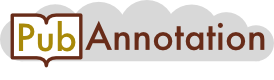
PMC:2667511 / 31027-32511
Annnotations
{"target":"https://pubannotation.org/docs/sourcedb/PMC/sourceid/2667511","sourcedb":"PMC","sourceid":"2667511","source_url":"https://www.ncbi.nlm.nih.gov/pmc/2667511","text":"To select a set of informative and discriminative rules for the extraction of knowledge, most of the existing approaches rank the association rules based on the confidence value of a individual rule. A strong rule which is highly confident and represents general knowledge, may not be a good discriminative rule for the classification. Instead, a better measure of the importance of a rule should include the following factors considered together: correlation between a property and a class, the degree of classification power, confidence and support, top K coverage and uniqueness of a rule. As noted in the previous section, the inclusion of the SSE content information in our ARBC approach has a positive effect on the classification accuracy (Table 4). The importance of a rule can be quantified by integrating the various factors including the SSE content information. We defined a importance factor (I in Tables 6 and 7) by an average value of all the factors. In order to illustrate the informativeness of the rules in understanding interface features, some representative rules within the top 30% (ranked higher than 48) of I are listed in Table 6. The list was complemented by some other rules ranked below 48 in order to explain overlapping rules and compare association rules to rules generated from a decision tree. Similarly, rules describing the ENZ type with varying different structural features are listed in Table 7. Rules in Tables 6 and 7 are sorted by Type and I.","tracks":[]}