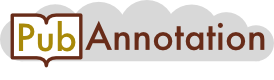
PMC:1540429 / 13202-20423
Annnotations
{"target":"https://pubannotation.org/docs/sourcedb/PMC/sourceid/1540429","sourcedb":"PMC","sourceid":"1540429","source_url":"https://www.ncbi.nlm.nih.gov/pmc/1540429","text":"2 Is this a hard dataset?\nAmong the questions arising from the assessment project, a particularly interesting one is this: what makes a particular dataset so hard to solve? The answer to this question would be helpful at both ends of the tools. For the users, it would save time and money if a certain assurance of the predictions is provided; for the designers, focus would be put upon factors that account for some for the poor performance of current methods.\nSome features of the datasets obviously show correlations with the tools' performance. For instance, a dataset of a large size intuitively is not easy to handle. But, when any feature is studied alone, its correlation with the performance of the tools is always too weak to be convincing, as the effects of all but this feature are ignored.\nWe applied multiple linear regression [8], a method of estimating the conditional expected value of one variable Y (the dependent variable) in terms of a set of other variables X (predictor variables). It is a special type of regression in which the dependent variable is a linear function of the \"missing data\" (regression coefficients W) in the model. A general form of multiple regression can be expressed as\nE(Y|X) = f(W, X) + ε\nwhere f is a linear function of W, a simple example of which is f(W, X) = W·X. ε is called regression residue. It has the expected value 0, and is independent of X (\"inequality of variance\").\nThe goodness-of-fit of regression is measured by the coefficient of determination R2. This is the proportion of the total variation in Y that can be explained or accounted for by the variation in the predictor variables {X}. The higher the value of R2, the better the model fits the data. Often R2 is adjusted for the bias brought by the degree of freedom of the model and the limited number of observations as = R2- p × , where n is the number of observations, and p is the number of predictors.\nIn our application of multiple linear regression, Y is the performance of the tools for a dataset, which is measured by the highest nucleotide-level correlation coefficient score nCC (see [9]) among all the tools. The reason for using the highest score is to smooth the disadvantages of each individual tool. The predictor variables are a set of features of a dataset which we think may be possible factors. These features include:\n1. the total size of a dataset;\n2. the median length of a single sequence in a dataset;\n3. the number of binding sites in a dataset;\n4. the density of the binding sites, which equals the number of binding sites divided by the total size of a dataset;\n5. the fraction of null sequences (ones that do not contain a binding site) in a dataset;\n6. relative entropy of binding sites in a dataset;\n7. the relative entropy-density in a dataset, which is the overall relative entropy times the density of the binding sites;\n8. the uniformity of the binding site locations within the sequences in a dataset. We quantified this position distribution information by performing a Kolmogorov-Smirnov test [10] against a uniform distribution and calculating its p-value.\nWe used least square fitting to calculate the regression coefficients. The most common forms of it include least square fitting of lines and least square fitting of polynomials. In the former, only the first-order term of the predictor variables are involved in the regression model; in the latter, higher order polynomial terms of them are also used. Due to a limited number of observations available (the number of \"Generic\" and \"Markov\" datasets in the analysis is about thirty) compared to the number of features, we confined ourselves to the simplest form of linear regression: only the first-order terms are used in the fitting. As we will discuss below, this simplification does not affect the regression result much.\nSome features are obviously not independent. For example, relative entropy-density is the non-linear operation (multiplication) of two other X variables, relative entropy and density. For every set of features that are highly correlated to each other, we replaced it by its subset with the highest adjusted correlation coefficient .\nThen the best subset of features is chosen to maximize the multiple linear regression output. The set of features that shows the most correlation to the performance consists of the relative entropy of the binding site alignment, the position distribution of the binding sites in the sequences, and the length of the single sequence in the dataset. The result is exhibited in Figure 5(a). The adjusted coefficient of determination is about 68%, with a p-value less than 0.001. The regression residues versus the estimated response (Figure 5(b)) doesn't indicate evident inequality of variance, which is an important assumption of linear regression the requires that regression residues are independent of the expected value of Y.\nFigure 5 Multiple linear regression result. (a)The best-fit line. Marks on the x-axis index the datasets, which are arrayed so that the estimated values of the dependent variable (the assessment scores) are in a straight line. For each dataset, the red dot is the assessment score, measured by the best correlation coefficient score nCC (see [9]) among all the tools, and the circle on the blue line shows the estimated value of the best-fit linear model. (b)Residues of the regression versus estimated nCC score. The x-axis is the estimated value of the dependent variable, the y-axis is the corresponding residue. This plot shows little indication of inequality of variance, which is an important assumption of linear regression. We then ran a least square fitting of second-order polynomials on these three features in the regression. The higher order form merely improves the regression result to ~70%. No second-order term has a significant coefficient in the model. Thus, although the simple linear regression model is learned through a greedy approach, we expect it's stable enough to indicate the importance of these three features in controlling the performance.\nWe also tried the transformations of the power family on the dependent variable Y using the Box-Cox method [11]. A lambda value other than 1 improves the to about 90%. The three features mentioned above again show significance in the model. But some other features – the fraction of null sequences and density particularly – which are skipped in the first model show impact here. This confirms that the three features are likely important for affecting the performance, but we can't rule out other features.\nIt's no surprise that the sequence conservation (relative entropy) is key to the hardness of a dataset. It turns out that tools are actually quite robust with respect to the size of the dataset in a large range (up to 10,000 bp). Rather, the length of each single sequence has a bigger impact. This is somewhat supported by our discussion of the objective functions that sequences in a dataset should be considered as individuals. Also, it is connected to the position distribution information, as the longer each single sequence is, the more significant it becomes that the binding sites are not uniformly distributed in the sequences.","divisions":[{"label":"title","span":{"begin":0,"end":25}},{"label":"p","span":{"begin":26,"end":461}},{"label":"p","span":{"begin":462,"end":802}},{"label":"p","span":{"begin":803,"end":1214}},{"label":"p","span":{"begin":1215,"end":1235}},{"label":"p","span":{"begin":1236,"end":1427}},{"label":"p","span":{"begin":1428,"end":1924}},{"label":"p","span":{"begin":1925,"end":2356}},{"label":"p","span":{"begin":2357,"end":2388}},{"label":"p","span":{"begin":2389,"end":2444}},{"label":"p","span":{"begin":2445,"end":2489}},{"label":"p","span":{"begin":2490,"end":2607}},{"label":"p","span":{"begin":2608,"end":2697}},{"label":"p","span":{"begin":2698,"end":2748}},{"label":"p","span":{"begin":2749,"end":2872}},{"label":"p","span":{"begin":2873,"end":3113}},{"label":"p","span":{"begin":3114,"end":3838}},{"label":"p","span":{"begin":3839,"end":4171}},{"label":"p","span":{"begin":4172,"end":4901}},{"label":"figure","span":{"begin":4902,"end":5634}},{"label":"label","span":{"begin":4902,"end":4910}},{"label":"caption","span":{"begin":4912,"end":5634}},{"label":"p","span":{"begin":4912,"end":5634}},{"label":"p","span":{"begin":5635,"end":6075}},{"label":"p","span":{"begin":6076,"end":6584}}],"tracks":[{"project":"2_test","denotations":[{"id":"16722558-8786136-1694718","span":{"begin":2114,"end":2115},"obj":"8786136"}],"attributes":[{"subj":"16722558-8786136-1694718","pred":"source","obj":"2_test"}]}],"config":{"attribute types":[{"pred":"source","value type":"selection","values":[{"id":"2_test","color":"#ec93c1","default":true}]}]}}