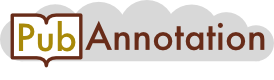
PMC:1635699 / 17547-22064
Annnotations
2_test
{"project":"2_test","denotations":[{"id":"17062125-15860779-1688908","span":{"begin":474,"end":476},"obj":"15860779"},{"id":"17062125-15860779-1688909","span":{"begin":711,"end":713},"obj":"15860779"},{"id":"17062125-16613908-1688910","span":{"begin":832,"end":834},"obj":"16613908"},{"id":"17062125-15661851-1688911","span":{"begin":1279,"end":1281},"obj":"15661851"},{"id":"17062125-15661851-1688912","span":{"begin":1491,"end":1493},"obj":"15661851"},{"id":"17062125-3118049-1688913","span":{"begin":2483,"end":2485},"obj":"3118049"},{"id":"17062125-16613908-1688914","span":{"begin":3392,"end":3394},"obj":"16613908"},{"id":"17062125-16690634-1688915","span":{"begin":3401,"end":3403},"obj":"16690634"},{"id":"17062125-15790387-1688916","span":{"begin":4000,"end":4001},"obj":"15790387"},{"id":"17062125-15657094-1688917","span":{"begin":4015,"end":4016},"obj":"15657094"},{"id":"17062125-15661851-1688918","span":{"begin":4255,"end":4257},"obj":"15661851"},{"id":"17062125-15034147-1688919","span":{"begin":4268,"end":4270},"obj":"15034147"},{"id":"17062125-15318951-1688920","span":{"begin":4271,"end":4273},"obj":"15318951"},{"id":"17062125-15687296-1688921","span":{"begin":4289,"end":4291},"obj":"15687296"},{"id":"17062125-16613908-1688922","span":{"begin":4301,"end":4303},"obj":"16613908"}],"text":"Conclusion\nWe have extended the previous \"Benchmark RNA Alignment dataBase\" BRAliBase II by a factor of 30 in terms of the alignment number and with respect to the range of sequences per alignment. With the new datasets of BRAliBase 2.1 we tested several sequence alignment programs. Obviously it is not possible to test all available programs; here we concentrated on well-known sequence alignment programs and those already identified as good aligners in our first study [22]. Additionally we showed that gap-parameters can be (easily) optimized and tested whether the incorporation of RNA-specific substitution matrices results in a performance change.\nFrom these tests, in comparison with the previous one [22], several conclusions can be drawn:\n• While testing the performance of several programs, as for example published in [36], with the k5 datasets of BRAliBase II and of BRAliBase 2.1, we found no statistically significant difference of results obtained by the use of these (data not shown); that is, there exists no bias due to the smaller alignment number and the restricted number of RNA families used in BRAliBase II.\n• Gap parameter optimization has previously been done only for protein alignment programs. The first BRAliBase benchmark enabled several authors [25] to optimize parameters of their programs for RNA alignments. For example the performance of the previously lowest ranking program MAFFT increased enormously: the new version 5 including optimized parameters [25] is now top ranking.\nThis result can be generalized: At least the gap costs are critical parameters especially in the low-homology range, but program's default parameters are in most cases not optimal for RNA (e. g. see Tables 2 and 3).\n• A further critical parameter set is the nucleotide substitution matrix. We compared the RIBOSUM 85–60 matrix with the default matrix of three programs (see Table 4). The performance of ALIGN-M and POA was either unchanged or improved; however, CLUSTALW performed worse with this RIBOSUM matrix.\n• The relative performance of iterative programs (e. g. MAFFT, MUSCLE, PRRN) improves with an increasing number of input sequences and/or decreasing sequence identity. The non-iterative, progressive programs show the opposite trend. With increasing number of sequences and decreasing sequence identity the progressive alignment approach is more likely to introduce errors, which cannot be corrected at a later alignment stage (\"once a gap, always a gap\" [37]). These errors are corrected by iterative programs during their refinement stage.\n• An APSI of 55 % seems to be a critical threshold where the performance boost of (i) iterative programs and of (ii) programs with optimized parameters becomes obvious.\n• Given the CPU and memory demand of structure (or sequence+structure) alignment programs, which is mostly above O MathType@MTEF@5@5@+=feaafiart1ev1aaatCvAUfKttLearuWrP9MDH5MBPbIqV92AaeXatLxBI9gBamrtHrhAL1wy0L2yHvtyaeHbnfgDOvwBHrxAJfwnaebbnrfifHhDYfgasaacH8akY=wiFfYdH8Gipec8Eeeu0xXdbba9frFj0=OqFfea0dXdd9vqai=hGuQ8kuc9pgc9s8qqaq=dirpe0xb9q8qiLsFr0=vr0=vr0dc8meaabaqaciaacaGaaeqabaWaaeGaeaaakeaaimaacqWFoe=taaa@383D@(n4) with sequence length n and two sequences, the use of BRAliBase 2.1 is too time consuming. Benchmarks with structure alignment programs are possible, however, with a restricted subset of BRAliBase 2.1 or with BRAliBase II (e. g. see [36] and [38]).\nBased upon these results we now provide recommendations to users on the current state of the art for aligning homologous sets of RNAs:\n1. Align the sequence set with a (fast) program of your choice.\n2. Check the sequence identity in the preliminary alignment:\n• if APSI ≥ 75 %, the preliminary alignment is already of high quality;\n• if 55 % \u003c APSI \u003c 75 %, realign with a good sequence alignment program; at present we recommend MAFFT (G-INS-i) (see Table 5);\n• if APSI ≤ 55 %, sequence alignment programs might not be sufficient; structure alignment programs might be of help (e. g. STEMLOC [5], FOLDALIGN [3], etc.), but be aware of memory and CPU usage.\nWe hope that the BRAliBase 2.1 reference alignments constitute a testing platform for developers, similarly as the BRAliBase II was already used for parameter optimization/training of MAFFT [25], MUSCLE [16,26], PROBCONSRNA [33], STRAL [36], and TLARA [39]. In the future we will try to provide a web interface, to which program authors may upload alignments created with their programs, that are than automatically scored and their performance plotted."}