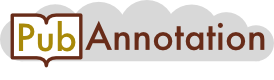
PMC:1524773 / 50180-55698
Annnotations
{"target":"https://pubannotation.org/docs/sourcedb/PMC/sourceid/1524773","sourcedb":"PMC","sourceid":"1524773","source_url":"https://www.ncbi.nlm.nih.gov/pmc/1524773","text":"Computing a partition of S from maximal cliques\nSince the membership of a sequence is almost never unambiguous, a heuristic is needed in order to partition the set of sequences into meaningful clusters. The set of all maximal cliques for a given constraint c guarantees us that every two members of a maximal clique will at least share a part of their sequence of length (|Ii| * c). Let Ic(Ci) denote that part of the query sequence that is overlapped by all sequences in clique Ci, i.e.,\nIc(Ci) = [max {xj|Ij ∈ Ci}, min {yj|Ij ∈ Ci}] (6)\nIt is an important observation that this common part of the sequence does not have to overlap each sequence in Ci by c* 100%, as is shown in Fig. 12. If now a sequence X is a member of many maximal cliques, it should be assigned to that clique Ci whose shared sequence part Ic(Ci) overlaps X best, i.e. is maximal.\nFigure 12 With a constraint of 0.6 intervals 1, 2, 3 build a clique. The common overlap of all 3 intervals is [4-10], which is only 3/7 of interval 3. The other problem is that the number and structure of maximal cliques is of course depending on the chosen constraint value c. There are two opposing situations that make it hard to find the best c-value: The first is sketched in Fig 13a) where three different clusters of sequences are visible, but they will only be found if c is larger than 0.6. In Fig. 13b) there is only one cluster, but if c is larger than 0.6 then two maximal cliques will emerge.\nFigure 13 a) There are three distinguishable clusters: {1, 2, 3}, {4, 5, 6}, {7, 8, 9} but as long as c is below 4/11 there is only one maximal clique embracing all intervals. Not until c is larger than 3/5, the wanted three sets will emerge as maximal cliques. b) Here, the situation is different. Intuitively, only one set embracing all intervals should emerge. But this homogenous set will be split into two maximal cliques when c is greater than 3/5: one contains interval 5 and one interval 6. Thus, we have two contradicting goals: To get a higher specificity of the maximal cliques it is good to use a large c-value. But if it is too large it will split 'good' cliques into too many parts. Our aim was to find a heuristic that is based on a c-value that is small enough as not to split 'good' clusters but can find subsets of the maximal cliques that are more specific than the whole clique.\nFor this, the following heuristic is used:\n1. Add all maximal cliques to a list L.\n2. For all cliques C in L compute the shared overlap interval IC, i.e., the maximal interval that is overlapped by all of the intervals in the clique.\n3. For every interval I that is in more than one clique, compute the overlap between IC and I and multiply this value with the number of intervals in this clique.\n4. Assign every interval I to that clique where the product of overlap with IC and number of intervals in that clique is maximal.\nThis basic variant yields good results but it can be improved by the following procedure: For every pair A, B of cliques compute the intersection A ⋂ B. If there are many intervals in this intersection set, e.g., |A ⋂ B| \u003e 3, and only a few intervals that are not, the intersection of the two cliques represents intervals that are very similar to each other. These intervals would be in one clique if the c-value would have been more restrictive, i.e., larger. But due to the low c-value, there are other intervals to the left and right of the intervals in the intersection set that interact with the intervals in A ⋂ B but not with each other. As discussed above, a low c-value has the advantage of keeping good clusters together, and thus c should not be too high in the beginning. However, the effect of a larger c-value can be mimicked by adding those intersection sets to the list L in the first step of the above described mechanism and to allow the intervals to assign them to any set in L be it a clique or an intersection set.\nThe given heuristic guarantees that every two intervals in a set tolerate each other, because it is built on the maximal cliques of tolerating intervals. The decision of membership has been built on a composite of the overlap with the shared sequence and the size of the set to reduce the number of sets globally. The size of a set is of course volatile because not all of its designated members will finally be assigned to it. But in each case the overlap with the shared sequence of any set will never decrease if another member is deleted from the set, so the heuristic is stable in this respect.\nWe have already indicated at the beginning of this article (s. Fig. 1), that many partitions of a set S of sequences seem to be reasonable by regarding only their begin and ends. In order to facilitate a manual revision of the calculated partition of each set of sequences these sets are displayed in the order of the diagonal of their smallest member, i.e., the sum of its x-position and its length.\nThe results of this heuristic applied to the three biological data sets are given in Fig. 14a–c. The colorization helps to identify the members of one clique. When comparing the cliques with the annotation of the input query sequence individual domains as well as combination of several domains were retrieved and corresponded almost perfectly to the annotated domains of the input protein sequence.\nFigure 14 The partitions found by the heuristic described in the text, for a constraint value of c = 0.65. a) Shankl, b) Celsr2, c) PpmaT. Members of the same clique are colored equally.","divisions":[{"label":"title","span":{"begin":0,"end":47}},{"label":"p","span":{"begin":48,"end":488}},{"label":"p","span":{"begin":489,"end":544}},{"label":"p","span":{"begin":545,"end":859}},{"label":"figure","span":{"begin":860,"end":1011}},{"label":"label","span":{"begin":860,"end":869}},{"label":"caption","span":{"begin":871,"end":1011}},{"label":"p","span":{"begin":871,"end":1011}},{"label":"p","span":{"begin":1012,"end":1466}},{"label":"figure","span":{"begin":1467,"end":1966}},{"label":"label","span":{"begin":1467,"end":1476}},{"label":"caption","span":{"begin":1478,"end":1966}},{"label":"p","span":{"begin":1478,"end":1966}},{"label":"p","span":{"begin":1967,"end":2366}},{"label":"p","span":{"begin":2367,"end":2409}},{"label":"p","span":{"begin":2410,"end":2449}},{"label":"p","span":{"begin":2450,"end":2600}},{"label":"p","span":{"begin":2601,"end":2763}},{"label":"p","span":{"begin":2764,"end":2893}},{"label":"p","span":{"begin":2894,"end":3929}},{"label":"p","span":{"begin":3930,"end":4529}},{"label":"p","span":{"begin":4530,"end":4930}},{"label":"p","span":{"begin":4931,"end":5330}},{"label":"label","span":{"begin":5331,"end":5340}}],"tracks":[]}