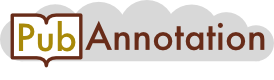
PMC:1570465 / 43194-44447
Annnotations
2_test
{"project":"2_test","denotations":[{"id":"16916460-8211139-1687092","span":{"begin":168,"end":170},"obj":"8211139"},{"id":"16916460-10743561-1687093","span":{"begin":171,"end":173},"obj":"10743561"},{"id":"16916460-8520488-1687094","span":{"begin":174,"end":176},"obj":"8520488"},{"id":"16916460-8211139-1687095","span":{"begin":287,"end":289},"obj":"8211139"},{"id":"16916460-8211139-1687096","span":{"begin":429,"end":431},"obj":"8211139"}],"text":"For each of the test protein datasets, our approach uncovers the optimal solution according to the SP-measure. These discovered motifs correspond to those reported by [15,36,43], and their SP-scores are highly significant, with e-values less than 10-15 for all of them. As described by [15], the HTH dataset is very diverse, and the detection of the motif is a difficult task. Nonetheless, our HTH motif is identical to that of [15], and agrees with the known annotations in every sequence. We likewise find the lipocalin motif; it is a weak motif with few generally conserved residues that is in perfect correspondence with the known lipocalin signature. We also precisely recover the immunoglobulin fold, TNF and zinc metallopeptidase motifs. The protein datasets demonstrate the strength of our graph pruning techniques. The five datasets are of varying difficulty to solve, with some employing the basic clique-bounds DEE technique to prune the graphs, while others requiring more elaborate pruning that is constrained by three-way alignments (see Table 1). In each case, the size of the reduced graph is at least an order of magnitude smaller. For three of the five datasets, the pruning procedures alone are able to identify the underlying motifs."}