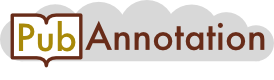
PMC:1698483 / 30284-118346
Annnotations
{"target":"https://pubannotation.org/docs/sourcedb/PMC/sourceid/1698483","sourcedb":"PMC","sourceid":"1698483","source_url":"https://www.ncbi.nlm.nih.gov/pmc/1698483","text":"The EXMOTIF algorithm\nWe first introduce our basic approach for common structured motif extraction problem. We then successively optimize it for various practical scenarios.\n\nThe basic approach\nLet's assume that we are extracting all structured motif instances from n sequence S MathType@MTEF@5@5@+=feaafiart1ev1aaatCvAUfKttLearuWrP9MDH5MBPbIqV92AaeXatLxBI9gBamrtHrhAL1wy0L2yHvtyaeHbnfgDOvwBHrxAJfwnaebbnrfifHhDYfgasaacH8akY=wiFfYdH8Gipec8Eeeu0xXdbba9frFj0=OqFfea0dXdd9vqai=hGuQ8kuc9pgc9s8qqaq=dirpe0xb9q8qiLsFr0=vr0=vr0dc8meaabaqaciaacaGaaeqabaWaaeGaeaaakeaaimaacqWFse=uaaa@3845@ = {Si, 1 ≤ i ≤ n}, each of which satisfies the template T MathType@MTEF@5@5@+=feaafiart1ev1aaatCvAUfKttLearuWrP9MDH5MBPbIqV92AaeXatLxBI9gBamrtHrhAL1wy0L2yHvtyaeHbnfgDOvwBHrxAJfwnaebbnrfifHhDYfgasaacH8akY=wiFfYdH8Gipec8Eeeu0xXdbba9frFj0=OqFfea0dXdd9vqai=hGuQ8kuc9pgc9s8qqaq=dirpe0xb9q8qiLsFr0=vr0=vr0dc8meaabaqaciaacaGaaeqabaWaaeGaeaaakeaaimaacqWFtepvaaa@3847@ and occurs at least in q sequences of S MathType@MTEF@5@5@+=feaafiart1ev1aaatCvAUfKttLearuWrP9MDH5MBPbIqV92AaeXatLxBI9gBamrtHrhAL1wy0L2yHvtyaeHbnfgDOvwBHrxAJfwnaebbnrfifHhDYfgasaacH8akY=wiFfYdH8Gipec8Eeeu0xXdbba9frFj0=OqFfea0dXdd9vqai=hGuQ8kuc9pgc9s8qqaq=dirpe0xb9q8qiLsFr0=vr0=vr0dc8meaabaqaciaacaGaaeqabaWaaeGaeaaakeaaimaacqWFse=uaaa@3845@. We assume for the moment that no substitutions are allowed in any of the simple motifs. We also assume that all Si ∈ S MathType@MTEF@5@5@+=feaafiart1ev1aaatCvAUfKttLearuWrP9MDH5MBPbIqV92AaeXatLxBI9gBamrtHrhAL1wy0L2yHvtyaeHbnfgDOvwBHrxAJfwnaebbnrfifHhDYfgasaacH8akY=wiFfYdH8Gipec8Eeeu0xXdbba9frFj0=OqFfea0dXdd9vqai=hGuQ8kuc9pgc9s8qqaq=dirpe0xb9q8qiLsFr0=vr0=vr0dc8meaabaqaciaacaGaaeqabaWaaeGaeaaakeaaimaacqWFse=uaaa@3845@, 1 ≤ i ≤ n and the extracted motifs are over the DNA alphabet, ΣDNA. EXMOTIF first converts each Si ∈ S MathType@MTEF@5@5@+=feaafiart1ev1aaatCvAUfKttLearuWrP9MDH5MBPbIqV92AaeXatLxBI9gBamrtHrhAL1wy0L2yHvtyaeHbnfgDOvwBHrxAJfwnaebbnrfifHhDYfgasaacH8akY=wiFfYdH8Gipec8Eeeu0xXdbba9frFj0=OqFfea0dXdd9vqai=hGuQ8kuc9pgc9s8qqaq=dirpe0xb9q8qiLsFr0=vr0=vr0dc8meaabaqaciaacaGaaeqabaWaaeGaeaaakeaaimaacqWFse=uaaa@3845@, 1 ≤ i ≤ n into an equivalent inverted format [25], where we associate with each symbol in the sequence Si its pos-list, a sorted list of the positions where the symbol occurs in Si. Then for each symbol we combine its pos-list in each Si to obtain its pos-list in S MathType@MTEF@5@5@+=feaafiart1ev1aaatCvAUfKttLearuWrP9MDH5MBPbIqV92AaeXatLxBI9gBamrtHrhAL1wy0L2yHvtyaeHbnfgDOvwBHrxAJfwnaebbnrfifHhDYfgasaacH8akY=wiFfYdH8Gipec8Eeeu0xXdbba9frFj0=OqFfea0dXdd9vqai=hGuQ8kuc9pgc9s8qqaq=dirpe0xb9q8qiLsFr0=vr0=vr0dc8meaabaqaciaacaGaaeqabaWaaeGaeaaakeaaimaacqWFse=uaaa@3845@. More formally, for a symbol X ∈ ΣDNA, its pos-list in Si is given as P (X, Si) = {j | Si[j] = X, j ∈ [1, |Si|]}, where Si[j] is the symbol at position j in Si, and |Si| denotes the length of Si. Its pos-list across all sequences S MathType@MTEF@5@5@+=feaafiart1ev1aaatCvAUfKttLearuWrP9MDH5MBPbIqV92AaeXatLxBI9gBamrtHrhAL1wy0L2yHvtyaeHbnfgDOvwBHrxAJfwnaebbnrfifHhDYfgasaacH8akY=wiFfYdH8Gipec8Eeeu0xXdbba9frFj0=OqFfea0dXdd9vqai=hGuQ8kuc9pgc9s8qqaq=dirpe0xb9q8qiLsFr0=vr0=vr0dc8meaabaqaciaacaGaaeqabaWaaeGaeaaakeaaimaacqWFse=uaaa@3845@ is obtained by grouping the pos-lists of each sequence, and is given as P (X, S MathType@MTEF@5@5@+=feaafiart1ev1aaatCvAUfKttLearuWrP9MDH5MBPbIqV92AaeXatLxBI9gBamrtHrhAL1wy0L2yHvtyaeHbnfgDOvwBHrxAJfwnaebbnrfifHhDYfgasaacH8akY=wiFfYdH8Gipec8Eeeu0xXdbba9frFj0=OqFfea0dXdd9vqai=hGuQ8kuc9pgc9s8qqaq=dirpe0xb9q8qiLsFr0=vr0=vr0dc8meaabaqaciaacaGaaeqabaWaaeGaeaaakeaaimaacqWFse=uaaa@3845@) = {⟨ i, | P (X, Si)|, P (X, Si)⟩ | Si ∈ S MathType@MTEF@5@5@+=feaafiart1ev1aaatCvAUfKttLearuWrP9MDH5MBPbIqV92AaeXatLxBI9gBamrtHrhAL1wy0L2yHvtyaeHbnfgDOvwBHrxAJfwnaebbnrfifHhDYfgasaacH8akY=wiFfYdH8Gipec8Eeeu0xXdbba9frFj0=OqFfea0dXdd9vqai=hGuQ8kuc9pgc9s8qqaq=dirpe0xb9q8qiLsFr0=vr0=vr0dc8meaabaqaciaacaGaaeqabaWaaeGaeaaakeaaimaacqWFse=uaaa@3845@}, where i is the sequence identifier of Si, and | P (X, Si)| denotes the cardinality of the pos-list P (X, Si) in sequence Si. For our example sequences in Table 1, the pos-list for each DNA base is given in Table 2. For example, A occurs in sequence S1 at the positions {5, 9, 10, 15, 16, 17}, thus the entries in A's pos-list are {1, 6, 5, 9, 10, 15, 16, 17}.\nTable 2 Pos-lists.\nX pos-lists\nA {1,6,5,9,10,15,16,17, 2,5,6,8,11,12,15, 3,4,2,6,7,10, 4,3,2,3,7}\nC {1,7,1,2,6,7,11,12,14, 2,4,1,2,13,14, 3,3,8,9,12, 4,4,4,9,10,11}\nG {1,2,3,8, 2,3,3,9,10, 3,2,4,5, 4, 2,5,6}\nT {1,2,4,13, 2,5,4,5,7,16,17 3,5,1,3,11,13,14, 4,5,1,8,12,13,14}\nSequence identifiers (i) and cardinality of P (X, Si) are marked in bold.\n\nPositional joins\nWe first extend the notion of pos-lists to cover structured motifs. The pos-list of ℳ MathType@MTEF@5@5@+=feaafiart1ev1aaatCvAUfKttLearuWrP9MDH5MBPbIqV92AaeXatLxBI9gBamrtHrhAL1wy0L2yHvtyaeHbnfgDOvwBHrxAJfwnaebbnrfifHhDYfgasaacH8akY=wiFfYdH8Gipec8Eeeu0xXdbba9frFj0=OqFfea0dXdd9vqai=hGuQ8kuc9pgc9s8qqaq=dirpe0xb9q8qiLsFr0=vr0=vr0dc8meaabaqaciaacaGaaeqabaWaaeGaeaaakeaaimaacqWFZestaaa@3790@ in Si ∈ S MathType@MTEF@5@5@+=feaafiart1ev1aaatCvAUfKttLearuWrP9MDH5MBPbIqV92AaeXatLxBI9gBamrtHrhAL1wy0L2yHvtyaeHbnfgDOvwBHrxAJfwnaebbnrfifHhDYfgasaacH8akY=wiFfYdH8Gipec8Eeeu0xXdbba9frFj0=OqFfea0dXdd9vqai=hGuQ8kuc9pgc9s8qqaq=dirpe0xb9q8qiLsFr0=vr0=vr0dc8meaabaqaciaacaGaaeqabaWaaeGaeaaakeaaimaacqWFse=uaaa@3845@ is given as the set of start positions of all the matches of ℳ MathType@MTEF@5@5@+=feaafiart1ev1aaatCvAUfKttLearuWrP9MDH5MBPbIqV92AaeXatLxBI9gBamrtHrhAL1wy0L2yHvtyaeHbnfgDOvwBHrxAJfwnaebbnrfifHhDYfgasaacH8akY=wiFfYdH8Gipec8Eeeu0xXdbba9frFj0=OqFfea0dXdd9vqai=hGuQ8kuc9pgc9s8qqaq=dirpe0xb9q8qiLsFr0=vr0=vr0dc8meaabaqaciaacaGaaeqabaWaaeGaeaaakeaaimaacqWFZestaaa@3790@ in Si. Let X, Y ∈ ΣDNA be any two symbols, and let ℳ MathType@MTEF@5@5@+=feaafiart1ev1aaatCvAUfKttLearuWrP9MDH5MBPbIqV92AaeXatLxBI9gBamrtHrhAL1wy0L2yHvtyaeHbnfgDOvwBHrxAJfwnaebbnrfifHhDYfgasaacH8akY=wiFfYdH8Gipec8Eeeu0xXdbba9frFj0=OqFfea0dXdd9vqai=hGuQ8kuc9pgc9s8qqaq=dirpe0xb9q8qiLsFr0=vr0=vr0dc8meaabaqaciaacaGaaeqabaWaaeGaeaaakeaaimaacqWFZestaaa@3790@ = X[l, u]Y be a structured motif. Given the pos-lists of X and Y in Si for 1 ≤ i ≤ n, namely, P (X, Si) and P (Y, Si), the pos-list of ℳ MathType@MTEF@5@5@+=feaafiart1ev1aaatCvAUfKttLearuWrP9MDH5MBPbIqV92AaeXatLxBI9gBamrtHrhAL1wy0L2yHvtyaeHbnfgDOvwBHrxAJfwnaebbnrfifHhDYfgasaacH8akY=wiFfYdH8Gipec8Eeeu0xXdbba9frFj0=OqFfea0dXdd9vqai=hGuQ8kuc9pgc9s8qqaq=dirpe0xb9q8qiLsFr0=vr0=vr0dc8meaabaqaciaacaGaaeqabaWaaeGaeaaakeaaimaacqWFZestaaa@3790@ in Si can be obtained by a positional join as follows: for a position x ∈ P (X, Si), if there exists a position y ∈ P (Y, Si), such that l ≤ y - x - 1 ≤ u, it means that Y follows X within the variable gap range [l, u] in the sequence Si, and thus we can add x to the pos-list of motif X[l, u]Y. Let d be the number of gaps between x ∈ P (X, Si) and y ∈ P (Y, Si), given as d = y - x - 1.\nThen, in general, there are three cases to consider in the positional join algorithm:\n• d \u003cl: Advance y to the next element in P (Y, Si).\n• d \u003e u: Advance x to the next element in P (X, Si).\n• l ≤ d ≤ u: Save this occurrence in P (X[l, u]Y, Si), and then advance x to the next element in P (X, Si).\nThe pos-list for X[l, u]Y can be computed in time linear in the lengths of P (X, Si) and P (Y, Si), i.e., the complexity of a positional join is O(|P (X, Si)| + |P (Y, Si)|). In essence, each time we advance x ∈ P (X, Si), we check if there exists a y ∈ P (Y, Si) that satisfies the given gap constraint. Instead of searching for the matching y from the beginning of the pos-list each time, we search from the last position used to compare with x. This results in fast positional joins. For example, during the positional join for the motif A[0,1]T in S4, with l = 0 and u = 1, we scan the pos-lists of A and T for S4 in Table 2, i.e. P (X, S4) = {2, 3, 7} and P (Y, S4) = {1, 8, 12, 13, 14}. Initially, x = 2 and y = 1. This gives d = 1 - 2 - 1 = - 2 \u003cl, thus we advance y to 8. Next, d = 8 - 2 - 1 = 5 \u003e u, thus we advance x to 3. Then, d = 8 - 3 - 1 = 4 \u003e u, thus we advance x to 7. Next, d = 8 - 7 - 1 = 0 ∈ [l, u], so we store x = 7 in P (A[0, 1]T, S4). We would advance x but since we have already reached the end of P (A, S4), the positional join stops. Thus the final pos-list of A[0,1]T in S4 is: P (A[0, 1]T, S4) = {7}. After we obtain the pos-list of ℳ MathType@MTEF@5@5@+=feaafiart1ev1aaatCvAUfKttLearuWrP9MDH5MBPbIqV92AaeXatLxBI9gBamrtHrhAL1wy0L2yHvtyaeHbnfgDOvwBHrxAJfwnaebbnrfifHhDYfgasaacH8akY=wiFfYdH8Gipec8Eeeu0xXdbba9frFj0=OqFfea0dXdd9vqai=hGuQ8kuc9pgc9s8qqaq=dirpe0xb9q8qiLsFr0=vr0=vr0dc8meaabaqaciaacaGaaeqabaWaaeGaeaaakeaaimaacqWFZestaaa@3790@ in each Si for 1 ≤ i ≤ n, we can combine them together to obtain the pos-list of ℳ MathType@MTEF@5@5@+=feaafiart1ev1aaatCvAUfKttLearuWrP9MDH5MBPbIqV92AaeXatLxBI9gBamrtHrhAL1wy0L2yHvtyaeHbnfgDOvwBHrxAJfwnaebbnrfifHhDYfgasaacH8akY=wiFfYdH8Gipec8Eeeu0xXdbba9frFj0=OqFfea0dXdd9vqai=hGuQ8kuc9pgc9s8qqaq=dirpe0xb9q8qiLsFr0=vr0=vr0dc8meaabaqaciaacaGaaeqabaWaaeGaeaaakeaaimaacqWFZestaaa@3790@ in S MathType@MTEF@5@5@+=feaafiart1ev1aaatCvAUfKttLearuWrP9MDH5MBPbIqV92AaeXatLxBI9gBamrtHrhAL1wy0L2yHvtyaeHbnfgDOvwBHrxAJfwnaebbnrfifHhDYfgasaacH8akY=wiFfYdH8Gipec8Eeeu0xXdbba9frFj0=OqFfea0dXdd9vqai=hGuQ8kuc9pgc9s8qqaq=dirpe0xb9q8qiLsFr0=vr0=vr0dc8meaabaqaciaacaGaaeqabaWaaeGaeaaakeaaimaacqWFse=uaaa@3845@. For example, the full pos-list of A[0,1]T for S MathType@MTEF@5@5@+=feaafiart1ev1aaatCvAUfKttLearuWrP9MDH5MBPbIqV92AaeXatLxBI9gBamrtHrhAL1wy0L2yHvtyaeHbnfgDOvwBHrxAJfwnaebbnrfifHhDYfgasaacH8akY=wiFfYdH8Gipec8Eeeu0xXdbba9frFj0=OqFfea0dXdd9vqai=hGuQ8kuc9pgc9s8qqaq=dirpe0xb9q8qiLsFr0=vr0=vr0dc8meaabaqaciaacaGaaeqabaWaaeGaeaaakeaaimaacqWFse=uaaa@3845@ is: {2, 2, 6, 15, 3, 2, 2, 10, 4, 1, 7}. Thus the support of A[0,1]T is 3. Note here for each non-empty pos-list, we insert its sequence identifier and length before it. The pseudo-code for the positional joins for a given sequence Si ∈ S MathType@MTEF@5@5@+=feaafiart1ev1aaatCvAUfKttLearuWrP9MDH5MBPbIqV92AaeXatLxBI9gBamrtHrhAL1wy0L2yHvtyaeHbnfgDOvwBHrxAJfwnaebbnrfifHhDYfgasaacH8akY=wiFfYdH8Gipec8Eeeu0xXdbba9frFj0=OqFfea0dXdd9vqai=hGuQ8kuc9pgc9s8qqaq=dirpe0xb9q8qiLsFr0=vr0=vr0dc8meaabaqaciaacaGaaeqabaWaaeGaeaaakeaaimaacqWFse=uaaa@3845@ is shown in Figure 1. The full pos-list is obtained by concatenating the pos-lists from each sequence Si.\nFigure 1 Positional Joins Algorithm. Given a longer motif ℳ MathType@MTEF@5@5@+=feaafiart1ev1aaatCvAUfKttLearuWrP9MDH5MBPbIqV92AaeXatLxBI9gBamrtHrhAL1wy0L2yHvtyaeHbnfgDOvwBHrxAJfwnaebbnrfifHhDYfgasaacH8akY=wiFfYdH8Gipec8Eeeu0xXdbba9frFj0=OqFfea0dXdd9vqai=hGuQ8kuc9pgc9s8qqaq=dirpe0xb9q8qiLsFr0=vr0=vr0dc8meaabaqaciaacaGaaeqabaWaaeGaeaaakeaaimaacqWFZestaaa@3790@, the positional joins start with the last two symbols, and proceed by successively joining the pos-list of the current symbol with the intermediate pos-list of the suffix. That is, the intermediate pos-list for a (l+1)-length pattern (with l ≥ 1) is obtained by doing a positional join of the pos-list of the pattern's first symbol, called the head symbol, with the pos-list of its l-length suffix, called the tail. As the computation progresses the previous tail pos-lists are discarded. Combined with the fact that only start positions are kept in a pos-list, this saves both time and space.\nIn order to enumerate all frequent motifs instances ℳ MathType@MTEF@5@5@+=feaafiart1ev1aaatCvAUfKttLearuWrP9MDH5MBPbIqV92AaeXatLxBI9gBamrtHrhAL1wy0L2yHvtyaeHbnfgDOvwBHrxAJfwnaebbnrfifHhDYfgasaacH8akY=wiFfYdH8Gipec8Eeeu0xXdbba9frFj0=OqFfea0dXdd9vqai=hGuQ8kuc9pgc9s8qqaq=dirpe0xb9q8qiLsFr0=vr0=vr0dc8meaabaqaciaacaGaaeqabaWaaeGaeaaakeaaimaacqWFZestaaa@3790@ in S MathType@MTEF@5@5@+=feaafiart1ev1aaatCvAUfKttLearuWrP9MDH5MBPbIqV92AaeXatLxBI9gBamrtHrhAL1wy0L2yHvtyaeHbnfgDOvwBHrxAJfwnaebbnrfifHhDYfgasaacH8akY=wiFfYdH8Gipec8Eeeu0xXdbba9frFj0=OqFfea0dXdd9vqai=hGuQ8kuc9pgc9s8qqaq=dirpe0xb9q8qiLsFr0=vr0=vr0dc8meaabaqaciaacaGaaeqabaWaaeGaeaaakeaaimaacqWFse=uaaa@3845@, EXMOTIF computes the pos-list for each ℳ MathType@MTEF@5@5@+=feaafiart1ev1aaatCvAUfKttLearuWrP9MDH5MBPbIqV92AaeXatLxBI9gBamrtHrhAL1wy0L2yHvtyaeHbnfgDOvwBHrxAJfwnaebbnrfifHhDYfgasaacH8akY=wiFfYdH8Gipec8Eeeu0xXdbba9frFj0=OqFfea0dXdd9vqai=hGuQ8kuc9pgc9s8qqaq=dirpe0xb9q8qiLsFr0=vr0=vr0dc8meaabaqaciaacaGaaeqabaWaaeGaeaaakeaaimaacqWFZestaaa@3790@ and report ℳ MathType@MTEF@5@5@+=feaafiart1ev1aaatCvAUfKttLearuWrP9MDH5MBPbIqV92AaeXatLxBI9gBamrtHrhAL1wy0L2yHvtyaeHbnfgDOvwBHrxAJfwnaebbnrfifHhDYfgasaacH8akY=wiFfYdH8Gipec8Eeeu0xXdbba9frFj0=OqFfea0dXdd9vqai=hGuQ8kuc9pgc9s8qqaq=dirpe0xb9q8qiLsFr0=vr0=vr0dc8meaabaqaciaacaGaaeqabaWaaeGaeaaakeaaimaacqWFZestaaa@3790@ only if its support is no less than the quorum (q). A straightforward approach is to directly perform positional joins on the symbols from the end to the start for each ℳ MathType@MTEF@5@5@+=feaafiart1ev1aaatCvAUfKttLearuWrP9MDH5MBPbIqV92AaeXatLxBI9gBamrtHrhAL1wy0L2yHvtyaeHbnfgDOvwBHrxAJfwnaebbnrfifHhDYfgasaacH8akY=wiFfYdH8Gipec8Eeeu0xXdbba9frFj0=OqFfea0dXdd9vqai=hGuQ8kuc9pgc9s8qqaq=dirpe0xb9q8qiLsFr0=vr0=vr0dc8meaabaqaciaacaGaaeqabaWaaeGaeaaakeaaimaacqWFZestaaa@3790@. This approach leads to much redundant computation since simple motif components may be shared among several structured motifs. EXMOTIF, in contrast, performs two steps: it first computes the pos-lists for all simple motifs in S MathType@MTEF@5@5@+=feaafiart1ev1aaatCvAUfKttLearuWrP9MDH5MBPbIqV92AaeXatLxBI9gBamrtHrhAL1wy0L2yHvtyaeHbnfgDOvwBHrxAJfwnaebbnrfifHhDYfgasaacH8akY=wiFfYdH8Gipec8Eeeu0xXdbba9frFj0=OqFfea0dXdd9vqai=hGuQ8kuc9pgc9s8qqaq=dirpe0xb9q8qiLsFr0=vr0=vr0dc8meaabaqaciaacaGaaeqabaWaaeGaeaaakeaaimaacqWFse=uaaa@3845@ by doing positional joins on pos-lists of its symbols, and it then computes the pos-list for each structured motif by doing positional joins on pos-lists of its simple motif components. EXMOTIF handles both simple and structured motifs uniformly, by adding the gap range [0, 0] between adjacent symbols within each simple motif Mi. For our example in Table 1, the structured motif template T MathType@MTEF@5@5@+=feaafiart1ev1aaatCvAUfKttLearuWrP9MDH5MBPbIqV92AaeXatLxBI9gBamrtHrhAL1wy0L2yHvtyaeHbnfgDOvwBHrxAJfwnaebbnrfifHhDYfgasaacH8akY=wiFfYdH8Gipec8Eeeu0xXdbba9frFj0=OqFfea0dXdd9vqai=hGuQ8kuc9pgc9s8qqaq=dirpe0xb9q8qiLsFr0=vr0=vr0dc8meaabaqaciaacaGaaeqabaWaaeGaeaaakeaaimaacqWFtepvaaa@3847@ becomes: N[0,0]N[0,0]N[0,1]N[0,0]N[2,3]N[0,0]N[0,0]N[0,0]N. Also since we only report frequent motifs, we can prune the candidate patterns during the positional joins based on the closure property of support (note however that this cannot be done for weighted support).\n\nExtraction of the simple motifs\nGiven a template motif T MathType@MTEF@5@5@+=feaafiart1ev1aaatCvAUfKttLearuWrP9MDH5MBPbIqV92AaeXatLxBI9gBamrtHrhAL1wy0L2yHvtyaeHbnfgDOvwBHrxAJfwnaebbnrfifHhDYfgasaacH8akY=wiFfYdH8Gipec8Eeeu0xXdbba9frFj0=OqFfea0dXdd9vqai=hGuQ8kuc9pgc9s8qqaq=dirpe0xb9q8qiLsFr0=vr0=vr0dc8meaabaqaciaacaGaaeqabaWaaeGaeaaakeaaimaacqWFtepvaaa@3847@, we know the lengths of the simple motif components desired. A naive approach is to directly do positional joins on the symbols from the end to the start of each simple motif. However, since some simple motifs are of the same length and the longer simple motifs can be obtained by doing positional joins on the shorter simple motifs/symbols, we can avoid some redundant computation. Note also that the gap range inside the simple motif is always [0,0].\nLet ℒ MathType@MTEF@5@5@+=feaafiart1ev1aaatCvAUfKttLearuWrP9MDH5MBPbIqV92AaeXatLxBI9gBaebbnrfifHhDYfgasaacH8akY=wiFfYdH8Gipec8Eeeu0xXdbba9frFj0=OqFfea0dXdd9vqai=hGuQ8kuc9pgc9s8qqaq=dirpe0xb9q8qiLsFr0=vr0=vr0dc8meaabaqaciaacaGaaeqabaqabeGadaaakeaat0uy0HwzTfgDPnwy1egaryqtHrhAL1wy0L2yHvdaiqaacqWFsectaaa@376D@ = {Li, 1 ≤ i ≤ m}, where Li is the length of each simple motif in T MathType@MTEF@5@5@+=feaafiart1ev1aaatCvAUfKttLearuWrP9MDH5MBPbIqV92AaeXatLxBI9gBamrtHrhAL1wy0L2yHvtyaeHbnfgDOvwBHrxAJfwnaebbnrfifHhDYfgasaacH8akY=wiFfYdH8Gipec8Eeeu0xXdbba9frFj0=OqFfea0dXdd9vqai=hGuQ8kuc9pgc9s8qqaq=dirpe0xb9q8qiLsFr0=vr0=vr0dc8meaabaqaciaacaGaaeqabaWaaeGaeaaakeaaimaacqWFtepvaaa@3847@ and assume ℒ MathType@MTEF@5@5@+=feaafiart1ev1aaatCvAUfKttLearuWrP9MDH5MBPbIqV92AaeXatLxBI9gBaebbnrfifHhDYfgasaacH8akY=wiFfYdH8Gipec8Eeeu0xXdbba9frFj0=OqFfea0dXdd9vqai=hGuQ8kuc9pgc9s8qqaq=dirpe0xb9q8qiLsFr0=vr0=vr0dc8meaabaqaciaacaGaaeqabaqabeGadaaakeaat0uy0HwzTfgDPnwy1egaryqtHrhAL1wy0L2yHvdaiqaacqWFsectaaa@376D@ is sorted in the ascending order. For each Li, 1 ≤ i ≤ m, we need to enumerate |ΣDNA|Li MathType@MTEF@5@5@+=feaafiart1ev1aaatCvAUfKttLearuWrP9MDH5MBPbIqV92AaeXatLxBI9gBaebbnrfifHhDYfgasaacH8akY=wiFfYdH8Gipec8Eeeu0xXdbba9frFj0=OqFfea0dXdd9vqai=hGuQ8kuc9pgc9s8qqaq=dirpe0xb9q8qiLsFr0=vr0=vr0dc8meaabaqaciaacaGaaeqabaqabeGadaaakeaadaabdaqaaiabfo6atnaaBaaaleaacqqGebarcqqGobGtcqqGbbqqaeqaaaGccaGLhWUaayjcSdWaaWbaaSqabeaacqWGmbatdaWgaaadbaGaemyAaKgabeaaaaaaaa@3799@ possible simple motifs. Let maxℒ MathType@MTEF@5@5@+=feaafiart1ev1aaatCvAUfKttLearuWrP9MDH5MBPbIqV92AaeXatLxBI9gBamrtHrhAL1wy0L2yHvtyaeHbnfgDOvwBHrxAJfwnaebbnrfifHhDYfgasaacH8akY=wiFfYdH8Gipec8Eeeu0xXdbba9frFj0=OqFfea0dXdd9vqai=hGuQ8kuc9pgc9s8qqaq=dirpe0xb9q8qiLsFr0=vr0=vr0dc8meaabaqaciaacaGaaeqabaWaaeGaeaaakeaaieGacqWFTbqBcqWFHbqycqWF4baEdaWgaaWcbaacdaGae4NeHWeabeaaaaa@3BBF@ be the maximum length in ℒ MathType@MTEF@5@5@+=feaafiart1ev1aaatCvAUfKttLearuWrP9MDH5MBPbIqV92AaeXatLxBI9gBaebbnrfifHhDYfgasaacH8akY=wiFfYdH8Gipec8Eeeu0xXdbba9frFj0=OqFfea0dXdd9vqai=hGuQ8kuc9pgc9s8qqaq=dirpe0xb9q8qiLsFr0=vr0=vr0dc8meaabaqaciaacaGaaeqabaqabeGadaaakeaat0uy0HwzTfgDPnwy1egaryqtHrhAL1wy0L2yHvdaiqaacqWFsectaaa@376D@. We can compute the pos-lists of simple motifs sequentially from length 1 to maxℒ MathType@MTEF@5@5@+=feaafiart1ev1aaatCvAUfKttLearuWrP9MDH5MBPbIqV92AaeXatLxBI9gBamrtHrhAL1wy0L2yHvtyaeHbnfgDOvwBHrxAJfwnaebbnrfifHhDYfgasaacH8akY=wiFfYdH8Gipec8Eeeu0xXdbba9frFj0=OqFfea0dXdd9vqai=hGuQ8kuc9pgc9s8qqaq=dirpe0xb9q8qiLsFr0=vr0=vr0dc8meaabaqaciaacaGaaeqabaWaaeGaeaaakeaaieGacqWFTbqBcqWFHbqycqWF4baEdaWgaaWcbaacdaGae4NeHWeabeaaaaa@3BBF@. But this may waste time in enumerating some simple motifs of lengths that are not in ℒ MathType@MTEF@5@5@+=feaafiart1ev1aaatCvAUfKttLearuWrP9MDH5MBPbIqV92AaeXatLxBI9gBaebbnrfifHhDYfgasaacH8akY=wiFfYdH8Gipec8Eeeu0xXdbba9frFj0=OqFfea0dXdd9vqai=hGuQ8kuc9pgc9s8qqaq=dirpe0xb9q8qiLsFr0=vr0=vr0dc8meaabaqaciaacaGaaeqabaqabeGadaaakeaat0uy0HwzTfgDPnwy1egaryqtHrhAL1wy0L2yHvdaiqaacqWFsectaaa@376D@. Instead, EXMOTIF first computes the pos-lists for the simple motifs of lengths that are powers of 2. Formally, let J be an integer such that 2J ≤ maxℒ MathType@MTEF@5@5@+=feaafiart1ev1aaatCvAUfKttLearuWrP9MDH5MBPbIqV92AaeXatLxBI9gBamrtHrhAL1wy0L2yHvtyaeHbnfgDOvwBHrxAJfwnaebbnrfifHhDYfgasaacH8akY=wiFfYdH8Gipec8Eeeu0xXdbba9frFj0=OqFfea0dXdd9vqai=hGuQ8kuc9pgc9s8qqaq=dirpe0xb9q8qiLsFr0=vr0=vr0dc8meaabaqaciaacaGaaeqabaWaaeGaeaaakeaaieGacqWFTbqBcqWFHbqycqWF4baEdaWgaaWcbaacdaGae4NeHWeabeaaaaa@3BBF@ \u003c 2J+1. We extract the patterns of length 2j by doing positional joins on the pos-lists of patterns of length 2j-1 for all 1 ≤ j ≤ J. For example, when maxℒ MathType@MTEF@5@5@+=feaafiart1ev1aaatCvAUfKttLearuWrP9MDH5MBPbIqV92AaeXatLxBI9gBamrtHrhAL1wy0L2yHvtyaeHbnfgDOvwBHrxAJfwnaebbnrfifHhDYfgasaacH8akY=wiFfYdH8Gipec8Eeeu0xXdbba9frFj0=OqFfea0dXdd9vqai=hGuQ8kuc9pgc9s8qqaq=dirpe0xb9q8qiLsFr0=vr0=vr0dc8meaabaqaciaacaGaaeqabaWaaeGaeaaakeaaieGacqWFTbqBcqWFHbqycqWF4baEdaWgaaWcbaacdaGae4NeHWeabeaaaaa@3BBF@ = 11, EXMOTIF first computes the pos-lists for simple motifs of length 20 = 1, 21 = 2, 22 = 4 and 23 = 8.\nEXMOTIF then computes the pos-lists for the simple motifs of Li ∈ ℒ MathType@MTEF@5@5@+=feaafiart1ev1aaatCvAUfKttLearuWrP9MDH5MBPbIqV92AaeXatLxBI9gBaebbnrfifHhDYfgasaacH8akY=wiFfYdH8Gipec8Eeeu0xXdbba9frFj0=OqFfea0dXdd9vqai=hGuQ8kuc9pgc9s8qqaq=dirpe0xb9q8qiLsFr0=vr0=vr0dc8meaabaqaciaacaGaaeqabaqabeGadaaakeaat0uy0HwzTfgDPnwy1egaryqtHrhAL1wy0L2yHvdaiqaacqWFsectaaa@376D@, by doing positional joins on simple motifs whose pos-list(s) have already been computed and their lengths sum to Li. For example, when Li = 11, EXMOTIF has to join motifs of lengths 8, 2, and 1. It first obtains all motifs of length 8 + 2 = 10, and then joins the motifs of lengths 10 and 1, to get the pos-lists of all simple motifs of length 10 + 1 = 11. The pos-lists for the simple motifs of length Li ∈ ℒ MathType@MTEF@5@5@+=feaafiart1ev1aaatCvAUfKttLearuWrP9MDH5MBPbIqV92AaeXatLxBI9gBaebbnrfifHhDYfgasaacH8akY=wiFfYdH8Gipec8Eeeu0xXdbba9frFj0=OqFfea0dXdd9vqai=hGuQ8kuc9pgc9s8qqaq=dirpe0xb9q8qiLsFr0=vr0=vr0dc8meaabaqaciaacaGaaeqabaqabeGadaaakeaat0uy0HwzTfgDPnwy1egaryqtHrhAL1wy0L2yHvdaiqaacqWFsectaaa@376D@ are kept for further use in the structured motif extraction. At the end of the first phase, EXMOTIF has computed the pos-lists for all simple motif components that can satisfy the template.\n\nExtraction of the structured motifs\nWe extract the structured motifs by doing positional joins on the pos-lists of the simple motifs from the end to the start in the structured motif ℳ MathType@MTEF@5@5@+=feaafiart1ev1aaatCvAUfKttLearuWrP9MDH5MBPbIqV92AaeXatLxBI9gBamrtHrhAL1wy0L2yHvtyaeHbnfgDOvwBHrxAJfwnaebbnrfifHhDYfgasaacH8akY=wiFfYdH8Gipec8Eeeu0xXdbba9frFj0=OqFfea0dXdd9vqai=hGuQ8kuc9pgc9s8qqaq=dirpe0xb9q8qiLsFr0=vr0=vr0dc8meaabaqaciaacaGaaeqabaWaaeGaeaaakeaaimaacqWFZestaaa@3790@. Formally, let H[l, u]T be an intermediate structured motif, with simple motif H as the head, and a suffix structured motif T as tail. Then P (H[l, u]T) can be obtained by doing positional joins on P (H) and P (T). Since P (H) keeps only the start positions, we need to compute the corresponding end positions for those occurrences of H, to check the gap constraints. Since only exact matches or substitutions are allowed for simple motifs, the end position is simply s + |H| - 1 for a start position s.\n\nFull-position recovery\nIn our positional join approach, to save time and space we retain only the motif start positions, however, in some applications, we may need to know the full position of each occurrence, i.e., the set of matching positions for each symbol in the motif. EXMOTIF records some \"indices\" during the positional joins in order to facilitate full position recovery.\nFor each suffix of a structured motif, ℳ MathType@MTEF@5@5@+=feaafiart1ev1aaatCvAUfKttLearuWrP9MDH5MBPbIqV92AaeXatLxBI9gBamrtHrhAL1wy0L2yHvtyaeHbnfgDOvwBHrxAJfwnaebbnrfifHhDYfgasaacH8akY=wiFfYdH8Gipec8Eeeu0xXdbba9frFj0=OqFfea0dXdd9vqai=hGuQ8kuc9pgc9s8qqaq=dirpe0xb9q8qiLsFr0=vr0=vr0dc8meaabaqaciaacaGaaeqabaWaaeGaeaaakeaaimaacqWFZestaaa@3790@, starting at position i with 1 ≤ i ≤ |ℳ MathType@MTEF@5@5@+=feaafiart1ev1aaatCvAUfKttLearuWrP9MDH5MBPbIqV92AaeXatLxBI9gBamrtHrhAL1wy0L2yHvtyaeHbnfgDOvwBHrxAJfwnaebbnrfifHhDYfgasaacH8akY=wiFfYdH8Gipec8Eeeu0xXdbba9frFj0=OqFfea0dXdd9vqai=hGuQ8kuc9pgc9s8qqaq=dirpe0xb9q8qiLsFr0=vr0=vr0dc8meaabaqaciaacaGaaeqabaWaaeGaeaaakeaaimaacqWFZestaaa@3790@|, we keep its pos-list, Pi, and an index list, N MathType@MTEF@5@5@+=feaafiart1ev1aaatCvAUfKttLearuWrP9MDH5MBPbIqV92AaeXatLxBI9gBamrtHrhAL1wy0L2yHvtyaeHbnfgDOvwBHrxAJfwnaebbnrfifHhDYfgasaacH8akY=wiFfYdH8Gipec8Eeeu0xXdbba9frFj0=OqFfea0dXdd9vqai=hGuQ8kuc9pgc9s8qqaq=dirpe0xb9q8qiLsFr0=vr0=vr0dc8meaabaqaciaacaGaaeqabaWaaeGaeaaakeaaimaacqWFneVtaaa@383B@i. For each entry, say Pi[j], in the pos-list Pi, the corresponding index entry N MathType@MTEF@5@5@+=feaafiart1ev1aaatCvAUfKttLearuWrP9MDH5MBPbIqV92AaeXatLxBI9gBamrtHrhAL1wy0L2yHvtyaeHbnfgDOvwBHrxAJfwnaebbnrfifHhDYfgasaacH8akY=wiFfYdH8Gipec8Eeeu0xXdbba9frFj0=OqFfea0dXdd9vqai=hGuQ8kuc9pgc9s8qqaq=dirpe0xb9q8qiLsFr0=vr0=vr0dc8meaabaqaciaacaGaaeqabaWaaeGaeaaakeaaimaacqWFneVtaaa@383B@i[j], points to the first entry, say f, in Pi+1 that satisfies the gap range with respect to Pi[j], i.e., Pi+1[f] - Pi[j] - 1 ∈ [li, ui]. Note that N|ℳ| MathType@MTEF@5@5@+=feaafiart1ev1aaatCvAUfKttLearuWrP9MDH5MBPbIqV92AaeXatLxBI9gBamrtHrhAL1wy0L2yHvtyaeHbnfgDOvwBHrxAJfwnaebbnrfifHhDYfgasaacH8akY=wiFfYdH8Gipec8Eeeu0xXdbba9frFj0=OqFfea0dXdd9vqai=hGuQ8kuc9pgc9s8qqaq=dirpe0xb9q8qiLsFr0=vr0=vr0dc8meaabaqaciaacaGaaeqabaWaaeGaeaaakeaaimaacqWFneVtdaWgaaWcbaWaaqWaaeaacqWFZestaiaawEa7caGLiWoaaeqaaaaa@3CAF@ is never used. Also note that P (ℳ MathType@MTEF@5@5@+=feaafiart1ev1aaatCvAUfKttLearuWrP9MDH5MBPbIqV92AaeXatLxBI9gBamrtHrhAL1wy0L2yHvtyaeHbnfgDOvwBHrxAJfwnaebbnrfifHhDYfgasaacH8akY=wiFfYdH8Gipec8Eeeu0xXdbba9frFj0=OqFfea0dXdd9vqai=hGuQ8kuc9pgc9s8qqaq=dirpe0xb9q8qiLsFr0=vr0=vr0dc8meaabaqaciaacaGaaeqabaWaaeGaeaaakeaaimaacqWFZestaaa@3790@) = P1. Let s be a start position for the structured motif in sequence S, and let s be the js-th entry in P1, i.e., s = P1[js]. Let F store a full position starting from s, and let ℱ MathType@MTEF@5@5@+=feaafiart1ev1aaatCvAUfKttLearuWrP9MDH5MBPbIqV92AaeXatLxBI9gBamrtHrhAL1wy0L2yHvtyaeHbnfgDOvwBHrxAJfwnaebbnrfifHhDYfgasaacH8akY=wiFfYdH8Gipec8Eeeu0xXdbba9frFj0=OqFfea0dXdd9vqai=hGuQ8kuc9pgc9s8qqaq=dirpe0xb9q8qiLsFr0=vr0=vr0dc8meaabaqaciaacaGaaeqabaWaaeGaeaaakeaaimaacqWFXeIraaa@3787@ store the set of all full positions. Figure 2 shows the pseudo-code for recovering full positions starting from s. This recursive algorithm has four parameters: i denotes a (suffix) position in ℳ MathType@MTEF@5@5@+=feaafiart1ev1aaatCvAUfKttLearuWrP9MDH5MBPbIqV92AaeXatLxBI9gBamrtHrhAL1wy0L2yHvtyaeHbnfgDOvwBHrxAJfwnaebbnrfifHhDYfgasaacH8akY=wiFfYdH8Gipec8Eeeu0xXdbba9frFj0=OqFfea0dXdd9vqai=hGuQ8kuc9pgc9s8qqaq=dirpe0xb9q8qiLsFr0=vr0=vr0dc8meaabaqaciaacaGaaeqabaWaaeGaeaaakeaaimaacqWFZestaaa@3790@, j gives the j-th entry in Pi, F denotes an intermediate full position, and ℱ MathType@MTEF@5@5@+=feaafiart1ev1aaatCvAUfKttLearuWrP9MDH5MBPbIqV92AaeXatLxBI9gBamrtHrhAL1wy0L2yHvtyaeHbnfgDOvwBHrxAJfwnaebbnrfifHhDYfgasaacH8akY=wiFfYdH8Gipec8Eeeu0xXdbba9frFj0=OqFfea0dXdd9vqai=hGuQ8kuc9pgc9s8qqaq=dirpe0xb9q8qiLsFr0=vr0=vr0dc8meaabaqaciaacaGaaeqabaWaaeGaeaaakeaaimaacqWFXeIraaa@3787@ denotes the set of all the full occurrences. The algorithm is initially called with i = 2, j = N MathType@MTEF@5@5@+=feaafiart1ev1aaatCvAUfKttLearuWrP9MDH5MBPbIqV92AaeXatLxBI9gBamrtHrhAL1wy0L2yHvtyaeHbnfgDOvwBHrxAJfwnaebbnrfifHhDYfgasaacH8akY=wiFfYdH8Gipec8Eeeu0xXdbba9frFj0=OqFfea0dXdd9vqai=hGuQ8kuc9pgc9s8qqaq=dirpe0xb9q8qiLsFr0=vr0=vr0dc8meaabaqaciaacaGaaeqabaWaaeGaeaaakeaaimaacqWFneVtaaa@383B@1[js], F = {s}, and ℱ MathType@MTEF@5@5@+=feaafiart1ev1aaatCvAUfKttLearuWrP9MDH5MBPbIqV92AaeXatLxBI9gBamrtHrhAL1wy0L2yHvtyaeHbnfgDOvwBHrxAJfwnaebbnrfifHhDYfgasaacH8akY=wiFfYdH8Gipec8Eeeu0xXdbba9frFj0=OqFfea0dXdd9vqai=hGuQ8kuc9pgc9s8qqaq=dirpe0xb9q8qiLsFr0=vr0=vr0dc8meaabaqaciaacaGaaeqabaWaaeGaeaaakeaaimaacqWFXeIraaa@3787@ = ∅. Starting at the first index in Pi, that satisfies the gap range with respect to the last position in F, we continue to compute all such positions j' ∈ [j, |Pi|] that satisfy the gap range (line 3). That is, we find all positions j', such that Pi[j'] - F[i - 1] - 1 = d ∈ [li, ui]. For each such position j', we add it in turn to the intermediate full position, and make another recursive call (line 5), passing the first index position Ni[j'] in Pi+1 that can satisfy the gap range with respect to Pi[j']. Thus in each call we keep following the indices from one pos-list to the next, to finally obtain a full position starting from s when we reach the last pos-list, P|ℳ|. Note that at each suffix position i, since j only marks the first position in Pi+1 that satisfies the gap constraints, we also need to consider all the subsequent positions j' \u003e j that may satisfy the corresponding gap range.\nFigure 2 Indexed Full Position Recovery Algorithm. Consider the example shown in Fig. 3 to recover the full positions for ℳ MathType@MTEF@5@5@+=feaafiart1ev1aaatCvAUfKttLearuWrP9MDH5MBPbIqV92AaeXatLxBI9gBamrtHrhAL1wy0L2yHvtyaeHbnfgDOvwBHrxAJfwnaebbnrfifHhDYfgasaacH8akY=wiFfYdH8Gipec8Eeeu0xXdbba9frFj0=OqFfea0dXdd9vqai=hGuQ8kuc9pgc9s8qqaq=dirpe0xb9q8qiLsFr0=vr0=vr0dc8meaabaqaciaacaGaaeqabaWaaeGaeaaakeaaimaacqWFZestaaa@3790@ = CCG[0,3]TA[1,3]GAAC. Under each symbol we show two columns. The left column corresponds to the intermediate pos-lists as we proceed from right to left, whereas the right column stores the indices into the previous pos-list. For example, the middle column gives the pos-list P (TA[1,3]GAAC) = {1, 1, 4, 2, 2, 5, 7, 3, 1, 1}. For each position x ∈ P (TA[l,3]GAAC) (excluding the sequence identifiers and the cardinality), the right column records an index in P (GAAC) which corresponds to the first position in P (GAAC) that satisfies the gap range with respect to x. For example, for position x = 5 (at index 6), the first position in P (GAAC) that satisfies the gap range [1,3] is 10 (since in this case there are 3 gaps between the end of TA at position 6 and start of GAAC at position 10), and it occurs at index 6. Likewise, for each position in the current pos-list we store which positions in the previous pos-list were extended. With this indexed information, full-position recovery becomes straightforward. We begin with the start positions of the occurrences. We then keep following the indices from one pos-list to the next, until we reach the last pos-list. Since the index only marks the first position that satisfies the gap range, we still need to check if the following positions satisfy the gap range. At each stage in the full position recovery, we maintain a list of intermediate position prefixes ℱ MathType@MTEF@5@5@+=feaafiart1ev1aaatCvAUfKttLearuWrP9MDH5MBPbIqV92AaeXatLxBI9gBamrtHrhAL1wy0L2yHvtyaeHbnfgDOvwBHrxAJfwnaebbnrfifHhDYfgasaacH8akY=wiFfYdH8Gipec8Eeeu0xXdbba9frFj0=OqFfea0dXdd9vqai=hGuQ8kuc9pgc9s8qqaq=dirpe0xb9q8qiLsFr0=vr0=vr0dc8meaabaqaciaacaGaaeqabaWaaeGaeaaakeaaimaacqWFXeIraaa@3787@ that match up to the j-th position in ℳ MathType@MTEF@5@5@+=feaafiart1ev1aaatCvAUfKttLearuWrP9MDH5MBPbIqV92AaeXatLxBI9gBamrtHrhAL1wy0L2yHvtyaeHbnfgDOvwBHrxAJfwnaebbnrfifHhDYfgasaacH8akY=wiFfYdH8Gipec8Eeeu0xXdbba9frFj0=OqFfea0dXdd9vqai=hGuQ8kuc9pgc9s8qqaq=dirpe0xb9q8qiLsFr0=vr0=vr0dc8meaabaqaciaacaGaaeqabaWaaeGaeaaakeaaimaacqWFZestaaa@3790@. For example, to recover the full position for ℳ MathType@MTEF@5@5@+=feaafiart1ev1aaatCvAUfKttLearuWrP9MDH5MBPbIqV92AaeXatLxBI9gBamrtHrhAL1wy0L2yHvtyaeHbnfgDOvwBHrxAJfwnaebbnrfifHhDYfgasaacH8akY=wiFfYdH8Gipec8Eeeu0xXdbba9frFj0=OqFfea0dXdd9vqai=hGuQ8kuc9pgc9s8qqaq=dirpe0xb9q8qiLsFr0=vr0=vr0dc8meaabaqaciaacaGaaeqabaWaaeGaeaaakeaaimaacqWFZestaaa@3790@ = CCG[0,3]TA[1,3]GAAC, considering start position 1 (with ℱ MathType@MTEF@5@5@+=feaafiart1ev1aaatCvAUfKttLearuWrP9MDH5MBPbIqV92AaeXatLxBI9gBamrtHrhAL1wy0L2yHvtyaeHbnfgDOvwBHrxAJfwnaebbnrfifHhDYfgasaacH8akY=wiFfYdH8Gipec8Eeeu0xXdbba9frFj0=OqFfea0dXdd9vqai=hGuQ8kuc9pgc9s8qqaq=dirpe0xb9q8qiLsFr0=vr0=vr0dc8meaabaqaciaacaGaaeqabaWaaeGaeaaakeaaimaacqWFXeIraaa@3787@ = {(1)}) in sequence 2, we follow index 6 to get position 5 in the middle pos-list, to get ℱ MathType@MTEF@5@5@+=feaafiart1ev1aaatCvAUfKttLearuWrP9MDH5MBPbIqV92AaeXatLxBI9gBamrtHrhAL1wy0L2yHvtyaeHbnfgDOvwBHrxAJfwnaebbnrfifHhDYfgasaacH8akY=wiFfYdH8Gipec8Eeeu0xXdbba9frFj0=OqFfea0dXdd9vqai=hGuQ8kuc9pgc9s8qqaq=dirpe0xb9q8qiLsFr0=vr0=vr0dc8meaabaqaciaacaGaaeqabaWaaeGaeaaakeaaimaacqWFXeIraaa@3787@ = {(1, 5)}. Since the next position after 5 is 7 which is also within the gap range [0,3], so we update ℱ MathType@MTEF@5@5@+=feaafiart1ev1aaatCvAUfKttLearuWrP9MDH5MBPbIqV92AaeXatLxBI9gBamrtHrhAL1wy0L2yHvtyaeHbnfgDOvwBHrxAJfwnaebbnrfifHhDYfgasaacH8akY=wiFfYdH8Gipec8Eeeu0xXdbba9frFj0=OqFfea0dXdd9vqai=hGuQ8kuc9pgc9s8qqaq=dirpe0xb9q8qiLsFr0=vr0=vr0dc8meaabaqaciaacaGaaeqabaWaaeGaeaaakeaaimaacqWFXeIraaa@3787@ = {(1, 5), (1, 7)}. For position 5, we follow index 6 to get position 10 in the rightmost pos-list, to get ℱ MathType@MTEF@5@5@+=feaafiart1ev1aaatCvAUfKttLearuWrP9MDH5MBPbIqV92AaeXatLxBI9gBamrtHrhAL1wy0L2yHvtyaeHbnfgDOvwBHrxAJfwnaebbnrfifHhDYfgasaacH8akY=wiFfYdH8Gipec8Eeeu0xXdbba9frFj0=OqFfea0dXdd9vqai=hGuQ8kuc9pgc9s8qqaq=dirpe0xb9q8qiLsFr0=vr0=vr0dc8meaabaqaciaacaGaaeqabaWaaeGaeaaakeaaimaacqWFXeIraaa@3787@ = {(1, 5, 10)}; for position 7, we follow index 6 to get position 10 in the right pos-list, to get ℱ MathType@MTEF@5@5@+=feaafiart1ev1aaatCvAUfKttLearuWrP9MDH5MBPbIqV92AaeXatLxBI9gBamrtHrhAL1wy0L2yHvtyaeHbnfgDOvwBHrxAJfwnaebbnrfifHhDYfgasaacH8akY=wiFfYdH8Gipec8Eeeu0xXdbba9frFj0=OqFfea0dXdd9vqai=hGuQ8kuc9pgc9s8qqaq=dirpe0xb9q8qiLsFr0=vr0=vr0dc8meaabaqaciaacaGaaeqabaWaaeGaeaaakeaaimaacqWFXeIraaa@3787@ = {(1, 7, 10)}. Likewise, we can recover the full-position in sequence 1, which is ℱ MathType@MTEF@5@5@+=feaafiart1ev1aaatCvAUfKttLearuWrP9MDH5MBPbIqV92AaeXatLxBI9gBamrtHrhAL1wy0L2yHvtyaeHbnfgDOvwBHrxAJfwnaebbnrfifHhDYfgasaacH8akY=wiFfYdH8Gipec8Eeeu0xXdbba9frFj0=OqFfea0dXdd9vqai=hGuQ8kuc9pgc9s8qqaq=dirpe0xb9q8qiLsFr0=vr0=vr0dc8meaabaqaciaacaGaaeqabaWaaeGaeaaakeaaimaacqWFXeIraaa@3787@ = {(1, 4, 8)}. During the full-position recovery, we can also count the number of full-positions, i.e., occurrences, of each structured motif. For example, there are 3 occurrences of CCG[0,3]TA[1,3]GAAC.\nFigure 3 Indexed Full-position Recovery Example.\n\nLength ranges for simple motifs\nEXMOTIF also allows variation in the lengths of the simple motifs to be found. For example, a motif template may be specified as M1[5,10] M2, |M1| ∈ [2,4], and |M2| ∈ [6,7], which means that we have to consider NN, NNN, and NNNN as the possible templates for M1 and similarly for M2. A straightforward way for handling length ranges is to enumerate exhaustively all the possible sub-templates of T MathType@MTEF@5@5@+=feaafiart1ev1aaatCvAUfKttLearuWrP9MDH5MBPbIqV92AaeXatLxBI9gBamrtHrhAL1wy0L2yHvtyaeHbnfgDOvwBHrxAJfwnaebbnrfifHhDYfgasaacH8akY=wiFfYdH8Gipec8Eeeu0xXdbba9frFj0=OqFfea0dXdd9vqai=hGuQ8kuc9pgc9s8qqaq=dirpe0xb9q8qiLsFr0=vr0=vr0dc8meaabaqaciaacaGaaeqabaWaaeGaeaaakeaaimaacqWFtepvaaa@3847@ with simple motifs of fixed lengths and then to extract each sub-template separately. Instead, EXMOTIF does an optimized extraction. EXMOTIF reuses the partial pos-lists created when using a depth first search to enumerate and extract the sub-templates.\n\nHandling substitutions\nAs mutations are a common phenomena in biological sequences, we allow substitutions in the extracted motifs. That is two motif instances may be considered to be the same if they are within the allowed substitution thresholds. EXMOTIF allows users to specify the number of substitutions allowed for the whole motif (ε), and also a per simple motif threshold (εi, i ∈ [1, k]). There are two types of substitutions we consider.\n\nPosition-specific substitutions\nHere we allow a position (a DNA symbol) in the instance motif ℳ MathType@MTEF@5@5@+=feaafiart1ev1aaatCvAUfKttLearuWrP9MDH5MBPbIqV92AaeXatLxBI9gBamrtHrhAL1wy0L2yHvtyaeHbnfgDOvwBHrxAJfwnaebbnrfifHhDYfgasaacH8akY=wiFfYdH8Gipec8Eeeu0xXdbba9frFj0=OqFfea0dXdd9vqai=hGuQ8kuc9pgc9s8qqaq=dirpe0xb9q8qiLsFr0=vr0=vr0dc8meaabaqaciaacaGaaeqabaWaaeGaeaaakeaaimaacqWFZestaaa@3790@ to be substituted with 1 or 2 other DNA symbols. All such neighbors will contribute to the frequency of ℳ MathType@MTEF@5@5@+=feaafiart1ev1aaatCvAUfKttLearuWrP9MDH5MBPbIqV92AaeXatLxBI9gBamrtHrhAL1wy0L2yHvtyaeHbnfgDOvwBHrxAJfwnaebbnrfifHhDYfgasaacH8akY=wiFfYdH8Gipec8Eeeu0xXdbba9frFj0=OqFfea0dXdd9vqai=hGuQ8kuc9pgc9s8qqaq=dirpe0xb9q8qiLsFr0=vr0=vr0dc8meaabaqaciaacaGaaeqabaWaaeGaeaaakeaaimaacqWFZestaaa@3790@. For example, for ℳ MathType@MTEF@5@5@+=feaafiart1ev1aaatCvAUfKttLearuWrP9MDH5MBPbIqV92AaeXatLxBI9gBamrtHrhAL1wy0L2yHvtyaeHbnfgDOvwBHrxAJfwnaebbnrfifHhDYfgasaacH8akY=wiFfYdH8Gipec8Eeeu0xXdbba9frFj0=OqFfea0dXdd9vqai=hGuQ8kuc9pgc9s8qqaq=dirpe0xb9q8qiLsFr0=vr0=vr0dc8meaabaqaciaacaGaaeqabaWaaeGaeaaakeaaimaacqWFZestaaa@3790@ = ACG[4,6]TT, if we allow e1 = 1 substitutions in motif M1 = ACG, at position 2, then AAG[4,6]TT, ACG[4,6]TT or AGG[4,6]TT may contribute to the frequency of ℳ MathType@MTEF@5@5@+=feaafiart1ev1aaatCvAUfKttLearuWrP9MDH5MBPbIqV92AaeXatLxBI9gBamrtHrhAL1wy0L2yHvtyaeHbnfgDOvwBHrxAJfwnaebbnrfifHhDYfgasaacH8akY=wiFfYdH8Gipec8Eeeu0xXdbba9frFj0=OqFfea0dXdd9vqai=hGuQ8kuc9pgc9s8qqaq=dirpe0xb9q8qiLsFr0=vr0=vr0dc8meaabaqaciaacaGaaeqabaWaaeGaeaaakeaaimaacqWFZestaaa@3790@. Instead of enumerating all of these separately, EXMOTIF can directly mine relevant motifs using IUPAC symbols (see Table 3). EXMOTIF simply constructs the pos-lists for the relevant IUPAC symbols by scanning sequences in S MathType@MTEF@5@5@+=feaafiart1ev1aaatCvAUfKttLearuWrP9MDH5MBPbIqV92AaeXatLxBI9gBamrtHrhAL1wy0L2yHvtyaeHbnfgDOvwBHrxAJfwnaebbnrfifHhDYfgasaacH8akY=wiFfYdH8Gipec8Eeeu0xXdbba9frFj0=OqFfea0dXdd9vqai=hGuQ8kuc9pgc9s8qqaq=dirpe0xb9q8qiLsFr0=vr0=vr0dc8meaabaqaciaacaGaaeqabaWaaeGaeaaakeaaimaacqWFse=uaaa@3845@ once. Then it mines the motif instances as in the basic approach, since all allowed substitutions have already been incorporated into the relevant IUPAC symbols. Let vi, 1 ≤ i ≤ k, to denote the set of IUPAC symbols that can appear in the motif. When vi = 1 (i.e., each position allows only 1 DNA symbol), the alphabet used is {A, C, G, T}; when vi = 2 (i.e., each position may allow up to 2 DNA symbols), the expanded alphabet is {A, C, G, T, R, Y, K, M, S, W}; and when vi = 3 (i.e., each position may allow up to 3 DNA symbols), the expanded alphabet is {A, C, G, T, R, Y, K, M, S, W, B, D, H, V}. For example, when v1 = 2, instead of reporting ℳ MathType@MTEF@5@5@+=feaafiart1ev1aaatCvAUfKttLearuWrP9MDH5MBPbIqV92AaeXatLxBI9gBamrtHrhAL1wy0L2yHvtyaeHbnfgDOvwBHrxAJfwnaebbnrfifHhDYfgasaacH8akY=wiFfYdH8Gipec8Eeeu0xXdbba9frFj0=OqFfea0dXdd9vqai=hGuQ8kuc9pgc9s8qqaq=dirpe0xb9q8qiLsFr0=vr0=vr0dc8meaabaqaciaacaGaaeqabaWaaeGaeaaakeaaimaacqWFZestaaa@3790@ = ACG[4,6]TT as the mined instance, EXMOTIF may report ASG[4,6]TT as an instance, where S stands for either C or G (see Table 3). EXMOTIF also allows the user to specify the maximum number of IUPAC symbols that can appear in each simple motif, ei, 1 ≤ i ≤ k.\nTable 3 IUPAC alphabet (ΣIUPAC).\nSymbol A C G T\nBases A C G T\nSymbol U R Y K\nBases U A,G C,T G,T\nSymbol M S W B\nBases A,C G,C A,T C,G,T\nSymbol D H V N\nBases A,G,T A,C,T A,C,G A,C,G,T\n\nArbitrary substitutions\nHere we allow a DNA symbol in ℳ MathType@MTEF@5@5@+=feaafiart1ev1aaatCvAUfKttLearuWrP9MDH5MBPbIqV92AaeXatLxBI9gBamrtHrhAL1wy0L2yHvtyaeHbnfgDOvwBHrxAJfwnaebbnrfifHhDYfgasaacH8akY=wiFfYdH8Gipec8Eeeu0xXdbba9frFj0=OqFfea0dXdd9vqai=hGuQ8kuc9pgc9s8qqaq=dirpe0xb9q8qiLsFr0=vr0=vr0dc8meaabaqaciaacaGaaeqabaWaaeGaeaaakeaaimaacqWFZestaaa@3790@ to be substituted with other symbols across all positions (i.e., in a position independent manner), up to the allowed maximum errors per motif (or per component). To count the support for a motif, EXMOTIF has to consider all of its neighbors as well, which are defined as all the motifs (including itself) within Hamming distance, ε (or per motif ei). Then the support of an instance motif is calculated as the total number of sequences in which its neighbors (including itself) are present. As always, the motif is frequent if its support meets the quorum q, that is, its neighbors are present in at least q distinct sequences.\nThe main challenge is that when arbitrary, position independent substitutions are allowed, we cannot do support checking during each positional join, since the support of the current motif may be below quorum, but combined with its neighbors it may meet quorum. Thus EXMOTIF does support checking at two points. First, it checks for quorum after the pos-lists of all the simple motifs in T MathType@MTEF@5@5@+=feaafiart1ev1aaatCvAUfKttLearuWrP9MDH5MBPbIqV92AaeXatLxBI9gBamrtHrhAL1wy0L2yHvtyaeHbnfgDOvwBHrxAJfwnaebbnrfifHhDYfgasaacH8akY=wiFfYdH8Gipec8Eeeu0xXdbba9frFj0=OqFfea0dXdd9vqai=hGuQ8kuc9pgc9s8qqaq=dirpe0xb9q8qiLsFr0=vr0=vr0dc8meaabaqaciaacaGaaeqabaWaaeGaeaaakeaaimaacqWFtepvaaa@3847@ have been computed, provided the per motif error thresholds ei have been specified. In this case each simple motif must be frequent to be extended to a structured motif. Second, it checks for quorum after the pos-lists of all the structured motifs that satisfy T MathType@MTEF@5@5@+=feaafiart1ev1aaatCvAUfKttLearuWrP9MDH5MBPbIqV92AaeXatLxBI9gBamrtHrhAL1wy0L2yHvtyaeHbnfgDOvwBHrxAJfwnaebbnrfifHhDYfgasaacH8akY=wiFfYdH8Gipec8Eeeu0xXdbba9frFj0=OqFfea0dXdd9vqai=hGuQ8kuc9pgc9s8qqaq=dirpe0xb9q8qiLsFr0=vr0=vr0dc8meaabaqaciaacaGaaeqabaWaaeGaeaaakeaaimaacqWFtepvaaa@3847@ are computed.\n\nDetermining neighbors\nIn order to quickly find all the existing neighbors of a motif within the allowed error thresholds, EXMOTIF first computes all the exact structured motifs, and stores them into a hash table to facilitate fast lookup. Then for each extracted structured motif ℳ MathType@MTEF@5@5@+=feaafiart1ev1aaatCvAUfKttLearuWrP9MDH5MBPbIqV92AaeXatLxBI9gBamrtHrhAL1wy0L2yHvtyaeHbnfgDOvwBHrxAJfwnaebbnrfifHhDYfgasaacH8akY=wiFfYdH8Gipec8Eeeu0xXdbba9frFj0=OqFfea0dXdd9vqai=hGuQ8kuc9pgc9s8qqaq=dirpe0xb9q8qiLsFr0=vr0=vr0dc8meaabaqaciaacaGaaeqabaWaaeGaeaaakeaaimaacqWFZestaaa@3790@, EXMOTIF enumerates all its possible neighbors and checks whether they exist in the hash table. One problem is that the number of possible neighbors of ℳ MathType@MTEF@5@5@+=feaafiart1ev1aaatCvAUfKttLearuWrP9MDH5MBPbIqV92AaeXatLxBI9gBamrtHrhAL1wy0L2yHvtyaeHbnfgDOvwBHrxAJfwnaebbnrfifHhDYfgasaacH8akY=wiFfYdH8Gipec8Eeeu0xXdbba9frFj0=OqFfea0dXdd9vqai=hGuQ8kuc9pgc9s8qqaq=dirpe0xb9q8qiLsFr0=vr0=vr0dc8meaabaqaciaacaGaaeqabaWaaeGaeaaakeaaimaacqWFZestaaa@3790@ can be quite large. When we allow εi substitutions for simple component Mi in ℳ MathType@MTEF@5@5@+=feaafiart1ev1aaatCvAUfKttLearuWrP9MDH5MBPbIqV92AaeXatLxBI9gBamrtHrhAL1wy0L2yHvtyaeHbnfgDOvwBHrxAJfwnaebbnrfifHhDYfgasaacH8akY=wiFfYdH8Gipec8Eeeu0xXdbba9frFj0=OqFfea0dXdd9vqai=hGuQ8kuc9pgc9s8qqaq=dirpe0xb9q8qiLsFr0=vr0=vr0dc8meaabaqaciaacaGaaeqabaWaaeGaeaaakeaaimaacqWFZestaaa@3790@, for 1 ≤ i ≤ k, the number of ℳ MathType@MTEF@5@5@+=feaafiart1ev1aaatCvAUfKttLearuWrP9MDH5MBPbIqV92AaeXatLxBI9gBamrtHrhAL1wy0L2yHvtyaeHbnfgDOvwBHrxAJfwnaebbnrfifHhDYfgasaacH8akY=wiFfYdH8Gipec8Eeeu0xXdbba9frFj0=OqFfea0dXdd9vqai=hGuQ8kuc9pgc9s8qqaq=dirpe0xb9q8qiLsFr0=vr0=vr0dc8meaabaqaciaacaGaaeqabaWaaeGaeaaakeaaimaacqWFZestaaa@3790@'s neighbors is given as ∏i=1k[∑j=0ei(|Mi|j)⋅3j] MathType@MTEF@5@5@+=feaafiart1ev1aaatCvAUfKttLearuWrP9MDH5MBPbIqV92AaeXatLxBI9gBaebbnrfifHhDYfgasaacH8akY=wiFfYdH8Gipec8Eeeu0xXdbba9frFj0=OqFfea0dXdd9vqai=hGuQ8kuc9pgc9s8qqaq=dirpe0xb9q8qiLsFr0=vr0=vr0dc8meaabaqaciaacaGaaeqabaqabeGadaaakeaadaqeWaqaaiabcUfaBnaaqadabaWaaeWaaeaafaqabeGabaaabaWaaqWaaeaacqWGnbqtdaWgaaWcbaGaemyAaKgabeaaaOGaay5bSlaawIa7aaqaaiabdQgaQbaaaiaawIcacaGLPaaacqGHflY1cqaIZaWmdaahaaWcbeqaaiabdQgaQbaaaeaacqWGQbGAcqGH9aqpcqaIWaamaeaacqWGLbqzdaWgaaadbaGaemyAaKgabeaaa0GaeyyeIuoakiabc2faDbWcbaGaemyAaKMaeyypa0JaeGymaedabaGaem4AaSganiabg+Givdaaaa@4B8B@. For example, for ℳ MathType@MTEF@5@5@+=feaafiart1ev1aaatCvAUfKttLearuWrP9MDH5MBPbIqV92AaeXatLxBI9gBamrtHrhAL1wy0L2yHvtyaeHbnfgDOvwBHrxAJfwnaebbnrfifHhDYfgasaacH8akY=wiFfYdH8Gipec8Eeeu0xXdbba9frFj0=OqFfea0dXdd9vqai=hGuQ8kuc9pgc9s8qqaq=dirpe0xb9q8qiLsFr0=vr0=vr0dc8meaabaqaciaacaGaaeqabaWaaeGaeaaakeaaimaacqWFZestaaa@3790@ = AACGTT[1,5]AGTTCC, when we allow one substitution for each simple motif, the number of its neighbors is 361; when we allow two substitutions per component, the number of its neighbors is 23,716. Instead of enumerating the potentially large number of neighbors (many of which may not even occur in the sequence set S MathType@MTEF@5@5@+=feaafiart1ev1aaatCvAUfKttLearuWrP9MDH5MBPbIqV92AaeXatLxBI9gBamrtHrhAL1wy0L2yHvtyaeHbnfgDOvwBHrxAJfwnaebbnrfifHhDYfgasaacH8akY=wiFfYdH8Gipec8Eeeu0xXdbba9frFj0=OqFfea0dXdd9vqai=hGuQ8kuc9pgc9s8qqaq=dirpe0xb9q8qiLsFr0=vr0=vr0dc8meaabaqaciaacaGaaeqabaWaaeGaeaaakeaaimaacqWFse=uaaa@3845@) for each structured motif ℳ MathType@MTEF@5@5@+=feaafiart1ev1aaatCvAUfKttLearuWrP9MDH5MBPbIqV92AaeXatLxBI9gBamrtHrhAL1wy0L2yHvtyaeHbnfgDOvwBHrxAJfwnaebbnrfifHhDYfgasaacH8akY=wiFfYdH8Gipec8Eeeu0xXdbba9frFj0=OqFfea0dXdd9vqai=hGuQ8kuc9pgc9s8qqaq=dirpe0xb9q8qiLsFr0=vr0=vr0dc8meaabaqaciaacaGaaeqabaWaaeGaeaaakeaaimaacqWFZestaaa@3790@ individually, EXMOTIF utilizes the observation that many motifs have shared neighbors, and thus previously computed support information can be reused. EXMOTIF enumerates neighbors in two steps. In the first step, for each ℳ MathType@MTEF@5@5@+=feaafiart1ev1aaatCvAUfKttLearuWrP9MDH5MBPbIqV92AaeXatLxBI9gBamrtHrhAL1wy0L2yHvtyaeHbnfgDOvwBHrxAJfwnaebbnrfifHhDYfgasaacH8akY=wiFfYdH8Gipec8Eeeu0xXdbba9frFj0=OqFfea0dXdd9vqai=hGuQ8kuc9pgc9s8qqaq=dirpe0xb9q8qiLsFr0=vr0=vr0dc8meaabaqaciaacaGaaeqabaWaaeGaeaaakeaaimaacqWFZestaaa@3790@, it enumerates aggregate neighbor motifs, replacing the allowed number of errors ei with as many 'N' symbols (which stands for A,C,G, or T). The number of possible aggregate neighbors is given as ∏i=1k(|Mi|εi) MathType@MTEF@5@5@+=feaafiart1ev1aaatCvAUfKttLearuWrP9MDH5MBPbIqV92AaeXatLxBI9gBaebbnrfifHhDYfgasaacH8akY=wiFfYdH8Gipec8Eeeu0xXdbba9frFj0=OqFfea0dXdd9vqai=hGuQ8kuc9pgc9s8qqaq=dirpe0xb9q8qiLsFr0=vr0=vr0dc8meaabaqaciaacaGaaeqabaqabeGadaaakeaadaqeWaqaamaabmaabaqbaeqabiqaaaqaamaaemaabaGaemyta00aaSbaaSqaaiabdMgaPbqabaaakiaawEa7caGLiWoaaeaaiiGacqWF1oqzdaWgaaWcbaGaemyAaKgabeaaaaaakiaawIcacaGLPaaaaSqaaiabdMgaPjabg2da9iabigdaXaqaaiabdUgaRbqdcqGHpis1aaaa@3DF8@. The second step, it computes the support for each aggregate neighbor by expanding each 'N' with each DNA symbol, looking up the hash table for the support of the corresponding motif, and adding the supports for all matching motifs. Since the motifs matching an aggregate are also neighbors of each other, the support of the aggregate can be re-used to compute the support of other matching motifs as well. Once the supports for all aggregate neighbors have been computed, the final support of the structured motif ℳ MathType@MTEF@5@5@+=feaafiart1ev1aaatCvAUfKttLearuWrP9MDH5MBPbIqV92AaeXatLxBI9gBamrtHrhAL1wy0L2yHvtyaeHbnfgDOvwBHrxAJfwnaebbnrfifHhDYfgasaacH8akY=wiFfYdH8Gipec8Eeeu0xXdbba9frFj0=OqFfea0dXdd9vqai=hGuQ8kuc9pgc9s8qqaq=dirpe0xb9q8qiLsFr0=vr0=vr0dc8meaabaqaciaacaGaaeqabaWaaeGaeaaakeaaimaacqWFZestaaa@3790@ can be obtained. Thus for each ℳ MathType@MTEF@5@5@+=feaafiart1ev1aaatCvAUfKttLearuWrP9MDH5MBPbIqV92AaeXatLxBI9gBamrtHrhAL1wy0L2yHvtyaeHbnfgDOvwBHrxAJfwnaebbnrfifHhDYfgasaacH8akY=wiFfYdH8Gipec8Eeeu0xXdbba9frFj0=OqFfea0dXdd9vqai=hGuQ8kuc9pgc9s8qqaq=dirpe0xb9q8qiLsFr0=vr0=vr0dc8meaabaqaciaacaGaaeqabaWaaeGaeaaakeaaimaacqWFZestaaa@3790@, the number of \"neighbors\" to consider can be as low as ∏i=1k(|Mi|εi) MathType@MTEF@5@5@+=feaafiart1ev1aaatCvAUfKttLearuWrP9MDH5MBPbIqV92AaeXatLxBI9gBaebbnrfifHhDYfgasaacH8akY=wiFfYdH8Gipec8Eeeu0xXdbba9frFj0=OqFfea0dXdd9vqai=hGuQ8kuc9pgc9s8qqaq=dirpe0xb9q8qiLsFr0=vr0=vr0dc8meaabaqaciaacaGaaeqabaqabeGadaaakeaadaqeWaqaamaabmaabaqbaeqabiqaaaqaamaaemaabaGaemyta00aaSbaaSqaaiabdMgaPbqabaaakiaawEa7caGLiWoaaeaaiiGacqWF1oqzdaWgaaWcbaGaemyAaKgabeaaaaaakiaawIcacaGLPaaaaSqaaiabdMgaPjabg2da9iabigdaXaqaaiabdUgaRbqdcqGHpis1aaaa@3DF8@!\nFor example, consider the example shown in Figure 4. Consider the structured motif ℳ MathType@MTEF@5@5@+=feaafiart1ev1aaatCvAUfKttLearuWrP9MDH5MBPbIqV92AaeXatLxBI9gBamrtHrhAL1wy0L2yHvtyaeHbnfgDOvwBHrxAJfwnaebbnrfifHhDYfgasaacH8akY=wiFfYdH8Gipec8Eeeu0xXdbba9frFj0=OqFfea0dXdd9vqai=hGuQ8kuc9pgc9s8qqaq=dirpe0xb9q8qiLsFr0=vr0=vr0dc8meaabaqaciaacaGaaeqabaWaaeGaeaaakeaaimaacqWFZestaaa@3790@ = TAA[0,3]GG[1,3]CCTT (taken from our example in Table 1); assume that ε1 = 1, ε2 = 0 and ε3 = 1. There are three possible aggregates for TAA, namely TAN, TNA, and NAA, and four aggregates for CCTT, namely CCTN, CCNT, CNTT, and NCTT, giving a total of 12 aggregate neighbors for ℳ MathType@MTEF@5@5@+=feaafiart1ev1aaatCvAUfKttLearuWrP9MDH5MBPbIqV92AaeXatLxBI9gBamrtHrhAL1wy0L2yHvtyaeHbnfgDOvwBHrxAJfwnaebbnrfifHhDYfgasaacH8akY=wiFfYdH8Gipec8Eeeu0xXdbba9frFj0=OqFfea0dXdd9vqai=hGuQ8kuc9pgc9s8qqaq=dirpe0xb9q8qiLsFr0=vr0=vr0dc8meaabaqaciaacaGaaeqabaWaaeGaeaaakeaaimaacqWFZestaaa@3790@, as illustrated in the figure. EXMOTIF processes each aggregate neighbor in turn. Using a hash-table (or direct lookup table if there are only a few neighbors), it checks if the aggregate neighbor has been processed previously. If yes, it moves on to the next aggregate. If not, it gathers the support information from all of its matching structured motifs, to compute its total support. Next, it also updates the neighbor support value for each of the matching motifs, so that once an aggregate is processed, we no longer require its information. All we need to know is whether it has been processed or not. For example, once the support of the first aggregate TAN[0,3]GG[1,3]CCTN for the example motif ℳ MathType@MTEF@5@5@+=feaafiart1ev1aaatCvAUfKttLearuWrP9MDH5MBPbIqV92AaeXatLxBI9gBamrtHrhAL1wy0L2yHvtyaeHbnfgDOvwBHrxAJfwnaebbnrfifHhDYfgasaacH8akY=wiFfYdH8Gipec8Eeeu0xXdbba9frFj0=OqFfea0dXdd9vqai=hGuQ8kuc9pgc9s8qqaq=dirpe0xb9q8qiLsFr0=vr0=vr0dc8meaabaqaciaacaGaaeqabaWaaeGaeaaakeaaimaacqWFZestaaa@3790@ above is computed, EXMOTIF also updates the neighbor supports for all other matching structured motifs, such as ℳ′ MathType@MTEF@5@5@+=feaafiart1ev1aaatCvAUfeBSjuyZL2yd9gzLbvyNv2CaerbwvMCKfMBHbqedmvETj2BSbWenfgDOvwBHrxAJfwnHbqeg0uy0HwzTfgDPnwy1aqee0evGueE0jxyaibaieIgFLIOYR2NHOxjYhrPYhrPYpI8F4rqqrFfpeea0xe9Lq=Jc9vqaqpepm0xbbG8FasPYRqj0=yi0lXdbba9pGe9qqFf0dXdHuk9fr=xfr=xfrpiWZqaaeaabiGaaiaacaqabeaadaqacqaaaOqaaGWaaiqb=ntinzaafaaaaa@406D@ = TAC[0,3]GG[1,3]CCTG. Later when processing ℳ′ MathType@MTEF@5@5@+=feaafiart1ev1aaatCvAUfeBSjuyZL2yd9gzLbvyNv2CaerbwvMCKfMBHbqedmvETj2BSbWenfgDOvwBHrxAJfwnHbqeg0uy0HwzTfgDPnwy1aqee0evGueE0jxyaibaieIgFLIOYR2NHOxjYhrPYhrPYpI8F4rqqrFfpeea0xe9Lq=Jc9vqaqpepm0xbbG8FasPYRqj0=yi0lXdbba9pGe9qqFf0dXdHuk9fr=xfr=xfrpiWZqaaeaabiGaaiaacaqabeaadaqacqaaaOqaaGWaaiqb=ntinzaafaaaaa@406D@, EXMOTIF can skip the above aggregate and focus on the not yet processed aggregates, e.g., NAC[0,3]GG[1,3]NCTG, and so on.\nFigure 4 Aggregate Neighbors. The pseudo-code for arbitrary substitutions is given in Figure 5. The procedure takes as input the hash-table ℍ containing all structured motifs ℳ MathType@MTEF@5@5@+=feaafiart1ev1aaatCvAUfKttLearuWrP9MDH5MBPbIqV92AaeXatLxBI9gBamrtHrhAL1wy0L2yHvtyaeHbnfgDOvwBHrxAJfwnaebbnrfifHhDYfgasaacH8akY=wiFfYdH8Gipec8Eeeu0xXdbba9frFj0=OqFfea0dXdd9vqai=hGuQ8kuc9pgc9s8qqaq=dirpe0xb9q8qiLsFr0=vr0=vr0dc8meaabaqaciaacaGaaeqabaWaaeGaeaaakeaaimaacqWFZestaaa@3790@ and their supports π(ℳ MathType@MTEF@5@5@+=feaafiart1ev1aaatCvAUfKttLearuWrP9MDH5MBPbIqV92AaeXatLxBI9gBamrtHrhAL1wy0L2yHvtyaeHbnfgDOvwBHrxAJfwnaebbnrfifHhDYfgasaacH8akY=wiFfYdH8Gipec8Eeeu0xXdbba9frFj0=OqFfea0dXdd9vqai=hGuQ8kuc9pgc9s8qqaq=dirpe0xb9q8qiLsFr0=vr0=vr0dc8meaabaqaciaacaGaaeqabaWaaeGaeaaakeaaimaacqWFZestaaa@3790@), the quorum q, and the per simple motif errors ei or the global error ε for the structured motifs. For each structured motif we also maintain its aggregate support πaggregate(ℳ MathType@MTEF@5@5@+=feaafiart1ev1aaatCvAUfKttLearuWrP9MDH5MBPbIqV92AaeXatLxBI9gBamrtHrhAL1wy0L2yHvtyaeHbnfgDOvwBHrxAJfwnaebbnrfifHhDYfgasaacH8akY=wiFfYdH8Gipec8Eeeu0xXdbba9frFj0=OqFfea0dXdd9vqai=hGuQ8kuc9pgc9s8qqaq=dirpe0xb9q8qiLsFr0=vr0=vr0dc8meaabaqaciaacaGaaeqabaWaaeGaeaaakeaaimaacqWFZestaaa@3790@), which is initially set to 0 (line 1). Initially we create all the aggregate neighbors for each extracted structured motif ℳ MathType@MTEF@5@5@+=feaafiart1ev1aaatCvAUfKttLearuWrP9MDH5MBPbIqV92AaeXatLxBI9gBamrtHrhAL1wy0L2yHvtyaeHbnfgDOvwBHrxAJfwnaebbnrfifHhDYfgasaacH8akY=wiFfYdH8Gipec8Eeeu0xXdbba9frFj0=OqFfea0dXdd9vqai=hGuQ8kuc9pgc9s8qqaq=dirpe0xb9q8qiLsFr0=vr0=vr0dc8meaabaqaciaacaGaaeqabaWaaeGaeaaakeaaimaacqWFZestaaa@3790@ (lines 3–7). For each such aggregate neighbor G (line 8), if it has not been processed, we compute its support by adding the individual supports of all its matching motifs ℳ′ MathType@MTEF@5@5@+=feaafiart1ev1aaatCvAUfeBSjuyZL2yd9gzLbvyNv2CaerbwvMCKfMBHbqedmvETj2BSbWenfgDOvwBHrxAJfwnHbqeg0uy0HwzTfgDPnwy1aqee0evGueE0jxyaibaieIgFLIOYR2NHOxjYhrPYhrPYpI8F4rqqrFfpeea0xe9Lq=Jc9vqaqpepm0xbbG8FasPYRqj0=yi0lXdbba9pGe9qqFf0dXdHuk9fr=xfr=xfrpiWZqaaeaabiGaaiaacaqabeaadaqacqaaaOqaaGWaaiqb=ntinzaafaaaaa@406D@ (lines 11–12). Note that these support values are found quickly via the hash-table ℍ. Once the support of an aggregate neighbor is known, we immediately update the aggregate support πaggregate) for each of its contributing matching motifs ℳ′ MathType@MTEF@5@5@+=feaafiart1ev1aaatCvAUfeBSjuyZL2yd9gzLbvyNv2CaerbwvMCKfMBHbqedmvETj2BSbWenfgDOvwBHrxAJfwnHbqeg0uy0HwzTfgDPnwy1aqee0evGueE0jxyaibaieIgFLIOYR2NHOxjYhrPYhrPYpI8F4rqqrFfpeea0xe9Lq=Jc9vqaqpepm0xbbG8FasPYRqj0=yi0lXdbba9pGe9qqFf0dXdHuk9fr=xfr=xfrpiWZqaaeaabiGaaiaacaqabeaadaqacqaaaOqaaGWaaiqb=ntinzaafaaaaa@406D@ (lines 13–14). Note that since each motif has already contributed to the support of the aggregate neighbor (π (G)), we must subtract the initial support of ℳ′ MathType@MTEF@5@5@+=feaafiart1ev1aaatCvAUfeBSjuyZL2yd9gzLbvyNv2CaerbwvMCKfMBHbqedmvETj2BSbWenfgDOvwBHrxAJfwnHbqeg0uy0HwzTfgDPnwy1aqee0evGueE0jxyaibaieIgFLIOYR2NHOxjYhrPYhrPYpI8F4rqqrFfpeea0xe9Lq=Jc9vqaqpepm0xbbG8FasPYRqj0=yi0lXdbba9pGe9qqFf0dXdHuk9fr=xfr=xfrpiWZqaaeaabiGaaiaacaqabeaadaqacqaaaOqaaGWaaiqb=ntinzaafaaaaa@406D@ (π(ℳ′ MathType@MTEF@5@5@+=feaafiart1ev1aaatCvAUfeBSjuyZL2yd9gzLbvyNv2CaerbwvMCKfMBHbqedmvETj2BSbWenfgDOvwBHrxAJfwnHbqeg0uy0HwzTfgDPnwy1aqee0evGueE0jxyaibaieIgFLIOYR2NHOxjYhrPYhrPYpI8F4rqqrFfpeea0xe9Lq=Jc9vqaqpepm0xbbG8FasPYRqj0=yi0lXdbba9pGe9qqFf0dXdHuk9fr=xfr=xfrpiWZqaaeaabiGaaiaacaqabeaadaqacqaaaOqaaGWaaiqb=ntinzaafaaaaa@406D@)) to avoid over-counting. Finally, once all the aggregate neighbors have been processed, we output the structured motif ℳ MathType@MTEF@5@5@+=feaafiart1ev1aaatCvAUfKttLearuWrP9MDH5MBPbIqV92AaeXatLxBI9gBamrtHrhAL1wy0L2yHvtyaeHbnfgDOvwBHrxAJfwnaebbnrfifHhDYfgasaacH8akY=wiFfYdH8Gipec8Eeeu0xXdbba9frFj0=OqFfea0dXdd9vqai=hGuQ8kuc9pgc9s8qqaq=dirpe0xb9q8qiLsFr0=vr0=vr0dc8meaabaqaciaacaGaaeqabaWaaeGaeaaakeaaimaacqWFZestaaa@3790@, provided π(ℳ MathType@MTEF@5@5@+=feaafiart1ev1aaatCvAUfKttLearuWrP9MDH5MBPbIqV92AaeXatLxBI9gBamrtHrhAL1wy0L2yHvtyaeHbnfgDOvwBHrxAJfwnaebbnrfifHhDYfgasaacH8akY=wiFfYdH8Gipec8Eeeu0xXdbba9frFj0=OqFfea0dXdd9vqai=hGuQ8kuc9pgc9s8qqaq=dirpe0xb9q8qiLsFr0=vr0=vr0dc8meaabaqaciaacaGaaeqabaWaaeGaeaaakeaaimaacqWFZestaaa@3790@) + πaggregate(ℳ MathType@MTEF@5@5@+=feaafiart1ev1aaatCvAUfKttLearuWrP9MDH5MBPbIqV92AaeXatLxBI9gBamrtHrhAL1wy0L2yHvtyaeHbnfgDOvwBHrxAJfwnaebbnrfifHhDYfgasaacH8akY=wiFfYdH8Gipec8Eeeu0xXdbba9frFj0=OqFfea0dXdd9vqai=hGuQ8kuc9pgc9s8qqaq=dirpe0xb9q8qiLsFr0=vr0=vr0dc8meaabaqaciaacaGaaeqabaWaaeGaeaaakeaaimaacqWFZestaaa@3790@) meets the quorum requirement (line 14).\nFigure 5 Arbitrary Substitutions.\n\nCounting support\nThere are two methods to record the support for each motif. In the first method, we associate each motif with a bit vector, V MathType@MTEF@5@5@+=feaafiart1ev1aaatCvAUfKttLearuWrP9MDH5MBPbIqV92AaeXatLxBI9gBamrtHrhAL1wy0L2yHvtyaeHbnfgDOvwBHrxAJfwnaebbnrfifHhDYfgasaacH8akY=wiFfYdH8Gipec8Eeeu0xXdbba9frFj0=OqFfea0dXdd9vqai=hGuQ8kuc9pgc9s8qqaq=dirpe0xb9q8qiLsFr0=vr0=vr0dc8meaabaqaciaacaGaaeqabaWaaeGaeaaakeaaimaacqWFveVvaaa@384B@. Each bit, V MathType@MTEF@5@5@+=feaafiart1ev1aaatCvAUfKttLearuWrP9MDH5MBPbIqV92AaeXatLxBI9gBamrtHrhAL1wy0L2yHvtyaeHbnfgDOvwBHrxAJfwnaebbnrfifHhDYfgasaacH8akY=wiFfYdH8Gipec8Eeeu0xXdbba9frFj0=OqFfea0dXdd9vqai=hGuQ8kuc9pgc9s8qqaq=dirpe0xb9q8qiLsFr0=vr0=vr0dc8meaabaqaciaacaGaaeqabaWaaeGaeaaakeaaimaacqWFveVvaaa@384B@i for 1 ≤ i ≤ n (where n = |S MathType@MTEF@5@5@+=feaafiart1ev1aaatCvAUfKttLearuWrP9MDH5MBPbIqV92AaeXatLxBI9gBamrtHrhAL1wy0L2yHvtyaeHbnfgDOvwBHrxAJfwnaebbnrfifHhDYfgasaacH8akY=wiFfYdH8Gipec8Eeeu0xXdbba9frFj0=OqFfea0dXdd9vqai=hGuQ8kuc9pgc9s8qqaq=dirpe0xb9q8qiLsFr0=vr0=vr0dc8meaabaqaciaacaGaaeqabaWaaeGaeaaakeaaimaacqWFse=uaaa@3845@|) indicates whether the motif is present in the sequence Si ∈ S MathType@MTEF@5@5@+=feaafiart1ev1aaatCvAUfKttLearuWrP9MDH5MBPbIqV92AaeXatLxBI9gBamrtHrhAL1wy0L2yHvtyaeHbnfgDOvwBHrxAJfwnaebbnrfifHhDYfgasaacH8akY=wiFfYdH8Gipec8Eeeu0xXdbba9frFj0=OqFfea0dXdd9vqai=hGuQ8kuc9pgc9s8qqaq=dirpe0xb9q8qiLsFr0=vr0=vr0dc8meaabaqaciaacaGaaeqabaWaaeGaeaaakeaaimaacqWFse=uaaa@3845@. The support of the motif is the number of set bits in V MathType@MTEF@5@5@+=feaafiart1ev1aaatCvAUfKttLearuWrP9MDH5MBPbIqV92AaeXatLxBI9gBamrtHrhAL1wy0L2yHvtyaeHbnfgDOvwBHrxAJfwnaebbnrfifHhDYfgasaacH8akY=wiFfYdH8Gipec8Eeeu0xXdbba9frFj0=OqFfea0dXdd9vqai=hGuQ8kuc9pgc9s8qqaq=dirpe0xb9q8qiLsFr0=vr0=vr0dc8meaabaqaciaacaGaaeqabaWaaeGaeaaakeaaimaacqWFveVvaaa@384B@. Thus to obtain the support for a motif, we can simply union the bit vectors of all its (aggregate) neighbors. Using one bit to represent a sequence saves space, and also saves time via the union operation. However, since we need n fixed bits for each motif to store its bit vector, this is not efficient if there are many sequences, and if a motif occurs only in a small number of sequences, which leads to a sparse bit vector. Thus in the second method, EXMOTIF associates each motif with an identifier array, Q MathType@MTEF@5@5@+=feaafiart1ev1aaatCvAUfKttLearuWrP9MDH5MBPbIqV92AaeXatLxBI9gBamrtHrhAL1wy0L2yHvtyaeHbnfgDOvwBHrxAJfwnaebbnrfifHhDYfgasaacH8akY=wiFfYdH8Gipec8Eeeu0xXdbba9frFj0=OqFfea0dXdd9vqai=hGuQ8kuc9pgc9s8qqaq=dirpe0xb9q8qiLsFr0=vr0=vr0dc8meaabaqaciaacaGaaeqabaWaaeGaeaaakeaaimaacqWFqeFuaaa@3841@, to only store the sequence identifiers in which the motif occurs. EXMOTIF can then obtain the support for a motif by scanning the identifier arrays of its neighbors in linear time. For example consider again our motif (from Table 1), TAT[0,1]GG[2,3]CCAT, which occurs in S2 and S3, Its bit vector is thus V MathType@MTEF@5@5@+=feaafiart1ev1aaatCvAUfKttLearuWrP9MDH5MBPbIqV92AaeXatLxBI9gBamrtHrhAL1wy0L2yHvtyaeHbnfgDOvwBHrxAJfwnaebbnrfifHhDYfgasaacH8akY=wiFfYdH8Gipec8Eeeu0xXdbba9frFj0=OqFfea0dXdd9vqai=hGuQ8kuc9pgc9s8qqaq=dirpe0xb9q8qiLsFr0=vr0=vr0dc8meaabaqaciaacaGaaeqabaWaaeGaeaaakeaaimaacqWFveVvaaa@384B@ = {0110} and its identifier array Q MathType@MTEF@5@5@+=feaafiart1ev1aaatCvAUfKttLearuWrP9MDH5MBPbIqV92AaeXatLxBI9gBamrtHrhAL1wy0L2yHvtyaeHbnfgDOvwBHrxAJfwnaebbnrfifHhDYfgasaacH8akY=wiFfYdH8Gipec8Eeeu0xXdbba9frFj0=OqFfea0dXdd9vqai=hGuQ8kuc9pgc9s8qqaq=dirpe0xb9q8qiLsFr0=vr0=vr0dc8meaabaqaciaacaGaaeqabaWaaeGaeaaakeaaimaacqWFqeFuaaa@3841@ = {2, 3}.\n\nCreating positional weight matrices\nFor any frequent structured motif ℳ MathType@MTEF@5@5@+=feaafiart1ev1aaatCvAUfKttLearuWrP9MDH5MBPbIqV92AaeXatLxBI9gBamrtHrhAL1wy0L2yHvtyaeHbnfgDOvwBHrxAJfwnaebbnrfifHhDYfgasaacH8akY=wiFfYdH8Gipec8Eeeu0xXdbba9frFj0=OqFfea0dXdd9vqai=hGuQ8kuc9pgc9s8qqaq=dirpe0xb9q8qiLsFr0=vr0=vr0dc8meaabaqaciaacaGaaeqabaWaaeGaeaaakeaaimaacqWFZestaaa@3790@, we can summarize the information about its neighbors (including ℳ MathType@MTEF@5@5@+=feaafiart1ev1aaatCvAUfKttLearuWrP9MDH5MBPbIqV92AaeXatLxBI9gBamrtHrhAL1wy0L2yHvtyaeHbnfgDOvwBHrxAJfwnaebbnrfifHhDYfgasaacH8akY=wiFfYdH8Gipec8Eeeu0xXdbba9frFj0=OqFfea0dXdd9vqai=hGuQ8kuc9pgc9s8qqaq=dirpe0xb9q8qiLsFr0=vr0=vr0dc8meaabaqaciaacaGaaeqabaWaaeGaeaaakeaaimaacqWFZestaaa@3790@) by computing a Positional Weight Matrix (PWM). The PWM for a structured motif ℳ MathType@MTEF@5@5@+=feaafiart1ev1aaatCvAUfKttLearuWrP9MDH5MBPbIqV92AaeXatLxBI9gBamrtHrhAL1wy0L2yHvtyaeHbnfgDOvwBHrxAJfwnaebbnrfifHhDYfgasaacH8akY=wiFfYdH8Gipec8Eeeu0xXdbba9frFj0=OqFfea0dXdd9vqai=hGuQ8kuc9pgc9s8qqaq=dirpe0xb9q8qiLsFr0=vr0=vr0dc8meaabaqaciaacaGaaeqabaWaaeGaeaaakeaaimaacqWFZestaaa@3790@ gives for each non-gap position the likelihood of occurrence for each symbol in ΣDNA. The PWM W MathType@MTEF@5@5@+=feaafiart1ev1aaatCvAUfKttLearuWrP9MDH5MBPbIqV92AaeXatLxBI9gBamrtHrhAL1wy0L2yHvtyaeHbnfgDOvwBHrxAJfwnaebbnrfifHhDYfgasaacH8akY=wiFfYdH8Gipec8Eeeu0xXdbba9frFj0=OqFfea0dXdd9vqai=hGuQ8kuc9pgc9s8qqaq=dirpe0xb9q8qiLsFr0=vr0=vr0dc8meaabaqaciaacaGaaeqabaWaaeGaeaaakeaaimaacqWFwe=vaaa@384D@ for ℳ MathType@MTEF@5@5@+=feaafiart1ev1aaatCvAUfKttLearuWrP9MDH5MBPbIqV92AaeXatLxBI9gBamrtHrhAL1wy0L2yHvtyaeHbnfgDOvwBHrxAJfwnaebbnrfifHhDYfgasaacH8akY=wiFfYdH8Gipec8Eeeu0xXdbba9frFj0=OqFfea0dXdd9vqai=hGuQ8kuc9pgc9s8qqaq=dirpe0xb9q8qiLsFr0=vr0=vr0dc8meaabaqaciaacaGaaeqabaWaaeGaeaaakeaaimaacqWFZestaaa@3790@ is calculated as follows:\nr i j = f i j + p i ∑ k = 1 | Σ DNA | f k j + p k , W i j = ln ( r i j p i ) ( 1 ) MathType@MTEF@5@5@+=feaafiart1ev1aaatCvAUfKttLearuWrP9MDH5MBPbIqV92AaeXatLxBI9gBamrtHrhAL1wy0L2yHvtyaeHbnfgDOvwBHrxAJfwnaebbnrfifHhDYfgasaacH8akY=wiFfYdH8Gipec8Eeeu0xXdbba9frFj0=OqFfea0dXdd9vqai=hGuQ8kuc9pgc9s8qqaq=dirpe0xb9q8qiLsFr0=vr0=vr0dc8meaabaqaciaacaGaaeqabaWaaeGaeaaakeaafaqabeqacaaabaGaemOCai3aaSbaaSqaaiabdMgaPjabdQgaQbqabaGccqGH9aqpdaWcaaqaaiabdAgaMnaaBaaaleaacqWGPbqAcqWGQbGAaeqaaOGaey4kaSIaemiCaa3aaSbaaSqaaiabdMgaPbqabaaakeaadaaeWaqaaiabdAgaMnaaBaaaleaacqWGRbWAcqWGQbGAaeqaaOGaey4kaSIaemiCaa3aaSbaaSqaaiabdUgaRbqabaaabaGaem4AaSMaeyypa0JaeGymaedabaWaaqWaaeaacqqHJoWudaWgaaadbaGaeeiraqKaeeOta4KaeeyqaeeabeaaaSGaay5bSlaawIa7aaqdcqGHris5aaaakiabcYcaSaqaaGWaaiab=zr8xnaaBaaaleaacqWGPbqAcqWGQbGAaeqaaOGaeyypa0JagiiBaWMaeiOBa42aaeWaaeaadaWcaaqaaiabdkhaYnaaBaaaleaacqWGPbqAcqWGQbGAaeqaaaGcbaGaemiCaa3aaSbaaSqaaiabdMgaPbqabaaaaaGccaGLOaGaayzkaaaaaiaaxMaacaWLjaWaaeWaaeaacqaIXaqmaiaawIcacaGLPaaaaaa@6FBB@\nwhere, fij and rij represent the observed and relative frequency of symbol i at position j, respectively, pi is the prior probability of symbol i, and W MathType@MTEF@5@5@+=feaafiart1ev1aaatCvAUfKttLearuWrP9MDH5MBPbIqV92AaeXatLxBI9gBamrtHrhAL1wy0L2yHvtyaeHbnfgDOvwBHrxAJfwnaebbnrfifHhDYfgasaacH8akY=wiFfYdH8Gipec8Eeeu0xXdbba9frFj0=OqFfea0dXdd9vqai=hGuQ8kuc9pgc9s8qqaq=dirpe0xb9q8qiLsFr0=vr0=vr0dc8meaabaqaciaacaGaaeqabaWaaeGaeaaakeaaimaacqWFwe=vaaa@384D@ij is the weight (log-likelihood) of observing symbol i at position j. Whereas W MathType@MTEF@5@5@+=feaafiart1ev1aaatCvAUfKttLearuWrP9MDH5MBPbIqV92AaeXatLxBI9gBamrtHrhAL1wy0L2yHvtyaeHbnfgDOvwBHrxAJfwnaebbnrfifHhDYfgasaacH8akY=wiFfYdH8Gipec8Eeeu0xXdbba9frFj0=OqFfea0dXdd9vqai=hGuQ8kuc9pgc9s8qqaq=dirpe0xb9q8qiLsFr0=vr0=vr0dc8meaabaqaciaacaGaaeqabaWaaeGaeaaakeaaimaacqWFwe=vaaa@384D@ gives the likelihood of observing a given symbol in a given position in ℳ MathType@MTEF@5@5@+=feaafiart1ev1aaatCvAUfKttLearuWrP9MDH5MBPbIqV92AaeXatLxBI9gBamrtHrhAL1wy0L2yHvtyaeHbnfgDOvwBHrxAJfwnaebbnrfifHhDYfgasaacH8akY=wiFfYdH8Gipec8Eeeu0xXdbba9frFj0=OqFfea0dXdd9vqai=hGuQ8kuc9pgc9s8qqaq=dirpe0xb9q8qiLsFr0=vr0=vr0dc8meaabaqaciaacaGaaeqabaWaaeGaeaaakeaaimaacqWFZestaaa@3790@ it does not account for the degree to which some symbols are conserved at some positions. We can adjust the weights W MathType@MTEF@5@5@+=feaafiart1ev1aaatCvAUfKttLearuWrP9MDH5MBPbIqV92AaeXatLxBI9gBamrtHrhAL1wy0L2yHvtyaeHbnfgDOvwBHrxAJfwnaebbnrfifHhDYfgasaacH8akY=wiFfYdH8Gipec8Eeeu0xXdbba9frFj0=OqFfea0dXdd9vqai=hGuQ8kuc9pgc9s8qqaq=dirpe0xb9q8qiLsFr0=vr0=vr0dc8meaabaqaciaacaGaaeqabaWaaeGaeaaakeaaimaacqWFwe=vaaa@384D@ij by considering the information content at each position. The information content for a PWM is given as:\nℐ i j = r i j ln ( r i j ) − p i ln ( p i ) , ℐ j = ∑ i = 1 | Σ DNA | ℐ i j , ℐ W = ∑ j = 1 K ℐ j ( 2 ) MathType@MTEF@5@5@+=feaafiart1ev1aaatCvAUfKttLearuWrP9MDH5MBPbIqV92AaeXatLxBI9gBamrtHrhAL1wy0L2yHvtyaeHbnfgDOvwBHrxAJfwnaebbnrfifHhDYfgasaacH8akY=wiFfYdH8Gipec8Eeeu0xXdbba9frFj0=OqFfea0dXdd9vqai=hGuQ8kuc9pgc9s8qqaq=dirpe0xb9q8qiLsFr0=vr0=vr0dc8meaabaqaciaacaGaaeqabaWaaeGaeaaakeaafaqabeqadaaabaacdaGae8heHK0aaSbaaSqaaiabdMgaPjabdQgaQbqabaGccqGH9aqpcqWGYbGCdaWgaaWcbaGaemyAaKMaemOAaOgabeaakiGbcYgaSjabc6gaUjabcIcaOiabdkhaYnaaBaaaleaacqWGPbqAcqWGQbGAaeqaaOGaeiykaKIaeyOeI0IaemiCaa3aaSbaaSqaaiabdMgaPbqabaGccyGGSbaBcqGGUbGBcqGGOaakcqWGWbaCdaWgaaWcbaGaemyAaKgabeaakiabcMcaPiabcYcaSaqaaiab=brijnaaBaaaleaacqWGQbGAaeqaaOGaeyypa0ZaaabCaeaacqWFqessdaWgaaWcbaGaemyAaKMaemOAaOgabeaaaeaacqWGPbqAcqGH9aqpcqaIXaqmaeaadaabdaqaaiabfo6atnaaBaaameaacqqGebarcqqGobGtcqqGbbqqaeqaaaWccaGLhWUaayjcSdaaniabggHiLdGccqGGSaalaeaacqWFqessdaWgaaWcbaGae8NfXFfabeaakiabg2da9maaqahabaGae8heHK0aaSbaaSqaaiabdQgaQbqabaaabaGaemOAaOMaeyypa0JaeGymaedabaGaem4saSeaniabggHiLdaaaOGaaCzcaiaaxMaadaqadaqaaiabikdaYaGaayjkaiaawMcaaaaa@7BF1@\nwhere K is the number of symbols in ℳ MathType@MTEF@5@5@+=feaafiart1ev1aaatCvAUfKttLearuWrP9MDH5MBPbIqV92AaeXatLxBI9gBamrtHrhAL1wy0L2yHvtyaeHbnfgDOvwBHrxAJfwnaebbnrfifHhDYfgasaacH8akY=wiFfYdH8Gipec8Eeeu0xXdbba9frFj0=OqFfea0dXdd9vqai=hGuQ8kuc9pgc9s8qqaq=dirpe0xb9q8qiLsFr0=vr0=vr0dc8meaabaqaciaacaGaaeqabaWaaeGaeaaakeaaimaacqWFZestaaa@3790@; ℐ MathType@MTEF@5@5@+=feaafiart1ev1aaatCvAUfKttLearuWrP9MDH5MBPbIqV92AaeXatLxBI9gBamrtHrhAL1wy0L2yHvtyaeHbnfgDOvwBHrxAJfwnaebbnrfifHhDYfgasaacH8akY=wiFfYdH8Gipec8Eeeu0xXdbba9frFj0=OqFfea0dXdd9vqai=hGuQ8kuc9pgc9s8qqaq=dirpe0xb9q8qiLsFr0=vr0=vr0dc8meaabaqaciaacaGaaeqabaWaaeGaeaaakeaaimaacqWFqessaaa@3769@ij is the information content of symbol i at position j; ℐ MathType@MTEF@5@5@+=feaafiart1ev1aaatCvAUfKttLearuWrP9MDH5MBPbIqV92AaeXatLxBI9gBamrtHrhAL1wy0L2yHvtyaeHbnfgDOvwBHrxAJfwnaebbnrfifHhDYfgasaacH8akY=wiFfYdH8Gipec8Eeeu0xXdbba9frFj0=OqFfea0dXdd9vqai=hGuQ8kuc9pgc9s8qqaq=dirpe0xb9q8qiLsFr0=vr0=vr0dc8meaabaqaciaacaGaaeqabaWaaeGaeaaakeaaimaacqWFqessaaa@3769@j is the information content over all bases at position j; and ℐW MathType@MTEF@5@5@+=feaafiart1ev1aaatCvAUfKttLearuWrP9MDH5MBPbIqV92AaeXatLxBI9gBamrtHrhAL1wy0L2yHvtyaeHbnfgDOvwBHrxAJfwnaebbnrfifHhDYfgasaacH8akY=wiFfYdH8Gipec8Eeeu0xXdbba9frFj0=OqFfea0dXdd9vqai=hGuQ8kuc9pgc9s8qqaq=dirpe0xb9q8qiLsFr0=vr0=vr0dc8meaabaqaciaacaGaaeqabaWaaeGaeaaakeaaimaacqWFqessdaWgaaWcbaGae8NfXFfabeaaaaa@3978@ is the information content of the entire matrix W MathType@MTEF@5@5@+=feaafiart1ev1aaatCvAUfKttLearuWrP9MDH5MBPbIqV92AaeXatLxBI9gBamrtHrhAL1wy0L2yHvtyaeHbnfgDOvwBHrxAJfwnaebbnrfifHhDYfgasaacH8akY=wiFfYdH8Gipec8Eeeu0xXdbba9frFj0=OqFfea0dXdd9vqai=hGuQ8kuc9pgc9s8qqaq=dirpe0xb9q8qiLsFr0=vr0=vr0dc8meaabaqaciaacaGaaeqabaWaaeGaeaaakeaaimaacqWFwe=vaaa@384D@. To allow mismatches at less conserved positions to be more easily tolerated than those at highly conserved positions, we multiply each W MathType@MTEF@5@5@+=feaafiart1ev1aaatCvAUfKttLearuWrP9MDH5MBPbIqV92AaeXatLxBI9gBamrtHrhAL1wy0L2yHvtyaeHbnfgDOvwBHrxAJfwnaebbnrfifHhDYfgasaacH8akY=wiFfYdH8Gipec8Eeeu0xXdbba9frFj0=OqFfea0dXdd9vqai=hGuQ8kuc9pgc9s8qqaq=dirpe0xb9q8qiLsFr0=vr0=vr0dc8meaabaqaciaacaGaaeqabaWaaeGaeaaakeaaimaacqWFwe=vaaa@384D@ij by ℐ MathType@MTEF@5@5@+=feaafiart1ev1aaatCvAUfKttLearuWrP9MDH5MBPbIqV92AaeXatLxBI9gBamrtHrhAL1wy0L2yHvtyaeHbnfgDOvwBHrxAJfwnaebbnrfifHhDYfgasaacH8akY=wiFfYdH8Gipec8Eeeu0xXdbba9frFj0=OqFfea0dXdd9vqai=hGuQ8kuc9pgc9s8qqaq=dirpe0xb9q8qiLsFr0=vr0=vr0dc8meaabaqaciaacaGaaeqabaWaaeGaeaaakeaaimaacqWFqessaaa@3769@j, which is larger for more conserved positions. As a result, the corrected weight of each element in the PWM W MathType@MTEF@5@5@+=feaafiart1ev1aaatCvAUfKttLearuWrP9MDH5MBPbIqV92AaeXatLxBI9gBamrtHrhAL1wy0L2yHvtyaeHbnfgDOvwBHrxAJfwnaebbnrfifHhDYfgasaacH8akY=wiFfYdH8Gipec8Eeeu0xXdbba9frFj0=OqFfea0dXdd9vqai=hGuQ8kuc9pgc9s8qqaq=dirpe0xb9q8qiLsFr0=vr0=vr0dc8meaabaqaciaacaGaaeqabaWaaeGaeaaakeaaimaacqWFwe=vaaa@384D@ becomes:\nW i j c = ℐ j ln ( r i j p i ) ( 3 ) MathType@MTEF@5@5@+=feaafiart1ev1aaatCvAUfKttLearuWrP9MDH5MBPbIqV92AaeXatLxBI9gBamrtHrhAL1wy0L2yHvtyaeHbnfgDOvwBHrxAJfwnaebbnrfifHhDYfgasaacH8akY=wiFfYdH8Gipec8Eeeu0xXdbba9frFj0=OqFfea0dXdd9vqai=hGuQ8kuc9pgc9s8qqaq=dirpe0xb9q8qiLsFr0=vr0=vr0dc8meaabaqaciaacaGaaeqabaWaaeGaeaaakeaaimaacqWFwe=vdaqhaaWcbaGaemyAaKMaemOAaOgabaGaem4yamgaaOGaeyypa0Jae8heHK0aaSbaaSqaaiabdQgaQbqabaGccyGGSbaBcqGGUbGBdaqadaqaamaalaaabaGaemOCai3aaSbaaSqaaiabdMgaPjabdQgaQbqabaaakeaacqWGWbaCdaWgaaWcbaGaemyAaKgabeaaaaaakiaawIcacaGLPaaacaWLjaGaaCzcamaabmaabaGaeG4mamdacaGLOaGaayzkaaaaaa@4F98@\nThen we can calculate the PWM score, ℛ MathType@MTEF@5@5@+=feaafiart1ev1aaatCvAUfKttLearuWrP9MDH5MBPbIqV92AaeXatLxBI9gBamrtHrhAL1wy0L2yHvtyaeHbnfgDOvwBHrxAJfwnaebbnrfifHhDYfgasaacH8akY=wiFfYdH8Gipec8Eeeu0xXdbba9frFj0=OqFfea0dXdd9vqai=hGuQ8kuc9pgc9s8qqaq=dirpe0xb9q8qiLsFr0=vr0=vr0dc8meaabaqaciaacaGaaeqabaWaaeGaeaaakeaaimaacqWFBeIuaaa@377D@, for a structured motif, ℳ MathType@MTEF@5@5@+=feaafiart1ev1aaatCvAUfKttLearuWrP9MDH5MBPbIqV92AaeXatLxBI9gBamrtHrhAL1wy0L2yHvtyaeHbnfgDOvwBHrxAJfwnaebbnrfifHhDYfgasaacH8akY=wiFfYdH8Gipec8Eeeu0xXdbba9frFj0=OqFfea0dXdd9vqai=hGuQ8kuc9pgc9s8qqaq=dirpe0xb9q8qiLsFr0=vr0=vr0dc8meaabaqaciaacaGaaeqabaWaaeGaeaaakeaaimaacqWFZestaaa@3790@, by summing up the positional weights for the bases in ℳ MathType@MTEF@5@5@+=feaafiart1ev1aaatCvAUfKttLearuWrP9MDH5MBPbIqV92AaeXatLxBI9gBamrtHrhAL1wy0L2yHvtyaeHbnfgDOvwBHrxAJfwnaebbnrfifHhDYfgasaacH8akY=wiFfYdH8Gipec8Eeeu0xXdbba9frFj0=OqFfea0dXdd9vqai=hGuQ8kuc9pgc9s8qqaq=dirpe0xb9q8qiLsFr0=vr0=vr0dc8meaabaqaciaacaGaaeqabaWaaeGaeaaakeaaimaacqWFZestaaa@3790@, given as ℛ=∑j=1KWℳ[j]jc MathType@MTEF@5@5@+=feaafiart1ev1aaatCvAUfKttLearuWrP9MDH5MBPbIqV92AaeXatLxBI9gBamrtHrhAL1wy0L2yHvtyaeHbnfgDOvwBHrxAJfwnaebbnrfifHhDYfgasaacH8akY=wiFfYdH8Gipec8Eeeu0xXdbba9frFj0=OqFfea0dXdd9vqai=hGuQ8kuc9pgc9s8qqaq=dirpe0xb9q8qiLsFr0=vr0=vr0dc8meaabaqaciaacaGaaeqabaWaaeGaeaaakeaaimaacqWFBeIucqGH9aqpdaaeWaqaaiab=zr8xnaaDaaaleaacqWFZestcqGGBbWwcqWGQbGAcqGGDbqxcqWGQbGAaeaacqWGJbWyaaaabaGaemOAaOMaeyypa0JaeGymaedabaGaem4saSeaniabggHiLdaaaa@48AB@. Thus for each ℳ MathType@MTEF@5@5@+=feaafiart1ev1aaatCvAUfKttLearuWrP9MDH5MBPbIqV92AaeXatLxBI9gBamrtHrhAL1wy0L2yHvtyaeHbnfgDOvwBHrxAJfwnaebbnrfifHhDYfgasaacH8akY=wiFfYdH8Gipec8Eeeu0xXdbba9frFj0=OqFfea0dXdd9vqai=hGuQ8kuc9pgc9s8qqaq=dirpe0xb9q8qiLsFr0=vr0=vr0dc8meaabaqaciaacaGaaeqabaWaaeGaeaaakeaaimaacqWFZestaaa@3790@, its PWM score and PWM information content can be further used to measure whether ℳ MathType@MTEF@5@5@+=feaafiart1ev1aaatCvAUfKttLearuWrP9MDH5MBPbIqV92AaeXatLxBI9gBamrtHrhAL1wy0L2yHvtyaeHbnfgDOvwBHrxAJfwnaebbnrfifHhDYfgasaacH8akY=wiFfYdH8Gipec8Eeeu0xXdbba9frFj0=OqFfea0dXdd9vqai=hGuQ8kuc9pgc9s8qqaq=dirpe0xb9q8qiLsFr0=vr0=vr0dc8meaabaqaciaacaGaaeqabaWaaeGaeaaakeaaimaacqWFZestaaa@3790@ is a significant motif.\n\nSolving repeated structured motif identification problem\nIn repeated structured motif identification problem, the frequency closure property (that all the subsequences of a frequent sequence must be frequent), does not hold any more. For example, the sequence GCTTT, has three occurrences of pattern G[1,3]T, but it sub-pattern, G, has only one occurrence. Thus we cannot apply the closure property for pruning candidates. Nevertheless, a bound on the frequency of a sub-pattern can be established, which can be used for pruning.\nTheorem 1. Let ℳ MathType@MTEF@5@5@+=feaafiart1ev1aaatCvAUfKttLearuWrP9MDH5MBPbIqV92AaeXatLxBI9gBamrtHrhAL1wy0L2yHvtyaeHbnfgDOvwBHrxAJfwnaebbnrfifHhDYfgasaacH8akY=wiFfYdH8Gipec8Eeeu0xXdbba9frFj0=OqFfea0dXdd9vqai=hGuQ8kuc9pgc9s8qqaq=dirpe0xb9q8qiLsFr0=vr0=vr0dc8meaabaqaciaacaGaaeqabaWaaeGaeaaakeaaimaacqWFZestaaa@3790@ = M1 ... Mk be a structured motif and ℳ′ MathType@MTEF@5@5@+=feaafiart1ev1aaatCvAUfeBSjuyZL2yd9gzLbvyNv2CaerbwvMCKfMBHbqedmvETj2BSbWenfgDOvwBHrxAJfwnHbqeg0uy0HwzTfgDPnwy1aqee0evGueE0jxyaibaieIgFLIOYR2NHOxjYhrPYhrPYpI8F4rqqrFfpeea0xe9Lq=Jc9vqaqpepm0xbbG8FasPYRqj0=yi0lXdbba9pGe9qqFf0dXdHuk9fr=xfr=xfrpiWZqaaeaabiGaaiaacaqabeaadaqacqaaaOqaaGWaaiqb=ntinzaafaaaaa@406D@ = Mi ... Mk be a suffix of ℳ MathType@MTEF@5@5@+=feaafiart1ev1aaatCvAUfKttLearuWrP9MDH5MBPbIqV92AaeXatLxBI9gBamrtHrhAL1wy0L2yHvtyaeHbnfgDOvwBHrxAJfwnaebbnrfifHhDYfgasaacH8akY=wiFfYdH8Gipec8Eeeu0xXdbba9frFj0=OqFfea0dXdd9vqai=hGuQ8kuc9pgc9s8qqaq=dirpe0xb9q8qiLsFr0=vr0=vr0dc8meaabaqaciaacaGaaeqabaWaaeGaeaaakeaaimaacqWFZestaaa@3790@, for 1 ≤ i ≤ k. If the weighted support of ℳ MathType@MTEF@5@5@+=feaafiart1ev1aaatCvAUfKttLearuWrP9MDH5MBPbIqV92AaeXatLxBI9gBamrtHrhAL1wy0L2yHvtyaeHbnfgDOvwBHrxAJfwnaebbnrfifHhDYfgasaacH8akY=wiFfYdH8Gipec8Eeeu0xXdbba9frFj0=OqFfea0dXdd9vqai=hGuQ8kuc9pgc9s8qqaq=dirpe0xb9q8qiLsFr0=vr0=vr0dc8meaabaqaciaacaGaaeqabaWaaeGaeaaakeaaimaacqWFZestaaa@3790@is πw (ℳ MathType@MTEF@5@5@+=feaafiart1ev1aaatCvAUfKttLearuWrP9MDH5MBPbIqV92AaeXatLxBI9gBamrtHrhAL1wy0L2yHvtyaeHbnfgDOvwBHrxAJfwnaebbnrfifHhDYfgasaacH8akY=wiFfYdH8Gipec8Eeeu0xXdbba9frFj0=OqFfea0dXdd9vqai=hGuQ8kuc9pgc9s8qqaq=dirpe0xb9q8qiLsFr0=vr0=vr0dc8meaabaqaciaacaGaaeqabaWaaeGaeaaakeaaimaacqWFZestaaa@3790@), then πw(ℳ′)≥πw(ℳ)∏m=1i−1Wm MathType@MTEF@5@5@+=feaafiart1ev1aaatCvAUfKttLearuWrP9MDH5MBPbIqV92AaeXatLxBI9gBamrtHrhAL1wy0L2yHvtyaeHbnfgDOvwBHrxAJfwnaebbnrfifHhDYfgasaacH8akY=wiFfYdH8Gipec8Eeeu0xXdbba9frFj0=OqFfea0dXdd9vqai=hGuQ8kuc9pgc9s8qqaq=dirpe0xb9q8qiLsFr0=vr0=vr0dc8meaabaqaciaacaGaaeqabaWaaeGaeaaakeaaiiGacqWFapaCdaWgaaWcbaGaem4DaChabeaakiabcIcaOGWaaiqb+ntinzaafaGaeiykaKIaeyyzIm7aaSaaaeaacqWFapaCdaWgaaWcbaGaem4DaChabeaakiabcIcaOiab+ntinjabcMcaPaqaamaaradabaGaem4vaC1aaSbaaSqaaiabd2gaTbqabaaabaGaemyBa0Maeyypa0JaeGymaedabaGaemyAaKMaeyOeI0IaeGymaedaniabg+Givdaaaaaa@500D@, where Wm = um - lm + 1 is the span of the gap range for m ∈ [1, k - 1].\nProof. Let O MathType@MTEF@5@5@+=feaafiart1ev1aaatCvAUfKttLearuWrP9MDH5MBPbIqV92AaeXatLxBI9gBamrtHrhAL1wy0L2yHvtyaeHbnfgDOvwBHrxAJfwnaebbnrfifHhDYfgasaacH8akY=wiFfYdH8Gipec8Eeeu0xXdbba9frFj0=OqFfea0dXdd9vqai=hGuQ8kuc9pgc9s8qqaq=dirpe0xb9q8qiLsFr0=vr0=vr0dc8meaabaqaciaacaGaaeqabaWaaeGaeaaakeaaimaacqWFoe=taaa@383D@(ℳ MathType@MTEF@5@5@+=feaafiart1ev1aaatCvAUfKttLearuWrP9MDH5MBPbIqV92AaeXatLxBI9gBamrtHrhAL1wy0L2yHvtyaeHbnfgDOvwBHrxAJfwnaebbnrfifHhDYfgasaacH8akY=wiFfYdH8Gipec8Eeeu0xXdbba9frFj0=OqFfea0dXdd9vqai=hGuQ8kuc9pgc9s8qqaq=dirpe0xb9q8qiLsFr0=vr0=vr0dc8meaabaqaciaacaGaaeqabaWaaeGaeaaakeaaimaacqWFZestaaa@3790@) be the occurrence set of ℳ MathType@MTEF@5@5@+=feaafiart1ev1aaatCvAUfKttLearuWrP9MDH5MBPbIqV92AaeXatLxBI9gBamrtHrhAL1wy0L2yHvtyaeHbnfgDOvwBHrxAJfwnaebbnrfifHhDYfgasaacH8akY=wiFfYdH8Gipec8Eeeu0xXdbba9frFj0=OqFfea0dXdd9vqai=hGuQ8kuc9pgc9s8qqaq=dirpe0xb9q8qiLsFr0=vr0=vr0dc8meaabaqaciaacaGaaeqabaWaaeGaeaaakeaaimaacqWFZestaaa@3790@ and O MathType@MTEF@5@5@+=feaafiart1ev1aaatCvAUfKttLearuWrP9MDH5MBPbIqV92AaeXatLxBI9gBamrtHrhAL1wy0L2yHvtyaeHbnfgDOvwBHrxAJfwnaebbnrfifHhDYfgasaacH8akY=wiFfYdH8Gipec8Eeeu0xXdbba9frFj0=OqFfea0dXdd9vqai=hGuQ8kuc9pgc9s8qqaq=dirpe0xb9q8qiLsFr0=vr0=vr0dc8meaabaqaciaacaGaaeqabaWaaeGaeaaakeaaimaacqWFoe=taaa@383D@(ℳ′ MathType@MTEF@5@5@+=feaafiart1ev1aaatCvAUfeBSjuyZL2yd9gzLbvyNv2CaerbwvMCKfMBHbqedmvETj2BSbWenfgDOvwBHrxAJfwnHbqeg0uy0HwzTfgDPnwy1aqee0evGueE0jxyaibaieIgFLIOYR2NHOxjYhrPYhrPYpI8F4rqqrFfpeea0xe9Lq=Jc9vqaqpepm0xbbG8FasPYRqj0=yi0lXdbba9pGe9qqFf0dXdHuk9fr=xfr=xfrpiWZqaaeaabiGaaiaacaqabeaadaqacqaaaOqaaGWaaiqb=ntinzaafaaaaa@406D@) be the occurrence set of ℳ′ MathType@MTEF@5@5@+=feaafiart1ev1aaatCvAUfeBSjuyZL2yd9gzLbvyNv2CaerbwvMCKfMBHbqedmvETj2BSbWenfgDOvwBHrxAJfwnHbqeg0uy0HwzTfgDPnwy1aqee0evGueE0jxyaibaieIgFLIOYR2NHOxjYhrPYhrPYpI8F4rqqrFfpeea0xe9Lq=Jc9vqaqpepm0xbbG8FasPYRqj0=yi0lXdbba9pGe9qqFf0dXdHuk9fr=xfr=xfrpiWZqaaeaabiGaaiaacaqabeaadaqacqaaaOqaaGWaaiqb=ntinzaafaaaaa@406D@. For each occurrence of ℳ′ MathType@MTEF@5@5@+=feaafiart1ev1aaatCvAUfeBSjuyZL2yd9gzLbvyNv2CaerbwvMCKfMBHbqedmvETj2BSbWenfgDOvwBHrxAJfwnHbqeg0uy0HwzTfgDPnwy1aqee0evGueE0jxyaibaieIgFLIOYR2NHOxjYhrPYhrPYpI8F4rqqrFfpeea0xe9Lq=Jc9vqaqpepm0xbbG8FasPYRqj0=yi0lXdbba9pGe9qqFf0dXdHuk9fr=xfr=xfrpiWZqaaeaabiGaaiaacaqabeaadaqacqaaaOqaaGWaaiqb=ntinzaafaaaaa@406D@ in O MathType@MTEF@5@5@+=feaafiart1ev1aaatCvAUfKttLearuWrP9MDH5MBPbIqV92AaeXatLxBI9gBamrtHrhAL1wy0L2yHvtyaeHbnfgDOvwBHrxAJfwnaebbnrfifHhDYfgasaacH8akY=wiFfYdH8Gipec8Eeeu0xXdbba9frFj0=OqFfea0dXdd9vqai=hGuQ8kuc9pgc9s8qqaq=dirpe0xb9q8qiLsFr0=vr0=vr0dc8meaabaqaciaacaGaaeqabaWaaeGaeaaakeaaimaacqWFoe=taaa@383D@(ℳ′ MathType@MTEF@5@5@+=feaafiart1ev1aaatCvAUfeBSjuyZL2yd9gzLbvyNv2CaerbwvMCKfMBHbqedmvETj2BSbWenfgDOvwBHrxAJfwnHbqeg0uy0HwzTfgDPnwy1aqee0evGueE0jxyaibaieIgFLIOYR2NHOxjYhrPYhrPYpI8F4rqqrFfpeea0xe9Lq=Jc9vqaqpepm0xbbG8FasPYRqj0=yi0lXdbba9pGe9qqFf0dXdHuk9fr=xfr=xfrpiWZqaaeaabiGaaiaacaqabeaadaqacqaaaOqaaGWaaiqb=ntinzaafaaaaa@406D@), we can extend it to get occurrences of ℳ MathType@MTEF@5@5@+=feaafiart1ev1aaatCvAUfKttLearuWrP9MDH5MBPbIqV92AaeXatLxBI9gBamrtHrhAL1wy0L2yHvtyaeHbnfgDOvwBHrxAJfwnaebbnrfifHhDYfgasaacH8akY=wiFfYdH8Gipec8Eeeu0xXdbba9frFj0=OqFfea0dXdd9vqai=hGuQ8kuc9pgc9s8qqaq=dirpe0xb9q8qiLsFr0=vr0=vr0dc8meaabaqaciaacaGaaeqabaWaaeGaeaaakeaaimaacqWFZestaaa@3790@ in O MathType@MTEF@5@5@+=feaafiart1ev1aaatCvAUfKttLearuWrP9MDH5MBPbIqV92AaeXatLxBI9gBamrtHrhAL1wy0L2yHvtyaeHbnfgDOvwBHrxAJfwnaebbnrfifHhDYfgasaacH8akY=wiFfYdH8Gipec8Eeeu0xXdbba9frFj0=OqFfea0dXdd9vqai=hGuQ8kuc9pgc9s8qqaq=dirpe0xb9q8qiLsFr0=vr0=vr0dc8meaabaqaciaacaGaaeqabaWaaeGaeaaakeaaimaacqWFoe=taaa@383D@(ℳ MathType@MTEF@5@5@+=feaafiart1ev1aaatCvAUfKttLearuWrP9MDH5MBPbIqV92AaeXatLxBI9gBamrtHrhAL1wy0L2yHvtyaeHbnfgDOvwBHrxAJfwnaebbnrfifHhDYfgasaacH8akY=wiFfYdH8Gipec8Eeeu0xXdbba9frFj0=OqFfea0dXdd9vqai=hGuQ8kuc9pgc9s8qqaq=dirpe0xb9q8qiLsFr0=vr0=vr0dc8meaabaqaciaacaGaaeqabaWaaeGaeaaakeaaimaacqWFZestaaa@3790@) by adding M1 ... Mi-1 before ℳ′ MathType@MTEF@5@5@+=feaafiart1ev1aaatCvAUfeBSjuyZL2yd9gzLbvyNv2CaerbwvMCKfMBHbqedmvETj2BSbWenfgDOvwBHrxAJfwnHbqeg0uy0HwzTfgDPnwy1aqee0evGueE0jxyaibaieIgFLIOYR2NHOxjYhrPYhrPYpI8F4rqqrFfpeea0xe9Lq=Jc9vqaqpepm0xbbG8FasPYRqj0=yi0lXdbba9pGe9qqFf0dXdHuk9fr=xfr=xfrpiWZqaaeaabiGaaiaacaqabeaadaqacqaaaOqaaGWaaiqb=ntinzaafaaaaa@406D@. This leads to at most ∏m=1i−1Wm MathType@MTEF@5@5@+=feaafiart1ev1aaatCvAUfKttLearuWrP9MDH5MBPbIqV92AaeXatLxBI9gBaebbnrfifHhDYfgasaacH8akY=wiFfYdH8Gipec8Eeeu0xXdbba9frFj0=OqFfea0dXdd9vqai=hGuQ8kuc9pgc9s8qqaq=dirpe0xb9q8qiLsFr0=vr0=vr0dc8meaabaqaciaacaGaaeqabaqabeGadaaakeaadaqeWaqaaiabdEfaxnaaBaaaleaacqWGTbqBaeqaaaqaaiabd2gaTjabg2da9iabigdaXaqaaiabdMgaPjabgkHiTiabigdaXaqdcqGHpis1aaaa@37E9@ occurrences of ℳ MathType@MTEF@5@5@+=feaafiart1ev1aaatCvAUfKttLearuWrP9MDH5MBPbIqV92AaeXatLxBI9gBamrtHrhAL1wy0L2yHvtyaeHbnfgDOvwBHrxAJfwnaebbnrfifHhDYfgasaacH8akY=wiFfYdH8Gipec8Eeeu0xXdbba9frFj0=OqFfea0dXdd9vqai=hGuQ8kuc9pgc9s8qqaq=dirpe0xb9q8qiLsFr0=vr0=vr0dc8meaabaqaciaacaGaaeqabaWaaeGaeaaakeaaimaacqWFZestaaa@3790@ for any occurrence of ℳ′ MathType@MTEF@5@5@+=feaafiart1ev1aaatCvAUfeBSjuyZL2yd9gzLbvyNv2CaerbwvMCKfMBHbqedmvETj2BSbWenfgDOvwBHrxAJfwnHbqeg0uy0HwzTfgDPnwy1aqee0evGueE0jxyaibaieIgFLIOYR2NHOxjYhrPYhrPYpI8F4rqqrFfpeea0xe9Lq=Jc9vqaqpepm0xbbG8FasPYRqj0=yi0lXdbba9pGe9qqFf0dXdHuk9fr=xfr=xfrpiWZqaaeaabiGaaiaacaqabeaadaqacqaaaOqaaGWaaiqb=ntinzaafaaaaa@406D@. Thus |O(ℳ′)|⋅∏m=1i−1Wm≥|O(ℳ)| MathType@MTEF@5@5@+=feaafiart1ev1aaatCvAUfKttLearuWrP9MDH5MBPbIqV92AaeXatLxBI9gBamrtHrhAL1wy0L2yHvtyaeHbnfgDOvwBHrxAJfwnaebbnrfifHhDYfgasaacH8akY=wiFfYdH8Gipec8Eeeu0xXdbba9frFj0=OqFfea0dXdd9vqai=hGuQ8kuc9pgc9s8qqaq=dirpe0xb9q8qiLsFr0=vr0=vr0dc8meaabaqaciaacaGaaeqabaWaaeGaeaaakeaadaabdaqaaGWaaiab=5q8pjabcIcaOiqb=ntinzaafaGaeiykaKcacaGLhWUaayjcSdGaeyyXIC9aaebmaeaacqWGxbWvdaWgaaWcbaGaemyBa0gabeaaaeaacqWGTbqBcqGH9aqpcqaIXaqmaeaacqWGPbqAcqGHsislcqaIXaqma0Gaey4dIunakiabgwMiZoaaemaabaGae8NdX=KaeiikaGIae83mH0KaeiykaKcacaGLhWUaayjcSdaaaa@5567@, which immediately gives πw(ℳ′)≥πw(ℳ)∏m=1i−1Wm MathType@MTEF@5@5@+=feaafiart1ev1aaatCvAUfKttLearuWrP9MDH5MBPbIqV92AaeXatLxBI9gBamrtHrhAL1wy0L2yHvtyaeHbnfgDOvwBHrxAJfwnaebbnrfifHhDYfgasaacH8akY=wiFfYdH8Gipec8Eeeu0xXdbba9frFj0=OqFfea0dXdd9vqai=hGuQ8kuc9pgc9s8qqaq=dirpe0xb9q8qiLsFr0=vr0=vr0dc8meaabaqaciaacaGaaeqabaWaaeGaeaaakeaaiiGacqWFapaCdaWgaaWcbaGaem4DaChabeaakiabcIcaOGWaaiqb+ntinzaafaGaeiykaKIaeyyzIm7aaSaaaeaacqWFapaCdaWgaaWcbaGaem4DaChabeaakiabcIcaOiab+ntinjabcMcaPaqaamaaradabaGaem4vaC1aaSbaaSqaaiabd2gaTbqabaaabaGaemyBa0Maeyypa0JaeGymaedabaGaemyAaKMaeyOeI0IaeGymaedaniabg+Givdaaaaaa@500D@. □\nWith Theorem 1, EXMOTIF can calculate a support bound for any suffix ℳ′ MathType@MTEF@5@5@+=feaafiart1ev1aaatCvAUfeBSjuyZL2yd9gzLbvyNv2CaerbwvMCKfMBHbqedmvETj2BSbWenfgDOvwBHrxAJfwnHbqeg0uy0HwzTfgDPnwy1aqee0evGueE0jxyaibaieIgFLIOYR2NHOxjYhrPYhrPYpI8F4rqqrFfpeea0xe9Lq=Jc9vqaqpepm0xbbG8FasPYRqj0=yi0lXdbba9pGe9qqFf0dXdHuk9fr=xfr=xfrpiWZqaaeaabiGaaiaacaqabeaadaqacqaaaOqaaGWaaiqb=ntinzaafaaaaa@406D@ of ℳ MathType@MTEF@5@5@+=feaafiart1ev1aaatCvAUfKttLearuWrP9MDH5MBPbIqV92AaeXatLxBI9gBamrtHrhAL1wy0L2yHvtyaeHbnfgDOvwBHrxAJfwnaebbnrfifHhDYfgasaacH8akY=wiFfYdH8Gipec8Eeeu0xXdbba9frFj0=OqFfea0dXdd9vqai=hGuQ8kuc9pgc9s8qqaq=dirpe0xb9q8qiLsFr0=vr0=vr0dc8meaabaqaciaacaGaaeqabaWaaeGaeaaakeaaimaacqWFZestaaa@3790@, given the quorum requirement q. For example, assume that the motif template is NN[3,5]NNN[0,4]NNN and q = 100, with W1 = 5 - 3 + 1 = 3 and W2 = 4 - 0 + 1 = 5. When processing the suffix component ℳ′ MathType@MTEF@5@5@+=feaafiart1ev1aaatCvAUfeBSjuyZL2yd9gzLbvyNv2CaerbwvMCKfMBHbqedmvETj2BSbWenfgDOvwBHrxAJfwnHbqeg0uy0HwzTfgDPnwy1aqee0evGueE0jxyaibaieIgFLIOYR2NHOxjYhrPYhrPYpI8F4rqqrFfpeea0xe9Lq=Jc9vqaqpepm0xbbG8FasPYRqj0=yi0lXdbba9pGe9qqFf0dXdHuk9fr=xfr=xfrpiWZqaaeaabiGaaiaacaqabeaadaqacqaaaOqaaGWaaiqb=ntinzaafaaaaa@406D@ = NNN, we require that πw(ℳ′ MathType@MTEF@5@5@+=feaafiart1ev1aaatCvAUfeBSjuyZL2yd9gzLbvyNv2CaerbwvMCKfMBHbqedmvETj2BSbWenfgDOvwBHrxAJfwnHbqeg0uy0HwzTfgDPnwy1aqee0evGueE0jxyaibaieIgFLIOYR2NHOxjYhrPYhrPYpI8F4rqqrFfpeea0xe9Lq=Jc9vqaqpepm0xbbG8FasPYRqj0=yi0lXdbba9pGe9qqFf0dXdHuk9fr=xfr=xfrpiWZqaaeaabiGaaiaacaqabeaadaqacqaaaOqaaGWaaiqb=ntinzaafaaaaa@406D@) ≥ 1003×5 MathType@MTEF@5@5@+=feaafiart1ev1aaatCvAUfKttLearuWrP9MDH5MBPbIqV92AaeXatLxBI9gBaebbnrfifHhDYfgasaacH8akY=wiFfYdH8Gipec8Eeeu0xXdbba9frFj0=OqFfea0dXdd9vqai=hGuQ8kuc9pgc9s8qqaq=dirpe0xb9q8qiLsFr0=vr0=vr0dc8meaabaqaciaacaGaaeqabaqabeGadaaakeaadaWcaaqaaiabigdaXiabicdaWiabicdaWaqaaiabiodaZiabgEna0kabiwda1aaaaaa@338B@ = 6; when processing ℳ′ MathType@MTEF@5@5@+=feaafiart1ev1aaatCvAUfeBSjuyZL2yd9gzLbvyNv2CaerbwvMCKfMBHbqedmvETj2BSbWenfgDOvwBHrxAJfwnHbqeg0uy0HwzTfgDPnwy1aqee0evGueE0jxyaibaieIgFLIOYR2NHOxjYhrPYhrPYpI8F4rqqrFfpeea0xe9Lq=Jc9vqaqpepm0xbbG8FasPYRqj0=yi0lXdbba9pGe9qqFf0dXdHuk9fr=xfr=xfrpiWZqaaeaabiGaaiaacaqabeaadaqacqaaaOqaaGWaaiqb=ntinzaafaaaaa@406D@ = NNN[0,4]NNN, we require that πw(ℳ′ MathType@MTEF@5@5@+=feaafiart1ev1aaatCvAUfeBSjuyZL2yd9gzLbvyNv2CaerbwvMCKfMBHbqedmvETj2BSbWenfgDOvwBHrxAJfwnHbqeg0uy0HwzTfgDPnwy1aqee0evGueE0jxyaibaieIgFLIOYR2NHOxjYhrPYhrPYpI8F4rqqrFfpeea0xe9Lq=Jc9vqaqpepm0xbbG8FasPYRqj0=yi0lXdbba9pGe9qqFf0dXdHuk9fr=xfr=xfrpiWZqaaeaabiGaaiaacaqabeaadaqacqaaaOqaaGWaaiqb=ntinzaafaaaaa@406D@) ≥ 1003 MathType@MTEF@5@5@+=feaafiart1ev1aaatCvAUfKttLearuWrP9MDH5MBPbIqV92AaeXatLxBI9gBaebbnrfifHhDYfgasaacH8akY=wiFfYdH8Gipec8Eeeu0xXdbba9frFj0=OqFfea0dXdd9vqai=hGuQ8kuc9pgc9s8qqaq=dirpe0xb9q8qiLsFr0=vr0=vr0dc8meaabaqaciaacaGaaeqabaqabeGadaaakeaadaWcaaqaaiabigdaXiabicdaWiabicdaWaqaaiabiodaZaaaaaa@307C@ = 33. Thus even the weaker bounds can lead to some pruning.\n\nThe complete EXMOTIF algorithm: complexity analysis\nThe pseudo-code for the complete EXMOTIF algorithm is shown in Figure 6. The program takes as inputs the set of sequences S={Si}i=1n MathType@MTEF@5@5@+=feaafiart1ev1aaatCvAUfKttLearuWrP9MDH5MBPbIqV92AaeXatLxBI9gBamrtHrhAL1wy0L2yHvtyaeHbnfgDOvwBHrxAJfwnaebbnrfifHhDYfgasaacH8akY=wiFfYdH8Gipec8Eeeu0xXdbba9frFj0=OqFfea0dXdd9vqai=hGuQ8kuc9pgc9s8qqaq=dirpe0xb9q8qiLsFr0=vr0=vr0dc8meaabaqaciaacaGaaeqabaWaaeGaeaaakeaaimaacqWFse=ucqGH9aqpcqGG7bWEcqWGtbWudaWgaaWcbaGaemyAaKgabeaakiabc2ha9naaDaaaleaacqWGPbqAcqGH9aqpcqaIXaqmaeaacqWGUbGBaaaaaa@43EE@, the motif template T MathType@MTEF@5@5@+=feaafiart1ev1aaatCvAUfKttLearuWrP9MDH5MBPbIqV92AaeXatLxBI9gBamrtHrhAL1wy0L2yHvtyaeHbnfgDOvwBHrxAJfwnaebbnrfifHhDYfgasaacH8akY=wiFfYdH8Gipec8Eeeu0xXdbba9frFj0=OqFfea0dXdd9vqai=hGuQ8kuc9pgc9s8qqaq=dirpe0xb9q8qiLsFr0=vr0=vr0dc8meaabaqaciaacaGaaeqabaWaaeGaeaaakeaaimaacqWFtepvaaa@3847@ = M1[l1, u1] ... [lk-1, uk-1] Mk, the quorum threshold q, the number of errors or IUPAC symbols allowed per simple motif e={ei}i=1k MathType@MTEF@5@5@+=feaafiart1ev1aaatCvAUfKttLearuWrP9MDH5MBPbIqV92AaeXatLxBI9gBaebbnrfifHhDYfgasaacH8akY=wiFfYdH8Gipec8Eeeu0xXdbba9frFj0=OqFfea0dXdd9vqai=hGuQ8kuc9pgc9s8qqaq=dirpe0xb9q8qiLsFr0=vr0=vr0dc8meaabaqaciaacaGaaeqabaqabeGadaaakeaaieqacqWFLbqzcqGH9aqpcqGG7bWEcqWGLbqzdaWgaaWcbaGaemyAaKgabeaakiabc2ha9naaDaaaleaacqWGPbqAcqGH9aqpcqaIXaqmaeaacqWGRbWAaaaaaa@39CC@, and the set of IUPAC symbols to use per simple motif, v={vi}i=1k MathType@MTEF@5@5@+=feaafiart1ev1aaatCvAUfKttLearuWrP9MDH5MBPbIqV92AaeXatLxBI9gBaebbnrfifHhDYfgasaacH8akY=wiFfYdH8Gipec8Eeeu0xXdbba9frFj0=OqFfea0dXdd9vqai=hGuQ8kuc9pgc9s8qqaq=dirpe0xb9q8qiLsFr0=vr0=vr0dc8meaabaqaciaacaGaaeqabaqabeGadaaakeaaieqacqWF2bGDcqGH9aqpcqGG7bWEcqWG2bGDdaWgaaWcbaGaemyAaKgabeaakiabc2ha9naaDaaaleaacqWGPbqAcqGH9aqpcqaIXaqmaeaacqWGRbWAaaaaaa@3A10@ (only for position specific substitutions). As outlined in Figure 6 EXMOTIF allows several different variations to motif extraction, as described above. These variations include, exact matching, position-specific substitutions via use of IUPAC symbols, arbitrary substitutions, and repeated motif identification.\nFigure 6 EXMOTIF Algorithm. EXMOTIF initially adjusts the support thresholds if the task is repeated motif identification (lines 1–2). The main approach for handling exact matches or position-specific substitutions is the same. The main difference is that while enumerating the simple motifs, EXMOTIF uses the appropriate IUPAC alphabet (specified by vi for component Mi; lines 6–7). The structured motifs are found via positional joins over the simple motifs (line 8). The positional joins are performed as described in Figure 1. For arbitrary substitutions, EXMOTIF first enumerates the simple motifs (line 9) and checks their aggregate support (i.e., including the supports of all neighbors within error εi). From these, the structured motifs are enumerated and stored in a hash-table (ℍ; line 11). Lastly, the aggregate support of all these motifs is computed as described in Figure 5 (line 12). Those that meet the quorum will be output. Finally, if desired, EXMOTIF recovers the full positions for each occurrence, via the procedure outlined in Figure 2.\nIn terms of the computational complexity of EXMOTIF, let's first consider the complexity of extracting the simple motifs. Assume that m is the length of the longest simple motif component in the structured template T MathType@MTEF@5@5@+=feaafiart1ev1aaatCvAUfKttLearuWrP9MDH5MBPbIqV92AaeXatLxBI9gBamrtHrhAL1wy0L2yHvtyaeHbnfgDOvwBHrxAJfwnaebbnrfifHhDYfgasaacH8akY=wiFfYdH8Gipec8Eeeu0xXdbba9frFj0=OqFfea0dXdd9vqai=hGuQ8kuc9pgc9s8qqaq=dirpe0xb9q8qiLsFr0=vr0=vr0dc8meaabaqaciaacaGaaeqabaWaaeGaeaaakeaaimaacqWFtepvaaa@3847@. Note that there are potentially |Σ|m frequent simple motifs at that length, but due to the quorum requirement, many of these will not be frequent. Nevertheless, in the worst case O(|Σ|m) simple components may be extracted. For a simple motif of length m, EXMOTIF uses O(log(m)) positional joins to obtain its support, and each such join takes O(N) time, where N=∑i=1n|Si| MathType@MTEF@5@5@+=feaafiart1ev1aaatCvAUfKttLearuWrP9MDH5MBPbIqV92AaeXatLxBI9gBaebbnrfifHhDYfgasaacH8akY=wiFfYdH8Gipec8Eeeu0xXdbba9frFj0=OqFfea0dXdd9vqai=hGuQ8kuc9pgc9s8qqaq=dirpe0xb9q8qiLsFr0=vr0=vr0dc8meaabaqaciaacaGaaeqabaqabeGadaaakeaacqWGobGtcqGH9aqpdaaeWaqaamaaemaabaGaem4uam1aaSbaaSqaaiabdMgaPbqabaaakiaawEa7caGLiWoaaSqaaiabdMgaPjabg2da9iabigdaXaqaaiabd6gaUbqdcqGHris5aaaa@3B71@ is the sum of the lengths of all the sequences Si in the database S MathType@MTEF@5@5@+=feaafiart1ev1aaatCvAUfKttLearuWrP9MDH5MBPbIqV92AaeXatLxBI9gBamrtHrhAL1wy0L2yHvtyaeHbnfgDOvwBHrxAJfwnaebbnrfifHhDYfgasaacH8akY=wiFfYdH8Gipec8Eeeu0xXdbba9frFj0=OqFfea0dXdd9vqai=hGuQ8kuc9pgc9s8qqaq=dirpe0xb9q8qiLsFr0=vr0=vr0dc8meaabaqaciaacaGaaeqabaWaaeGaeaaakeaaimaacqWFse=uaaa@3845@. Thus, extracting the simple motifs takes time O(N log(m)|Σ|m) in the worst case.\nWith |Σ|m simple motifs, there are O(|Σ|mk) potential structured motifs, though a vast majority of these will not meet the quorum requirement. Extracting the structured motifs then takes time O(kN|Σ|mk) for the exact match and position-specific substitution cases. For arbitrary substitutions there is additional cost of enumerating aggregate neighbors and computing their support. For each motif we have to consider ∏i=1k(|Mi|εi) MathType@MTEF@5@5@+=feaafiart1ev1aaatCvAUfKttLearuWrP9MDH5MBPbIqV92AaeXatLxBI9gBaebbnrfifHhDYfgasaacH8akY=wiFfYdH8Gipec8Eeeu0xXdbba9frFj0=OqFfea0dXdd9vqai=hGuQ8kuc9pgc9s8qqaq=dirpe0xb9q8qiLsFr0=vr0=vr0dc8meaabaqaciaacaGaaeqabaqabeGadaaakeaadaqeWaqaamaabmaabaqbaeqabiqaaaqaamaaemaabaGaemyta00aaSbaaSqaaiabdMgaPbqabaaakiaawEa7caGLiWoaaeaaiiGacqWF1oqzdaWgaaWcbaGaemyAaKgabeaaaaaakiaawIcacaGLPaaaaSqaaiabdMgaPjabg2da9iabigdaXaqaaiabdUgaRbqdcqGHpis1aaaa@3DF8@ = kme aggregate neighbors, where e = maxi{ei}. Furthermore, an aggregate neighbor can have k|Σ|e matching motifs. Thus the time complexity of extracting all the structured motifs is O(kN|Σ|mk + k2me|Σ|e) for arbitrary substitutions. Since typically mk \u003e e and N \u003e me, the time complexity is essentially O(kN|Σ|mk). Combined with the cost for simple motif extraction, the computational complexity of EXMOTIF is then given as O(log(m) N |Σ|m + kN|Σ|km) = O(kN|Σ|km).","divisions":[{"label":"title","span":{"begin":0,"end":21}},{"label":"p","span":{"begin":22,"end":173}},{"label":"sec","span":{"begin":175,"end":33868}},{"label":"title","span":{"begin":175,"end":193}},{"label":"p","span":{"begin":194,"end":4302}},{"label":"table-wrap","span":{"begin":4303,"end":4656}},{"label":"label","span":{"begin":4303,"end":4310}},{"label":"caption","span":{"begin":4312,"end":4322}},{"label":"p","span":{"begin":4312,"end":4322}},{"label":"table","span":{"begin":4323,"end":4582}},{"label":"tr","span":{"begin":4323,"end":4336}},{"label":"td","span":{"begin":4323,"end":4324}},{"label":"td","span":{"begin":4326,"end":4336}},{"label":"tr","span":{"begin":4337,"end":4404}},{"label":"td","span":{"begin":4337,"end":4338}},{"label":"td","span":{"begin":4340,"end":4404}},{"label":"tr","span":{"begin":4405,"end":4472}},{"label":"td","span":{"begin":4405,"end":4406}},{"label":"td","span":{"begin":4408,"end":4472}},{"label":"tr","span":{"begin":4473,"end":4516}},{"label":"td","span":{"begin":4473,"end":4474}},{"label":"td","span":{"begin":4476,"end":4516}},{"label":"tr","span":{"begin":4517,"end":4582}},{"label":"td","span":{"begin":4517,"end":4518}},{"label":"td","span":{"begin":4520,"end":4582}},{"label":"table-wrap-foot","span":{"begin":4583,"end":4656}},{"label":"p","span":{"begin":4583,"end":4656}},{"label":"sec","span":{"begin":4658,"end":14624}},{"label":"title","span":{"begin":4658,"end":4674}},{"label":"p","span":{"begin":4675,"end":6922}},{"label":"p","span":{"begin":6923,"end":7008}},{"label":"p","span":{"begin":7009,"end":7060}},{"label":"p","span":{"begin":7061,"end":7113}},{"label":"p","span":{"begin":7114,"end":7221}},{"label":"p","span":{"begin":7222,"end":10377}},{"label":"figure","span":{"begin":10378,"end":10415}},{"label":"label","span":{"begin":10378,"end":10386}},{"label":"caption","span":{"begin":10388,"end":10415}},{"label":"p","span":{"begin":10388,"end":10415}},{"label":"p","span":{"begin":10416,"end":11334}},{"label":"p","span":{"begin":11335,"end":14624}},{"label":"sec","span":{"begin":14626,"end":20797}},{"label":"title","span":{"begin":14626,"end":14657}},{"label":"p","span":{"begin":14658,"end":15437}},{"label":"p","span":{"begin":15438,"end":19524}},{"label":"p","span":{"begin":19525,"end":20797}},{"label":"sec","span":{"begin":20799,"end":21789}},{"label":"title","span":{"begin":20799,"end":20834}},{"label":"p","span":{"begin":20835,"end":21789}},{"label":"sec","span":{"begin":21791,"end":32881}},{"label":"title","span":{"begin":21791,"end":21813}},{"label":"p","span":{"begin":21814,"end":22172}},{"label":"p","span":{"begin":22173,"end":27420}},{"label":"figure","span":{"begin":27421,"end":27472}},{"label":"label","span":{"begin":27421,"end":27429}},{"label":"caption","span":{"begin":27431,"end":27472}},{"label":"p","span":{"begin":27431,"end":27472}},{"label":"p","span":{"begin":27473,"end":32831}},{"label":"figure","span":{"begin":32832,"end":32881}},{"label":"label","span":{"begin":32832,"end":32840}},{"label":"caption","span":{"begin":32842,"end":32881}},{"label":"p","span":{"begin":32842,"end":32881}},{"label":"sec","span":{"begin":32883,"end":33868}},{"label":"title","span":{"begin":32883,"end":32914}},{"label":"p","span":{"begin":32915,"end":33868}},{"label":"sec","span":{"begin":33870,"end":69431}},{"label":"title","span":{"begin":33870,"end":33892}},{"label":"p","span":{"begin":33893,"end":34317}},{"label":"sec","span":{"begin":34319,"end":37861}},{"label":"title","span":{"begin":34319,"end":34350}},{"label":"p","span":{"begin":34351,"end":37645}},{"label":"table-wrap","span":{"begin":37646,"end":37861}},{"label":"label","span":{"begin":37646,"end":37653}},{"label":"caption","span":{"begin":37655,"end":37679}},{"label":"p","span":{"begin":37655,"end":37679}},{"label":"table","span":{"begin":37680,"end":37861}},{"label":"tr","span":{"begin":37680,"end":37698}},{"label":"td","span":{"begin":37680,"end":37686}},{"label":"td","span":{"begin":37688,"end":37689}},{"label":"td","span":{"begin":37691,"end":37692}},{"label":"td","span":{"begin":37694,"end":37695}},{"label":"td","span":{"begin":37697,"end":37698}},{"label":"tr","span":{"begin":37699,"end":37716}},{"label":"td","span":{"begin":37699,"end":37704}},{"label":"td","span":{"begin":37706,"end":37707}},{"label":"td","span":{"begin":37709,"end":37710}},{"label":"td","span":{"begin":37712,"end":37713}},{"label":"td","span":{"begin":37715,"end":37716}},{"label":"tr","span":{"begin":37717,"end":37735}},{"label":"td","span":{"begin":37717,"end":37723}},{"label":"td","span":{"begin":37725,"end":37726}},{"label":"td","span":{"begin":37728,"end":37729}},{"label":"td","span":{"begin":37731,"end":37732}},{"label":"td","span":{"begin":37734,"end":37735}},{"label":"tr","span":{"begin":37736,"end":37759}},{"label":"td","span":{"begin":37736,"end":37741}},{"label":"td","span":{"begin":37743,"end":37744}},{"label":"td","span":{"begin":37746,"end":37749}},{"label":"td","span":{"begin":37751,"end":37754}},{"label":"td","span":{"begin":37756,"end":37759}},{"label":"tr","span":{"begin":37760,"end":37778}},{"label":"td","span":{"begin":37760,"end":37766}},{"label":"td","span":{"begin":37768,"end":37769}},{"label":"td","span":{"begin":37771,"end":37772}},{"label":"td","span":{"begin":37774,"end":37775}},{"label":"td","span":{"begin":37777,"end":37778}},{"label":"tr","span":{"begin":37779,"end":37806}},{"label":"td","span":{"begin":37779,"end":37784}},{"label":"td","span":{"begin":37786,"end":37789}},{"label":"td","span":{"begin":37791,"end":37794}},{"label":"td","span":{"begin":37796,"end":37799}},{"label":"td","span":{"begin":37801,"end":37806}},{"label":"tr","span":{"begin":37807,"end":37825}},{"label":"td","span":{"begin":37807,"end":37813}},{"label":"td","span":{"begin":37815,"end":37816}},{"label":"td","span":{"begin":37818,"end":37819}},{"label":"td","span":{"begin":37821,"end":37822}},{"label":"td","span":{"begin":37824,"end":37825}},{"label":"tr","span":{"begin":37826,"end":37861}},{"label":"td","span":{"begin":37826,"end":37831}},{"label":"td","span":{"begin":37833,"end":37838}},{"label":"td","span":{"begin":37840,"end":37845}},{"label":"td","span":{"begin":37847,"end":37852}},{"label":"td","span":{"begin":37854,"end":37861}},{"label":"sec","span":{"begin":37863,"end":69431}},{"label":"title","span":{"begin":37863,"end":37886}},{"label":"p","span":{"begin":37887,"end":38849}},{"label":"p","span":{"begin":38850,"end":40120}},{"label":"sec","span":{"begin":40122,"end":54281}},{"label":"title","span":{"begin":40122,"end":40143}},{"label":"p","span":{"begin":40144,"end":46638}},{"label":"p","span":{"begin":46639,"end":49552}},{"label":"figure","span":{"begin":49553,"end":49583}},{"label":"label","span":{"begin":49553,"end":49561}},{"label":"caption","span":{"begin":49563,"end":49583}},{"label":"p","span":{"begin":49563,"end":49583}},{"label":"p","span":{"begin":49584,"end":54246}},{"label":"figure","span":{"begin":54247,"end":54281}},{"label":"label","span":{"begin":54247,"end":54255}},{"label":"caption","span":{"begin":54257,"end":54281}},{"label":"p","span":{"begin":54257,"end":54281}},{"label":"sec","span":{"begin":54283,"end":57872}},{"label":"title","span":{"begin":54283,"end":54299}},{"label":"p","span":{"begin":54300,"end":57872}},{"label":"sec","span":{"begin":57874,"end":69431}},{"label":"title","span":{"begin":57874,"end":57909}},{"label":"p","span":{"begin":57910,"end":59731}},{"label":"p","span":{"begin":59732,"end":60771}},{"label":"p","span":{"begin":60772,"end":62510}},{"label":"p","span":{"begin":62511,"end":63660}},{"label":"p","span":{"begin":63661,"end":66579}},{"label":"p","span":{"begin":66580,"end":67203}},{"label":"p","span":{"begin":67204,"end":69431}},{"label":"sec","span":{"begin":69433,"end":81750}},{"label":"title","span":{"begin":69433,"end":69489}},{"label":"p","span":{"begin":69490,"end":69962}},{"label":"p","span":{"begin":69963,"end":72288}},{"label":"p","span":{"begin":72289,"end":78766}},{"label":"p","span":{"begin":78767,"end":81750}},{"label":"title","span":{"begin":81752,"end":81803}},{"label":"p","span":{"begin":81804,"end":83918}},{"label":"figure","span":{"begin":83919,"end":83947}},{"label":"label","span":{"begin":83919,"end":83927}},{"label":"caption","span":{"begin":83929,"end":83947}},{"label":"p","span":{"begin":83929,"end":83947}},{"label":"p","span":{"begin":83948,"end":84980}},{"label":"p","span":{"begin":84981,"end":86711}}],"tracks":[]}