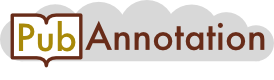
PMC:1630425 / 6841-9489
Annnotations
2_test
{"project":"2_test","denotations":[{"id":"17049089-11331237-1690127","span":{"begin":87,"end":88},"obj":"11331237"},{"id":"17049089-12611807-1690128","span":{"begin":89,"end":90},"obj":"12611807"},{"id":"17049089-11895567-1690129","span":{"begin":91,"end":93},"obj":"11895567"},{"id":"17049089-15501469-1690129","span":{"begin":91,"end":93},"obj":"15501469"},{"id":"17049089-14734312-1690129","span":{"begin":91,"end":93},"obj":"14734312"},{"id":"17049089-12537561-1690130","span":{"begin":505,"end":507},"obj":"12537561"},{"id":"17049089-14751999-1690130","span":{"begin":505,"end":507},"obj":"14751999"},{"id":"17049089-11895567-1690131","span":{"begin":876,"end":878},"obj":"11895567"},{"id":"17049089-8506142-1690132","span":{"begin":1213,"end":1214},"obj":"8506142"},{"id":"17049089-11331237-1690133","span":{"begin":1357,"end":1358},"obj":"11331237"},{"id":"17049089-15501469-1690134","span":{"begin":1621,"end":1623},"obj":"15501469"},{"id":"17049089-11895567-1690135","span":{"begin":1925,"end":1927},"obj":"11895567"}],"text":"Towards an accurate kernel density function\nAs shown in previous work discussed above [3,9,14-16], the fact that similar sequence distance is not equidistant (Euclidean) to the preceding position is a serious limitation to sequence comparison. On the other hand, it was also shown [17] that pursuing discriminant analysis using representations that are not constrained by predefined scales or succession orders, even when those scales are systematically screened such as in variable length Markov models [18-20], leads to more accurate models of sequences. The two results put together point to the need for a density kernel that resolves scale (succession order) such that predictive patterns can be investigated more efficiently in the iterative map representation.\nIn spite of the attractiveness of iterative functions in general, and the bidirectional USM implementation [14] in particular, for enabling the scale independent representation of motifs in biological sequences, its segmentation is still typically approached by considering quadrants that only correspond to Markovian transition. This usage indeed has no fundamental advantage over the better established use of fixed order transition matrices [2]. To go beyond that, the fractal nature [21] set by the consecutive scales that can be spanned by multi-order or fractal order segmentations [3,17] has to be accommodated by the density estimation procedure. As mentioned earlier, we have subsequently approached the investigation of the distributions of motifs of variable length using continuous kernels on the USM positions, such as the Gaussian kernel [15] with only partial success. The limitation of that approach, clearer in the investigation of local entropy, reflected the indetermination of sequence similarity between equidistant positions in the map, which had actually been anticipated, and mathematically modeled, by the original USM proposition [14]. In this report we solve the problem by identifying a kernel for density distribution in the USM space that matches the fractal succession of Markov transition orders. For ease of representation, the procedure will be illustrated for nucleotide sequences, which is also the scale for which unidirectional USM is equivalent to the CGR procedure. This achievement enables the computation of scale independent distribution of motifs in biological sequences which allows different scales to be combined in the same representation of density of motifs in the sequence. The critical advance is that it is no longer affected by the sequence composition itself or, which is the same thing, by the position in the iterative map."}