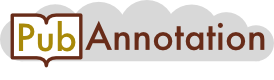
PMC:1852316 / 35504-38065
Annnotations
{"target":"https://pubannotation.org/docs/sourcedb/PMC/sourceid/1852316","sourcedb":"PMC","sourceid":"1852316","source_url":"https://www.ncbi.nlm.nih.gov/pmc/1852316","text":"To construct a synthetic TRN, for each TF we assigned un = c1 + c2e−c3z MathType@MTEF@5@5@+=feaafiart1ev1aaatCvAUfKttLearuWrP9MDH5MBPbIqV92AaeXatLxBI9gBaebbnrfifHhDYfgasaacH8akY=wiFfYdH8Gipec8Eeeu0xXdbba9frFj0=OqFfea0dXdd9vqai=hGuQ8kuc9pgc9s8qqaq=dirpe0xb9q8qiLsFr0=vr0=vr0dc8meaabaqaciaacaGaaeqabaqabeGadaaakeaacqWGJbWydaWgaaWcbaGaeGOmaidabeaakiabdwgaLnaaCaaaleqabaGaeyOeI0Iaem4yam2aaSbaaWqaaiabiodaZaqabaWccqWG6bGEaaaaaa@3588@ where c1, c2, c3 are constants (taken to be 0.02, 0.15, and 5, respectively) and z is a random number (between 0 and 1). Then for each gene/TF pair, we assigned a random number hin (between 0 and 1). For parameter e, which determines how dense the synthetic TRN is, if hinun \u003ce we set bin = -1 (down regulation), if e ≤ hinun \u003c 2e, we set bin = 1 (up regulation), assuming the probability of up and down regulation is the same. The Qin were allowed to change 20 fold and were generated randomly (in the logarithmic scale). TF activities were assumed to be random as well. Our synthetic examples with large TRNs show that, despite the simplicity of the FTF approach, the constructed TF activity profiles are reliable. To test the approach, one can compare the TF activities constructed and those used in the generation of synthetic expression data. For example, for a TRN that has the properties shown in Fig. 2, even when we eliminate 50% of the TRN to create a \"preliminary TRN\", 90% of the constructed TF activities have a Pearson correlation coefficient of at least 0.70 with the TF activities used to generate the synthetic expression data (when 20 or more microarray experimental conditions were used). Fig. 3 shows the dependence of the results on the number of experiments. This graph shows that, for practical reason, it is not feasible to recover the full network. Fig. 4a shows the effect of network structure on the results. As the network gets denser, the percentage of the network that can be recovered decreases. Fig. 4b illustrates the dependence of the percentage of recovery on the degree of incompleteness in the preliminary TRN. As anticipated, more complete preliminary TRNs allow a higher percentage of the unknown part of the network to be recovered using expression data. These results suggest that in a real world application such as E. coli (for which we have probably less than 40% of the TRN – based on the number of gene/TF interactions known and expected number of TFs), one can not expect to construct the full TRN using expression data alone, regardless of the number of expression datasets available.","tracks":[]}