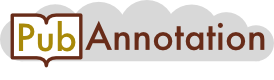
PMC:1540429 / 10239-13200
Annnotations
{"target":"https://pubannotation.org/docs/sourcedb/PMC/sourceid/1540429","sourcedb":"PMC","sourceid":"1540429","source_url":"https://www.ncbi.nlm.nih.gov/pmc/1540429","text":"Sequence specificity\nAnother type of objective function emphasizes the likelihood that most, if not all, sequences are potentially bound by the transcription factor. That means a prediction having multiple binding sites in one sequence and none in the others is much less significant than a prediction having a balanced number of binding sites in each sequence. This idea is designed into ANN-Spec [6] and Weeder [7]. The objective function, named sequence specificity, is defined in [7] as follows.\nwhere Ei(m|p0) is the expected number of motif m's occurrences in sequence i assuming the background model p0, and L is the total number of sequences in the dataset.\nWe calculated the scores of the predictions of Weeder and ANN-Spec and the planted motifs. The planted motif has a higher score than the predictions of the tools for most datasets, as illustrated in Figure 4. The obvious gap between the scores of planted binding sites and the predictions reflects a lack of optimum of the search strategies adopted by these tools. Recall that ANN-Spec is a generalized version of SEM (Stochastic EM), and Weeder uses a greedy and heuristic search method.\nFigure 4 Objective function: sequence specificity score. The figure shows the comparison between the sequence specificity scores of the planted motifs (named TFBS in the legend) and the predictions of Weeder and ANN-Spec. For the same reason as in Figure 1, only datasets of \"Generic\" and \"Markov\" types are tested. The x-axis tells the indices of the datasets. The datasets are sorted in ascending order of TFBS scores for clarity. For each dataset, there are three scores: the score of TFBS motif, Weeder's prediction, and ANN-Spec's prediction, colored in red, blue and green respectively. Points on the x-axis corresponds to the datasets for which the tool didn't make any prediction. Comparing Figure 4 with the other objective functions (Figure 1, 3), this result shows certain promise that using the sequence specificity score may often lead to the true binding sites. From objective function point of view solely, sequence specificity seems to have the edge for our datasets. An assumption of this objective function is that most sequences in the datasets should have binding sites of the motif. Although our data shows that tools such as Weeder and ANN-Spec are not too sensitive to the slight departure from this assumption, we have not tested them on datasets with more deviation. The Z-score function is based on the statistical over-representation solely without any reference to biological theories. The log likelihood ratio relies on high-quality non-gapped alignments, but it's not clear that non-gapped alignments are powerful enough to model the true binding sites. No objective function meets our standard that all planted motifs should have scores at least as high as those of the predictions. We need to understand better the conservation information hidden among those binding sites.","divisions":[{"label":"title","span":{"begin":0,"end":20}},{"label":"p","span":{"begin":21,"end":499}},{"label":"p","span":{"begin":500,"end":665}},{"label":"p","span":{"begin":666,"end":1154}},{"label":"figure","span":{"begin":1155,"end":1844}},{"label":"label","span":{"begin":1155,"end":1163}},{"label":"caption","span":{"begin":1165,"end":1844}},{"label":"p","span":{"begin":1165,"end":1844}}],"tracks":[{"project":"2_test","denotations":[{"id":"16722558-10902194-1694717","span":{"begin":399,"end":400},"obj":"10902194"}],"attributes":[{"subj":"16722558-10902194-1694717","pred":"source","obj":"2_test"}]}],"config":{"attribute types":[{"pred":"source","value type":"selection","values":[{"id":"2_test","color":"#93ecbc","default":true}]}]}}