQTLs in the family might be the consequence of heterozygous variants that manifest in ASE. This idea is further supported by our observation of a direct and simple linear relationship between cis-eQTL effect size among children and ASE effect size among parents (Figure S20).\n\nLarge-Effect cis-eQTLs in the Family Are Enriched with Rare Variants\nWe hypothesized that rare noncoding variants might be responsible for a considerable proportion of the large-effect-size cis-eQTLs in the family. Taking advantage of full genome data in the family, we assessed the enrichment of rare and potentially regulatory variants near the transcription start site (TSS) of genes with different magnitudes of relative effect sizes between the family and the population. Here, we used PhyloP to define potentially regulatory variants on the basis of ENCODE TF peaks, DNase I hypersensitivity peaks, and evolutionarily constrained regions across 99 vertebrate genomes; we will later further explore the relative importance of each of these annotations. We observed enrichment of rare and potentially regulatory noncoding variants in genes that had the largest effect sizes (CI \u003e 0.95 and CI \u003e 0.80; Figure 2A). This relationship was most pronounced within the first 5 kb close to the TSS and decayed as a function of distance. It was also related to the degree to which the family effect was larger than that detected in the population across the full distribution of measured effects (Figure 2B). Likewise, we tested both large-effect cis-eQTLs by fit (R2) and large-effect ASE genes and observed similar strong enrichment of rare and potentially regulatory variants (Figures S23 and S25C).\nWe also evaluated the utility of known regulatory annotations in predicting eQTLs for rare variants. Comparing annotated rare variants with all rare variants, we observed strong enrichment (up to a 2-fold increase near the TSS) of annotated variants, indicating that annotation is highly informative in predicting eQTLs (Figure S22). Furthermore, we observed that the enrichment was higher in family-based eQTLs than in population eQTLs as a function of effect size (Figure S24). To test the contribution of different annotations to a large effect in the family, we further stratified by MAF and strength of evolutionary constraint. We observed that variants with lower MAF and with increasing degree of evolutionary constraint were the most informative factors indicative of large cis-eQTL effects in the family (Figure 2C).\n\nLarge-Effect cis-eQTLs in the Family Influence Essential Genes\nIt has been previously reported that cis-eQTLs based on population studies are depleted among essential genes.19 We hypothesized that if rare variation was indeed responsible for large-effect cis-eQTLs in the family, reduced impact of purifying selection on rare variants would result in family eQTLs disproportionately affecting essential genes. We tested this hypothesis in two ways: defining gene essentiality by (1) its degree of evolutionary constraint and (2) its centrality within a PPI network. To assess evolutionary constraint, we used dN/dS ratios between humans and chimps to compare large-effect cis-eQTL genes in the family to cis-eQTL genes in the population. We observed that large-effect cis-eQTL genes in the family had significantly higher conservation status than population cis-eQTL genes (Figure 3A). This was even more pronounced for genes with a rare and potentially regulatory variant within 5 kb of the TSS. By contrast, cis-eQTL genes in the population were less constrained for increasingly stringent p values.\nWe next applied PPI networks with the premise that genes that are more central in the network or have more connections to other genes are more essential than less connected ones. We found significantly higher connectivity for large-effect cis-eQTL genes in the family than for cis-eQTL genes in the population (Figure 3B). Furthermore, this contrast became stronger when we focused only on those genes that also contained a proximal rare and potentially regulatory variant (Figure 3B).\n\nFamily Transcriptome Sequencing Identifies Large-Effect sQTLs\nBy comparing cis-sQTLs between the family and the population, we also ranked genes with larger relative effect sizes (measured as R2) in the family than in the population (n = 726, \u003e95% population, n = 5,622 genes; FDR = 39%). Differences in isoform-quantification pipelines probably overestimate the excess number of large-effect sQTLs because there is also more noise in isoform quantification in the population. However, as for large-effect eQTLs, we also observed enrichment of rare and potentially functional variants for large-effect sQTL genes in the family (Figure 4A). Furthermore, by stratifying on allele frequency, distance to splice sites, and evolutionary-constraint thresholds, we found that large-effect sQTLs in the family were much better predicted by rare variants than by common variants, especially for conserved regions near splice sites (Figure 4B). In addition to observing large effect sizes, we also found that sQTLs could exhibit very high heritabilities, nearly as high as those for Mendelian traits (examples in Figures S26 and S27).\n\nLarge-Effect cis-eQTLs in the Family Might Further Modify Complex-Disease-Associated Genes\nThere has been considerable interest in whether rare variants modify risk of complex disease.46,47 Although we were unable to directly test disease associations within this family because of the anonymity of the individuals, we sought to quantify the number of genome-wide association study (GWAS) genes in which cis-eQTLs exhibited larger effects in the family than in the population. We identified 315 GWAS genes in which the known GWAS variant was an eQTL in the population (at an FDR of 5%), suggesting a regulatory basis to disease pathogenesis. Of these genes, we identified 65 with a larger-effect cis-eQTL in the family (\u003e80th percentile). Of those, 17 (Table S9) were not polymorphic for the known GWAS SNP in the family, and two had a rare and potentially regulatory variant (within \u003c100 kb of the TSS, within an ENCODE TF binding and DNase I hypersensitivity peak, and with a PhyloP score \u003e 0) influencing genes implicated in body mass index, hypertension, and obesity. In addition, regardless of relative effect sizes of eQTLs between the family and population, we identified four GWAS genes (Table S10) in which the known GWAS SNP was an eQTL in the population and that had a rare and potentially regulatory variant (within \u003c100 kb of the TSS, within an ENCODE TF binding and DNase I hypersensitivity peak, and with a PhyloP score \u003e 3) in the family according to strong predictor variables. Although increased risk in this family is not known, the presence of rare and potentially regulatory variants in complex-disease-associated genes whose expression is implicated in disease pathogenesis suggests that complex traits and genes should be further studied with rare-variant association tests.\n\nFunctional Noncoding Annotations Are Informative of the Impact of Rare Noncoding Variants\nGenome and transcriptome data from a single large family allowed us to test the utility of various noncoding annotations for predicting the impact of noncoding variants on expression. Here, our goal was to identify those annotations that could inform a functional variant from genome sequence alone. We chose to include the following as potentially informative annotations: ENCODE TF binding, DNase I hypersensitivity peaks, evolutionary constraint, motif disruption as computed by HaploReg, and distance to the TSS. We identified that each noncoding annotation was more informative for predicting the impact of rare variants than the impact of common variants on expression (Figure 5A; Table S7). We observed that evolutionary constraint and distance to the TSS were the most informative for rare variants, and they further increased their utility with increasing strength of constraint and shorter distances, respectively. One potential concern we identified is that we might be only predicting a gene’s ability to harbor an eQTL such that having a rare variant possessing specific annotation might indirectly inform genes tolerant of arbitrary functional variants (both common and rare). However, when assessing whether genes containing different annotations for rare variants were also more likely to have common eQTLs in the population, we saw no significant difference (Figure 5A, right panel). This demonstrates that particular species of rare noncoding variants might be interpretable from genome sequence data alone provided that there is sufficiently high-confidence genotyping of those rare variants. Furthermore, provided increasing availability of genome-interpretation methods, this method offers a means of determining and calibrating the efficacy of different approaches.\nThrough finer stratification of allele frequency, we were able to observe the degree to which genome annotation influenced predictions of cis-eQTLs. We observed that predictions of eQTLs were most informative for potentially regulatory variants when those variants were rare (Figure 5B). This was also the case for sQTLs: predictor variables such as evolutionary constraint and distance to splice sites were the most informative factors for predicting a sQTL when a variant was rare (Figure 5C).","divisions":[{"label":"title","span":{"begin":0,"end":7}},{"label":"p","span":{"begin":8,"end":818}},{"label":"sec","span":{"begin":820,"end":4995}},{"label":"title","span":{"begin":820,"end":885}},{"label":"p","span":{"begin":886,"end":3450}},{"label":"p","span":{"begin":3451,"end":4995}},{"label":"sec","span":{"begin":4997,"end":7219}},{"label":"title","span":{"begin":4997,"end":5065}},{"label":"p","span":{"begin":5066,"end":6393}},{"label":"p","span":{"begin":6394,"end":7219}},{"label":"sec","span":{"begin":7221,"end":8808}},{"label":"title","span":{"begin":7221,"end":7283}},{"label":"p","span":{"begin":7284,"end":8322}},{"label":"p","span":{"begin":8323,"end":8808}},{"label":"sec","span":{"begin":8810,"end":9934}},{"label":"title","span":{"begin":8810,"end":8871}},{"label":"p","span":{"begin":8872,"end":9934}},{"label":"sec","span":{"begin":9936,"end":11733}},{"label":"title","span":{"begin":9936,"end":10026}},{"label":"p","span":{"begin":10027,"end":11733}},{"label":"title","span":{"begin":11735,"end":11824}},{"label":"p","span":{"begin":11825,"end":13612}}],"tracks":[{"project":"2_test","denotations":[{"id":"25192044-24092820-2055646","span":{"begin":7394,"end":7396},"obj":"24092820"},{"id":"25192044-22251874-2055647","span":{"begin":10123,"end":10125},"obj":"22251874"},{"id":"25192044-20479773-2055647","span":{"begin":10123,"end":10125},"obj":"20479773"}],"attributes":[{"subj":"25192044-24092820-2055646","pred":"source","obj":"2_test"},{"subj":"25192044-22251874-2055647","pred":"source","obj":"2_test"},{"subj":"25192044-20479773-2055647","pred":"source","obj":"2_test"}]}],"config":{"attribute types":[{"pred":"source","value type":"selection","values":[{"id":"2_test","color":"#9793ec","default":true}]}]}}